1 Introduction
Countries across the globe are struggling to cope with the most severe refugee crisis since the aftermath of World War II. Since the spring of 2015, more than 2 million (UNHCR 2017) new asylum claims were submitted in Europe alone, most of them from Syrian refugees who crossed into Europe via the Mediterranean Sea. In addition to the direct financial costs and economic consequences (Foged and Peri Reference Foged and Peri2016) that transition and receiving countries bear, the crisis has resulted in severe political repercussions in many destination countries, including protests against asylum seekers and political violence directed at refugees (Editorial Board 2015). Perhaps most importantly, the ongoing refugee crisis may also have long-lasting electoral consequences by affecting natives’ political preferences and their attitudes toward asylum seekers.
Does the influx of refugees increase support for extreme-right parties? Despite heated debates about asylum policies and the allocation of refugees across Europe (Bansak, Hainmueller, and Hangartner Reference Bansak, Hainmueller and Hangartner2017), there exists very little evidence regarding the impact of refugee arrivals on native voters’ political preferences and behavior. While there is a sizeable literature that documents a positive relationship between labor migration and vote shares for anti-immigrant parties in receiving countries (Barone et al. Reference Barone, D’Ignazio, de Blasio and Naticchioni2016; Mendez and Cutillas Reference Mendez and Cutillas2014; Halla, Wagner, and Zweimüller Reference Halla, Wagner and Zweimüller2015; Brunner and Kuhn Reference Brunner and Kuhn2014; Becker et al. Reference Becker and Fetzer2016), it is unclear how these findings translate to the context of refugee migration. Since humanitarian concerns are particularly important in structuring native attitudes toward asylum seekers (Bansak, Hainmueller, and Hangartner Reference Bansak, Hainmueller and Hangartner2016), we would expect that host communities are ceteris paribus more welcoming to refugees than to labor migrants. Only recently have two important studies attempted to isolate the effects of refugee migration on electoral outcomes in Austria (Steinmayr Reference Steinmayr2016) and Denmark (Dustmann, Vasiljeva, and Damm Reference Dustmann, Vasiljeva and Damm2016), with partially contradictory conclusions. This lack of knowledge is particularly problematic with respect to countries that have experienced a large influx of refugees within a very short period of time, such as Greece, which received more than 50% of all refugees crossing into Europe in 2015 (UNHCR 2016).
When trying to isolate the effect that exposure to refugees has on the political attitudes and behavior of the host population, the key problem for causal inference is that the number of arrivals is far from randomly assigned. Instead, the process through which refugees select between different potential host countries and communities depends on factors such as religious or cultural proximity, labor market conditions, and openness toward refugees (Neumayer Reference Neumayer2004). In other words, refugees will, as much as possible, flee to communities that they can reach and where they are welcome. This selection process severely confounds most existing comparisons of the political responses of natives to the presence of asylum seekers and refugees. If we find that communities that experienced a large influx of refugees are less (or more) supportive of anti-immigrant parties than places that have not, we cannot conclude that these differences are caused by refugee arrivals, because the selection ensures that these two groups of communities are also different on many other confounding characteristics.
By focusing on Greece, one of the European countries most affected by the current refugee crisis, we overcome this issue by taking advantage of a natural experiment to identify the electoral consequences of refugee arrivals. As the refugee crisis unfolded in the waters of the Aegean in spring 2015, Greek islands were converted into the main intermediate points for the bulk of refugees passing through Greece to Europe. As such, Greek islands were differentially exposed to the influx of refugees merely because they were closer to or farther away from the Turkish coast where boats with refugees departed. For example, the island of Lesvos (with a population of about 80,000) saw more than 200,000 asylum seekers passing through between May and September 2015. Yet the neighboring island of Lemnos, which belongs to the same geographical and administrative unit and features similar socioeconomic and political conditions, did not receive any refugees over this period, simply because it is a few miles farther away from the Turkish coast (see Figure 1, Panel A, for a map of the Aegean Sea).

Figure 1. Panel A shows that islands close to the Turkish border received the most refugee arrivals per capita. Panel B shows the monthly number of asylum seekers arriving at Greek islands over the period from January 2014 to March 2016. During the study period, the first election took place just before the onset of the refugee crisis on January 25, 2015. A second election took place at the height of the refugee crisis on September 20, 2015.
Several factors facilitate our identification strategy. First, among the 95 habitable islands in the Aegean Sea, distance to the Turkish border caused significant variation in the number of refugees arriving in each of these islands. Second, refugees tended to be housed within specific areas on each island. This allows us to exploit both between- and within-island variation in the number of refugee arrivals. Third, Greece held an election on September 20, 2015, right after the first wave of refugee arrivals that the country encountered throughout the spring and summer of 2015. The previous election had taken place only eight months prior in January 2015, before significant numbers of refugees arrived (see Figure 1, Panel B). The SI Appendix Sections S2.1 and S2.2 provide more information about the elections, which took place in the context of the Greek financial crisis. Two more elections, both in 2012, also serve as possible counterfactuals of support for extreme-right parties in the absence of the crisis. Fourth, many of these islands belong to the same electoral and administrative district, which ensures that they are identical across a plethora of observable and unobservable characteristics, such as the candidates running for office, regional government, police, judiciary, and access to EU funds.
Our study makes four main contributions. First, we provide rare causal evidence of the effects of exposure to the refugee crisis on support for extreme-right parties. For a number of reasons, the Aegean refugee crisis is an ideal case to test for the “flash potential” (Sniderman, Hagendoorn, and Prior Reference Sniderman, Hagendoorn and Prior2004) of immigration politics, when widespread concerns about asylum seekers (Bansak, Hainmueller, and Hangartner Reference Bansak, Hainmueller and Hangartner2016) coupled with a sudden influx of immigrants are hypothesized to lead to large-scale electoral mobilization (see also Hopkins Reference Hopkins2010). Specifically, Greece received about 400,000 new asylum seekers between April 2015 and September 2015 (UNHCR 2016), which translates to more than 2.8 refugees per Greek resident in affected islands. These sudden inflows reached their first peak just before the September 2015 election, thereby ensuring that the refugee crisis would be a highly salient issue when island residents cast their vote in the midst of the concurrent financial and economic crisis. The results suggest that this sudden and massive increase in refugee arrivals indeed fueled support for the radical anti-immigrant and anti-asylum-seeker party Golden Dawn (GD), but only moderately so: vote shares for GD increased about 2 percentage points (a 44 percent increase at the average) because of the exposure to the refugee crisis. This increase in vote shares, however, is statistically significant and substantively meaningful. GD became the third-largest (and minor opposition) party in the fragmented Greek multi-party system, and was awarded certain constitutionally protected privileges, such as the right to appoint the second deputy Speaker of the house and important vice-chair positions in parliamentary committees. In a companion study, we fielded a targeted survey to examine the impact of refugee arrivals on natives’ political attitudes and policy preferences (Hangartner et al. Reference Hangartner, Dinas, Marbach, Matakos and Xefteris2018).
Second, by employing within-island variation in proximity to refugee hotspots and measuring the number of refugee arrivals per resident, we show that the intensity of exposure further catalyzes the electoral boost of the extreme-right. Third, our study fills a gap by examining the political repercussions of the refugee crisis in Greece, the European country arguably most affected by the current refugee crisis and home to GD, the most extreme-right and neo-fascist party currently represented in a European parliament (Heinö Reference Heinö2016).
Fourth, Greek islands served as temporary transit destinations during our study period, and most refugees continued their journeys to Athens within 48 hours of arrival (Capon Reference Capon2015). This very unusual feature of our case enables us to make two important contributions to our theoretical understanding of the drivers of antirefugee attitudes. The refugees’ very temporary presence on the island eliminated most avenues for sustained interactions between locals and refugees, a prerequisite for the contact theory to work (Allport Reference Allport1979). Hence, this setting allows us to isolate the impact of exposure to (rather than contact with) refugee populations on political behavior—something that previous studies could not achieve. In addition, our findings are difficult to reconcile with realistic group conflict theory (Campbell Reference Campbell and Levine1965), in which competition for scarce resources is a necessary condition for conflict between the outgroup and the dominant group. Our study shows that mere exposure is sufficient to fuel prejudice and change political behavior.
2 The Unfolding of the Refugee Crisis in the Aegean Sea
2.1 Setting
Our study focuses on Greece, which was for Syrian refugees the main entry point to Europe, due to its proximity to the Turkish border, a long coastline that marks the EU external borders, and many difficult-to-patrol islands. Out of the 1.3 million new asylum seekers who reached European territory in 2015, more than 850,000 of them did so by arriving in one of the Greek Aegean islands (UNHCR 2016). But while some islands were strongly affected by these sudden refugee inflows, many other islands did not experience any contact with refugees. Most refugees left the islands of first arrival within a very short period, typically less than 48 hours, to continue their journeys via the ports of Piraeus or Thessaloniki to central and northern Europe. The SI Appendix Section S.2 provides more information about how the refugee crisis unfolded in Greece.
2.2 Data
This study draws upon a new panel data setFootnote
1
that covers all habitable Greek islands. The units of analysis are either municipalities (
$N=95$
) or townships (
$N=248$
). The data come from three different sources: electoral outcomes (at the municipality and township level) are provided for all four elections between 2012 and 2015 by the official record of the Greek Ministry of Interior and Public Administration; monthly data on refugee arrivals per island are provided by the UNHCR (UNHCR 2015), and geographic data on island’s distance from the Turkish coast are obtained from the online mapping service Google Maps, which provides satellite imagery and geospatial data visualization and measurement. The SI Appendix Sections S3 and S4 provide more information about the data sources, descriptive statistics and coding decisions.
2.3 Empirical Strategies
We employ two complementary empirical strategies to identify the causal effect of exposure to the refugee crisis on electoral support for the GD. First, we apply a difference-in-differences (DID) estimator to obtain an estimate of the effect of refugee arrivals on the September 2015 vote share for GD. The underlying logic behind the design is that for the islands that received refugees in the summer of 2015, we construct the counterfactual change in GD vote share between January and September 2015 had they not received refugees, by using the change in GD vote share in the unaffected islands. This strategy yields causal estimates so long as the parallel trends assumption holds. In the current context, this assumption implies that the vote share for GD would follow the same trajectory from January to September 2015 among treated and nontreated islands in the absence of the refugee crisis.
To assess whether this assumption holds and thus to evaluate the validity of the design, we turn to past elections, exploring whether the over-time trend in GD vote share differs between treatment and control units. The left panel of Figure 2 uses the municipality-level analysis, whereas the right panel depicts the results from the township-level analysis. In both graphs two lines are shown, each representing the over-time change in the vote share for GD from the first election of 2012 until the last election of 2015. The red line denotes treated units, and the blue line denotes control units. We see that both groups travel in parallel until September 2015, the first election after the onset of the refugee crisis in Greece. Moreover, consistent with our expectation that the within-island variation helps to account more effectively for unobservable differences in support for GD, we see that the treated and control groups are similar not only in terms of changes, but also in levels before the refugee crisis. Taken together, the two graphs strengthen our confidence in the validity of the DID design.
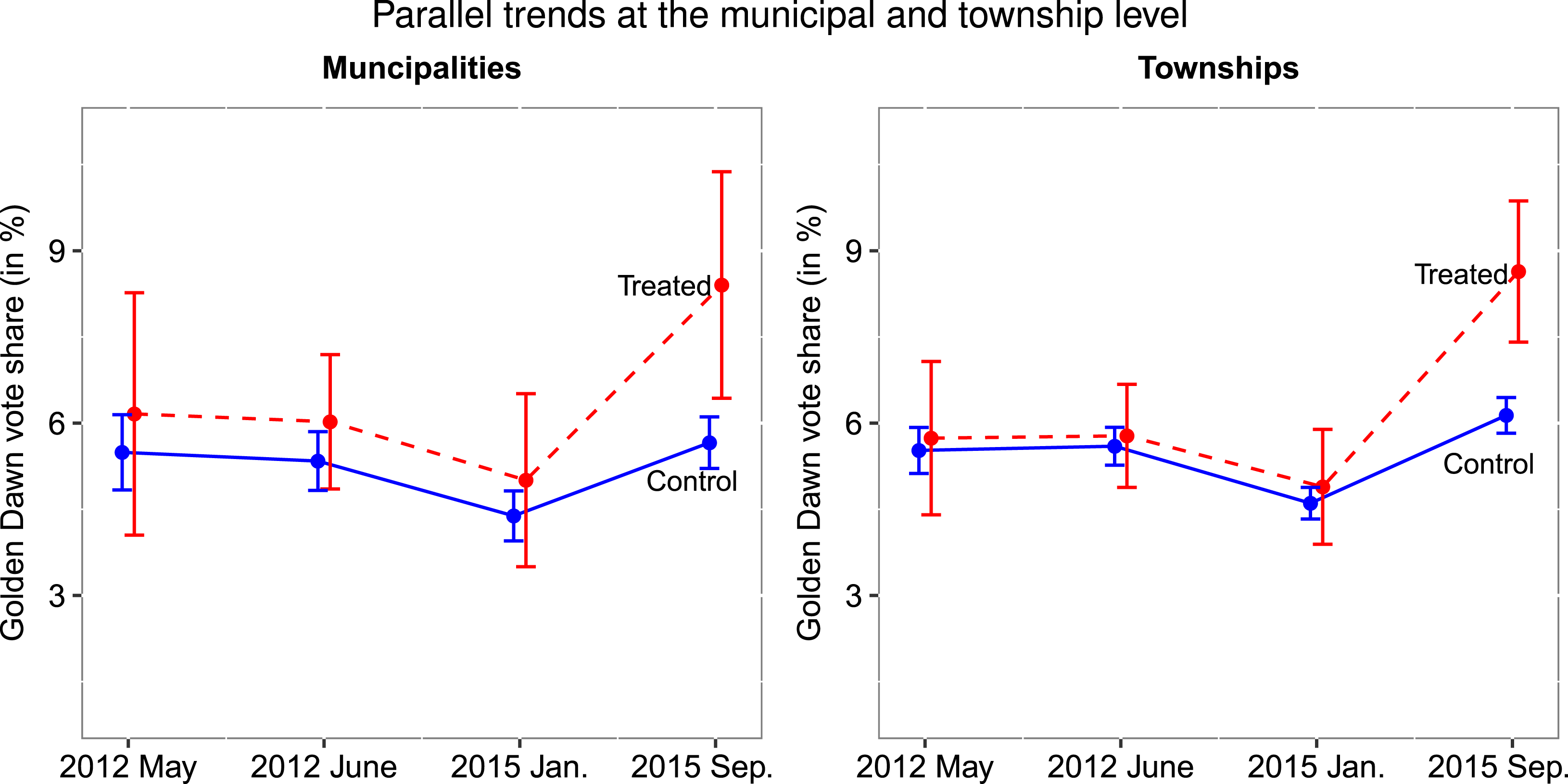
Figure 2. The analyses at the municipality (left panel) and township level (right panel) show that treated and control islands experience highly similar changes in support for GD prior to the refugee crisis, thereby strengthening our confidence in the parallel trend assumption. The blue connected line indicates the average vote share for GD in the municipalities (left panel) and townships (right panel) that received refugees. The red dashed line denotes the average GD vote share in municipalities and townships without refugee exposure.
The second empirical strategy employs an Instrumental Variables (IV) approach to identify the impact of the refugee crisis on the GD vote share. In particular, we use each island’s distance to the Turkish coast as an instrument for the number of refugee arrivals. To test whether the first-stage regression is sufficiently strong (Stock and Yogo Reference Stock, Yogo, Andrews and Stock2005), we must determine whether the islands closer to the coast were indeed more likely to have received refugees than those farther away. Figure 3 shows that this the case. The left panel displays the probability of having received any refugees as a function of the distance from the coast. The blue curve represents a local regression smoother that is fitted to the data. We find a monotonically descending relationship, indicating that the farther away an island is from the coast, the less likely it is to have received any refugees during the current crisis. The right panel shows that the same monotonically decreasing relationship exists for the intensity of refugee exposure (measured as the relative number of refugee arrivals per island resident). Both graphs indicate that this relationship can be accurately modeled by a logarithmic function (red line). Accordingly, we use logged distance from the Turkish coast as an instrument of refugee exposure in the subsequent analysis.
The two identification strategies are complementary. While the DID strategy recovers the average treatment effect for the treated islands, the IV strategy, recovers a different causal estimand, namely the local average treatment effect (i.e., the complier treatment effect). Moreover, whereas the DID relies on the parallel trends assumption, the IV strategy relies on the exclusion restriction (Angrist, Imbens, and Rubin Reference Angrist, Imbens and Rubin1996), namely the assumption that the only way proximity to the Turkish coast affects support for GD is through refugee arrival. The SI Appendix Section S5 provides more information about the estimation strategy for the DID and IV analyses.

Figure 3. First stage: islands’ distance from the Turkish coast predicts the number of refugee arrivals. The blue curves denote the local linear regression smoother (
$\text{span}=0.5$
), with the shaded area capturing the 95% confidence intervals. The left panel shows the propensity of receiving refugees against the distance to the Turkish coast, whereas the right panel shows the number of refugee arrivals per capita against the distance. The red curves display the predicted level of treatment exposure conditional on logged distance. In both graphs, the logarithmic function of distance from the Turkish coast provides a good approximation to the data.
A placebo test confirms that control islands provide a credible counterfactual for treated islands. For this, we exploit a particular feature of the Greek election law: Registered nonresident voters cast their ballot in their town of residency, even when they do not live in the town they are registered in. We use this group to examine the behavior of nonresidents who live on the Greek mainland but are registered to vote for islands with refugee exposure. These placebo tests, presented in the SI Appendix S6.3, reveal that electoral support for GD did not change substantially among (i) nonresident voters of treated islands over time and (ii) between nonresidents of treated and control islands.
Table 1. Impact of refugee arrivals on GD vote share.

Note: Models 1–12 display ordinary least squares (OLS) regression coefficients with clustered standard errors in parentheses. Models 1–8 use a binary treatment indicator while models 9–12 use the number of refugee arrivals per capita. Models 1, 2, 5, 6, 9 and 10 show the effect on GD vote share. Models 3, 4, 7, 8, 11, and 12 use the GD vote share from the previous election as placebo outcome. All models control for election and unit of analysis (municipality or township) fixed effects. In addition, models 2, 4, 6, 8, 10 and 12 also include unit-specific linear time trends.
3 Results
3.1 Panel Data Estimates
Massive refugee arrivals from the Turkish coast to the Greek islands started in spring and escalated over the summer of 2015. Our panel data estimates exploit that Greece held two elections in the same year, in January and September. Furthermore, refugee arrivals were highly clustered among a subset of the Aegean islands. We use the cross-island as well as within-island variation (Figure S2, Panel A) to assess the impact of refugee presence of vote share for GD (Figure S2, Panel B). Employing a DID estimator, we treat the September 2015 election as the posttreatment election and the three last elections preceding the January 2015 election as the pretreatment elections. A detailed description of empirical strategy is provided in the SI Appendix Section S5.
Table 1 presents the main result and shows that refugee arrivals catalyze GD vote share.Footnote
2
Model 1 uses municipalities as the unit of analysis and shows that in islands that received refugees, support for GD rose by 2 percentage points (std. error 0.35, two-tailed
$p<0.001$
). The average vote share for GD in 2015 among all municipalities in the estimation sample is 4.5 percentage points. Thus, the magnitude of the backlash effect amounts to a 44 percent increase in the party’s vote share. Model 2 shows that the results are virtually identical when adding linear municipality-specific trends that capture smooth changes in unobserved confounders. Models 3 and 4 are the placebo analogues of Models 1 and 2, respectively. They present the same result but using GD’s lagged vote share as the outcome. Reassuringly, we find no effect of exposure to the refugee crisis on GD’s vote share in the previous election.
Models 5 and 6 use townships as the unit of analysis. Recall that townships are clustered within municipalities and thus allow for within-island variation. The results are very similar to the municipality-level analysis. We find that townships receiving refugees see an increase in the GD vote share by slightly more than 2 percentage points (std. error 0.26, two-tailed
$p<0.001$
) compared to townships that were not exposed to refugee arrivals. Model 6 shows that the effect remains robust to the inclusion of township-specific linear trends. This result suggests that the increase in the party’s vote share is not uniform within the affected islands, but rather more concentrated among those areas directly exposed to the refugee crisis. The placebo tests shown in Models 7 and 8 confirm that treated and control townships follow parallel trends prior to the January 2015 election. The difference in the vote share for GD between treated and untreated townships before that election is essentially zero, both with and without the inclusion of township-specific trends.
Finally, Models 9 to 12 replace the binary treatment indicator with the per capita number of refugees arriving in each island. Models 9 and 10 present the treatment effects and indicate that the arrival of one refugee per resident increases GD’s vote share by 0.77 percentage points. To see more concretely what this means, take Samos, which received approximately 2.5 refugee arrivals per resident. As a result, the vote share for GD in September 2015 is expected to have risen by almost 2 percentage points because of the refugee arrivals. This estimate remains robust when adding municipality-specific linear trends. Models 11 and 12 display the placebo results, which use the lagged values of support for GD. Reassuringly, we find that exposure to refugee arrivals in 2015 plays no role in the vote share for GD in the pretreatment elections. The average treatment effect is again very close to zero both with and without municipality-specific trends. Figure S2 in the SI Appendix provides a visual summary of those results.
3.2 Instrumental Variables Estimates
Distance to the Turkish coast plays a key role in whether and how many refugees arrived on a particular island in the Aegean in 2015. As discussed in the previous section, we exploit this natural experiment by using distance from the Turkish coast as an instrument of refugee exposure.
Figure 4 illustrates the logic of the estimation strategy. The right panel of Figure 4 shows the change in GD’s vote share, from May 2012 to January 2015, as a function of the (logged) distance of each island from the Turkish coast. Since the Syrian refugee crisis impacted Europe only after the spring of 2015, we expect no relationship between distance from the coast and support for GD. This is exactly what we find: The linear regression coefficient measuring the effect of logged distance to the Turkish coast on change in GD support between May 2012 and January 2015 is 0.048 (std. error 0.181, two-tailed,
$p<0.782$
), i.e., close to zero and far away from conventional level of statistical significance. The left panel of Figure 4 shows the same analysis but using the change in support for GD from January to September 2015 as outcome. For this period, we estimate a linear regression coefficient of
$-0.433$
(std. error 0.110, two-tailed
$p<0.001$
), indicating that after the onset of the crisis, proximity to the coast is associated with a significantly higher increase in GD vote share.

Figure 4. Intention-to-treat effect: Proximity to the Turkish coast increases GD vote share after the inflow of refugees in September 2015, but has no impact on previous elections. The blue lines indicate linear OLS regressions with 95% confidence intervals (shaded areas). The left panel shows the change in GD vote shares from January to September 2015, the right panel shows the change in GD vote shares between May 2012 and January 2015. Both graphs use the logged distance from the Turkish coast in the horizontal axis.
Table S3 in the SI Appendix presents the IV estimates, using the binary and the continuous treatment indicators. Models 1 and 3 show the first-stage regression results. Both the binary and continuous measures for refugee arrivals are negatively related to distance to the Turkish coast. The
$F$
statistic measuring the strength of the first stage is 46 and 31, respectively, well above the critical value (Stock and Yogo Reference Stock, Yogo, Andrews and Stock2005) of 10. Model 2 shows that the presence of refugees during 2015 increases the GD vote share by more than 2 percentage points. Model 4 employs the continuous measure and shows that for an additional refugee per capita the vote share for GD increases by almost 1 percentage point. Both estimates are very close to those obtained using the DID design.Footnote
3
Model 5 presents the intent-to-treat result using all four elections since May 2012. The result shows that the effect disappears entirely when moving from an island that is 2 km away from the Turkish coast (e.g. Samos) to an island that is almost 100 km away (e.g. Karpathos).Footnote
4
Taken together, both empirical strategies lead to the same conclusion: The refugee crisis resulted in a statistically significant and politically substantial increase in electoral support for Europe’s most radical right-wing party, GD. In the SI appendix, we extend this evidence in two related dimensions. First we look at the intent-to-treat effect in greater detail, showing that distance to the Turkish coast predicts GD vote share only in September 2015, but not in any previous election (see SI Appendix Table S5). Second, we drop observations far from the Turkish coast. We use several cut-off points, ranging from 500 to 50 kilometer maximum distance to the coast. The results appear in Figure S7 of the SI Appendix and appear remarkably robust to changes in the range of distance to the Turkish coast.
Is GD ‘stealing’ votes from other parties or mobilizing citizens that would otherwise abstain? While a full analysis of the mechanism underlying these shifts in electoral support is beyond the scope of this study, our data provides some evidence that allows us to examine these two hypotheses. The results, shown in Tables S6 and S7 of the SI Appendix Section S6.5, show that the rise of GD caused by the refugee crisis did not affect the vote shares of the governing coalition parties of the leftist SYRIZA and ANEL, but that the major opposition party Nea Dimokratia suffered significant electoral losses. This suggests that a significant segment of voters turned from the center-right Nea Dimokratia, whose electoral agenda was dominated by economic issues and the financial bail-out negotiations, to the extreme-right GD, which established itself as the fiercest anti-immigrant and anti-asylum-seeker platform. At the same time, the DID and IV results show that overall turnout increased significantly in treated islands, which suggests that the refugee crisis also enabled GD to mobilize new voters who previously had not participated in elections.
4 Conclusion
This study exploits a natural experiment in the Aegean Sea to examine the effect of exposure to the refugee crisis on natives’ support for extreme-right parties. Using two complementary identification strategies and multiple outcomes and placebo tests, we find that in municipalities and townships that experienced sudden and sizeable refugee inflows, electoral support for the extreme-right party GD increased by 2 percentage points, a more than 40 percent increase on average. These effects are further amplified by the degree of exposure, measured by the number of refugees per resident who arrived in the treated islands. However, given the size and suddenness of the refugee inflows, the ensuing chaos on affected islands just before the election, and the concurrent economic downturn caused by the financial crisis—all factors that have been theorized to fuel electoral backlash (Sniderman, Hagendoorn, and Prior Reference Sniderman, Hagendoorn and Prior2004)—we interpret the 2 percentage point increase in GD vote shares as a relatively modest effect.
These findings have important implications for our theoretical understanding of the dynamic nature of attitudes toward asylum seekers. Mirroring findings from research on how immigration to the U.S. can trigger local anti-immigrant sentiment and policies (Hopkins Reference Hopkins2010), we find strong evidence that extreme-right parties can successfully convert prevalent negative attitudes toward Muslim asylum seekers (Bansak, Hainmueller, and Hangartner Reference Bansak, Hainmueller and Hangartner2016) into vote shares in times of crisis. Previous research on the electoral consequences of refugee migration has found negative effects on support for far-right parties in Austria (Steinmayr Reference Steinmayr2016), lending support to the theory that contact between natives and refugees alleviates animus (Allport Reference Allport1979). Our study shows that hostility prevails in the Greek context, where asylum seekers quickly continue their journeys from the arrival island to the Greek mainland and extensive contact and interactions between island residents and asylum seekers are therefore not possible. Given the only temporary presence of the refugee population on most of these islands, our findings are also hard to reconcile with theories of realistic group conflict (Campbell Reference Campbell and Levine1965), which posits that conflict between the outgroup and dominant group emerges over scarce resources such as access to jobs, housing, or education. In our context, there is no specific competition between refugees and residents on treated islands over any of these resources (see Hangartner et al. Reference Hangartner, Dinas, Marbach, Matakos and Xefteris2018 for details). Our study therefore shows that mere exposure to the refugee crisis is sufficient to fuel support for extreme-right parties.
Supplementary materials
For supplementary materials accompanying this paper, please visithttps://doi.org/10.1017/pan.2018.48.