Systems biology and precision nutrition
Precision nutrition seeks to address the challenge that not all dietary interventions prove beneficial for every individual. Essentially the ‘one healthy diet benefits all’ paradigm is insufficient. Thus precision (or personalised) nutrition seeks to provide dietary advice tailored to the individual. The precision or personalisation involved can encompass behavioural factors, including dietary intake, physical activity, etc. and/or biological factors, including age, sex, metabolic phenotypes and/or genomic profiles(Reference Ordovas, Ferguson and Tai1,Reference Adams, Anthony and Carvajal2) . It is hoped that designing more precise nutrition approaches based on behavioural and/or biological traits may increase the effectiveness of dietary interventions to improve health(Reference Blaak, Roche and Afman3). While there is no doubt that a ‘healthy’ diet has the potential to improve health, there is still a knowledge gap with respect to (i) accurately defining the most optimal dietary approach for groups of individuals and (ii) understanding how different individuals respond (or not respond) to dietary interventions. To this end, systems biology approaches employing high-throughput omics technologies, spanning functional genomics to characterise the host, as well as metagenomic approaches to characterise the microbiome, have great potential elucidating the full impact of diet on health (Fig. 1). It is hoped that such a comprehensive suite of tools, at the genome, transcriptome, proteome and/or metabolome levels, illustrated in Fig. 1, will improve our understanding of different diet-induced biological phenotypes, with a view to designing more effective therapeutic options.
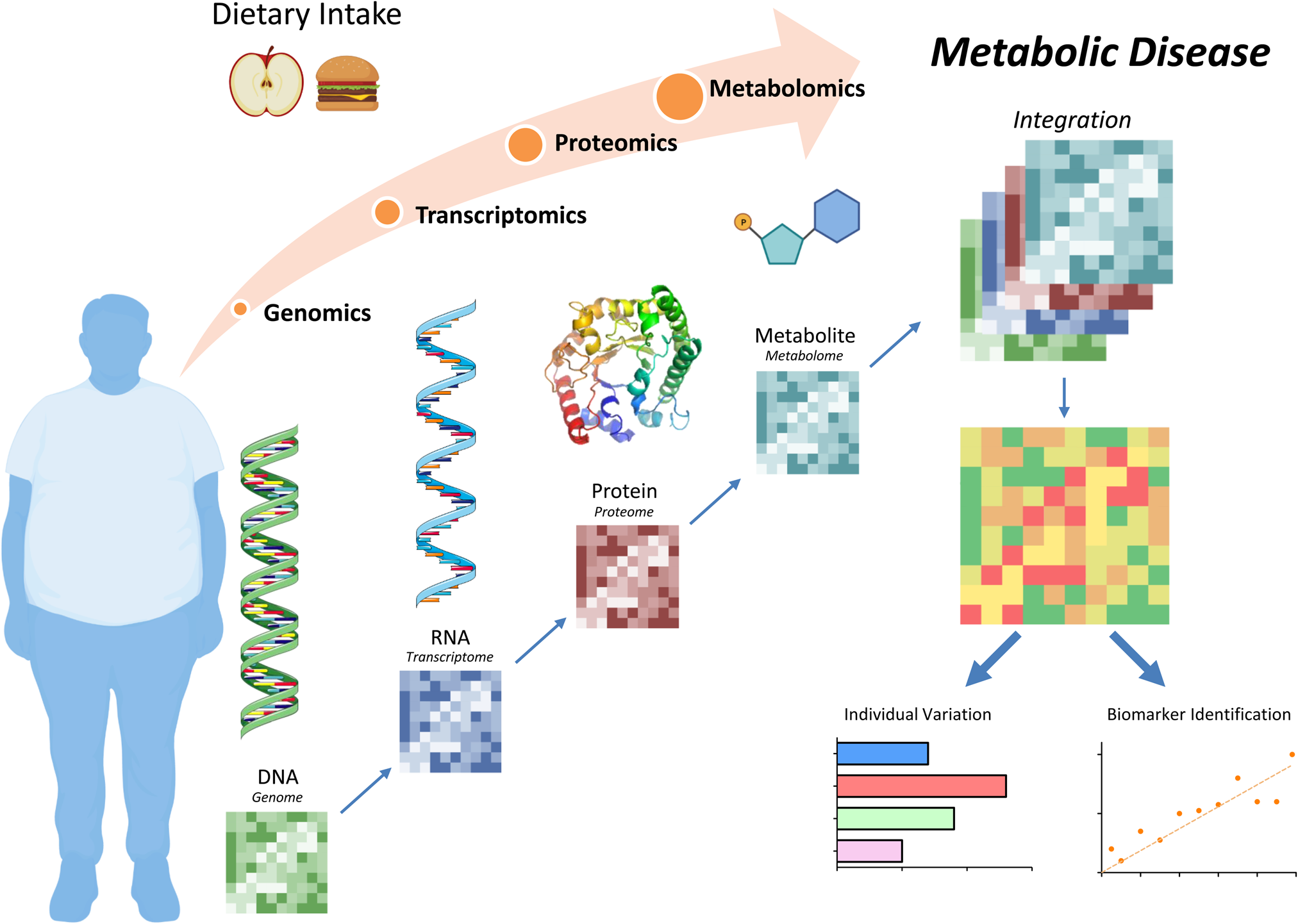
Figure 1. Diet and nutrition systems biology tools to understand metabolic disease. Dietary intake can affect metabolic health at the genome, transcriptome, proteome and/or metabolome levels. Integration of these analyses allows for personalised biomarker identifications with the goal to design unique, effective therapeutic options. Figure was created using Biorender.com.
Systems biology refers to the use of multiple omics technologies including genomics, epigenetics, transcriptomics, proteomics and metabolomics to generate high-dimensional datasets to characterise biological contexts. Over the past number of years, nutrition systems biology has taken advantage of the full gamut of omics applications to elucidate the interaction between food and nutrient intake on the genome, transcriptome, proteome and metabolome. In that time, it has also become evident that the interaction between different dietary or nutritional factors is not necessarily linear, as suggested in Fig. 1, as in a nutrient does not necessarily firstly interact with the genome, then the epigenome and transcriptome, etc. But there is a far more complex and dynamic interaction at multiple possible points within the systems biology network. Fig. 2 attempts to illustrate this paradigm, wherein feeding a diet can simultaneously co-regulate the transcriptome, proteome and metabolome. Also, resultant diet-derived metabolites may be the factor that alters the transcriptome or proteome, rather than the nutrient itself. To this end, precision nutrition systems biology is highly reliant upon computational and mathematical modelling to decipher biology. It is a very comprehensive approach to understand diverse biological scenarios and has great potential to support precision nutrition based on metabolic phenotype which may, in turn, increase the effectiveness of dietary and nutrition interventions.

Figure 2. Dietary, behavioural and environmental effects on systems biology networks to develop a personalised treatment plan. Interactions between dietary, physical activity (i.e. voluntary bodily movement) or environmental factors (i.e. cultural, social, economic, etc.) are not necessarily linear with respect to where the initial impact is seen within the systems biology analysis. This complex interaction highlights the importance of investigation at multiple points in order to fully elucidate the complex nutrient interactions and create and individualised personal treatment plan. Figure was created using Biorender.com.
This review will focus on the interrelationships that exist between metabolism and inflammation. Within the context of obesity-related disruption of immuno-metabolism, we will explore areas in which nutritional systems biology approaches have improved our understanding of the impact of different dietary elements. Obesity-related metabolic inflammation is a nice example to illustrate this paradigm, as in that biological context there is plenty of phenotypic diversity, both in terms of different phenotypes with variable clinical presentations and variable responses to different dietary interventions. Additionally, omics approaches facilitate determination of both metabolism and inflammation concurrently. In the future, it is hoped that this greater knowledge will facilitate precision nutrition.
Obesity-related metabolic dysfunction in disease
Obesity is a very heterogenous condition, which can give rise to several different co-morbidities, including insulin resistance (IR), type 2 diabetes and non-alcoholic fatty liver disease/non-alcoholic steatohepatitis. Recently, obesity has also emerged as a major determinant of cancer. The classical hallmark of obesity is the increase in adiposity, as a result of excessive dietary energy intake and insufficient energy expenditure. Although adipose tissue was initially thought to just store energy, it is now appreciated to have important endocrine functions, secreting hormones, cytokines and adipokines(Reference Crewe, An and Scherer4). When adipocyte storage capacity is exceeded, NEFA ‘spill out’ from adipose, increasing circulating NEFA concentrations with accumulation in other peripheral metabolic tissues contributing to metabolic disease development(Reference Guilherme, Virbasius and Puri5). Furthermore, in terms of understanding variable phenotypes, obesity is highly heterogenous wherein greater BMI and body fat, indicative of expanding adipose, does not always result in metabolic dysfunction and a pro-inflammatory phenotype(Reference Neeland, Ross and Despres6). There are several differentiating factors, including the location of the adipose depot, which has been shown to affect developmental gene expression, adipokine secretion, fatty acid handling and inflammatory cell infiltration amongst other characteristics(Reference Christiansen, Urena and Borisevich7,Reference Tchkonia, Thomou and Zhu8) . There are two main adipose depots, subcutaneous adipose tissue (SAT) located just beneath the skin and visceral adipose tissue (VAT) which surrounds the internal organs. Interestingly, in a lean cohort, matched SAT and VAT adipocyte transcriptome and DNA methylome shows different pathways fundamental to adipocyte function, including distinct transcription factors and developmental genes, highlighting the importance of adipocyte origins specific to different fat depots(Reference Bradford, Nair and Statham9). This displays that adipocytes contain varied gene activity in SAT v. VAT even before obesity is developed. The increase in obesity-driven metabolic disorders and inflammation is specifically linked to expanding VAT in comparison to SAT(Reference Meza-Perez and Randall10–Reference Porter, Massaro and Hoffmann12). Obese VAT proteins linked to innate immunity and transcriptional regulation were increased while fatty acid synthesis (or lipogenesis) and detoxification and mitochondrial function-related proteins were decreased in comparison to lean VAT(Reference Carruthers, Strieder-Barboza and Caruso13). Additionally, adipose tissue macrophages from obese human subjects lack classical activation surface markers following treatment with glucose, insulin and palmitate thus producing a metabolically activated phenotype with higher levels of lipid metabolism (i.e. PPARγ) and altered pro- and anti-inflammatory pathway activation(Reference Navarro-Ruiz, Lopez-Alcala and Diaz-Ruiz14). Interestingly, obese and overweight SAT transcriptomic analysis showed distinct tissue-specific IR signatures. Hepatic IR displayed an altered extracellular matrix gene expression profile, while skeletal IR showed an increased inflammatory gene expression profile(Reference van der Kolk, Kalafati and Adriaens15). Indeed, the postprandial response to ingesting a high-fat meal is greater in subjects with abdominal rather than gluteofemoral fat depots, independent of fasting lipid concentrations and BMI(Reference Christiansen, Urena and Borisevich7). When examining the genome-wide transcriptome of VAT v. SAT, IR-associated gene expression and DNA methylation was consistent between the VAT and SAT suggesting no distinct transcriptome signature of IR between depots(Reference Arner, Sahlqvist and Sinha16). However, paired SAT and VAT from severely obese humans' proteomic signatures showed many differentially expressed proteins. Differentially expressed proteins which were correlated with clinical and body composition data showed SAT was more active in vesicular transport and secretion with increase lipid metabolism. VAT was higher in mitochondrial energy metabolism and translation or biosynthetic activity(Reference Hruska, Kucera and Pekar17). One strength of proteome analysis is the ability to study post-translation modifications which are key to the functional protein structure. Important post-translation modifications in the acetylation of FABP4 within obese, insulin-resistant adipose SAT not VAT demonstrated a better understanding of depot-specific lipid handling metabolism and adipocyte differentiation in metabolically unhealthy human subjects(Reference Navarro-Ruiz, Lopez-Alcala and Diaz-Ruiz14). Furthermore, obese VAT proteome displayed an increase in glycolysis, lipogenesis, oxidative stress and mitochondrial dysfunction while gluteofemoral adipose had increased activation of fatty acid and branch-chain amino acid oxidation with an enhanced tricarboxylic acid (TCA) cycle flux and oxidative phosphorylation(Reference Raajendiran, Krisp and Souza18). Adipose depot investigations have proven heterogeneity at both the transcriptome and proteome stages. While adipose distribution can specifically determine the nature of metabolic phenotypes, there are other considerations with respect to metabolic complications.
Adipose tissue morphology is another important factor affecting biological variability. Adipose tissue expands by a combination of an increase in adipocyte number, hyperplasia and adipocyte size, hypertrophy (Fig. 3). Hypertrophic obesity occurs from the increase in pre-existing adipocyte size resulting in larger adipocyte volume. This is normally associated with IR. Alternatively, hyperplastic adipose results from new adipocytes formation, characterised by increased adipocyte number, which is usually associated with insulin sensitivity and minimal inflammation(Reference Sun, Kusminski and Scherer19). Euglycaemic–hyperinsulinaemic clamp studies show that adipocyte size is negatively correlated with insulin sensitivity(Reference Weyer, Foley and Bogardus20,Reference Lundgren, Svensson and Lindmark21) . Furthermore, IR obese subjects displayed larger adipocyte size compared to BMI-matched insulin-sensitive individuals(Reference McLaughlin, Lamendola and Coghlan22,Reference McLaughlin, Sherman and Tsao23) . It is hypothesised that the increase in adipocyte number allows for the excess lipid storage resulting in a metabolically healthy obese state and thus considered a protective mechanism. Another possibility may be the phenotype of the individual adipose cells present. Spatial and single-cell transcriptomics identified three distinct fat cell types in the SAT of obese individuals, named AdipoLEP, AdipoPLIN and AdipoSAA, reflecting leptin, perilipin and serum amyloid A within the adipocyte fraction. Interestingly, only AdipoPLIN showed a transcriptional response to insulin stimulation(Reference Backdahl, Franzen and Massier24). Even with advancements in knowledge of adipocyte heterogeneity, it is still unclear how and why an individual may develop hypertrophic v. hyperplastic adipose tissue. Adipose morphology may in turn affect the metabolic phenotype associated with obesity.

Figure 3. Adipose morphology may explain heterogeneity between obese individuals. Excess energy intake leads to expansion of lean adipose to either a hyperplastic adipose which is metabolically healthy or a hypertrophic adipose aligned with a metabolically unhealthy phenotype. The metabolically healthy phenotype has delayed insulin resistance with reduced inflammation while the metabolically unhealthy has an increase immune cell infiltration paired with a shift towards an inflammatory phenotype. Figure was created using Biorender.com. NK cell, natural killer cell; TH1 cell, T helper type 1 cell; TH2 cell, T helper type 2 cell.
From the dietary perspective, some exploratory transcriptomic analysis of adipose tissue from a large cohort of European subjects with diverse habitual dietary fat intake, showed that habitual long-chain n-3 PUFA and MUFA intake was associated with adipogenic transcriptomic signatures, indicative of hyperplastic adipose morphology, with less inflammatory signatures and metabolic health(Reference Morine, Tierney and van Ommen25). Nevertheless, the cross-sectional nature of the cohort only allows suggested associations. Therefore, more sophisticated personalised analytical methods studying habitual diet, adipose morphology and adipogenesis are necessary to elucidate whether or not diet-induced individualised mechanisms are at play, or not. Indeed, a subsequent animal feeding study showed that high-fat diets (HFD) enriched with MUFA induced a hyperplastic adipose morphology, as opposed to the hyperplastic phenotype induced with feeding SFA-HFD(Reference Finucane, Lyons and Murphy26). This altered morphology was ascribed to differential metabolic and inflammatory crosstalk in adipose tissue, wherein feeding MUFA was associated with attenuated adipose IL-1β expression, coupled with greater pre-adipocyte PPARγ and peroxisome proliferator-activated receptor gamma coactivator 1-alpha (PGC-1α) expression indicative of greater adipogenic potential, more functional hyperplastic adipose tissue and improved insulin sensitivity despite obesity(Reference Finucane, Lyons and Murphy26). It was interesting to note that a regression study showed that the adverse hyperplastic adipose architecture could not be reversed by feeding MUFA, once the animals had been exposited to SFA-HFD initially(Reference Finucane, Lyons and Murphy26). In an informative human study, van Dijk et al. used ingenuity pathway analysis to investigate the RNA expression profile of adipose tissue before and after a dietary SFA intervention. Their analyses revealed increased expression of inflammatory genes in adipose tissue of patients at risk of metabolic syndrome after consuming a diet rich in SFA for 8 weeks(Reference van Dijk, Feskens and Bos27). Their demonstration that a diet rich in MUFA caused a more anti-inflammatory profile suggests that changing from an SFA-rich diet to MUFA-rich diet could decrease the risk of inflammation-related diseases. Further dietary intervention studies investigating this hypothesis are however required.
While adipocytes represent the primary fat-storing cell components of adipose, the surrounding stromal vascular fraction is an important heterogeneous collection of cells including fibroblasts, pre-adipocytes, endothelial cells and immune cells among others. Chronic inflammation in obesity can lead to premature senescence in pre-adipocytes, reducing their ability to differentiate to mature adipocytes, contributing to adipose pathology. Furthermore, the markers of senescence correlate with an increase in adipose cell size(Reference Gustafson, Nerstedt and Smith28). The transcriptome of obese-differentiated adipocytes were devoid of mitotic gene expression, thus preventing the cell-cycle completion(Reference Li, Hagberg and Silva Cascales29). Analysis of pre-adipocytes shows how obesity disrupts adipogenesis and mature adipocyte functionality within the stromal vascular fraction. The immune cell fraction is integral to the immuno-metabolic phenotype of adipose. The number of immune cells, including macrophages and T cells, increases through infiltration paired with phenotypic alteration of cell populations to further increase metabolic inflammation(Reference Feuerer, Herrero and Cipolletta30,Reference Murphy, Lyons and Finucane31) . Several animal studies clearly demonstrate that expanding adipose tissue is associated with a phenotypic switch, wherein the anti-inflammatory M2 macrophages become pro-inflammatory M1 macrophages(Reference Lumeng, DelProposto and Westcott32). However, characterisation of this immuno-metabolic switch in adipose in human subjects is less clear cut. Nevertheless, we have prioritised insightful human work here. Obese type 2 diabetic VAT proteome displayed mitochondrial dysfunction and decreased adipocyte differentiation which increases M1 macrophage recruitment(Reference Gomez-Serrano, Camafeita and Garcia-Santos33). The presence of infiltrating B cells, and T cells, CD4+ Th1, CD8 and natural killer cells paired with a reduction in regulatory T cells and innate lymphoid cells disrupts the normal immune response in obese adipose(Reference Cox, Chernis and Masschelin34). Single-cell suspensions isolated from human obese SAT displayed an increase in adipose-resident dendritic cells, unconventional T cells, innate lymphoid cells and natural killer-like cells. Furthermore, genomic profiling of these obese distinct cell populations shows a shift towards pro-inflammatory cellular subsets influenced by specific cytokines (TNF, interferon-γ, IL-1) and hypoxia. Additionally, the lean adipose displayed protective anti-inflammatory interactomes(Reference Hildreth, Ma and Wong35). Single-cell genomics studies aim to determine the heterogeneity and distinct signature of metabolic disease and depots. These found that metabolic disease status is correlated with adipose-resident immune cells being metabolically active with immune dysfunction(Reference Vijay, Gauthier and Biswell36). Interestingly, sex-specific differences in adipose-resident populations have been discovered. Transcriptomic murine investigations showed an increase in adipose regulatory T cells in males paired with increased inflammation compared to females(Reference Vasanthakumar, Chisanga and Blume37). The human transcriptome highlights sex-specific differences with female SAT having more CD4+ T cells and male SAT having more CD8+ T cell subsets in adipose(Reference Lenz, Arts and Peeters38). These differences indicated a sex-specific difference in adipose tissue cellular populations and likely within phenotypic profiles. The increased population and altered phenotype of adipose-resident immune cells during obesity are essential to the development of chronic low-grade inflammation integral to metabolic disease and thought to play an important role in obesity-related cancer risk(Reference Gregor and Hotamisligil39,Reference Deng, Lyon and Bergin40) .
Diet, metabolism and inflammation: cellular perspectives
Diet is one of the most important drivers of obesity-related metabolic disease and chronic inflammation, which is evident at both the cellular and whole-body levels. In immune cells, metabolic inflammation refers to the process wherein the metabolic configuration of a cell determines and drives the nature of an immune response. This insight was generated by Jha et al., employing elegant system biology approaches, integrating 13C-glucose and 13C-glutamine stable isotope tracer experiments with both transcriptomic and metabolomic tools, to demonstrate the immuno-metabolic phenotype of immune cells(Reference Jha, Huang and Sergushichev41). This was one of the first studies which demonstrated that pro-inflammatory M1 macrophage metabolism was associated with a ‘metabolic break’ in the mitochondrial TCA cycle, preventing the conversion of isocitrate to α-ketoglutarate. This study used elegant metabolomics showing the important impact of metabolism on immune function. This insight resonates with the Warburg effect, characterised by enhanced glycolysis, that typifies pro-inflammatory cells including M1 macrophages. In contrast, immunoregulatory and/or resolving M2 macrophages rely more on fatty acid oxidation as their energy source and display an intact TCA cycle. A metabolic shift to M1 macrophages with disrupted TCA cycle shows an increase in acetyl-CoA, citrate and succinate(Reference Tannahill, Curtis and Adamik42). Obese SAT adipose tissue macrophages displayed altered succinate–SUCNR1 pathway highlighting disrupted TCA cycle within obese adipose-resident macrophages paired with decreased anti-inflammatory markers compared to a lean control(Reference Keiran, Ceperuelo-Mallafre and Calvo43). In whole adipose, SAT transcriptomic integration with plasma metabolomics displayed an increase in glutaminase paired with a decrease in glutamine synthase and changes in branch-chain amino acids in mitochondria. Furthermore, integration of SAT transcriptomic and proteomic profiles from obese individuals show down-regulation of many genes involved in the TCA cycle, specifically citrate synthase and acetyl-CoA production along with mitochondrial pyruvate carrier 1 and 2, suggesting reduced mitochondrial respiration(Reference Mardinoglu, Kampf and Asplund44). Both obese adipose tissue and adipose tissue macrophages display characteristics suggesting a disrupted TCA cycle.
Diet is a key source of both exogenous, as well as endogenous metabolites including glucose, fatty acids, cholesterol and amino acids, which are key elements of immune cell metabolism. To date, most research has demonstrated that this paradigm is relevant in obesity, wherein obesity is associated with metabolic dysregulation and pro-inflammatory phenotypes. The question is whether the paradigm extends beyond obesity per se. Substantial research suggests that different dietary elements (e.g. SFA, etc.) and/or their resultant metabolites (e.g. ceramides, cholesterol, etc.) can promote inflammation. There is less clarity with respect to whether dietary manipulations, with subsequent whole-body metabolic reconfiguration, modulated cellular metabolism to attenuate inflammation.
In terms of exploring whether dietary elements can differentially impact metabolic inflammation, the NLRP3 inflammasome pathway, which is integral to obesity-associated adipose tissue inflammation and metabolism, is a helpful example. The NLRP3 inflammasome is a protein complex that responds to diverse stress stimuli, both endogenous and exogenous (i.e. lipopolysaccharide (LPS), lipids), to produce pro-inflammatory cytokines (e.g. IL-1β and IL-18) and disrupt insulin signalling(Reference Finucane, Lyons and Murphy26,Reference Dunne, Ross and Pospisilova45–Reference Wen, Gris and Lei47) . The NLRP3 inflammasome pathway is activated through a complex two-stage process that has been reviewed previously(Reference Ralston, Lyons and Kennedy48,Reference Charles-Messance, Mitchelson and De Marco Castro49) . Briefly, there is an initial priming stage with Toll-like receptor stimulation by exogenous stimuli followed by a secondary activation stage induced by multiple triggers (i.e. ATP, reactive oxygen species, ceramides, etc.) to induce cleavage of pro-inflammatory cytokines into their active form. Western diets generally consist of high daily intake of processed foods rich in SFA, cholesterol, refined sugar and simple carbohydrates. SFA-rich high-fat, high-cholesterol diets can prime both IL-1β and IL-18 pro-inflammatory cytokine production in conjunction with Toll-like receptor activation, driving adipose tissue inflammation through NLRP3 inflammasome activation(Reference Wen, Gris and Lei47,Reference Ralston, Lyons and Kennedy48,Reference Reynolds, McGillicuddy and Harford50–Reference Tall and Westerterp52) , co-incident with significant metabolic re-configuration of the TCA cycle. A disrupted TCA cycle may also lead to an increase in reactive oxygen species production, which is a requirement of SFA-driven inflammasome activation(Reference Wen, Gris and Lei47,Reference Huang, Rutkowsky and Snodgrass51,Reference Martinez-Reyes and Chandel53) .
In contrast, it is often purported that the Mediterranean style diet, providing more MUFA than SFA, does not promote inflammation. While animal feeding studies show that MUFA do not induce NLRP3 priming and activation of pro-inflammatory IL-1β and IL-18 bone marrow-derived macrophages and adipose tissue, which is often seen in response to an SFA-rich HFD(Reference Finucane, Lyons and Murphy26), translation of this has not been demonstrated in human subjects. The mechanisms which underlie this include an increase in anti-inflammatory gene expression (Mrc1, Tgfb1, IL-10, Mgl2) and a shift in macrophage metabolism towards M2 phenotype with an increase in oxygen consumption rate(Reference Chan, Pillon and Sivaloganathan54). There is also evidence of an AMP-activated protein kinase-dependent mechanism, where MUFA-induced obesity is not associated with lower AMP-activated protein kinase activity, as is usually the case in SFA-associated obesity and a heightened inflammatory response(Reference Finucane, Lyons and Murphy26,Reference Chan, Pillon and Sivaloganathan54) . Indeed, exposing bone marrow-derived macrophages to MUFA increases oxidative phosphorylation, compared to SFA. Additionally, when bone marrow-derived macrophages were exposed to both MUFA and SFA, they displayed similar oxygen consumption levels with a MUFA alone(Reference Chan, Pillon and Sivaloganathan54). Since oxidative phosphorylation is tightly paired with the TCA cycle, this may suggest that MUFA may maintain TCA cycle activity to a greater extent than SFA. These animal and cell studies suggest that replacing SFA with MUFA may reduce chronic inflammation state and IR. Human studies exploring the interactions between different dietary fats within obesity-associated metabolic-inflammation are warranted to potentially tailor dietary recommendations to specific biological requirements.
Innate immune training and metabolism
Innate immune training (IIT) is defined as the long-term functional reprogramming of innate immune cells, evoked by endogenous or exogenous factors, leading to a time- and context-adjusted response towards a secondary challenge once the cells have returned to their non-activated state. Immune cells can become trained, or primed, in response to a range of different immune-stimulants, inducing a state of hyper- or hypo-responsiveness, that can play either a protective or pathogenic role in reconfiguring the nature of the innate immune response(Reference Netea, Domínguez-Andrés and Barreiro55). A recent review elegantly explored the nature of heighted innate immune perturbation in cardio-metabolic disease(Reference Bahrar, Bekkering and Stienstra56). Extensive work has demonstrated that background diet, in particular dietary fats and fructose, can affect long-term immune function, via metabolic reprogramming(Reference Finucane, Lyons and Murphy26,Reference Ralston, Lyons and Kennedy48,Reference Reynolds, McGillicuddy and Harford50,Reference Christ, Lauterbach and Latz57,Reference Kajani, Curley and O'Reilly58) .
Extensive prior research has demonstrated that several elements within the ‘Western diet’ can function as pathogenic inducers of IIT, including SFA, cholesterol, etc., as previously reviewed(Reference Charles-Messance, Mitchelson and De Marco Castro49,Reference Reynolds, McGillicuddy and Harford50) . Christ et al. advanced the state of the art by showing that background diet ‘resets’ cholesterol metabolism and inflammation, such that feeding mice a Western diet, composed of 21⋅2 % fat (12⋅8 % SFA, 5⋅6 % MUFA and 1 % PUFA), induced ‘pathogenic’ IIT, typified by a sustained pro-inflammatory innate immune state(Reference Christ, Günther and Lauterbach59). Christ et al. demonstrated that bone marrow-derived macrophage cytokine expression remained increased following Toll-like receptor ligand stimulation, even though systemic inflammation returned to baseline and the animals returning to a low-fat diet, following the initial Western diet challenge(Reference Christ, Günther and Lauterbach59). This indicates that the Western diet induced myeloid cell reprogramming, resulting in long-term hyperresponsiveness despite a return to a chow diet. To study the interplay between diet and the inflammatory response, RNAseq, principal component analysis, and hierarchical clustering of variable genes revealed changes in transcriptional response following a Western diet. Investigating which gene ontology terms and Kyoto encyclopaedia of genes and genomes pathways were enriched following a Western diet determined that upregulated genes were associated with immune response, regulation of metabolic processes, response to external stimuli and stress and cell proliferation and differentiation. Further analysis for gene ontology terms and Kyoto encyclopaedia of genes and genomes pathways following in vivo LPS administration showed that adopting a Western diet primed the cells for LPS responses and promoted increased inflammatory signalling. Assay for transposase-accessible chromatin sequencing investigated if Western diet changed the overall accessibility of chromatin. Their analyses revealed that several of the genes associated with the changes in accessible genomic loci were linked to IL-6 production and Janus kinase/signal transducer and activator of transcription pathway, both key to inflammatory signalling. This study demonstrated that a Western diet results in bone marrow cellular compartment remodelling, on both transcriptional and epigenetic levels, leading to increased proliferation, downregulated cholesterol biosynthesis, generation of exaggerated inflammatory responses and that the NLRP3 inflammasome is critical for these changes. It also showed that despite returning the mice to normal chow diet, transcriptomic reprogramming did not reverse(Reference Christ, Günther and Lauterbach59).
The effects of dietary-related inducers of IIT are not necessarily detrimental. One of the most investigated IIT inducers is plant/fungal/bacterial cell wall component β-glucan. Treatment of murine macrophages with β-glucan primes macrophages to exhibit increased cytokine response upon infection, offering protection against subsequent infections (e.g. antibiotic-resistant Staphylococcus aureus)(Reference Liang, Melican and Cafro60). Increased expression of TNF-α and IL-6 in response to β-glucan stimulation followed by Toll-like receptor 4/2 re-stimulation in monocytes is a hallmark of IIT. A metabolic shift towards aerobic glycolysis is also a key component of IIT. It is hypothesised that this metabolic shift is pivotal for the induction of an altered inflammatory response following a secondary stimulus. Researchers investigated this by demonstrating that inhibition of key enzymes involved in glycolytic pathways and the citric acid cycle resulted in decreased TNF-α and IL-6 expression, therefore decreasing the IIT response. This demonstrates the relationship between metabolic reprogramming and inflammation in the context of IIT(Reference Pan, Hao and Zheng61). A gap in the knowledge remains, as the relationship between metabolism and inflammation in IIT is yet to be fully elucidated. Current research is investigating the potential uses of β-glucan containing foods/supplements for supporting a healthy immune system and metabolic health in obesity(Reference Vetvicka, Vannucci and Sima62).
In terms of mechanisms, epigenetic reprogramming of immune genes and corresponding enhancers plays a pivotal role in IIT. Epigenetic changes can be induced by changes in the metabolites produced by the gut microbiome or host cells. Metabolites provide energy and substrates for active remodelling of the chromatin and genome, which have been reviewed extensively elsewhere(Reference Fanucchi, Domínguez-Andrés and Joosten63). Changes in gene transcription then influence metabolism, bringing the relationship between the metabolome, epigenome and transcriptome full circle as these changes in metabolism have a further knock-on effect on epigenetics(Reference Fanucchi, Domínguez-Andrés and Joosten63,Reference Chiefari, Foti and Sgarra64) . Human monocytes were exposed to oxidised-LDL and re-stimulated with LPS and Pam3Cys, a synthetic compound that mimics bacterial lipoproteins. Both LPS and Pam3Cys are cell wall components of Gram-negative bacteria which are increased in cases of obesity, Western diet consumption and dysbiosis(Reference Bekkering, Quintin and Joosten65–Reference Hersoug, Moller and Loft67). Following a rest period, the mRNA expression and protein formation of proatherogenic proteins, e.g. IL-18, IL-6, TNF-α, monocyte chemoattractant protein-1, etc., were increased, as well as elevated trimethylation of lysine 4 at histone 3 in promoter regions of il-18, il-6, tnfα, mcp-1, etc. This shows the transcriptional and epigenetic changes resulting from oxidised-LDL exposure and second hit stimulation from LPS/Pam3Cys and how this could contribute to metabolic syndrome and promote inflammation in the context of obesity/Western diet(Reference Bekkering, Quintin and Joosten65).
The heterogeneity in IIT responses represented in patients results in complications for effective treatment of conditions, increasing the interest in precision nutrition. Increasing evidence indicates that the changes induced by innate immune trainers are highly dependent on the gut microbiome; influenced by e.g. genetics, environment and diet(Reference Jandhyala, Talukdar and Subramanyam68,Reference McCoy, Burkhard and Geuking69) . Despite the intensive research that has been carried out over the past number of decades to understand the transcriptomic, epigenomic and metabolomic implications of IIT, much remains unknown. To fully benefit from the potential therapeutic uses of dietary compounds to positively induce IIT, it is pivotal to understand the heterogenous nature of the mechanisms underpinning IIT.
Phenotype-specific dietary responses relating to metabolism and inflammation
Precision nutrition aims to understand the complex interrelationships to optimise metabolic responses to diet and tailor dietary approaches to ultimately make more effective, targeted, individual dietary recommendations to prevent and/or treat diseases and improve health. A recent NIH workshop identifying ‘Research gaps and opportunities in precision nutrition’(Reference Lee, Ordovas and Parks70) highlighted that many factors, beyond individual biological responses reflecting differences in disease risk addressed herein, including, socio-environmental, behavioural, cultural factors and psychosocial factors that alter responses to dietary interventions, need to be defined/refined. Nevertheless, for brevity we will use the postprandial response to food intake as a paradigm to illustrate advances attained using systems biology approaches to better understand the nature of phenotype-specific dietary responses. Over the past number of years, the evidence underpinning the impact of the microbiome on postprandial responses to food intake in human subjects has grown substantially. Several groups successfully used the acute postprandial response to food intake to highlight diverse metabolic and inflammatory phenotypes(Reference Fatima, Connaughton and Weiser71,Reference O'Grada, Morine and Morris72) , which has been further extended as a tool to design personalised nutrition approaches. Zeevi et al. elegantly demonstrated systems approaches integrating traditional blood biomarkers, dietary intake and anthropometric data, with detailed gut microbiota profiling to more accurately predict an individual's postprandial glycaemic response(Reference Zeevi, Korem and Zmora73). The gut microbiome also emerged as the most important person-specific factor influencing the large inter-individual variability in postprandial TAG responses beyond meal composition, fasting parameters and host genetics in the PREDICT study which sought to determine if the metabolic responses to food could inform cardiometabolic disease risk profiling in n 1002 twins and unrelated healthy adults(Reference Berry, Valdes and Drew74). Indeed, PREDICT also used a meal-induced metabolic trigger approach to elicit an acute inflammatory response indicative of chronic inflammation(Reference Berry, Valdes and Drew74), wherein they used NMR metabolomics to quantify glycoprotein acetylation, another emerging inflammatory biomarker. Taken one step further, a blinded dietary intervention based on an algorithm, integrating both host phenotypic and gut microbiota data, resulted in lower postprandial responses to food intake with altered gut microbiome composition – suggesting we can now not only use biological phenotypes to predict response but also the microbiome(Reference Zeevi, Korem and Zmora73). Indeed, the same group recently demonstrated that artificial sweeteners are not inert dietary compounds, rather they may impact the human microbiome to induce a person-specific, microbiome-dependent glycaemic response(Reference Suez, Cohen and Valdes-Mas75). Thus, it is insufficient to just look at the individual without the gut microbiome. To this end human faecal microbiome transfer studies are a potential research tool to moving beyond associations in terms of understanding the functional impact of human microbiota on metabolism and inflammation. Indeed, our group demonstrated that faecal microbiome transfer of an obese diabetic human's microbiome specifically augments HFD-induced hepatic steatosis and IR, whereas transplantation of a microbiome from a metabolically healthy obese individual did not(Reference Mitchelson, Tran and Dillon76). Interestingly, the faecal microbiome transfer-induced adverse metabolic phenotype was somewhat resolved by yeast β-glucan supplementation(Reference Mitchelson, Tran and Dillon76). Therefore, if potential efficacy of yeast β-glucan supplementation is partly microbiome dependent, then reverting adverse metabolic phenotypes requires precision nutrition, with an understanding of potential interactions between diet, gut microbiota and host metabolism.
Conclusions
Heterogeneity in an individual's response to obesity-driven disease and dietary intervention efficacy calls for greater insights into the mechanistic basis of that diversity, requiring a shift towards precision nutrition with more targeted investigation and treatment options. Advancements in systems biology technologies have provided many invaluable insights into heterogeneity within obesity, wherein there can be people, often with less progressed disease, who remain somewhat metabolically healthy v. those obese people with profound adverse metabolic phenotypes. However, there still remains a gap with respect to how an individual's habitual diet behaviours drive specific adipose morphology, adipogenesis and IIT mechanisms. In order to elucidate these mechanisms, large cohort studies involving dietary interventions in metabolically healthy and unhealthy patients using integration of multiple systems biology technologies are necessary to effectively predict a personal response. Indeed, coupled with emerging studies that address precision nutrition responses in a phenotype-based approach, such as that recently reported in the PERSON study(Reference Gijbels, Trouwborst and Jardon77), will greatly advance our knowledge in relation to the context and capability precision nutrition can facilitate the adoption of healthier diet and lifestyle programmes for enhanced efficacy.
Financial Support
K. A. J. M. and H. M. R. are funded by Precision Oncology Ireland (18/SPP/3522), a Science Foundation Ireland Strategic Partnership Programme. M. B. Ní C. and H. M. R. are funded by Science Foundation Ireland Frontiers Award Programme (SFI 19/FFP/6625).
Conflict of Interest
None.
Authorship
The authors had sole responsibility for all aspects of preparation of the present paper.