Introduction
Many important policy challenges, like climate change, pandemics, and digitalization, entail a high degree of complexity, both with respect to the policy problem itself and its solutions (Weber, Reference Weber2017; Sewerin, Reference Sewerin, Capano and Howlett2020). In reality, effective policy solutions to ‘super-wicked problems’ like climate change (Levin et al., Reference Levin, Cashore, Bernstein and Auld2012, p. 124) typically require a fundamental transformation of socio-technical systems and take the form of complex policy packages – that is, a holistic combination of several policy instruments, rather than single policy measures (Givoni et al., Reference Givoni, Macmillen, Banister and Feitelson2013; Howlett and Rayner, Reference Howlett and Rayner2013; Kivimaa and Kern, Reference Kivimaa and Kern2016; Rogge and Reichardt, Reference Rogge and Reichardt2016; Schmidt et al., Reference Schmidt, Matsuo and Michaelowa2017; Bataille et al., Reference Bataille, Guivarch, Hallegatte, Rogelj and Waisman2018; Markard, Reference Markard2018; Kern et al., Reference Kern, Rogge and Howlett2019). However, policymakers who propose ambitious policies for tackling climate change can face serious public opposition, given that effective instruments for accelerating system transformation can also interfere with citizens’ consumption behaviors in costly ways and are perceived as restrictive (Buchanan and Tullock, Reference Buchanan and Tullock1975; Oates and Portney, Reference Oates, Portney, Mäler and Vincent2003; Bernauer, Reference Bernauer2013; Creutzig et al., Reference Creutzig, Roy, Lamb, Azevedo, Bruin, Dalkmann, Edelenbosch, Geels, Grubler, Hepburn, Hertwich, Khosla, Mattauch, Minx, Ramakrishnan, Rao, Steinberger, Tavoni, Ürge-vorsatz and Weber2018). Public backlash creates major hurdles to the adoption of transformative and behavioral climate policies given that, in democracies, the political feasibility of publicly salient policies with perceptible implications for citizens’ everyday lives is – among other factors – a function of public support (Bernauer, Reference Bernauer2013; Anderson et al., Reference Anderson, Böhmelt and Ward2017; Fesenfeld, Reference Fesenfeld2020; Goldberg et al., Reference Goldberg, Gustafson, Ballew, Rosenthal and Leiserowitz2021; Rinscheid et al., Reference Rinscheid, Pianta and Weber2021). In other words, policymakers face a trade-off between problem-solving effectiveness and political feasibility.
A growing body of literature has recently suggested that bundling costly and compensatory measures into holistic policy packages can increase public support for ambitious climate policies across different ideological groups (Häusermann et al., Reference Häusermann, Kurer and Traber2018; Ingold et al., Reference Ingold, Stadelmann-Steffen and Kammermann2019; Wicki et al., Reference Wicki, Fesenfeld and Bernauer2019, Reference Wicki, Huber and Bernauer2020; Bergquist et al., Reference Bergquist, Mildenberger and Stokes2020; Fesenfeld et al., Reference Fesenfeld, Wicki, Sun and Bernauer2020). Nevertheless, increasing the political feasibility of ambitious climate policies by adding compensatory measures to policy packages comes at a price: increased policy design complexity. However, thus far most public opinion research has studied public support for single policy instruments, thus knowledge is lacking about the relationship between the complexity of proposed policy designs and public opinion. This paper addresses this research gap.
The study contributes to public policy, public opinion, and policy design literature by presenting an analysis of how the complexity of proposed policy designs impacts public opinion. The paper thus focuses on the contested and salient issue of climate change, which requires a rapid and fundamental transformation of socio-technical systems, for example, transport and food systems (Creutzig et al., Reference Creutzig, Roy, Lamb, Azevedo, Bruin, Dalkmann, Edelenbosch, Geels, Grubler, Hepburn, Hertwich, Khosla, Mattauch, Minx, Ramakrishnan, Rao, Steinberger, Tavoni, Ürge-vorsatz and Weber2018; Fesenfeld et al., Reference Fesenfeld, Schmidt and Schrode2018; Poore and Nemecek, Reference Poore and Nemecek2018; Steg, Reference Steg2018). Specifically, I experimentally assess if citizens differently evaluate isolated and combined climate policies with perceptible cost implications for citizens’ day-to-day lives.
Theoretically, the study speaks to the burgeoning literature on the design of policy mixes, as well as to theories of rational choice and bounded rationality in political science and public opinion research. While classical rational choice models assume that people have stable preferences, bounded rationality theory rests on the assumption that peoples’ capacity to process information in a fully rational way is restricted by cognitive limitations and resource constraints (e.g., time and knowledge) (Simon, Reference Simon1957; Jones, Reference Jones1999; Druckman, Reference Druckman2004; Jones and Baumgartner, Reference Jones and Baumgartner2005; Chong and Druckman, Reference Chong and Druckman2007a; Kahneman, Reference Kahneman2011). The apparent consensus in much of the public opinion and framing literature is that people are ‘cognitive misers’ who seek to reduce mental effort, and that ‘elites can manipulate popular preferences to serve their own interests’ (Chong and Druckman, Reference Chong and Druckman2007a, p. 104) through ‘(often small) changes in the presentation of an issue or an event’ (Chong and Druckman, Reference Chong and Druckman2007a, p. 104). By assessing how changes in the presentation and the complexity of proposed policy designs affect public opinion, this study hence also aims at contributing to the public choice-related debate about bounded rationality, framing, and the instability of citizens’ attitudes (Converse, Reference Converse and Apter1964; Achen, Reference Achen1975; Page and Shapiro, Reference Page and Shapiro1992; Zaller, Reference Zaller1992; Zaller and Feldman, Reference Zaller and Feldman1992; Jones, Reference Jones1999; Freeder et al., Reference Freeder, Lenz and Turney2019).
Going beyond the existing policy design and public opinion literature, I here argue that complex climate policy packages affect public opinion through a compensation, policy perception, and design complexity mechanism.
First, in line with classical rational choice models, the emerging public opinion literature on policy packaging often claims that policymakers need to design politically feasible policy packages in ways that compensate voters for perceived policy-induced costs (Häusermann et al., Reference Häusermann, Kurer and Traber2018; Ingold et al., Reference Ingold, Stadelmann-Steffen and Kammermann2019; Wicki et al., Reference Wicki, Fesenfeld and Bernauer2019, Reference Wicki, Huber and Bernauer2020; Bergquist et al., Reference Bergquist, Mildenberger and Stokes2020; Fesenfeld et al., Reference Fesenfeld, Wicki, Sun and Bernauer2020; Reference Fesenfeld, Rudolph and Bernauer2022). Policymakers can do this by bundling desired and undesired policy instruments into more complex policy packages (Häusermann et al., Reference Häusermann, Kurer and Traber2018; Ingold et al., Reference Ingold, Stadelmann-Steffen and Kammermann2019; Wicki et al., Reference Wicki, Fesenfeld and Bernauer2019, Reference Wicki, Huber and Bernauer2020; Bergquist et al., Reference Bergquist, Mildenberger and Stokes2020; Fesenfeld et al., Reference Fesenfeld, Wicki, Sun and Bernauer2020).
Second, going beyond the existing literature, I hypothesize that complex policy packages also alter citizens’ perceptions of policy effectiveness in reducing emissions and of policy restrictiveness (i.e., intrusiveness in personal life) (Drews and van den Bergh, Reference Drews and van den Bergh2016; Huber et al., Reference Huber, Wicki and Bernauer2019; Fesenfeld, Reference Fesenfeld2020). Here, I argue that citizens perceive more complex policy package designs that combine multiple climate policy instruments as more effective at mitigating climate pollutants, but also as more restrictive than isolated climate policies.
Third, in line with bounded rationality theory (Simon, Reference Simon1957), the complexity hypothesis proposed here implies that citizens have limited cognitive capacity and that their policy preferences can thus be altered by confronting voters with an increasing information load, a tactic that may be employed by politicians to avoid blame by diluting citizens’ focus on policy-induced losses (Weaver, Reference Weaver1986; Jacobs, Reference Jacobs2011).
To empirically examine the three potential mechanisms through which policy design complexity and packaging can affect public opinion, a two-stage, conjoint-based experimental approach was designed and embedded in original surveys fielded in Germany and the USA (total N = 9115), using quota sampling to ensure representative samples in terms of age, gender, income, rural-urban, and region. This conjoint design is novel since it assigns individuals to choose between randomly designed packages with varying degrees of policy design complexity, policy stringencyFootnote 1 (i.e., the level of ambitiousness compared to the status quo), and combinations of different policy measures. Namely, respondents in the two experimental conditions were confronted with policy proposals that included single instruments of varying stringency, or a combination of the same instruments, also of varying stringency (see Methods for details).
I selected the USA and Germany as country cases because of their importance in the global climate policy debate and their large per capita carbon footprints. Moreover, these two countries represent two mature democracies with high per capita income but significant differences in terms of political institutions, population size, cultural norms, and individual ideological predispositions towards ambitious climate policy. Employing a survey-embedded experimental study design across these two different cases permits the comparison of results in two distinct settings and increases the external validity of findings.
In this study, I specifically focus on the case of behavioral climate policies aimed at reducing meat consumption and the use of cars that run on fossil fuels. The practical relevance of identifying politically feasible policy solutions in these two sectors is high (McCollum et al., Reference McCollum, Wilson, Bevione, Carrara, Edelenbosch, Emmerling, Guivarch, Karkatsoulis, Keppo and Krey2018; Poore and Nemecek, Reference Poore and Nemecek2018). At least 26% of worldwide greenhouse gas (GHG) emissions are associated with the food sector (Poore and Nemecek, Reference Poore and Nemecek2018), and it is the main source of global methane emissions, which increase the risk of runaway climate change in the near term (Fesenfeld et al., Reference Fesenfeld, Schmidt and Schrode2018). Without mitigating emissions from the global food system and changing diets to include more plant-based products, it will be very difficult to achieve the Paris climate targets (Clark et al., Reference Clark, Domingo, Colgan, Thakrar, Tilman, Lynch, Azevedo and Hill2020). Similarly, road transport contributes more than 18% of global greenhouse gas emissions and transport-related emissions have grown steadily in recent years, constituting an important roadblock to climate change mitigation (Creutzig et al., Reference Creutzig, Jochem, Edelenbosch, Mattauch, van Vuuren, McCollum and Minx2015).
Moreover, I selected these two cases because effective climate policy packages in the food and transport sector typically require complex policy solutions along supply chains (Bajželj et al., Reference Bajželj, Richards, Allwood, Smith, Dennis, Curmi, Gilligan, Lee-gammage, Baj, Richards, Allwood, Smith, Dennis, Curmi and Gilligan2014; Poore and Nemecek, Reference Poore and Nemecek2018; Springmann et al., Reference Springmann, Clark, Mason-D'Croz, Wiebe, Bodirsky, Lassaletta, de Vries, Vermeulen, Herrero and Carlson2018). It is especially climate policies that target the behavior that can successfully trigger socio-technical transformation to meet climate change mitigation targets which are characterized by a high level of policy design complexity, and also interfere substantially with citizens’ consumption behaviors (Sparkman et al., Reference Sparkman, Howe and Walton2021; van der Linden et al., Reference van der Linden, Pearson and Van Boven2020). This makes the two sectors particularly prone to public scrutiny and increases the relevance of making reliable and accurate evaluations of public opinion. Finally, comparing results across these two sectors and country cases increases the external validity of the findings.
The study proceeds as follows: First, I provide a brief review of the current policy design and packaging debate literature and identify to what extent the link between policy design complexity, packaging, and public opinion is a relevant research gap. Based on this literature review, I then develop arguments involving why and how policy packaging affects public opinion via a compensation, perception, and design complexity mechanism. After outlining the study design, I discuss empirical results and potential avenues for future research.
Linking the complexity of policy designs and public opinion research
While policy proposals can be comprised of single policy instruments (also called policy measures, such as a standalone carbon tax), they are often complex arrangements of different policy goals and policy instruments that are mixed in a more (or less) systematic fashion (Howlett and Rayner, Reference Howlett and Rayner2013). Givoni defines a policy package as ‘a combination of policy measures designed to address one or more policy objectives, created in order to improve the effectiveness of the individual policy measures, and implemented while minimizing possible unintended effects, and/or facilitating interventions’ legitimacy and feasibility’ (Givoni et al., Reference Givoni, Macmillen, Banister and Feitelson2013, p. 3).
Givoni et al. (Reference Givoni, Macmillen, Banister and Feitelson2013) differentiate between primary and ancillary measures. Primary measures are designed to directly affect the object of the policy – for example, reducing GHG emissions by setting regulatory emission standards for producers (Givoni et al., Reference Givoni, Macmillen, Banister and Feitelson2013). Ancillary measures, in contrast, aim at rectifying the potentially unintended externalities of primary measures – such as the regressive cost structure of specific consumption taxes (Hsu et al., Reference Hsu, Walters and Purgas2008; Sælen and Kallbekken, Reference Sælen and Kallbekken2011; Drews and van den Bergh, Reference Drews and van den Bergh2016; Carattini et al., Reference Carattini, Kallbekken and Orlov2019; Fairbrother, Reference Fairbrother2019). According to Givoni et al. (Reference Givoni, Macmillen, Banister and Feitelson2013), the mixing of primary and ancillary measures might lead to more effective and legitimate outcomes than applying unilateral interventions (see also Rogge and Reichardt, Reference Rogge and Reichardt2016; Schmidt and Sewerin, Reference Schmidt and Sewerin2019).
In theory, policymakers combine different policy instruments in an effort to meet policy goals effectively and efficiently, yet in reality, they often do not adhere to policy design principles such as consistency, coherence, and congruence (Howlett and Rayner, Reference Howlett and Rayner2013); that is, many policymakers seek to maximize political feasibility rather than aiming for ideal-type policy designs that could more effectively solve policy problems (Gunningham and Sinclair, Reference Gunningham and Sinclair1999).
Flanagan et al. (Reference Flanagan, Uyarra and Laranja2011) and Rogge and Reichardt (Reference Rogge and Reichardt2016) claim that the debate about policy mix designs originally emerged in the economic policy literature in the 1960s (see, e.g., Mundell, Reference Mundell1962) and has only more recently spread to environmental economics (see, e.g., Lehmann, Reference Lehmann2012), transportation research (see, e.g., Givoni et al., Reference Givoni, Macmillen, Banister and Feitelson2013), transition and innovation studies (see, e.g., Magro and Wilson, Reference Magro and Wilson2013; Kivimaa and Kern, Reference Kivimaa and Kern2016), and the field of policy analysis (see, e.g., Howlett and Rayner, Reference Howlett and Rayner2007). However, thus far this academic debate surrounding policy mix designs has mainly focused on how combining different policy instruments increases the effectiveness of policies through the creation of positive synergies between measures (Erp and Huisman, Reference Erp and Huisman2010; Howlett, Reference Howlett2011; Howlett and Rayner, Reference Howlett and Rayner2013).
The study of the politics of policy packaging has received less attention (Howlett and Rayner, Reference Howlett and Rayner2013; Rogge and Reichardt, Reference Rogge and Reichardt2016; Schmidt and Sewerin, Reference Schmidt and Sewerin2019). An important gap in the literature on the politics of policy packaging concerns their effect on public opinion. Public opinion is an important driver of policy action, but also a constraint on the political feasibility of costly policies (Page and Shapiro, Reference Page and Shapiro1983; Stimson et al., Reference Stimson, MacKuen and Erikson1995; Burstein, Reference Burstein2003; Bernauer, Reference Bernauer2013; Anderson et al., Reference Anderson, Böhmelt and Ward2017; Stokes and Warshaw, Reference Stokes and Warshaw2017), particularly when issues become publicly salient (Culpepper, Reference Culpepper2010). Thus far, public opinion studies have primarily concentrated on analyzing citizens’ preferences for single policy measures (Fesenfeld, Reference Fesenfeld2020), but besides some notable exceptions (see below), they have not accounted for the reality of policy packaging.
The small number of empirical studies that have investigated the relationship between public opinion and packaging have primarily built on small-n, qualitative case studies in the field of transportation policy (see, e.g., Eriksson et al., Reference Eriksson, Garvill and Nordlund2008; Sørensen et al., Reference Sørensen, Isaksson, Macmillen, Åkerman and Kressler2014). A small-n experimental study by Milkman et al. (Reference Milkman, Mazza, Shu, Tsay and Bazerman2012) suggests that packaging could be an effective strategy for overcoming the loss aversion of individuals. Some more recent studies also examined the effects of policy packaging on public opinion in the field of pension, energy, transport, and food policies with larger survey samples (Häusermann et al., Reference Häusermann, Kurer and Traber2018; Ingold et al., Reference Ingold, Stadelmann-Steffen and Kammermann2019; Wicki et al., Reference Wicki, Fesenfeld and Bernauer2019, Reference Wicki, Huber and Bernauer2020; Bergquist et al., Reference Bergquist, Mildenberger and Stokes2020; Fesenfeld et al., Reference Fesenfeld, Wicki, Sun and Bernauer2020). However, these studies have not yet tested to what extent policy design complexity affects public opinion; that is, these studies did not directly compare the stability of individuals’ policy perceptions and preferences for packages in contrast to single policies.
Policy packaging affects public opinion through altering policy perceptions, compensation, and complexity
The emerging public opinion literature on policy packaging has primarily focused on the compensation mechanism to explain changes in public support. In the following, I extend this line of reasoning and outline why we should not only expect public opinion to change as a result of a compensation mechanism, but also a perception and design complexity mechanism.
Compensation mechanism
Based on the assumption that voters form their policy preferences in line with their perceptions of policy-induced costs and benefits, recent research has shown that policymakers can change public support for policy proposals by combining measures that voters perceive as beneficial with measures they perceive as costly (Häusermann et al., Reference Häusermann, Kurer and Traber2018; Jagers et al., Reference Jagers, Martinsson and Matti2019; Wicki et al., Reference Wicki, Fesenfeld and Bernauer2019, Reference Wicki, Huber and Bernauer2020; Bergquist et al., Reference Bergquist, Mildenberger and Stokes2020; Fesenfeld et al., Reference Fesenfeld, Wicki, Sun and Bernauer2020). This argument builds on a classical rational choice and utility maximization logic and assumes that voters consciously reason about their policy choices (Downs, Reference Downs1957; Becker, Reference Becker1976; Popkin, Reference Popkin1994). From this perspective, a policy's utility – that is, voters’ perception of the net sum of policy-induced costs and benefits – depends on the type of policy instrument (Drews and van den Bergh, Reference Drews and van den Bergh2016; Stadelmann-Steffen and Dermont, Reference Stadelmann-Steffen and Dermont2018) and its level of stringency (Schaffrin et al., Reference Schaffrin, Sewerin and Seubert2015; Pahle et al., Reference Pahle, Burtraw, Flachsland, Kelsey, Biber and Meckling2018). For example, citizens tend to perceive push-based measures like high environmental taxes as more costly and less effective than pull-based measures, such as subsidies for environmentally friendly alternatives (Drews and van den Bergh, Reference Drews and van den Bergh2016; Stadelmann-Steffen and Dermont, Reference Stadelmann-Steffen and Dermont2018; Huber et al., Reference Huber, Wicki and Bernauer2019; Wicki et al., Reference Wicki, Fesenfeld and Bernauer2019). Moreover, while stringent measures are usually effective at achieving environmental goals (Carley and Miller, Reference Carley and Miller2012; Rhodes et al., Reference Rhodes, Axsen and Jaccard2014), greater stringency can also reduce public support by increasing the perceived policy-induced (behavioral and monetary) cost, particularly when targeting consumers directly, rather than producers (Creutzig et al., Reference Creutzig, Roy, Lamb, Azevedo, Bruin, Dalkmann, Edelenbosch, Geels, Grubler, Hepburn, Hertwich, Khosla, Mattauch, Minx, Ramakrishnan, Rao, Steinberger, Tavoni, Ürge-vorsatz and Weber2018; Fesenfeld et al., Reference Fesenfeld, Wicki, Sun and Bernauer2020).
Most recent literature explains policy packaging effects on public opinion by referring to a compensation mechanism. Consequently, public support is believed to depend on the combination of policy measures. Policymakers can increase support if packages that include instruments perceived by citizens as costly simultaneously contain instruments perceived as beneficial. In essence, packaging can help to ‘pay off’ opponents for accepting undesired elements of a package (Cox and McCubbins, Reference Cox and McCubbins2005; Knox-Hayes, Reference Knox-Hayes2012; Milkman et al., Reference Milkman, Mazza, Shu, Tsay and Bazerman2012; Jacobs, Reference Jacobs2016, Reference Jacobs2011; Häusermann et al., Reference Häusermann, Kurer and Traber2018). Accordingly, I hypothesize the following:
H1: Public support for packages including climate policy instruments that induce costs for citizens is higher if these packages simultaneously include instruments that induce benefits.
Perception mechanism
In addition to the compensation mechanism, I here propose an additional mechanism through which policy packaging can affect public opinion: altered policy perceptions. The environmental policy design literature has highlighted the importance of citizens’ perceptions of policy effectiveness and restrictiveness (i.e., perceived intrusiveness in citizens’ personal lives) to explain public support for different isolated climate policy proposals. Climate policy with higher perceived effectiveness to mitigate climate change is argued to increase public support (Krosnick et al., Reference Krosnick, Holbrook, Lowe and Visser2006; Drews and van den Bergh, Reference Drews and van den Bergh2016; Huber et al., Reference Huber, Wicki and Bernauer2019), particularly for citizens that favor climate action (Bechtel and Scheve, Reference Bechtel and Scheve2013). In contrast, lower perceived effectiveness tends to decrease public support for climate policies (Bord et al., Reference Bord, O'connor and Fisher2000; Steg et al., Reference Steg, Dreijerink and Abrahamse2006; Drews and van den Bergh, Reference Drews and van den Bergh2016; Baranzini and Carattini, Reference Baranzini and Carattini2017; Stadelmann-Steffen and Dermont, Reference Stadelmann-Steffen and Dermont2018; Maestre-Andrés et al., Reference Maestre-Andrés, Drews and van den Bergh2019; Levi, Reference Levi2021). Moreover, Bostrom et al. (Reference Bostrom, O'Connor, Böhm, Hanss, Bodi, Ekström, Halder, Jeschke, Mack and Qu2012) and Stadelmann-Steffen and Dermont (Reference Stadelmann-Steffen and Dermont2018) show that people often have misperceptions about the effectiveness of policies and use these (mis-)perceptions to justify their (non) support for specific climate policies.
Similarly, the degree of a policy's restrictiveness or level of intrusiveness in citizens’ personal lives is an important predictor of public support (Cherry et al., Reference Cherry, Kallbekken and Kroll2012; Huber et al., Reference Huber, Wicki and Bernauer2019; Wicki et al., Reference Wicki, Fesenfeld and Bernauer2019; Fesenfeld et al., Reference Fesenfeld, Wicki, Sun and Bernauer2020). More restrictive or coercive policies are generally perceived as more costly for individuals (ego-tropic concern) and other members of society (socio-tropic concern). They thus receive less support than policies that are perceived to be less restrictive (Cherry et al., Reference Cherry, Kallbekken and Kroll2012; Huber et al., Reference Huber, Wicki and Bernauer2019; Wicki et al., Reference Wicki, Fesenfeld and Bernauer2019; Fesenfeld et al., Reference Fesenfeld, Wicki, Sun and Bernauer2020). Generally, more stringent policy instruments are also perceived to be more restrictive, but often also more effective at mitigating climate change (Cherry et al., Reference Cherry, Kallbekken and Kroll2012; Huber et al., Reference Huber, Wicki and Bernauer2019; Wicki et al., Reference Wicki, Fesenfeld and Bernauer2019; Fesenfeld et al., Reference Fesenfeld, Wicki, Sun and Bernauer2020). The existing literature, however, has not yet studied the effects of policy packaging on policy perceptions.
Here, I put forward that combining multiple policies into packages can alter perceptions of policy effectiveness and restrictiveness because individuals evaluate policy proposals in comparison to other policy options (i.e., change their frame of reference). On the one hand, I argue that adding more policy instruments to a package leads individuals to perceive a proposal as more effective because of a simple ‘more is better’ heuristic. The premise here is that individuals think that multiple policy measures address different elements of the climate issue, create positive synergies, and are thus in combination more effective than in isolation at reducing climate pollutants. On the other hand, I argue that adding policy instruments to a package also leads people to perceive policy proposals as more restrictive, because each policy instrument added to a package is perceived to restrict citizens’ personal lives to some degree.
Accordingly, I hypothesize the following:
H2a: On average, citizens perceive climate policy packages including different instruments as more effective than proposals for the same instruments made in isolation.
H2b: On average, citizens perceive proposed climate policy packages including different instruments as more restrictive than proposals for the same instruments made in isolation.
Complexity mechanism
In addition to the perception and compensation mechanisms, I here argue that the complexity of proposed policy designs per se can also affect public opinion. This argument is in line with bounded rationality theory (Simon, Reference Simon1957; Green and Shapiro, Reference Green and Shapiro1996; Jones, Reference Jones1999; Kahneman, Reference Kahneman2003) and political communication research which has highlighted the importance of heuristics in complex decision situations (Zaller, Reference Zaller1992; Druckman, Reference Druckman2004; Chong and Druckman, Reference Chong and Druckman2007a).
Increased information complexity is a key process through which proposed policy designs could affect public opinion. Per definition, increasing policy design complexity implies that new policy instruments added to a policy package simultaneously increase the cognitive burden on individuals and can lead to information satisficing (Krosnick, Reference Krosnick1999; Bansak et al., Reference Bansak, Hainmueller, Hopkins and Yamamoto2018, Reference Bansak, Hainmueller, Hopkins and Yamamoto2019). Generally, humans tend to be able to process a maximum of nine pieces of information at a time (Miller, Reference Miller1994). In information-rich situations, individuals often engage in more unconscious decision-making processes (Wason and Evans, Reference Wason and Evans1974; Petty and Cacioppo, Reference Petty and Cacioppo1986; Chaiken and Trope, Reference Chaiken and Trope1999; Kahneman, Reference Kahneman2003). In line with bounded rationality theory (Simon, Reference Simon1957), information satisficing occurs when respondents are faced with an amount of information that they cannot fully process, and hence use cognitive heuristics (Slovic, Reference Slovic1995; Kahneman, Reference Kahneman2003; Lichtenstein and Slovic, Reference Lichtenstein and Slovic2006) to simplify decision-making and cope with this information complexity (Krosnick, Reference Krosnick1999; Bansak et al., Reference Bansak, Hainmueller, Hopkins and Yamamoto2019; Jenke et al., Reference Jenke, Bansak, Hainmueller and Hangartner2021).
For example, the tax instrument may serve as a decision heuristic for respondents in complex choice situations. Depending on individuals’ ideological points of view, including a tax instrument into a policy package might lead to automatic opposition or support of the package. In these situations, the support effects on the proposed package of the other included instruments would become relatively smaller. Because individuals hold different ideological priors, citizens are likely to use different instrument types (not only taxes) as their decision heuristics in complex situations. Yet, according to prospect theory (Kahneman and Tversky, Reference Kahneman and Tversky1979; Tversky and Kahneman, Reference Tversky and Kahneman1992), loss aversion predicts that citizens focus more on costs than on equivalent benefits. Arguably, costly and restrictive instruments, such as taxes and consumer restrictions, are hence politically more controversial and salient. Thus, one can expect that on average such costly and more controversial instruments serve more often as decision heuristics in complex decision situations compared to the less controversial instruments.
Overall, I thus argue that the policy design complexity mechanism affects the evaluation of individual policy instruments included in complex packages because citizens’ focus of attention is altered when forming their policy preferences. Accordingly, I hypothesize the following:
H3a: The public support effects of policy instruments proposed as part of complex policy packages are smaller than the support effects of the same instruments proposed in isolation.
H3b: This effect difference is larger for those instruments that citizens perceive as less costly.
Methods
To examine the effects of policy design complexity and packaging on public opinion, I designed conjoint choice experiments in two democratic countries. The experiments were embedded in quota-based, representative, and non-probability online public opinion surveys in Germany and the USA (N = 9115; see details in Supplementary Material, pp. 3 and 4, Supplementary Tables 1 and 2). The survey was conducted through Lightspeed Research (Kantar TNS group), a leading company for online surveys. A matching algorithm was applied to these panels to obtain (non-probability based) samples that are representative for core sociodemographic variables (age, gender, income) of the population of eligible voters aged 18 years or older in Germany and the USA. In a choice-based conjoint experiment, individuals are given the choice between two different proposals with a number of attributes; in this case, different policy instruments (Hainmueller et al., Reference Hainmueller, Hopkins and Yamamoto2014). This allows the researcher to nonparametrically estimate the average marginal component effect (AMCE); that is, the estimated marginal effect of a discretely valued attribute averaged over the joint distribution of the remaining attributes (Hainmueller et al., Reference Hainmueller, Hopkins and Yamamoto2014). Given the randomization and the resulting orthogonality of each attribute with respect to every other one, the estimation of treatment effects is straightforward. In this study, the AMCE thus denotes the average marginal effect on perceptions of and support for a policy proposal when specific policy instruments are included in a package.
Choice-based conjoint experiments are particularly useful for studying the causal effects of multiple variables in complex decision-making processes and, given the realistic trade-off situation between choice options, generate higher external and ecological validity than simple stated-preference measures (Hainmueller et al., Reference Hainmueller, Hangartner and Yamamoto2015; Bansak et al., Reference Bansak, Hainmueller and Hangartner2016). I employed a conjoint-based experiment that assigned individuals to evaluate and choose between randomly designed packages with varying degrees of policy design complexity (i.e., varying numbers of policy instruments were included in the packages – see study design in Figure 2). Previous studies have not directly compared choices across different levels of policy design complexity (Häusermann et al., Reference Häusermann, Kurer and Traber2018; Jagers et al., Reference Jagers, Martinsson and Matti2019; Wicki et al., Reference Wicki, Fesenfeld and Bernauer2019; Fesenfeld et al., Reference Fesenfeld, Wicki, Sun and Bernauer2020).Footnote 2
The survey experiment was structured as follows: Before citizens evaluated two randomly designed policy proposals side-by-side, I briefly described the different climate policy proposals intended to reduce meat consumption or the use of cars that run on fossil fuels (see detailed policy description texts in Supplementary Material, pp. 28ff.). Based on a review of the literature (Bajželj et al., Reference Bajželj, Richards, Allwood, Smith, Dennis, Curmi, Gilligan, Lee-gammage, Baj, Richards, Allwood, Smith, Dennis, Curmi and Gilligan2014; Poore and Nemecek, Reference Poore and Nemecek2018; Springmann et al., Reference Springmann, Clark, Mason-D'Croz, Wiebe, Bodirsky, Lassaletta, de Vries, Vermeulen, Herrero and Carlson2018), and six explorative expert interviews (see details in Supplementary Material, p. 32), I selected four policy instruments that respondents evaluated one after the other. The four policy measures correspond to classical policy types, that is, market- versus regulatory-based, and push versus pull measures (Howlett, Reference Howlett2011). The four policy measures are: (1) government financial support for low-emission products in the food and transport sector; (2) emission standards and environmental regulations for meat and car producers; (3) taxes on meat and fossil fuels; and (4) restrictions for public cafeterias concerning which days they may offer meat, and banning cars that run on fossil fuels from city centers on specific days of the week. All four policy measures vary in terms of levels of policy stringency [i.e., the increase in policy ambitiousness compared to the status quo (Pahle et al., Reference Pahle, Burtraw, Flachsland, Kelsey, Biber and Meckling2018): no change to the status quo, a medium level of policy stringency, or a high level of stringency], and in line with previous research (Stadelmann-Steffen and Dermont, Reference Stadelmann-Steffen and Dermont2018; Huber et al., Reference Huber, Wicki and Bernauer2019; Ingold et al., Reference Ingold, Stadelmann-Steffen and Kammermann2019; Fesenfeld et al, Reference Fesenfeld, Wicki, Sun and Bernauer2020) which are likely to be perceived as either costly or beneficial by respondents (see more details in Figure 1). Furthermore, the policy goals and the corresponding policy instruments were randomly varied between reducing the use of cars that run on fossil fuels and reducing meat consumption. The obvious condition during the randomization process was that policy instruments related to the food case (transport case, resp.) were only shown when the policy goal was randomly selected to be the reduction of meat consumption (car use, resp.).

Figure 1. Conjoint Experimental Attribute Features. I expect the green-shaded instruments to have a positive effect on public support and compensate for the negative effects on public support of the red-shaded instruments. A majority of citizens are likely to perceive the green-shaded instruments as materially or immaterially beneficial (desired), while the red-shaded instruments as materially or immaterially costly (undesired) (see more in Stadelmann-Steffen and Dermont, Reference Stadelmann-Steffen and Dermont2018; Huber et al., Reference Huber, Wicki and Bernauer2019; Ingold et al., Reference Ingold, Stadelmann-Steffen and Kammermann2019; Fesenfeld et al, Reference Fesenfeld, Wicki, Sun and Bernauer2020). The expectation is that the higher stringency of green-shaded instruments will increase perceived policy benefits and hence public support, while the higher stringency of red-shaded instruments will increase perceived policy costs and hence decrease public support.
To test how much individuals’ policy perceptions and preferences vary as a function of the level of policy design complexity, I created a two-stage randomization design.
At the first stage (see Figure 2 for details), I presented each study participant (N = 4228 in Germany; N = 4876 in the USA) with four pairs of randomly designed policy proposals of low complexity (i.e., without packaging different instruments together). These low-complexity proposals consisted of two attributes, namely a policy goal and one of the four individual policy instruments. The different policy instruments were described to respondents prior to the four choice tasks (see details in Supplementary Material, pp. 29ff.). Respondents were asked to decide which proposal they preferred within each pair. Besides this forced-choice question, all respondents also rated their support for each proposal on a seven-point Likert scale. In addition, individuals rated the perceived effectiveness at reducing climate pollutants of each policy proposal, as well as perceived policy restrictiveness on a seven-point Likert scale.

Figure 2. Experimental Study Design – Stage I: In addition to the outcome choice and support ratings, respondents expressed their (dis-)agreement with the two statements for each of the two packages on a seven-point Likert scale [from ‘Strongly disagree’ (1) to ‘Strongly agree’ (7)]: Statement 1: ‘Policy-package A/B is effective at protecting the climate’; Statement 2: ‘Policy-package A is restrictive in terms of my lifestyle.’
In the second stage (see Figure 3 for details), a sub-sample of randomly selected respondents (N = 1409 in Germany; N = 1624 in the USA) was assigned to a high-complexity conjoint task with five conjoint attributes, including a policy goal and packaging all of the four policy instruments. Again, the design of the pairs of policy packages was varied randomly. Respondents received four pairs of such randomly sorted policy packages and were asked to choose one of the two proposals within each pair in a forced-choice question, and also to rate their support and policy perceptions of each proposal on a seven-point Likert scale.
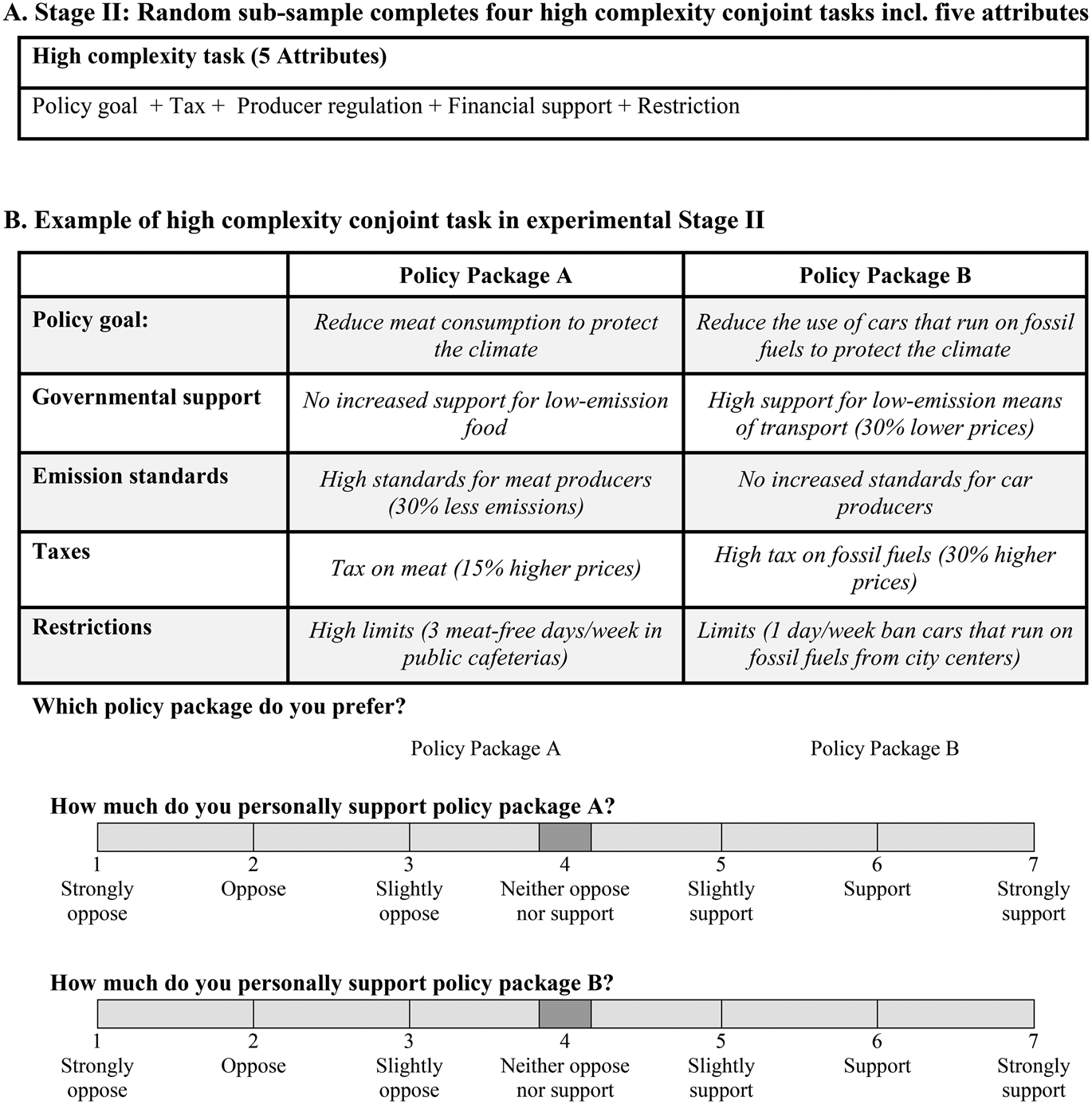
Figure 3. Experimental Study Design – Stage II: In addition to the outcome choice and support ratings, respondents expressed their (dis-)agreement with the two statements for each of the two packages on a seven-point Likert scale [from ‘Strongly disagree’ (1) to ‘Strongly agree’ (7)]: Statement 1: ‘Policy-package A/B is effective at protecting the climate’; Statement 2: ‘Policy-package A is restrictive in terms of my lifestyle.’
Both the full sample (N = 9115) for the first stage and the sub-sample of randomly selected respondents for the second stage are representative of the voting age populations in both countries (see details in Supplementary Material, pp. 3and 4, Supplementary Tables 1 and 2). The units of analysis were randomly designed policy proposals, and the primary issue of interest was the effect of policy packaging on policy perceptions and public support for these proposals in the two design stages:
First, to test H1, I investigated the AMCEs of including a specific policy instrument into a package on support for a policy proposal. Following the standard nonparametric estimation approach proposed by Hainmueller et al. (Reference Hainmueller, Hopkins and Yamamoto2014), the AMCEs were estimated by least-squares regressions, wherein the proposal attributes were entered as a series of dummy variables. Standard errors were clustered by respondents to account for autocorrelation (Hainmueller et al., Reference Hainmueller, Hopkins and Yamamoto2014).
Second, to test H2a/H2b, I estimated the average marginal effects of proposing a policy package compared to an isolated policy on respondents’ perceived policy effectiveness and restrictiveness. To do this, I used least squares regressions with clustered standard errors to regress the policy effectiveness and restrictiveness ratings on the variable that indicated if the respective policy proposal was made in isolation (i.e., stage 1, low-complexity condition) or in combination (i.e., stage 2, high-complexity condition). Given the fact that exactly the same policy instruments were assessed during the two experimental stages, this approach allowed me to identify the effect of packaging on policy perceptions independent of policy attribute levels.
Third, to test H3a/H3b, I identified the change between estimated AMCEs for specific policy instruments as a function of the number of policy instruments included in a package. In other words, I studied if the size and direction of the AMCEs for a specific policy instrument differed if assessed in isolation (i.e., stage 1) or in combination with other policy instruments (i.e., stage 2).
Even if not essential for testing the theoretical arguments made in this paper, for increasing the policy relevance I have included in the appendix (Supplementary Material, pp. 19ff., Supplementary Figures 1–8) additional interaction analyses that show how the two policy goals and the different policy instruments potentially interact.
Results
In the following, I first buttress existing arguments that packaging affects public support via a compensation mechanism (H1). In the next step, I present original evidence about the two novel arguments made in this study that packaging affects policy perceptions (H2a/H2b) and can alter preferences via an increase in policy design complexity (H3a/H3b).
Policy packaging affects public opinion via a compensation mechanism
Figure 4 presents the AMCEs for the high-complexity tasks (see detailed regression results in Supplementary Material, pp. 5 and 7, Supplementary Tables 3 and 5). Results show that the AMCEs for some attributes are substantial in a negative direction, while others are substantial in a positive direction. For example, the estimated effect of high taxes being included in a policy package on the probability of choosing that package is significantly negative [Germany: 8.9 percentage points (SE = 1.2) lower choice probability; USA: 18.2 percentage points (SE = 1.1) lower choice probability], while the estimated effect for including governmental support for low-emission product alternatives is significantly positive [Germany: 14.1 percentage points (SE = 1.1) higher choice probability; USA: 7.8 percentage points (SE = 1.1) higher choice probability]. Furthermore, the inclusion of strong consumption restrictions reduces the choice probability for a policy package in both countries by around 5–7 percentage points (SE = 1.1). Interestingly, the choice effects of the consumption restriction attribute are, however, not monotonic – that is, while strong restrictions slightly reduce the choice probabilities in both countries, weaker restrictions do not have a significant negative effect. The presence of high producer standards increases choice probability in Germany by 9.4 percentage points (SE = 1.2) and in the USA by 4.5 percentage points (SE = 1.1). In line with the literature, the results thus buttress the first hypothesis (H1) that public support for proposed policy packages including undesired climate policy instruments is higher if these packages simultaneously include desired instruments.

Figure 4. Estimated AMCEs of policy instruments (in high-complexity task) on the probability of choosing a package. The zero line denotes the baseline category of ‘No change to the status quo.’ For the policy goal, the reference category is ‘Reduction of cars that run on fossil fuels.’ Standard errors are clustered by respondents. The figure depicts 95% confidence intervals.
The first hypothesis receives further support when taking a closer look at predicted choice probabilities (see Supplementary Material, p. 27, Supplementary Figure 9). The predicted choice probabilities of policy packages clearly depend on the exact combination of instruments included in the package. Both for Germany and the USA, we can identify a wide range of choice probabilities for differently designed policy packages that include a specific policy instrument (see Supplementary Figure 9). For example, in Germany, a package that includes a high tax on fossil fuels is chosen by 59% of respondents if the package also includes strong support for low-emission modes of transportation, a strong increase in emission standards for car producers, and no limits on accessing city centers with fossil-fueled cars. On the other hand, a package that includes a high tax on fossil fuels but no compensatory measures only receives 30% support.
Policy packaging affects public opinion via a perception mechanism
The second set of hypotheses postulate that, on average, citizens perceive proposed climate policy packages including different instruments as more effective (H2a) and restrictive (H2b) than proposals for the same instruments made in isolation. The results presented in Figure 5 support this expectation (see regression results in Supplementary Tables 15–18). The findings show that both in Germany and the USA, respondents rate policy package proposals as significantly more restrictive (in Germany by 0.13 points; in the USA by 0.16 points on a seven-point Likert scale) and more effective (in Germany by 0.12 points; in the USA by 0.08 points on a seven-point Likert scale) than isolated policy proposals (baseline). All effects, except the effect on perceived effectiveness in the USA, are significant at the 95% confidence level. The results thus show that whether policies are presented in isolation or in combination can significantly alter respondents’ policy perceptions. These findings are also supported by comparing AMCEs on policy perceptions in the two experimental stages (i.e., low- and high-complexity conditions). As shown in the appendix (Supplementary Material, pp. 9–16, Supplementary Tables 7–14), packaging not only affects average ratings, but also the size of AMCEs.

Figure 5. Estimated average marginal effects of policy packaging on respondents’ perceptions of policy restrictiveness and policy effectiveness. The zero line denotes the baseline category of policies proposed in isolation (low-complexity condition). The points indicate the estimated average marginal effect of policy packaging (high-complexity condition) on the rating of perceived restrictiveness and effectiveness measured on seven-point Likert scales. Standard errors are clustered by respondents. The figure depicts 95% confidence intervals.
Policy packaging affects public opinion via a complexity mechanism
Turning to the third set of hypotheses, I first expect that public support effects of policy instruments proposed as part of complex policy packages are significantly smaller than the public support effects of the same instruments proposed in isolation (H3a).
Figure 6 presents the estimated AMCEs for when a policy instrument is evaluated in isolation (i.e., stage 1, low-complexity condition) or as part of a policy package (i.e., stage 2, high-complexity condition) (see detailed regression results in Supplementary Material, pp. 5–8, Supplementary Tables 3–6). The plot clearly shows that, on average, the size of the estimated attribute effects (AMCEs) is smaller when citizens choose policies contained in a package rather than in isolation.

Figure 6. Estimated AMCEs of policy attributes on the choice probability for proposals in low (purple) versus high-complexity (orange) conditions. The zero line denotes the baseline category of ‘No change to the status quo.’ For the policy goal, the reference category is ‘Reduction of cars that run on fossil fuels.’ Standard errors are clustered by respondents. The figure depicts 95% confidence intervals.
For instance, a large tax increase, if evaluated in isolation (i.e., in a low-complexity condition), reduces the choice probability of a policy proposal by 15.0 percentage points in Germany (SE = 1.5) and 27.2 percentage points in the USA (SE = 1.3). In contrast, a large tax increase evaluated as part of a policy package (i.e., high-complexity condition) reduces the choice probability significantly less – by only 8.9 percentage points in Germany (SE = 1.2) and 18.2 percentage points in the USA (SE = 1.1).
Moreover, in line with hypothesis H3b, the differences in AMCEs between the high- and low-complexity conditions are smaller for instruments perceived as costly by citizens. Arguably, citizens perceive the tax and restriction instruments as personally more costly and ideologically more controversial than support for low-emission products and producer emission standards (see Figure 1). Indeed, Figure 6 shows that the difference in AMCEs between the high- and low-complexity conditions for the tax and restriction instruments is smaller than the difference in AMCEs for the other two instruments, namely the support and producer standard instruments. Thus, it is more likely that, on average, respondents focus their attention on these two instruments rather than on the less costly support and standard instruments. In other words, this finding supports the expectation grounded in prospect theory (loss aversion) that in high-complexity situations, respondents focus particularly on costly and controversial instruments as heuristics to reduce cognitive burden.
Overall, the empirical results are in line with bounded rationality theory and confirm the third set of hypotheses that public support effects of policy instruments proposed as part of complex policy packages are significantly smaller than the public support effects of the same instruments proposed in isolation.
Discussion of compensation, perception, and complexity mechanisms
Finally, I briefly discuss the key contribution of this paper – i.e., that policy packaging affects public opinion not only via a compensation mechanism but also via a perception and complexity mechanism.
First, the clear implication of the compensation mechanism is that the predicted choice probabilities of policy packages vary as a function of the combination of policy instruments that are included therein. As shown in Figure 4 (and Supplementary Figure 9), the negative effects on the public support of undesired climate policy instruments can be compensated for by combining these instruments with desired instruments. In other words, in line with the burgeoning literature on policy packaging and public opinion, I find clear evidence that compensation effects can substantially affect and increase public support for ambitious climate policies with perceptible behavioral implications.
Second, this paper provides the first empirical evidence that policy packaging also affects public opinion via a perception mechanism. As outlined in Figure 5, respondents perceive policy package proposals as significantly more effective at combatting climate change, but also as more restrictive in terms of their personal lifestyles. This finding adds to the current policy design and public opinion literature by highlighting that policy perceptions depend on proposing policies in isolation or combination (see further details in Supplementary Tables 8–18).
Third, the effect of the design complexity mechanism is that the AMCEs for different policy instruments evaluated as part of a package (i.e., in the high-complexity condition) are significantly smaller compared to the AMCEs for the same policies when evaluated in isolation (i.e., in a low-complexity condition). If policy design complexity did not matter, we would expect no significant differences between the AMCEs in the two complexity conditions. As illustrated in Figure 6, however, we can observe substantially different effect sizes between the two complexity conditions.
In sum, the presented findings permit the conclusion that policy packaging affects public opinion through a compensation, perception, and complexity mechanism. In this regard, an important observation, however, is that both positive and negative effects of policy instruments on public support are smaller when such policies are proposed as part of the policy package (i.e., in the high-complexity condition) rather than in isolation (i.e., in the low-complexity condition). However, especially for costly and presumably more controversial instruments, such as high taxes and consumer restrictions, the differences between the low- and high-complexity conditions are smaller than for less costly policies. Thus, in high-complexity choice situations respondents tend to use the costly parts of policy packages as decision heuristics. The increased complexity thus seems to affect less controversial instruments to a larger degree. Future research should investigate this result in more depth (see further details in Supplementary Material, pp. 34–38).
While respondents’ capacity to form policy preferences can be bounded by an increase in design complexity, the higher information load does not lead citizens to fundamentally (and arguably, irrationally) reverse the overall direction of their preferences. In other words, citizens maintain their general pattern of preferences and their ability to employ a compensation logic when forming their policy attitudes. This finding is in line with the literature about the limits of framing in relation to manipulating citizens’ political attitudes (Druckman, Reference Druckman2004, Reference Druckman2001; Chong and Druckman, Reference Chong and Druckman2007b; Bechtel et al., Reference Bechtel, Hainmueller, Hangartner and Helbling2015). Especially with respect to salient and contested policy issues with perceptible implications for citizens’ everyday lives, such as transformative climate policies that affect peoples’ consumption habits, one may expect citizens to have more stable and consciously formed preferences (Fesenfeld et al., Reference Fesenfeld, Sun, Wicki and Bernauer2021b). Overall, the present results thus underline the crucial importance of combining costly and compensatory measures to increase support for transformative climate policies.
Comparing results across countries
Comparing the results across the two country samples shows that, taken as a whole, the results related to all three mechanisms are comparable across the two countries. In both countries, combining costly and compensatory measures leads to higher support for policy proposals (H1), packaging affects policy perceptions (H2a/H2b), and AMCEs for policies integrated into packages are smaller than when policies are evaluated in isolation (H3a). In both countries, the difference in AMCEs between the high- and low-complexity conditions is also smaller for the more costly instruments (taxes and restrictions) compared to the less costly instruments (H3b).
However, we also observe some interesting differences between Germany and the USA. First, while most policy instruments integrated into packages affect public support to a similar degree in both countries, in line with previous research (Klenert et al., Reference Klenert, Mattauch, Combet, Edenhofer, Hepburn, Rafaty and Stern2018; Beiser-McGrath and Bernauer, Reference Beiser-McGrath and Bernauer2019), high taxes have a particularly large negative support effect in the USA (minus 18.2 percentage points) compared to Germany (minus 8.9 percentage points) (see Figure 4). Second, while in Germany policy packaging increases citizens’ perceived policy effectiveness and restrictiveness, in the USA packaging only increases perceived policy restrictiveness but not effectiveness (see Figure 5). This result echoes previous climate policy research that indicates the special sensibility of US citizens toward restrictions on personal liberties (Drews and van den Bergh, Reference Drews and van den Bergh2016; Dunlap et al., Reference Dunlap, McCright and Yarosh2016; Harring et al., Reference Harring, Jagers and Matti2019; Fesenfeld, Reference Fesenfeld2020). Third, generally speaking, in Germany the differences between AMCEs of policy instruments assessed in isolation (low-complexity condition) versus combination (high-complexity condition) are larger than in the USA (see Figure 6). One potential interpretation of this result is that in Germany climate policies are less polarized and AMCEs of policies evaluated in isolation are hence, on average, substantially larger than in the USA. Thus, also the differences between AMCEs in the low- and high-complexity conditions in Germany are more likely to be larger than in the USA.
Conclusion and future research
In reality, many policy problems are complex and require transformative policy responses that take the form of holistic policy packages. Nevertheless, most public opinion research has studied public support for single policy instruments, while it remains unclear if the complexity of proposed policy designs and combining different policy instruments affects public opinion. This study uses a novel, two-stage conjoint experimental design that assigns German and US survey respondents to choose between randomly designed policy packages with varying degrees of policy design complexity and various combinations of differently stringent policy instruments. The study provides the first systematic, cross-country, and large-n experimental evidence about the effects of policy design complexity and packaging on public opinion about transformative climate policies. This study thereby contributes to the burgeoning literature on policy design and public opinion about climate change specifically, and on policy preference formation more generally. While studies have thus far primarily argued that policy packaging affects public opinion via a compensation mechanism, this study proposes two additional mechanisms: a perception mechanism and a design complexity mechanism.
By comparing citizens’ preferences for proposed policies evaluated in isolation and combination, the study shows that packaging affects public opinion and can increase public support for ambitious but complex climate policies that have the potential to transform socio-technical systems in line with international mitigation targets. Importantly, such packaging effects are driven not only by a compensation mechanism but also by a policy perception and complexity mechanism. While both changes in policy perceptions and increased design complexity can shift public opinion, the results also suggest that compensation is still a key mechanism for shifting public support.
While recent studies have found relatively stable AMCEs in complex conjoint experiments (Bansak et al., Reference Bansak, Hainmueller, Hopkins and Yamamoto2018, Reference Bansak, Hainmueller, Hopkins and Yamamoto2019; Jenke et al., Reference Jenke, Bansak, Hainmueller and Hangartner2021), in line with models of bounded rationality and framing theories this study shows that the level of design complexity can reduce support effects of instruments included in packages. This effect of smaller AMCEs in more complex decision tasks is particularly strong in respect to the less costly instruments. Thus, in more complex decision situations citizens seem to focus more on the costly and controversial design elements.
This highlights that both intuition and reasoning guide information processing, particularly in complex decision-making environments. Nevertheless, the results presented here also show that increased policy design complexity does not fundamentally reverse citizens’ policy preferences.
Therefore, a key finding of this study is that policy design complexity itself is unlikely to fundamentally shift public opinion about ambitious climate policies. Facing information-rich and complex proposals for policy packages, respondents slightly adapt their policy perceptions but still apply a compensation logic when forming policy preferences. In other words, carefully combining costly and compensatory policy instruments is still crucial for increasing public support for transformative climate policies with perceptible behavioral implications for peoples’ everyday lives. Given the apparent stronger focus of citizens on costly policies in more complex packages, it is however essential that policymakers particularly emphasize the beneficial design elements to compensate for the negative support effects.
More generally, this study contributes to a growing literature on the limits of framing in relation to manipulating citizens’ policy attitudes and behaviors (Reference DruckmanDruckman, Reference Druckman2001, 2004; Chong and Druckman, Reference Chong and Druckman2007b; Bechtel et al., Reference Bechtel, Hainmueller, Hangartner and Helbling2015; Amsalem and Zoizner, Reference Amsalem and Zoizner2020; Fesenfeld, Reference Fesenfeld2020). Especially with respect to salient climate policies that have perceptible effects on peoples’ consumption behaviors, citizens are likely to have more stable preferences and simple framing effects might thus be limited (Bechtel et al., Reference Bechtel, Hainmueller, Hangartner and Helbling2015; Fesenfeld et al., Reference Fesenfeld, Sun, Wicki, Beiser-McGrath and Bernauer2021a, Reference Fesenfeld, Sun, Wicki and Bernauer2021b).
One central limitation of this study is that it does not directly compare the public opinion effects of proposals for complex policy designs across issue areas with more and less perceptible cost implications for citizens’ everyday lives. Future research should hence validate the findings of this study across different more or less salient issue areas, also accounting for potential logrolling effects across policy issue communities. Moreover, research could test how public opinion and interest group preferences interact with regard to such complex cross-issue ‘omnibus packages.’
It would also be useful to conduct further experiments that randomly vary the number of instruments in policy packages to assess the potentially non-monotonic effects of policy design complexity. Moreover, in addition to increasing information complexity, higher policy design complexity might also shift citizens’ relative reference points during the decision-making process (so-called choice bracketing effects). Future research should investigate to what extent information complexity and choice bracketing are empirically distinguishable from each other. In the appendix (see Supplementary Material, pp. 34–38), I derive hypotheses on potential choice bracketing and outline in more detail how future experiments could go beyond the limitations of this study to disentangle information complexity and choice bracketing. Such future studies could build on recent advances in combined eye-tracking and conjoint experimental methods (Jenke et al., Reference Jenke, Bansak, Hainmueller and Hangartner2021).
Moreover, further field experimental, panel, and comparative qualitative research should buttress the ecological and external validity of this study's findings. In the real world, journalists, politicians, and organized interest groups are likely to cherry-pick and deliberately frame the public debate around specific policy instruments, rather than address the full complexity of proposed policy packages. In such situations, the effect of policy design complexity and packaging on public opinion may differ from results obtained in the more sterile environment of a survey-embedded experiment.
Overall, the results show that there appear to be certain opportunities but also clear limits to the extent that elites can shift citizens’ policy perceptions and preferences through increasing policy design complexity and obscuring policy-induced costs. In contrast to such simple communication strategies, careful policy package designs that account for compensation effects are key to increasing public support for transformative climate policies. The study calls for more cautious reflection on the standard methods for studying public opinion and policy preferences. In the real world, many policy instruments are not adopted in isolation but are part of complex policy packages. This fact should be reflected in future public opinion research across different issue areas. Accordingly, I hope the present study contributes to a fruitful debate about how policy design complexity and packaging can influence public opinion.
Supplementary material
To view supplementary material for this article, please visit https://doi.org/10.1017/bpp.2022.3.
Acknowledgments
The author is grateful to Brilé Anderson, Thomas Bernauer, Gracia Brückmann, Liam F. Beiser-McGrath, Florian Egli, Dominik Hangartner, Regula Hess, Robert Huber, Dennis Kolcava, Quynh Nguyen, David Presperger, Adrian Rinscheid, Lukas Rudolph, Nicholas Schmid, Sebastian Sewerin, Michael L. Wicki, Franziska Quoss, and Karin Ingold; to two anonymous reviewers; and to the participants of the Swiss Political Science Conference 2020 and the Swiss-German Political Methodology Conference 2019 for their support and comments.