Introduction
Population dynamics, regional development and social disparities are interconnected issues in the urban history of advanced economies. From a morphological perspective, the progressive shift from mono-centric structures – typical of the post-war cities – to polycentric settlement models is a characteristic of long-term urbanization processes, especially in the old continent.Footnote 1 From a functional perspective, socio-economic transformations at the heart of settlement expansion were dependent on increasingly divergent patterns of regional development. When there is an understanding of the intrinsic nature of socio-spatial structures, the identification and characterization of metropolitan cycles can benefit from a broad range of approaches and methodologies.Footnote 2
Theoretical and operational frameworks based on the notion of the city life cycle (CLC) – a classical approach to urban growth adopted in economic, geographical and historical studies – suggest that it is possible to identify distinctive waves of metropolitan development based on a quantitative analysis of target variables assessing the evolution of urban, suburban and rural locations over time. While providing a simplistic interpretation of urban cycles, the intrinsic focus of the CLC theory on sequential stages of growth is appropriate when investigating long-term mechanisms of metropolitan expansion. The role of a broad set of socio-economic forces acting as the intrinsic ‘engine’ of urban growth has also been debated in recent literature.Footnote 3 If we assume that long-term metropolitan development is a result of sequential transformations, where intensity and spatial direction depend on past dynamics, it is clear that urbanization paths have more recently been associated with push and pull factors delineating stable stages of growth and abrupt shifts toward another steady state with different conditions for equilibrium.Footnote 4
Despite the inherent complexity of metropolitan transformations in advanced economies, spatial heterogeneity in population dynamics has occasionally been considered key to understanding the historical path of regional growth,Footnote 5 although path-dependent regulation of urban expansion has been demonstrated to affect population trends in some cases.Footnote 6 While comparative investigations of local-scale demographic dynamics have helped to shed light on the emerging complexity of urbanization patterns and processes, few such analyses have been undertaken in Europe, and especially in Mediterranean locations, where individual countries and regions exhibit different traits derived from their intrinsic structure, history and political/cultural background.
To test path-dependent urban growth and the role of territorial heterogeneity in demographic patterns and processes, we compared the outcome of spatially explicit (global) econometric models with Geographically Weighted (local) Regressions (GWRs). These models predicted population growth rates as a function of (i) a lagged variable reflecting short-term population dynamics in the previous time interval, (ii) the initial level of population density, (iii) a set of ancillary variables quantifying economic and non-economic drivers of growth and (iv) space.
This approach has informed the spatially explicit investigation of long-term mechanisms of population growth and decline in metropolitan Athens (Greece) performed in our study and covering the last century (1920–2021), using municipal units as the elementary analysis domain.Footnote 7 Athens reflects the secular development trajectories characteristic of several Mediterranean cities,Footnote 8 and settlement expansion in the last century is a result of multiple historical events with distinctive economic and social impacts. One is the progressive shift from an industry-based to a service-oriented economy and the socio-political turbulence it brought. One consequence of the Turkey–Greece War of 1911 was the greatest influx of refugees from Asia Minor that the city has ever seen.Footnote 9 More recent population trends in Athens (low fertility, ageing and steady immigration) can also be observed in other cities in the Northern Mediterranean basin.Footnote 10
In this context, the notion of ‘path dependence’ – applied extensively and broadly discussed in the social sciences, from economics to geography and history – lends itself to innovative interpretations of socio-economic transitions. Assuming a mono-centric structure that resembles the (dominant) spatial organization of the study area, we have adopted an approach based on evolutionary economics in order to shed light on the transformation of urban, suburban and rural districts within a metropolitan region, to evaluate the role of path dependence in their growth trajectories, and to identify the factors that influence their developmental stages. We can demonstrate a growth pattern which changes radically over time, and is influenced by background conditions at the beginning of the study period and by heterogeneous capabilities cultivated by local communities.Footnote 11 We can thus contribute both to urban history and regional science.
The article is organized as follows: the first section consists of a literature review of metropolitan cycles and path-dependent urban growth, informed by multiple economic, geographical and historical perspectives. The second section describes the econometric strategy, the results of which are illustrated in the third section. The main findings of this study are discussed in the fourth section, where some policy implications for planning future development paths of Mediterranean cities are outlined. The fifth section concludes the article by commenting on the originality of our approach, and delineating future approaches to path-dependent complexity in urban expansion at broader spatial scales.
Literature review
Path dependence has been studied in a broad range of disciplines, and models testing for this condition have been applied to a number of practical issues. Radzicki and Sterman have defined path dependency as ‘a characteristic of models that get locked into the particular dynamical path they initially “choose” (usually by chance)’;Footnote 12 these models represent a useful means of simulating dynamic change. From an economic perspective, path dependency can be seen as a way of understanding transformations as a process of cumulative causation where the dominant feedback loops are self-reinforcing.Footnote 13 On the assumption that an existing path is determined by a chance event in the past or by the cumulative effects of past actions, policy choices can intervene in a system and alter the direction of development.Footnote 14 However, finding the correct policy choice may be difficult since these feedback loops (or cause-effect linkages) may not be linear or there may be a time lag between an action and its effect. Path dependency provides a useful perspective for understanding how these processes operate over time to produce and maintain certain places as comparatively attractive for defined targets, such as population growth.
Moving from economics to geography, we can see that a functionalist approach to urban transformations based on human ecology has influenced the theory of path dependency.Footnote 15 According to Brown et al., ‘geographic communities develop through a linear series of stages animated by changing technological regimes, large-scale population movements, or other perturbations’.Footnote 16 Delineating a linear, evolutionary model of development, interpretations of urban change as a series of discrete stages – each of which unfolds from the preceding one – were criticized as simplistic and difficult to demonstrate, since stable criteria for determining the number and order of stages forming a developmental sequence are lacking.Footnote 17 However, the idea that existing conditions influence (or limit) the successive development dynamics of a given place/community has had a major influence on social science.
From a historical perspective, path dependency suggests that actions which have occurred in the past affect the options available in the future, since events are shaped in specific ways by historical trajectories involving self-reinforcing sequences that reproduce particular developmental patterns over time. Since path dependency may explain how history limits developmental options, it can produce durable differences between societies. Rather than being a predetermined linear process, the demographic response to urban growth is contingent on a set of decisions about economic development made at strategic moments. Decisions taken at turning points in history have a powerful positive (or negative) impact on future developmental paths.
The use of path dependency as a theoretical guide to empirical research is therefore not limited to examining the negative implications of particular developmental trajectories, because certain events, circumstances and decisions can also trigger advantageous future paths.Footnote 18 The long-term development of individual communities within a given city or metropolitan region is an example of such an evolutionary path, where the intrinsic characteristics of local development depend on the degree of path dependence.Footnote 19 Urban science and development studies have argued over whether geography (i.e. natural advantages) or history (i.e. man-made advantages) are the main determinants for the spatial distribution of population and economic activities. The strong influence of geography on spatial development implies that localized historical shocks only have temporary effects while regional policies may shape spatial development permanently.Footnote 20
However, localized historical shocks can have permanent spatial effects, which suggests the importance of path dependence in space and reflects the existence of multiple spatial equilibriums, with the selection of one of these conditions being determined by history.Footnote 21 This historical importance leaves a role for regional policies since they can have an effect on spatial development.Footnote 22 We therefore considered a series of exogenous shocks to investigate the extent of urban path dependence in a European country. Urban growth – its extent and pattern – was spatially allocated according to local conditions and regional driving forces, assuming a path-dependent approach, as elucidated in the sub-section Logical framework and econometric specification.
Methodology
A century of socio-economic development in Greece and its capital city, Athens
The Greek population increased from 5 million inhabitants in 1920 to 10.5 million inhabitants in 2021. A young demographic structure was at the base of pre-war population dynamics leading to a positive natural balance, which remained stable until the early 1970s. Together with other factors, population growth has ensured continuous economic development since the 1950s, which in turn fuelled internal migration toward urban areas.Footnote 23 In the 1950s, Athens’ share in the total Greek population was over 30 per cent and remained stable in the following decades. The history of Athens is complex (Figure 1), as the city experienced rapid expansion under different economic and political conditions. Since the early 1920s, the city has seen periods of accelerated population growth, including the influx of refugees from Asia Minor, and internal migration during the Civil War of the late 1940s, which started a long wave of dense urbanization encompassing half of the twentieth century.
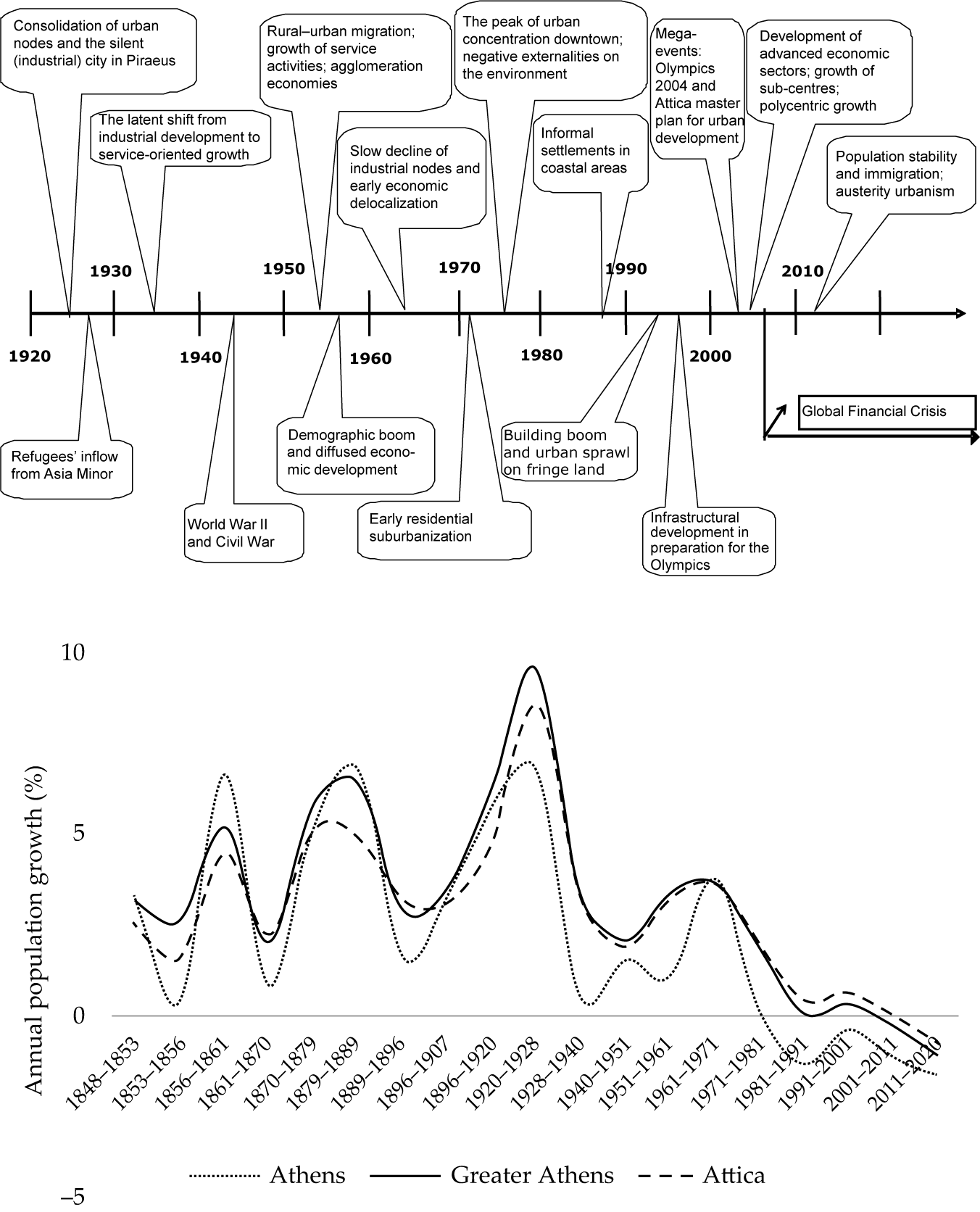
Figure 1. A conceptual map illustrating the main events shaping Athens’ expansion in the last century (top) and annual population growth rates in downtown Athens, the Greater Athens’ area and Attica between 1848 and 2021 (bottom).
Athens’ population increased substantially in the 1950s, fuelling compact urbanization. This spatial pattern was consolidated in the following two decades reflecting radio-centric urbanization. A latent shift toward suburbanization in the 1970s was associated with the decline of inner cities and the increase of fertility in outer districts.Footnote 24 An evident modification of the spatial structure of population growth rates was observed between 1981 and 1991, since the polarization in urban areas (with a declining population) and suburban districts (with an increasing population) consolidated in response to suburbanization – with residential mobility becoming a powerful driver of metropolitan growth.
Some demographic traits have distinguished metropolitan Athens (basically corresponding with the administrative region of Attica) from the rest of the country since the 1970s. The natural rate of population growth was high in Attica and fell significantly in the late 2000s, 10 years later than in the rest of Greece, at the end of the ‘golden’ decade of economic expansion culminating in the 2004 Olympics. International migration has contributed to demographic dynamics in both the region of Attica and in the country, albeit in different ways. The migration balance in Attica was positive until the early 1970s; it declined in the following decade and recovered in the 1990s and 2000s. In the rest of Greece, the migration balance was negative until the early 1970s, highlighting the role of internal migration in the long-term expansion of Greek cities.Footnote 25 The migration balance in Attica has been positive since the early 1980s, declining slightly in the following decades.
Recent growth paths in Attica have resulted in spatial heterogeneity with local specificities that reflect gentrification, class segregation, ageing and inner-city shrinkage because of low fertility and stable (or slightly declining) migration flows. Despite rapid immigration, the most recent developmental stage has featured reversed population trends, with demographic decline as a result of recession.Footnote 26 The migration balance was weakly negative in the 2010s, albeit with low emigration rates. In 2021, the resident population of Athens amounted to 3.7 million inhabitants (nearly 1,200 inhabitants/km2), although density still divides urban settlements (up to 7,000 inhabitants/km2) from rural communities (less than 200 inhabitants/km2).
Based on these premises, the long-term developmental trajectory of metropolitan Athens represents a quasi-experiment where continuous urban expansion reflects (and is fed by) the sedimentation of different exogenous shocks and the contemporary feedback characteristic of informal settlement expansion and weak spatial planning – at least up to the 1980s. Because of complex stratification of multiple urban agents,Footnote 27 the geography of municipalities resembles the fine-grain and inherent specificities in local communities interacting over time and competing for growth. Assuming path-dependent population dynamics as a result of this mutual interplay of social forces,Footnote 28 Athens’ metropolitan space was therefore regarded as anisotropic – generating spatially differentiated and mostly heterogeneous conditions for regional and local development.
Study area
Metropolitan Athens, basically coinciding with the region of Attica (3,025 km2) delineated by the Urban Atlas initiative (European Environment Agency, 2006), was the object of our study. Urban Atlas boundaries are in line with the conventional definition of Athens as a mono-centric region, a morphological structure which has been defined since the 1920s. The use of stable geographies reduces the effect of variable boundaries, allowing for the delineation of coherent metropolitan growth along a predetermined urban–rural gradient based on the distance from central Athens. Municipalities were selected as the elementary domain, which allowed an appropriate multi-temporal analysis of statistical data, mainly from general censuses. The local administrative structure was articulated in 115 municipalities (and local communities), half of which formed the Athens–Piraeus’ conurbation (430 km2). As representative of the (fine-grain) geography of urban neighbourhoods and small communities, Local Administrative Units have an important role in official statistics because of the availability of data from the population (and other) censuses and its relevance for spatial planning and implementation of local policy. ArcGIS release 10 (ESRI, Inc., Redwoods, USA) allowed for the use of a shapefile of municipal boundaries produced and disseminated by the Hellenic Statistical Authority (ELSTAT) for descriptive analysis.
Logical framework and econometric specification
A comparative investigation of local demographic dynamics provides explicit evidence of path-dependent urban growth. Our analysis integrates the notion of path-dependent growth into a broader interpretation of metropolitan cycles based on the spatial variability of long-term population growth rates and the related distribution of socio-economic functions. Changes over time in the relationship between population dynamics and key factors of urban expansion (e.g. agglomeration, scale economies, amenities, planning constraints) were delineated as an intrinsic function of metropolitan growth, which identified stable patterns and regime shifts over space.Footnote 29 Assuming population dynamics as the latent engine of growth, such a relationship was interpreted as a ‘production function’ – a fundamental representation of urban expansion based on multiple conditions for equilibrium that reflect the long-term development path of a given city.Footnote 30
If we assume that path-dependent regulation of population growth is an intrinsic property of local systems that delineates the sequence and timing of a given urban cycle, a refined knowledge of path-dependent dynamics at the heart of metropolitan growth and decline can clarify the intrinsic mechanisms shaping developmental stages and the transitional periods in between. Since different levels of path dependency delineate independent and distinctive stages of urban development, this provides a novel framework to estimate the rapidity of change in the different components (and forces) underlying metropolitan expansion. Functions estimating the frontier of urban growth may identify sequential steady states of metropolitan systems, with distinctive population dynamics as the basis of their shifts toward another development regime.
If we look beyond the supposed rigidity of spatial implicit models, a consensus analysis of the outcome of spatially explicit models – both global and local regressions – may effectively interpret spatial dependence and heterogeneity in population growth rates and outline latent patterns of urban expansion.Footnote 31 Accordingly, metropolitan growth has been divided in our study into the individual factors contributing to settlement expansion and depending on the dynamic interplay between economic and non-economic forces.Footnote 32 By investigating a sufficiently long interval approximately divided into decades, we ensured that the study captured independent urban waves, profiled using different growth functions. The goodness-of-fit of models estimating such functions was taken as a preliminary verification of the existence of path-dependent mechanisms of population growth.Footnote 33 Furthermore, regression coefficients assessed the net impact of path dependence on total growth over time.Footnote 34 Other predictors have helped to identify the role of alternative mechanisms of population growth or decline against path dependence. In other words, our analysis concentrates on the long-term evolution of functional coefficients as a manifestation of dynamic mechanisms shaping urban growth.Footnote 35
Statistical data and variables
Total population of each municipality was made available from the official statistics of the ELSTAT disseminated at 10 dates (1920, 1940, 1951, 1961, 1971, 1981, 1991, 2001, 2011 and 2021) through the institutional website,Footnote 36 printed yearbooks, other technical publications, as well as unpublished materials that include scanned copies of past censuses’ bulletins and records accessible at the ‘digital library’ of the same website, or by a specific request. Boundaries of municipalities in Greece were stable over time, but underwent minor changes. The total population in municipalities with slight changes in administrative boundaries was estimated with regard to additional demographic information derived from the ELSTAT (total population by settlement/village/local communities) or estimated as the average of the five nearest spatial units. In the last case, the reconstructed population for the spatial series of municipalities during the last century was controlled with ancillary variables from independent statistical sources with a (direct or indirect) relationship with population such as building censuses, agricultural censuses and annual surveys of economic activities, both industry and services.
The annual percentage change in resident population over nine inter-census decades (see above) elaborated from total population data for each municipality in metropolitan Athens was the dependent variable of this study. A number of variables were considered as candidate factors of urban growthFootnote 37 and were made available from official statistics at the same spatial scale: (i) the annual percentage population growth rate (Gro) measured one time window before (i.e. lag(-1)) as an intrinsic measure of path dependency, (ii) population density (Den), that is, the ratio of resident population in the total municipal area (km2, log-transformed) as a proxy of agglomeration economies, (iii) absolute municipal size (Siz), that is, the total number of inhabitants (log-transformed) in each municipality at a given time as a proxy of congestion externalities; (iv) average elevation of each municipality (Ele, m), (v) a dummy identifying coastal (‘1’) and inland (‘0’) municipalities (Sea), (vi) a Soil Quality Index (Sqi) and (vii) a Climate Quality Index (Cqi) both derived from raster maps released by the European Environment AgencyFootnote 38 ranging from 1 (worst condition) to 0 (best condition). Variables (iv–vii) quantified natural amenities and delineated territorial conditions in the study area. Additional variables include (viii) a dummy identifying affluent (‘1’) and economically disadvantaged (‘0’) districts (Poo) following a territorial classification proposed in earlier studies, (ix) a dummy identifying municipalities (Rai) with at least one railway station (‘1’) from those without a station (‘0’), (x) a dummy (Hea) distinguishing municipalities that act as the prefectural head town (‘1’) from those that are not (‘0’) and, finally, (xi) perimeter-to-area ratio (Pat), a control variable derived from landscape ecology and assessing the overall configuration and spatial form of local administrative units, which was computed from the shapefile of municipal boundaries released by the ELSTAT as noted above. All variables were standardized prior to analysis. To check for predictors’ multi-collinearity, a Variance Inflation Factor (VIF) was finally calculated for each time interval and input variable delineating a non-redundant structure of predictors’ matrix.
The design of the analysis
Our work generalizes and expands a simplified framework illustrated in Ciommi et al.Footnote 39 Assuming different spatial regimes of population dynamics associated with each stage of the metropolitan cycle, we specified the spatial variability in population growth rates as a function of path dependency, density dependency, congestion externalities, economic factors including agglomeration and accessibility, and non-economic factors including natural amenities. To model such relationships, a comparative econometric approach was adopted. A Principal Component Analysis has provided a summary of the outcomes of this approach delineating non-redundant drivers of urban change. These outcomes may shed further light on the complex paths of metropolitan development in advanced economies.
Econometric specification
We assumed that the administrative size of municipalities does not influence population dynamics. Cross-section regressions were run for each time interval mentioned above, with each stage of the metropolitan cycle taken as representative of distinctive socio-economic contexts at the local scale.Footnote 40 We specified population growth rates (G) as a function of five dimensions regulating metropolitan development:Footnote 41 path dependency (P), density dependency (D), congestion externalities (C), other economic forces including agglomeration and accessibility (E), and non-economic forces (S) as follows:

This specification allowed for a coherent identification of the degree of path dependence in urban growth and the impact of density-dependent regulation of population dynamics and of ancillary variables describing the background context.
Regression models
Regression models were run controlling for time (i.e. distinguishing the impact of distinctive stages of the cycle) and space (i.e. using global, spatially explicit econometric specifications and comparing results with those from spatially implicit, global specifications and from spatially explicit, local approaches). We assumed an Ordinary Least Square (OLS) regression as a baseline model exploring spatial variability of the dependent variables as follows:

where Y denotes an N × 1 vector consisting of one observation on the dependent variable for every unit in the sample (i = 1, …, 115). X indicates an N × K matrix of predictors associated with the K × 1 parameter vector
$ \beta $
. Finally,
$ {i}_N $
is an N×1 vector of ones associated with the constant term (parameter
$ \alpha $
) and
$ \epsilon $
is an N×1 vector of independently and identically distributed disturbance terms with zero mean and variance
$ {\sigma}^2 $
. Spatial models were derived by augmenting OLS regressions to capture different types of spatial autocorrelations among the observation units. A commonly applied spatial model that accounts for at least one of the spatial autocorrelations above is the Spatial Autoregressive Model (SAR) wherein the spatial spillovers arise from the dependent variable:

A second model was applied when interdependence among prefectures takes place through the error term, namely the Spatial Error Model (SEM):

where W is a positive N × N spatial weights matrix that describes the dependence structure between observation units and reflects the spatial influence of location j on location i or vice versa. Here, we adopted an inverse-distance (normalized) matrix of spatial weights with zero diagonal elements and off-diagonal elements
$ {\omega}_{ij} $
quantifying the reciprocal of the distance between the units i and j; WY and WX denotes respectively the endogogenous and exogenous interaction effects, while Wu is the interaction effects among the disturbance terms of the different municipalities. The scalar parameters
$ \rho $
and
$ \lambda $
measure the strength of dependence between units, and
$ \theta $
is a K × 1 vector of response parameters. Models were estimated with the generalized method-of-momentsFootnote 42 and best-fit estimation of the above-mentioned models was evaluated using adjusted-R2 or pseudo-R2.
A spatially explicit strategy based on Geographically Weighted Regressions (GWRs) with the same econometric specification illustrated above was finally adopted to predict the intrinsic (local-scale) variability of population growth rates across metropolitan Athens, identifying the role of spatial heterogeneity and controlling for spatial dependence. The model’s goodness-of-fit was assessed using global R2 coefficients significant regression coefficients at p < 0.01 with bi-square nearest neighbour kernel to calculate spatial weights.
Principal Component Analysis
A Principal Component Analysis was run on a matrix composed of the 11 predictors presented in the sub-section Statistical data and variables and the adjusted-R2 estimating the strength of the respective urban function (as column inputs); and 24 observations (3 regression models: SAR, SEM and GWR, and 8 time windows (as row inputs). The growth function was estimated at the scale of individual municipalities (n = 115) with the regression models illustrated in the sub-section The design of the analysis by fixing an a priori eigenvalue threshold (> 3) to select significant components. Assuming statistical independence among components by construction, loadings and scores were illustrated using a biplot allowing for the interpretation of each extracted dimension.
Results
Results of regression models
We used the spatially implicit Ordinary Least Square (OLS) regressions as the baseline and we reported the outcomes of spatially explicit (global: SAR and SEM; local: GWR) econometric models in Appendix 1. Table 1 illustrates a consensus analysis of the most relevant econometric results in the four tested models (OLS, SAR, SEM, GWR) reporting the sign of the related regression coefficient. Figure 2 shows the main trends over time for the significant predictors in the models. The models’ goodness-of-fit (adjusted-R2) was largely variable over time, reaching the highest values in correspondence with the 1960s, the 1970s and the 1980s, irrespective of the model used. Although all models were found significant at p < 0.05, the lowest goodness-of-fit was observed in the first observation time window (1920–40 vs. 1940–51) and in the last observation time window (2001–11 vs. 2011–21). Local regressions systematically outperformed global models, providing similar results for all predictors as far as the sign and value of slope coefficients are concerned.
Table 1. A consensus analysis of the sign of significant (p < 0.05) regression coefficients for all models (OLS, SAR, SEM, GWR) tested in this study.

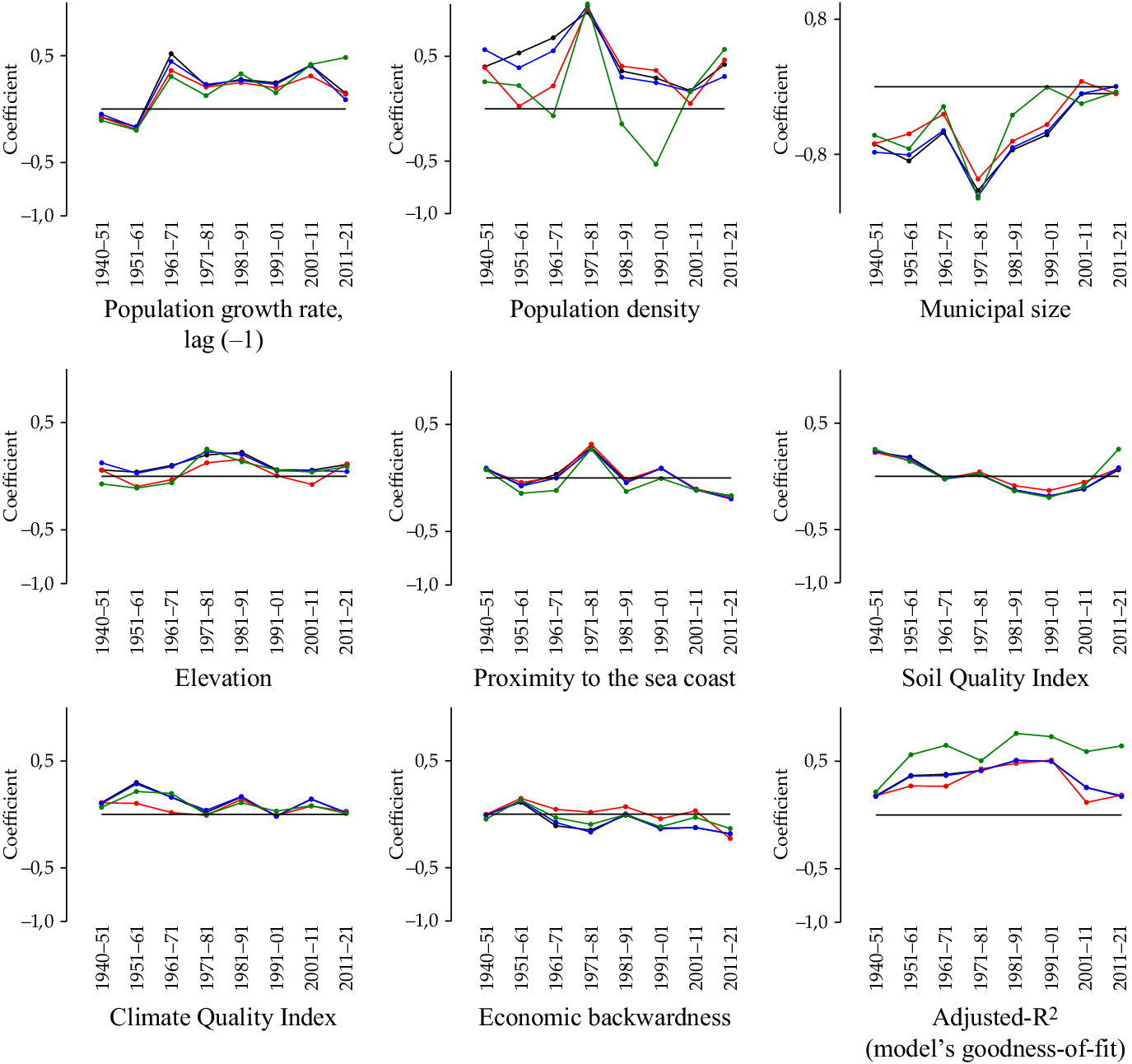
Figure 2. Trends over time in regression coefficients by predictor, time window and econometric model (black: OLS; blue: SAR; red: SEM; green: GWR).
The non-significant coefficients of Rai, Hea and Pat underline the importance of controlling for the area-to-perimeter patch index (Pat); the modest role of railways (Rai), probably because of the limited extent of the network. The neutrality of medium-size towns (Hea) in the metropolitan growth of Attica indirectly confirms the persistence of a mono-centric structure in the study area, in line with the working hypothesis. The remaining predictors were statistically significant only for one of few (sometimes consecutive) decades. In line with the goodness-of-fit of each model, the number of significant variables in the estimated growth function changed between the time window; for example, only two predictors in the first time window and four predictors in the fourth and fifth windows.
The predictor estimating path-dependent growth (Gro) was found to be statistically insignificant in the first and the last time window, and highly significant for the remaining observations, assuming a negative regression coefficient for the 1950s and a positive coefficient in the subsequent decades up to the 2000s. Recession-driven spatial heterogeneity characteristic of the most recent growth stage may explain the irrelevance of path dependency in the 2010s urban expansion. At the same time, exogenous shocks leading to huge, spatially uncoordinated and largely unplanned population increases may explain path independence in the time window encompassing the two world wars, and the negative coefficient of Gro in the immediate aftermath of World War II – testifying to a relevant regime shift in the spatial distribution of population before and after the Greek Civil War.
The reverse pattern was observed for the predictor assumed to estimate the negative externalities of urban concentration (Siz), as it was significant from the first to the sixth time window. The sign and time patterns of Siz were consistent with the role of population hyper-concentration in central cities (Athens, Piraeus and the neighbouring municipalities), delineating the role of congestion externalities in triggering suburbanization, that is, pushing a segment of the resident population to move to less crowded districts in the Greater Athens area or in peri-urban locations. Although earlier studies dated the early stages of suburbanization to the 1960s, our results indicate how congestion externalities were an important force of suburbanization at least one decade before, anticipating the increase in residential mobility observed in the 1960s and the 1970s. At the same time, Siz regression coefficients declined abruptly in the 2000s and the 2010s, and were systematically insignificant for all models. These results may depend on the latent shrinkage of central Athens and Piraeus, which started in the 1950s. Population contraction continued at a reduced pace, leading to a substantial de-concentration of inner cities; Athens and Piraeus lost nearly 3,000 inhabitants/km2 in a few decades. These (mostly unplanned) de-congestion processes answered the issue of population hyper-concentration of central settlements characteristic of contemporary Athens.
The regression coefficients associated with population density (Den) were significant (and positive) only in the 1970s, corresponding with the highest goodness-of-fit of all econometric models run in this exercise. Such results delineate a density-dependent process of population growth, in addition to path dependence and the net effect of congestion externalities in a particularly dynamic stage of Athens’ growth. It evidences the end of compact urbanization and the consolidation of a less dense settlement model. From a morphological perspective, the 1970s was the decade with the highest settlement concentration in Attica and the greatest density polarization in urban and rural districts in the last two centuries. These results clarify the dominance of path-dependent mechanisms over density-dependent processes of urban growth, delineating in turn the synergic impact of congestion externalities.
The econometric analysis outlined a less relevant role of non-economic predictors of urban growth. Soil quality (Sqi) was significantly associated with population growth and urban expansion in the first and second decades (with positive coefficients) and in the sixth decade (with a negative coefficient). These findings indicate that urban settlements expanded into (i) low-quality land (located in the immediate outskirts of the Greater Athens area) in a developmental stage corresponding with compact urbanization and into (ii) high-quality land (located in remote, rural districts) during suburbanization. This confirms the key role of Athens’ sprawl in high-quality land development, and the validity of our approach in depicting the recent history of Athens.
Climate quality (Cqi) was significantly associated with population growth rates in the 1950s and 1980s. In both cases, positive coefficients indicate that population increased more rapidly in municipalities with a higher Climate Quality Index. This corresponded with a settlement expansion into drier land (i.e. with a higher Cqi) in accessible flat districts.Footnote 43 Significantly higher than random population growth rates were additionally observed in (i) coastal municipalities (Sea) and in (ii) hilly municipalities located in the mountains (Ele) surrounding Athens from east to west (Imitos, Pendeli, Parnitha, Egaleo) respectively in the 1970s and the 1980s.
Taken together, these findings document the important role of natural amenities driving urban expansion at specific stages of the metropolitan cycle, namely with early suburbanization in the 1970s and 1980s, according to the timing proposed by Morelli et al.Footnote 44 In that period, the most affluent population’s capacity to purchase buildable land in expensive locations became the main social actor in the residential movement toward the suburbs. Trends over time in a soil quality indicator confirm this pattern, since better land (lower Sqi levels), probably sold at higher prices, was the main target of exurban development in Athens. Despite the importance of socio-spatial disparities in the area throughout the study period, the wealth-income gradient was only found to be negatively associated with population growth rates in the last decade. These findings are not counterintuitive if framed within the broad framework of the great recession – probably the worst crisis to impact Greece since the Civil War. Results indicate that, in a period of economic stagnation and spatially heterogeneous urban expansion – with small settlement projects predominating over larger real estate because of capital and investment shortage – population and settlements grew less rapidly in economically backward areas and more rapidly in wealthier districts.
Affluent (mostly suburban) municipalities attracted a resident population more frequently than (urban) municipalities with compact settlements, agglomeration economies, infrastructures, accessibility conditions or amenities, as was observed in recent decades, and probably at the expense of the neighbouring disadvantaged districts. The indirect competition for population, a possibly planned way of growth because of the large inflow of services guaranteed by local policies in affluent districts, was the last frontier for urban growth and may justify the irrelevance of path dependency in recession. This environment, aggravated by a long-term demographic decline because of ageing and low fertility, may testify to another regime shift in the long-term growth of Athens. The COVID-19 pandemic exacerbated the consequent economic stagnation after moderate growth in the late 2010s.
Summarizing urban growth functions with a multivariate exploratory analysis
The results of a Principal Component Analysis presented in the sub-section Principal Component Analysis are illustrated in Figure 3. In accordance with the restrictive eigenvalue threshold fixed a priori, we extracted two principal components accounting for 59 per cent of the total variance that provided a parsimonious description of the growth functions in metropolitan Athens. By scrutinizing the most significant predictors in regression models, we found Component 1 was uniquely associated with Gro (-0.88), Poo (0.69) and Sqi (0.66). Component 2 was in turn associated uniquely with Den (0.93), Siz (-0.76), Sea (0.78) and Ele (0.66). These findings confirm the statistical independence of Gro and Den, since components are orthogonal by construction and provide proof of the conceptual independence of path-dependent and density-dependent patterns of population growth in the study area. Additionally, these results document the reverse role of Den and Siz (oriented toward opposite signs along the second axis) respectively as proxies of agglomeration economies and congestion externalities, confirming the validity of these two variables as non-redundant predictors of urban growth.

Figure 3. Biplot of a Principal Component Analysis depicting the multivariate relationship among predictors of urban growth by time window (the end year for each period is indicated with the following symbols: 1951 ▫ ; 1961 ▫; 1971 □ ; 1981 • ; 1991 ∇; 2001 * ; 2011 x; 2021 +).
Considering two principal components allows for a simplified representation of the latent (multivariate) relationship between predictors (columns) and time windows (rows) within a biplot controlling for the model’s heterogeneity (adj-R2) and regression approach (SAR, SEM, GWR). The analysis effectively discriminated between the outcomes of the three regression models at different times, irrespective of the model’s type: 1940–51 and 1951–61 coefficients clustered along the positive size of Component 1 and 1971–81 coefficients clustered along the positive size of Component 2; 1961–71 and 1981–91 coefficients clustered along the negative size of Component 1; 1981–91 coefficients clustered in a less evident (bari-centric) position; finally, 1991–2001 and 2001–11 assumed a mixed position in the biplot associated with the third quadrant (negative scores for both Axis 1 and 2).
The biplot was revealed to be a particularly appropriate graphical tool expressing the latent evolution of urban growth functions over time, that is, delineating the intrinsic relationship between population dynamics and economic/non-economic factors of growth. In this way, the role of (i) path dependence (time-varying), density dependence (occasional), congestion externalities (mostly in the first stage of the cycle: i.e. compact urbanization) and amenities (mostly in the second stage of the cycle: i.e. semi-dense suburbanization) was graphically summarized and helps us gain a more nuanced understanding of the history of contemporary Athens.
Discussion
If metropolitan agglomerations are the main engine of economic development, studying why cities emerge and persist is crucial to our understanding of growth processes and the evolution of socio-spatial disparities. While affecting demographic dynamics mostly at the local scale, spatial variability in settlement patterns and socio-economic processes has consolidated heterogeneous demographic dynamics shaping the evolution of metropolitan agglomerations on the European continent. This provides optimal conditions for testing path dependency. From this perspective, path-dependent regulation of population growth and decline can be regarded as one of the most common mechanisms leveraging urban expansion.
The empirical results of our study help to adapt the simplified idea of the city life cycle to more realistic growth waves depending on divergent demographic dynamics that reflect the joint evolution of urban, suburban and rural districts. In other words, this work has re-contextualized long-term urban development through the lens of path dependency, considering the intrinsic polarization in urban and rural areas into a non-linear growth process with sequential (and spatially differentiated) demographic accelerations and decelerations. The degree of path dependency, as estimated in this study, became more intense with acceleration waves and less intense with deceleration waves. These results suggest how non-linear and spatially variable mechanisms of demographic regulation are reflective of important feedbacks on urban expansion, and demonstrate the validity of econometric approaches qualifying the role of path-dependent mechanisms of population growth at the fine grain of local communities.
These findings also delineate distinctive development paths based on push-and-pull factors that reflect a ‘life cycle’ when initial dynamics affect subsequent population growth in variable ways. In line with these results, Tapia et al.Footnote 45 – who examined district population in Spain between 1860 and 1991 – observed a path-dependent population growth between 1910 and 1970, which was abruptly interrupted by the Civil War and the subsequent autarkic period. As in Greece, the degree of path dependency decreased from the 1970s. A comparable finding was observed in southern Italy. This study outlined the role of agglomeration economies in a period of intense path dependency (between the early 1950s and the early 1980s) and illustrated congestion externalities and a subtle process of peri-urbanization that intensified in recent decades. This process caused latent changes in population distribution across metropolitan regions that intrinsically reduced path dependency.
Accentuating the divide between high-density and low-density areas, sequential waves of settlement concentration and de-concentration were considered to be a manifestation of path-dependent population dynamics. These findings corroborate the idea that a path-dependent regulation of population growth is intrinsically (and negatively) associated with exogenous social dynamics. It explains the double effect of economic downturns as influencing positively (expansion) or negatively (recession) the degree of path dependence. Metropolitan populations experienced a transition toward low fertility, ageing and steady immigration, which paralleled socio-economic trends at regional and broader scales. Economic activities were in turn distributed heterogeneously over space, reflecting a local-scale polarization into dynamic and disadvantaged districts that were increasingly decoupled from the spatial distribution of agglomeration economies and wealth. The distance from central districts – a proxy of housing and infrastructure vintage in Athens – was tested as an alternative factor of urban growth. While explaining population growth effectively in the decades immediately after World War II, its explanatory power has reduced over time, evidencing a progressive weakening of the Athens mono-centric model.
Taken together, the results of our study document how spatially explicit models may appropriately characterize the individual, path-dependent development of Mediterranean cities. While identifying specific urban regimes, the empirical results outline the intrinsic characteristics of urban regions and the relationship between settlement density, metropolitan structure, economic dynamics and population growth across time and space. Such relationships were found to be more intense in demographically dynamic regions. Assuming that space is a factor promoting patterns of local development based on path dependence, a comparative analysis of population trends across metropolitan regions emphasizes the socio-economic complexity of different European contexts and the importance of a diachronic investigation of demographic processes associated with economic growth and social change in cities.
Path-dependent regulation of population dynamics has thus been demonstrated to be an important dimension of urban growth under heterogeneous socio-economic conditions. From this perspective, our study provides a novel approach to regional science, re-contextualizing metropolitan cycles within a more general debate on path-dependent urban growth. Results of this study should stimulate a comparative investigation of population dynamics aimed at identifying spatial regimes of urban growth under variable socio-economic conditions and heterogeneous local contexts. However, while our findings are consistent with a (time-varying) path-dependent development process, our county-level secondary-data analysis is removed from past and actual community decisions (e.g. planning, policy) and local histories in order to elucidate fully the intrinsic (e.g. lock-in) dynamics that differentiate the development paths of urban, suburban and rural communities within the same metropolitan region. Future research may combine secondary-data analysis, such as we have proposed here, with in-depth case-studies and archival surveys that examine the political economy, geography and history of public and private decisions. The quality and readability of official statistics (mainly census figures) over long intervals deserve further investigation.
Conclusions
Metropolitan expansion in advanced countries is the result of inherent transformations in settlement forms and socio-economic functions. A spatially explicit framework comparing the results of global and local regressions was adopted to evaluate the impact of different (economic and non-economic) drivers of urban expansion on path-dependent population growth as a key dimension of urban development along a complete metropolitan cycle (1920–2021) in Athens. In contrast to other aspects of urban expansion, path-dependent regulation of population growth was particularly intense at the end of urbanization and during early suburbanization, declining in the subsequent decades and becoming negligible in recent years. In a context of changing relationships among relevant economic actors, our analysis has shown that the spatial structure of population growth rates characterizes (and, possibly, anticipates) regional development processes, reflecting both the intensity and direction of path-dependent urban expansion.
Competing interests
The authors declare none.
Appendix 1. Analytical results of the econometric models
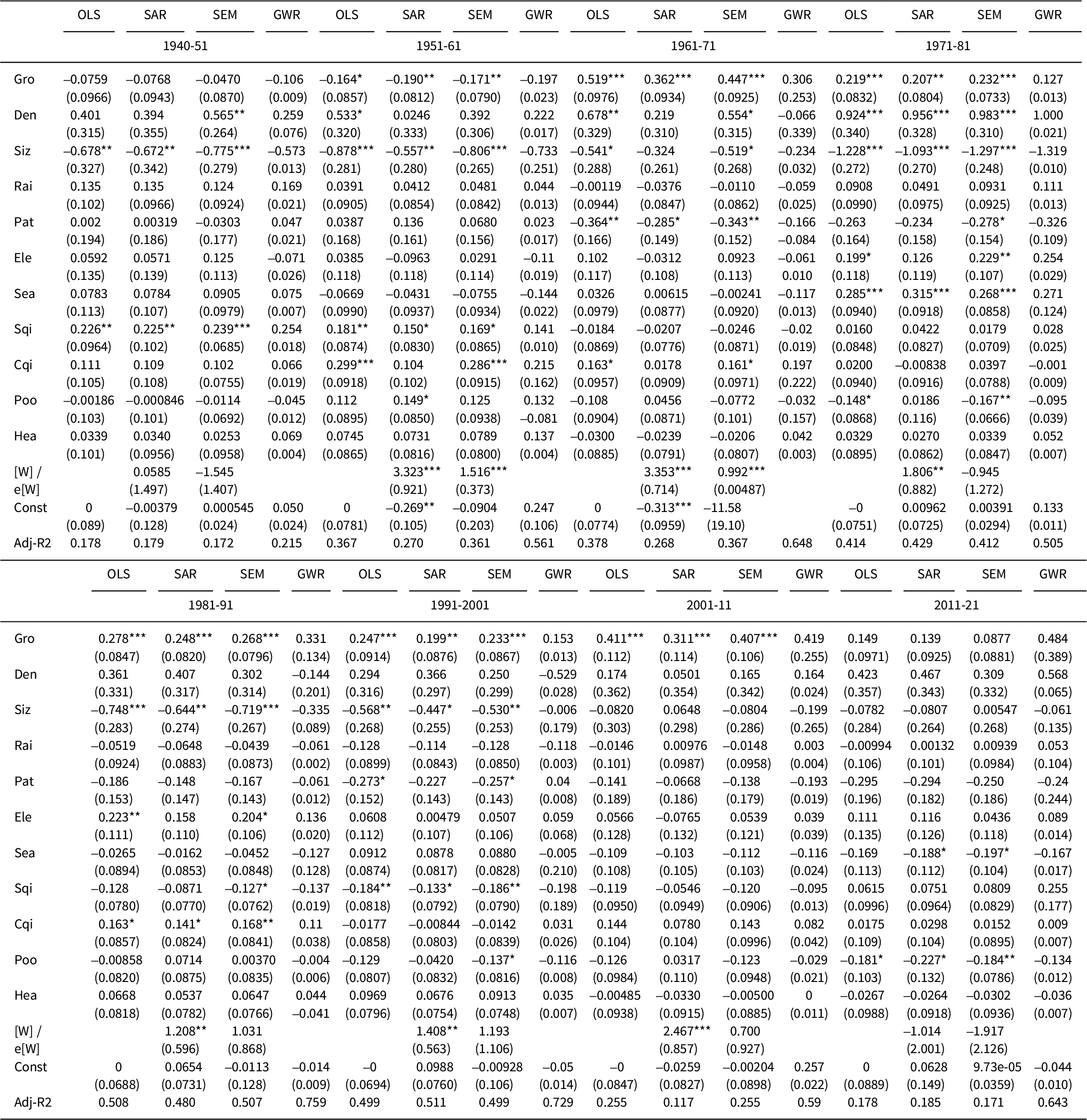