Introduction
The dynamics of agricultural systems have attracted considerable attention and have been the subject of debate in the last two decades and associated with increasing research on the adaptive capacity of these systems to cope with multiple and simultaneous shocks and changes. Today, North African countries are experiencing climate warming with an overall decrease in rainfall, particularly in the semi-arid zones that account for more than half of cultivated land in the region (Ouessar et al., Reference Ouessar, Sghaier, Frija, Sghaier, Baig, Behnassi, Barjees Baig, El Haiba and Reed2021). In this climate zone, the dynamics of settlement and land appropriation on the collective lands since the early 1960s have favoured crop cultivation, especially cereals and few forage crops associated with livestock raising (Alary and El Mourid, Reference Alary and El Mourid2005). With growing public support and guaranteed markets for cereal crops such as wheat and barley, farmers started cultivating more of these cereals, leading to unbalanced rotation systems and affecting the availability and cost of animal feed. This trend towards specialization accentuated their vulnerability to abiotic stresses, especially to the droughts that regularly affect arid and semi-arid zones. Global warming and trends in farming systems question the long-term sustainability of family farms in this zone with a decreasing trend of precipitation expected to lead to a further 10–23% decrease by the end of the 21st century (Huang et al., Reference Huang, Ji, Xie, Wang, He and Ran2016; Cherlet et al., Reference Cherlet, Hutchinson, Reynolds, Hill, Sommer and von Maltitz2018).
Walker et al. (Reference Walker, Holling, Carpenter and Kinzig2004) proposed two attributes to qualify the trajectory of agricultural production system: adaptability and transformability. Adaptability is the capacity to change some components of the system (e.g. use of inputs, marketing management) in the face of change (mainly shocks or stresses), while transformability is the capacity to change the structure of the existing system leading to the emergence of a system with new properties and mechanisms (Darnhofer, Reference Darnhofer2014; Meuwissen et al., Reference Meuwissen, Feindt, Spiegel, Termeer, Mathijs and Mey2019). The transformative capacity of a system was a novel way of thinking about system dynamics at the farm level (Darnhofer, Reference Darnhofer2014, Reference Darnhofer2020) or at the socio- and/or agro-ecosystem level (Colloff et al., Reference Colloff, Lavorel, Wise, Dunlop, Overton and Williams2016). In these approaches, transformative capacity is often considered to be the ability to reorganize a flow of resources related to on- and off-farm activities and to imagine alternative futures (Schoon et al., Reference Schoon, Fabricius, Anderies and Nelson2011). This way of thinking about farm systems stimulated a search for – and an understanding of – changes in farm systems at the interface between the buffer capacity to resist shocks, the adaptive capacity to temporally reorganize a system and the capacity to transform the system. A central question concerning the transformation of agricultural production systems is whether a given system is becoming more productive and sustainable over time, and if so, how. It is thus essential to track what stimulates both agricultural system productivity and sustainability, and which types of activities and system components (e.g. technologies) and drivers (internal and external system drivers) determine shifts in and/or the transformation of these systems.
In the agricultural economics discipline, this type of question is addressed by reviewing historical panel data and assessing productivity overtime to produce indicators of trends in technology change and the efficiency of the changes. However, although there is a growing literature on these approaches to farm dynamics and on how to address adaptive capacity in the face of the current climatic challenges, both methodological and empirical advances and evidence are required to better understand the drivers of changes in production systems. According to Jagustović et al. (Reference Jagustović, Zougmoré, Kessler, Ritsema, Keesstra and Reynolds2019), ‘a system renders a set of connected interdependent elements as a web of interrelationships, producing a pattern of behavior seen by someone as generating a purpose’. In agriculture, this definition refers to a complex, human-managed land-use system intended to provide both market and non-market goods and services (Volk and Ewert, Reference Volk and Ewert2011). However, the complexity of agricultural systems can only be tracked through relevant information across multiple scales, disciplines and time horizons, meaning that systems analysis needs different qualitative and quantitative models, tools and approaches (van Ittersum et al., Reference van Ittersum, Ewert, Heckelei, Wery, Alkan Olsson, Andersen, Bezlepkina, Brouwer, Donatelli, Flichman, Olsson, Rizzoli, van der Wal, Wien and Wolf2008). However, the availability of long data time series in comparable situations is often a limiting factor, and it is also acknowledged that many indicators of both productivity and sustainability need to be used simultaneously for a more comprehensive view of changes in agricultural production systems. The present study capitalizes on some of this recent literature (e.g. Bernues et al., Reference Bernues, Ruiz, Olaizola, Villalba and Casasús2011; Meuwissen et al., Reference Meuwissen, Feindt, Spiegel, Termeer, Mathijs and Mey2019; Muñoz-Ulecia et al., Reference Muñoz-Ulecia, Bernués, Casasús, Olaizola, Lobón and Martín-Collado2021) and builds on existing system performance indicators that refer to crop–livestock integration, diversification and intensification, linked to system sustainability.
Regarding the drivers of change, Chayanov's approach highlights the links between the ‘natural history’ of the family (marriage and children reaching working age) and the changing size of peasant farms from generation to generation (Chayanov, [1924] Reference Chayanov, Thorner, Kerblay and Smith1966). This process naturally influences the allocation of resources within the farm, particularly the labour–consumer balance between satisfying family needs and reinvestment in labour on or off the farm linked with available assets (based on knowledge and tangible goods) and their transmission depending on the household demographic parameters. According to the literature, livestock activities in mixed crop–livestock systems are being increasingly recognized as a pillar of sustainable intensification (SI) by improving resource-use efficiency for both households and territories (Herrero, Reference Herrero, Thornton, Notenbaert, Wood, Msangi, Freeman, Bossio, Dixon, Peters, van de Steeg, Lynam, Parthasarathy Rao, Macmillan, Gerard, McDermott, Seré and Rosegrant2010), thereby enabling diversification of sources of income, and increased autonomy (Ashley, Reference Ashley and Carney1999; Alary et al., Reference Alary, Corniaux and Gautier2011) and even consolidating assets and investments (Duteurtre and Faye, Reference Duteurtre and Faye2009). However, the problems concerning farm succession are often only investigated with respect to the workload created by the combination of activities or land fragmentation (Hendrickson et al., Reference Hendrickson, Hanson, Tanaka and Sassenrath2008; Ryschawy et al., Reference Ryschawy, Choisis, Choisis, Joannon and Gibon2013), while the role of livestock in the life-cycle of the family farm has rarely been considered. Moreover, in addition to the life-cycle of rural families, which is embedded in the demography and social and cultural organization of the family, understanding the dynamics of farm production systems needs to account for the permanent diversification and intensification processes that in turn influence opportunities for future generations. In the specific case of mixed crop–livestock systems, one well-known sustainable indicator is the level of integration between these two components, crops and livestock, of the system. The way both components interact is highly dynamic and also varies with the context. Changes in market prices and biotic and abiotic stresses over time may destabilize the production system and result in the dominance of one component over the other; this dominance may only last a short time (revealing the capacity of the system to withstand stresses and shocks) or a longer period, indicating the ability to change the internal structure and feedback mechanisms of the farming system, as shown by Meuwissen et al. (Reference Meuwissen, Feindt, Spiegel, Termeer, Mathijs and Mey2019).
The current paper tracks transformations of family agricultural production systems in the semi-arid zone south of the Mediterranean at an interval of 10 years, by analysing changes in assets and in the accumulation of skills. Assets refer to natural, physical, structural and live (animals) capital. The accumulation of skills refers to changes in system integration and intensification in addition to human and capacity development. Here, it was assumed that the two processes, integration and intensification, depend to a great extent on human capacity. To implement the proposed analysis, a representative community was selected in the semi-arid zone located in southern central Tunisia. A baseline survey was conducted in 2002/03 in the framework of the FEMISE project (https://agritrop.cirad.fr/540413/). A second baseline survey was conducted in 2013/14 in the framework of the Consortium Research Program on Dryland Systems (http://drylandsystems.cgiar.org/). A set of shared structural, technical, and socio-economic indicators was extracted from the two successive baseline surveys in the same zone, and the data from the two surveys were cross-compared. A complementary typology analysis of the production systems in the two periods was conducted to identify the dominant farm systems at the time of the first survey and to trace how the systems changed in the decade between the first and second survey. The analysis of long data time series has already proved to be useful in systemic approaches, particularly in ecology, as it captures the multiple effects or interrelations of internal or external factors on population dynamics (Thioulouse et al., Reference Thioulouse, Simier and Chessel2004, Reference Thioulouse, Dray, Dufour, Siberchicot, Jombart and Pavoine2018). The approach is used as cluster analysis in farming systems analysis, but its use in a dynamic perspective is only recent (see Novotny et al., Reference Novotny, Fuentes-Ponce, Lopez-Ridaura, Tittonell and Rossing2021). One of the objectives of the current study is to show that system transformation analysis through factorial analysis is useful to track possible transformation pathways. The main goal is to characterize and identify the capacity of medium-term dynamic processes of farm systems and policymaking to enhance agricultural system sustainability. The obtained results will help adjust existing conceptual frameworks to address potential intensification pathways and their sustainability.
Analytical framework for tracking systems transformation
Agricultural production systems are conceptualized in the literature as illustrative cases of socio-ecological systems (SES) due to their complexity and embeddedness in larger ecological, social and political systems (Walker et al., Reference Walker, Holling, Carpenter and Kinzig2004; Sterk et al., Reference Sterk, van de Leemput and Peteers2017). A SES refers to the interactions between the social system (considered through its social and economic dimension of agricultural decision making) and the ecological system representing the natural resource-based system. The sustainability of an SES results from a trade-off between short- and medium-term objectives of increasing productivity and profitability and the long-term objective of resource conservation. Although some systems are productivity-oriented and have substantial negative impacts on ecological processes, i.e. threaten their sustainability, others are highly conservative but productivity is very low (represented respectively by (1) and (2) in Fig. 1). In addition to this dualistic view of agriculture, a farming system based on integrated crop–livestock systems aimed at achieving household socio-economic self-sufficiency (farm system (3) in Fig. 1) has received increasing interest in the last decade. Figure 1 illustrates the cohabitation of these three family farm systems. In this approach, one can clearly distinguish the integration processes based on the creation of value by interaction between several activities (mainly valuation of products and by-products between the crop and livestock system) and intensification processes based on capital investment to improve productivity. Extensive systems are mainly based on joint social and natural viability. The aim of much of the current literature is to achieve technical, managerial or political agreement between the farming systems' orientations (intensification, integration and/or diversification) by insuring SI, which can simultaneously help increase incomes (see Lopez-Ridaura, Reference Lopez-Ridaura2005; Hammond et al., Reference Hammond, van Wijk, Teufel, Mekonnen and Thorne2021).

Fig. 1. Colour online. Theoretical illustration of three farm types and their relationship with sustainable intensification.
The applied conceptual framework used in the current paper is divided into four steps (Fig. 2): starting by identifying the agricultural production systems, building an indicator matrix for the farm system (based on intensification, diversification and viability indicators), clustering of SI-farm types and, finally, trajectory analysis based on the resulting SI-farm types in different time periods using the cases analysed.

Fig. 2. Colour online. Conceptual and methodological steps for farm profiling and trajectory analysis (SI stands for sustainable intensification).
In step 1, the main interactions that take place in family farm systems are characterized. This step assumes that family farming systems are considered as a set of components, in addition to external and internal drivers. It is focused on interactions between the four main components of small crop–livestock production systems (family, livestock, cultivated land and rangelands) using the systemic approach to livestock farming systems conceived by Landais and Faye (Reference Landais and Faye1986) and Lhoste et al. (Reference Lhoste, Dollé, Rousoseau and Soltner1993), and deepened by Dedieu et al. (Reference Dedieu, Faverdin, Dourmad and Gibon2008, Reference Dedieu, Aubin, Duteurtre, Alexandre, Vayssières, Bommel and Faye2011), using hierarchical cluster analysis (HCA).
Based on characterization of the farm type, step 2 identifies SI performance indicators that reflect the degree of intensification, diversification and viability and describe the flow of inputs and services among them. Intensification is characterized by a set of indicators based on the production and use of inputs for the three main assets: labour, land/crops and livestock. System diversification indicators reflect the different activities and their respective contribution to total family income. Attention is paid to diversification within each sub-system, i.e. livestock, crop and off-farm activities, as described in the previous step (1). The viability of the family farm system is assessed using the tri-capital framework (level of social and natural capital based on resource access, mainly water and pasture, as well as the balance between them and the level of economic viability on wheat self-consumption and monetary income per capita). Here, the viability of the family farm system is referred to as an entity.
Step 3 is methodological and aims to identify ‘Sustainable intensification-based farm systems’, hereafter called ‘SI-based farm types’, based on SI profiles using the SI performance-based indicators. A cross-cutting analysis of the different sets of indicators related to interaction (farm and off-farm assets), intensification, diversification and socio-environmental viability to characterize the different SI-based farm systems is proposed. For that purpose, a multiple factor analysis (MFA) is conducted.
Step 4 is the analysis of the trajectories of SI-based farm types in the community in Tunisia over two time-periods. For that purpose, data obtained in the two surveys have been aggregated in one database using the set of indicators identified in step 3.
Table 1 lists the most important themes used to produce indicators for the characterization and profiling of crop–livestock systems in an SI perspective.
Table 1. Thematic pillars of crop–livestock system characterization and profiling in Tunisia with the list of indicators

Material and methods
Case studies and sample
The agricultural sector in North Africa is composed of two contrasting systems: family farms and business farms in irrigated or coastal zones, while in arid and semi-arid areas, the majority of farms are small and medium and are very vulnerable due to their size and the uncertain climatic conditions (Marzin et al., Reference Marzin, Bonnet, Bessaoud and Ton-Nu2016). The current study focuses on small and medium farms in the semi-arid areas with a case study on dryland mixed systems in Tunisia (governorate of Sidi Bouzid). This area has less than 350 mm of rainfall per year and is characterized by periodic drought. The majority of these family farms combine livestock rearing (mainly small ruminants) with cereal crops and olive trees, which are the primary sources of income.
Surveys used in this study were conducted of the same rural community, even if it was not possible to link individual respondents in the two surveys due to the anonymization of the two databases. Zoghmar, the community surveyed, is located in the Sidi Bouzid province of central Tunisia (see Table 2). For the current study, two data sets were collected from family farms in 2002/03 and in 2013/14 as part of two research projects. The first data set was collected as part of the FEMISE research project on ‘Constraints to the technology adoption by small and medium farms in the arid and semi-arid areas of Maghreb’ (FEM2-02-21-05) (Elloumi et al., Reference Elloumi, Alary and Selmi2006; Shideed et al., Reference Shideed, Alary, Laamari, Nefzaoui, El Mourid, Waibel and Zilberman2007). The aim of the FEMISE project was to understand resistance to the adoption of innovation considering the complexity of the farm and community systems in the arid and semi-arid areas and their institutional and policy context. The second data set was collected in 2013/14 as part of the CRP-Drylands programme funded by the CGIAR between 2011 and 2016Footnote 1 focused on the assessment of SI in dryland systems.
Table 2. Description of the geographical and socio-economic environment of Zoghmar community in Tunisia

Both 2002/03 and 2013/14 data sets provided the raw materials used to develop a set of indicators and to assess the trajectories of change of SI-based farm types over the last 10 years. In 2002/03, the sample included 39 family farms in Zoghmar selected using stratified random sampling based on flock size. A similar family farm survey was conducted in 2013/14 as part of the CRP-Drylands programme based on random sampling. The second data collection included 238 households in the community studied.
The two-household farm surveys were based on a specific semi-structured questionnaire. Although the questionnaire was not exactly the same in both surveys, similar information was collected related to (1) family composition and characteristics (e.g. age and education level of the household head, number of active members, their education level and main social expenditure); (2) main physical assets (land, equipment and livestock); (3) cropping systems (allocation of land, level of intensification and production); (4) livestock systems (composition of the flock, sales/purchasing management and feeding system); and (5) institutional constraints (credit, supply of inputs and meat market). However, the differences between the two survey periods led to adjust our choice of indicators according to the data available for each period. The three profiling pillars were still reflected in the set of indicators for the two periods (see Table 1).
Method
Principal component analysis (PCA) helps describe a large data set with j individuals and i variables, using a smaller number of uncorrelated variables (Sârbu et al., Reference Sârbu, Nascu-Briciu, Kot-Wasik, Gorinstein, Wasik and Namies2012). The reduction in the number of variables is achieved by generating a new data set of continuous variables called ‘principal components’ or ‘factors’. This data transformation retains as much information as possible from the original data set. The first factor embeds most of the data variation; the second principal factor is orthogonal and covers much of the remaining variation, and so on (Keenan et al., Reference Keenan, Valverde, Gormley, Butler and Brunton2012). Continuous variables generated from the PCA can then be considered for cluster analysis with no risk of variable correlations.
Cluster analysis is a multivariate analysis technique performed on the principal components. With no noise in the data (denoising is insured by PCA), clustering is more stable than that obtained from the original distances. In the current study, cluster analysis of the early first dimensions of the PCA was performed to account for most of the variance of the sample. HCA uses a defined metric to form clusters sequentially, grouping the most similar objects first, and these initial groups are then merged based on their similarities (Goyeneche et al., Reference Goyeneche, Roura and Di Scala2014). As the similarity decreases, all groups are merged into a single cluster (Keenan et al., Reference Keenan, Valverde, Gormley, Butler and Brunton2012). Ward's method (Ward, Reference Ward1963) and the squared Euclidean distance are used as a metric to establish clusters. With the Ward method, each observation starts in one cluster, and pairs of clusters are merged as one moves up the hierarchy. For this purpose, an appropriate metric (distance between pairs of observations) is required. The Euclidian distance was used, which is the most widely used for clustering purposes and is defined as the length of the line segment connecting two points. It can be calculated as shown in Eqn (1) (Córcoles et al., Reference Córcoles, Juan, Ortega, Tarjuelo and Moreno2010):
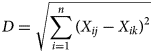
where Xij is the value of the indicator i of sample j and Xik is the value of the indicator i of sample k. The cluster analysis was performed with the first factors of the PCA, representing more than one-third of the variability in Tunisia in 2003 and 2014, respectively.
In the second step, a search was performed for links between farm assets (see description of the farming system) and the four sets of variables reflecting, respectively, the intensification, diversification and viability related to natural resource use and socio-economic viability. The variables that described farm assets were classified in three themes illustrating the three main components of the farming system: household characteristics, land and crop area, and the size of the sheep and goat flocks. The data sets were organized in seven themes. MFA was used to analyse the proximity between the composition of the assets and synthetic indicators reflecting diversification, intensification, and natural and human viability. MFA is a comprehensive analysis that considers the number and strength of relationships between indicators within their respective theme and between the themes (see Alary et al., Reference Alary, Messad, Aboul-Naga, Osman, Daoud, Bonnet and Tourrand J2014). The technical details of MFA are described in Escofier and Pagès (Reference Escofier and Pagès1983, Reference Escofier and Pagès1994). The current analysis focused on within-group variables (themes) to compare changes in interactions between the assets and the degree of intensification, diversification and viability over the decade concerned. This analysis compared the contribution of each set of variables to the overall typology. The quantities denoted by Lg (0 ⩽ Lg ⩽ 1) give a measurement of links between variables vk in group X (k) and the factor of rank α denoted zα (i.e. the linear combination of all the variables of the k groups that summarizes a certain amount of the total variance). Proximities measured by Lg between tables allowed to conclude on the existence of common factors for all or part of the set of tables involved, indicating whether building a common typology was useful or not. In the analysis, the standard liaison indicator Lg, named RVFootnote 2 was used. All the calculations were performed using XLSTAT software (XLSTAT, 2020). The current cross-cutting analysis of the different sets of indicators (asset, diversification, intensification and viability) using MFA led to the identification of SI-based farm types.
Finally, a second MFA was conducted on the two survey populations (2002/03 and 2013/14). An indicator database comprising the two data sets collected in 2002/03 and 2013/14 was compiled; clustering was based on the main factors of the MFA applied to the whole survey population. Clustering may (or may not) result in farm profiles that are exclusive for a given period of time, along with some profiles based on observations made in both 2003 and 2014. This analysis allowed to identify and analyse the dynamic change in the SI-based farm type and, consequently, the trajectories followed by the local population in the decade between the two surveys.
Results
Changes in the structural characteristics of farm types over a decade
HCA on the main factorial axis of the MFA allowed identifying three types of family farms in each survey (Table 3). In 2002/03, the first farm type, small-scale farms (T1a), was characterized by an average area of around 9 ha including rainfed and irrigated land. Medium-scale farms (T2a) and (T3a) were 24 and 27 ha in size, respectively, the majority of land was located in rainfed areas. These two medium-scale farm types were defined based on the size of their flock and on the diversification of on-farm and off-farm activities. Type (T3a) had the biggest flocks with around 120 sheep and goats. Type T2a was the most diversified, and included olive groves (around 4 ha) and an average of two family members working off farm.
In 2013/14, the first factor of differentiation across farm types still contrasted large- and small-scale farms, and there was an increase in inequality in access to land. Around 0.07 of the population owned c. 41 ha of agricultural land, while around 0.93 owned an average of c. 10 ha, almost only located in rainfed areas. A new typology criterion referring to land access emerged in the 2014 typology, which referred to small-scale farm type (T2b). This type included farmers who had purchased as opposed to inherited their land. Moreover, in contrast to type T1b, type T2b funded their farm activity through off-farm activities. Another change was the overall reduction in the size of the sheep and goat flocks, with a maximum mean of 64 head per flock in 2014 compared to 104 in 2002/03.
Understanding these changes requires considering the contribution of the different processes of intensification, diversification, and social and natural resource use to farm viability.
Identification of trajectories using SI performance indicators between 2003 and 2014
A cross-cutting analysis of the different sets of indicators, or ‘themes’ related to the main assets, intensification, diversification and viability, was conducted using MFA to identify the different SI profiles.
Figure 3 shows the relationships between the composition of the assets (family, land, livestock) and the level of intensification, diversification and viability in the two periods the surveys were conducted. One important result was the critical weight of the intensification process in sample populations (with standard r correlation (RV) between 0.57 and 0.71 of the total variability). In 2002/03, the intensification process was highly proportional to the land and livestock assets and, to a lesser extent, to the degree of diversification. In 2013/14, the distance between the degree of intensification and the composition of the assets or the process of diversification decreased. More specifically, in 2013/14, the intensification process (based on the crop system) aggregated part of the sample population on the first factor of the MFA quite far from diversification based on livestock or off-farm activities, in contrast to in 2002/03. Additionally, the level of viability of farms in the sample population surveyed in 2013/14 was fully linked to the level of intensification. Natural resource use was another significant component that differed between the trajectories in Zoghmar. Management of resource use (‘natural resource and mobility’), which was located close to livestock assets in 2002/03, was closer to the ‘family’ component in 2013/14.
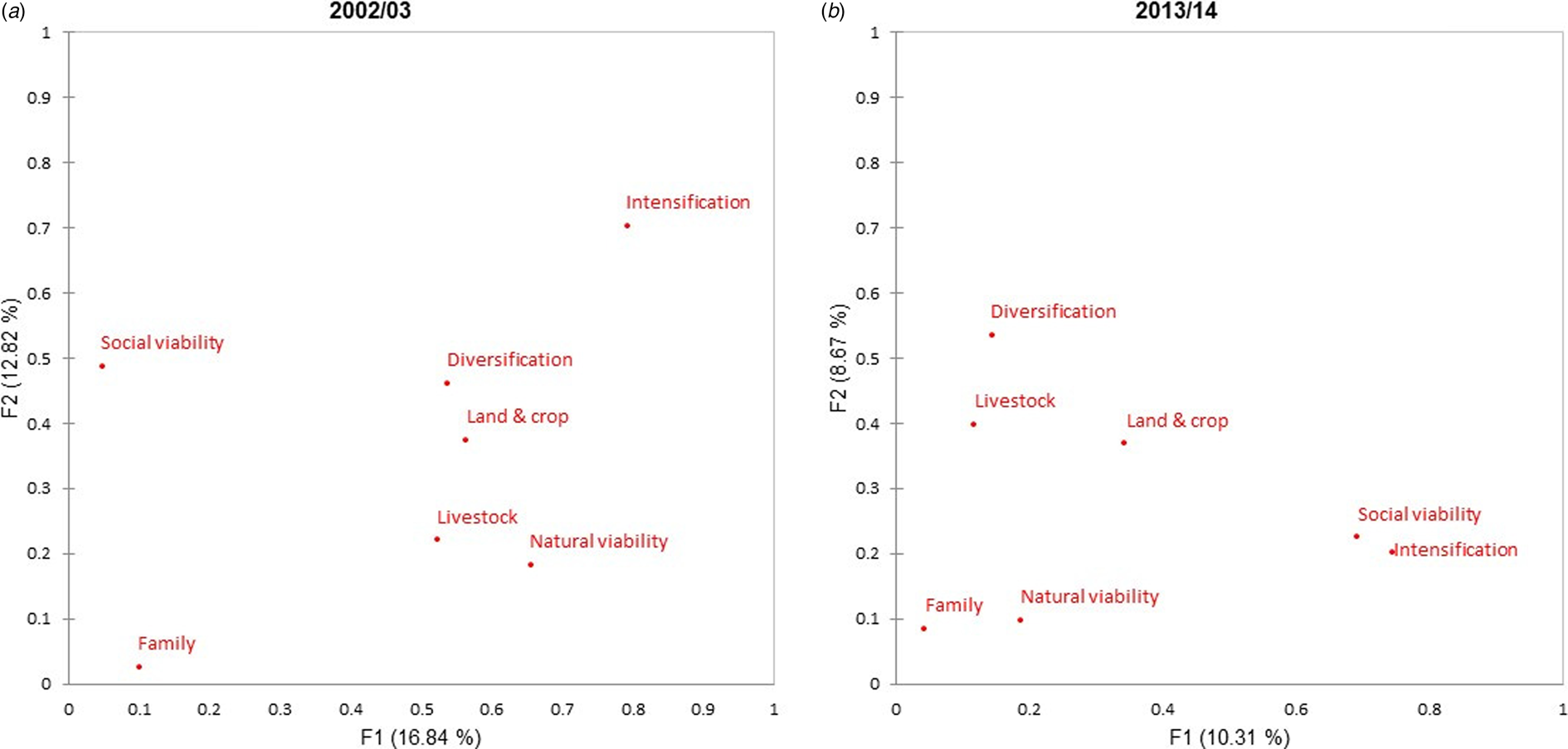
Fig. 3. Colour online. Projection of the composition of assets and levels of intensification, diversification and viability for the two periods, i.e. (a) 2002/03 and (b) 2013/14 in Zoghmar (Tunisia) (factors 1 and 2 represent 29.66% of variability in 2002/03 and 18.99% in 2013/14).
Analysis of SI-based farm types in the two survey periods
The current section presents the main results of the MFA of all the sample populations surveyed in 2002/03 and 2013/14, counting in total 281 household farms, by focusing on the respective trajectories to identify their transformative capacities. Based on the coordinates of the three first factors of the MFA representing 0.26 of the variability, an HCA of the whole sample was conducted. Table 4 lists the descriptive statistics of the SI-based farm types that resulted from the cluster analysis.
Table 4. Results of the cluster analysis (sample: 281 family farms)
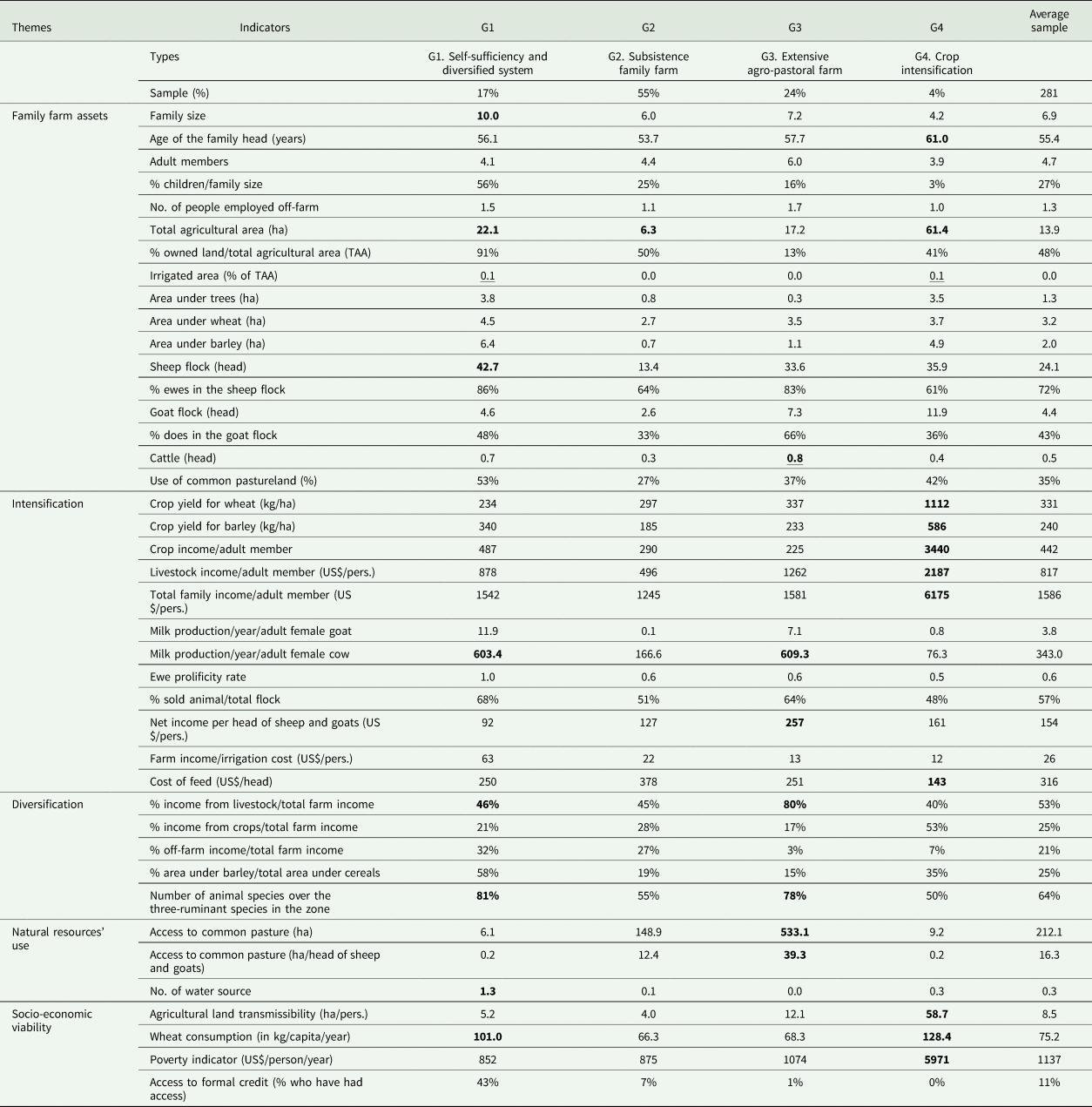
Descriptive statistics of the integrated approach to the SI profiles.
Values are marked in bold the main differentiation values between the types.
First, three main factors differentiated the SI-based farm types across farmers over the 10-year period. The first factor differentiated the SI-based farm types according to physical assets (land and livestock) and to the degree of intensification based on the outputs generated per unit of asset (productivity and net income per ha or per livestock unit). This factor differentiated groups (G1) and (G4) (farms comprising more than 20 ha in size on average) from the two other groups (G2) and (G3). However, group (G4) constitutes a recent group of families who invested in large areas of land in the dry agricultural area: around 61 ha of agricultural land or about 15 ha per active member compared to around 5.4 ha per working family member in group (G1). Groups (G1) and (G4) differed mainly according to their labour productivity (net income per family member) along the second factor. In the group (G4), labour production was 2.5 times higher for the overall livestock rearing, up to seven times higher for crops, than in the group (G1). Their agricultural performance was partially due to inputs and labour intensification. Along the third factor, the two last groups (G2) and (G3) differed according to the type of diversification. Group (G3) diversified through livestock rearing thanks to access to pasture, while group (G2) diversified mainly through off-farm activities, mostly occasional jobs outside the farm.
Figure 4 shows the dynamics of system transformation with changes in the frequency of the identified SI-farm types between the two survey periods. The figure highlights some challenging transformations in this semi-arid zone. Notably, self-sufficiency systems partially or completely disappeared or were transformed in the period between the two surveys to be replaced by the low-endowed subsistence types oriented to farm and off-farm diversification. More precisely, the distribution of the surveyed population shows that 87% of the rural population aimed at self-sufficiency (G1) in 2003, while around 62% were in the group of subsistence families (G2) in 2014, and took occasional off-farm jobs to sustain their livelihoods. Overall, the population in the rainfed zone thus became impoverished in the decade between 2002/03 and 2013/14. On the other hand, 28% of the population developed a diversified livestock activity system mainly based on grazing (G3). A type oriented to crop intensification and specialization also emerged in 2013/14 in the zone where irrigation was introduced (corresponding to G4). In areas where irrigation was not introduced, a similar group (G3) developed that diversified their livestock system towards dairy cattle or sheep fattening in agropastoral areas.
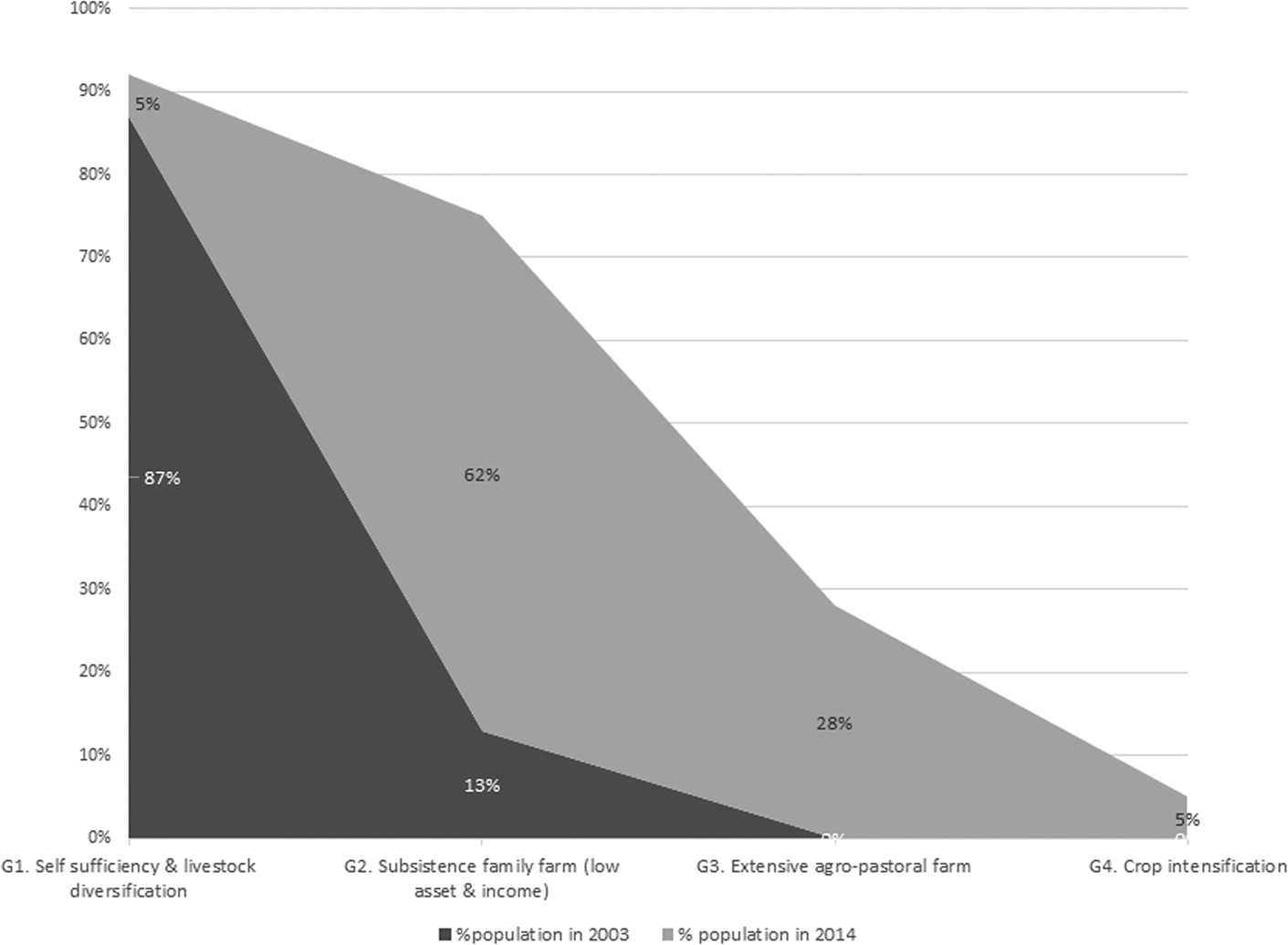
Fig. 4. Trajectory of the SI-based farm types between 2002/03 and 2013/14 in the community studied in Tunisia.
Discussion
The role of household demographic factor in the trajectories of different family farms types
The descriptive analysis of FS over the decade from 2002/03 to 2013/14 revealed an increasing number of small-scale farms associated with the process of land transmission and land fragmentation, thereby jeopardizing intergenerational sustainability, as also reported by White (Reference White2012) and Bernues et al. (Reference Bernues, Ruiz, Olaizola, Villalba and Casasús2011) in different southern countries. In the current study, the land transmission process concerned a non-negligible proportion of medium and large-size farms in which the family head was 55–60 years old in 2003. In the majority of these rural families, the limited number of people of working age at the farm level prevented keeping a large flock. The limited number of people of working age has also been accentuated with the increasing trend of rural exodus in this dry region. However, Table 3 shows overall diversification of livestock activities towards cattle and goats. This reflects the current trend towards increased livestock rearing in this semi-arid environment, especially of dairy cattle if water is accessible, or of goats in dry areas. In addition, a new type of large agropastoral farm emerged in the rainfed areas when the family members of working age were not able to quit agriculture, with the parallel emergence of a family farm type with increased crop intensification and specialization, already reported by Alary et al. (Reference Alary, Moulin, Lasseur, Aboulnaga and Srairi2019) in a comparative analysis of case studies in north and south Mediterranean regions.
Table 3. Cluster analysis of farming systems in Zoghmar, Tunisia, in 2003 (a) and 2014 (b)

In italic the average for the sample in 2003 and 2014. these numbers can be put in normal.
The current results enabled to identify two SI-based farm types to serve as SI benchmarks (1) the ‘traditional’ extensive agropastoral system oriented to pastureland restoration and (2) a more integrated crop–livestock system based on crop and livestock diversification (notably with dairy cattle or sheep and goat fattening). These two SI-based farm types are deeply dependent on and specific to the semi-arid context but reveal two viable systems mainly oriented towards agricultural activities. In both cases, livestock extensification (based on natural resources) or diversification with the expansion of dairy cattle or small ruminant fattening has emerged as a major driver of subsistence and even of SI in this semi-arid environment, as also observed in sub-Saharan African countries (Ellis, Reference Ellis2000; Barrett et al., Reference Barrett, Reardon and Webb2001; Niehof, Reference Niehof2004). This trend shows that the medium-term viability goals should be increasingly sought when the aim is to develop innovative activities in agropastoral systems in these harsh environments.
From a temporal perspective, the analysis of the transformation of the agricultural production system through diversification and intensification revealed a change in the trajectories of SI pathways over the 10-year period. If in 2003, the level of intensification was closely linked to the natural and physical assets, in 2014, the process depended more on external factors. Among external factors, the development of irrigation infrastructure or access to external financial resources through remittances or subsidies may have played a major role. However, the increasing distance between intensification and physical or natural assets is not a guarantee of sustainability in terms of reproducibility. Nonetheless, SI appears to be closely linked with the groups that increasingly diversified their livestock activities, which was mainly linked to the availability of labour in sheep and goat grazing systems (e.g. G3) and monetary capital in intensified crop–livestock systems (G4) (See Table 4).
The results also underline the importance of household factors (e.g. the age of the farmer, the size of the family and the level of education) in the family farm trajectories (e.g. Darnhofer et al., Reference Darnhofer, Bellon, Dedieu and Milestad2010; Tenza et al., Reference Tenza, Martínez-Fernández, Pérez-Ibarra and Giménez2019) in relation to the combination of family farm assets, in particular, the value of the assets (Corsi, Reference Corsi2006). In addition to these structural factors, social changes are also hypothesized to influence farm trajectories. For instance, the unwillingness of farm children to continue traditional farming may explain some of the dynamics such as the abandonment of traditional livestock raising in some areas (Caballero, Reference Caballero2001). More broadly, the reluctance of young people to continue ‘business as usual’ is a major limitation to farm reproducibility (Bernues et al., Reference Bernues, Ruiz, Olaizola, Villalba and Casasús2011) and further explains the shift towards more intensified and specialized systems in many cases (Kazakopoulos et al., Reference Kazakopoulos, Alexandridis and Zioganas1996; Riedel et al., Reference Riedel, Casasús and Bernués2007).
SI profiles shift towards more agroecological systems
When referring to the theoretical framework proposed in the first section, the specialized crop systems with input intensification (G4) was the most socio-economically viable group in terms of profit and income. However, this system is extremely dependent on the market for supplies of inputs and consequently on price variability, and is also fragile due to the increasing scarcity of water in this type of environment. The integrated crop–livestock systems with diversified livestock activities based on natural resource use (G3) was the most sustainable group based on the SI-performance indicators of intensification, diversification and the viability indicators like the monetary poverty and food security (see Table 4). An SI-based farm type that depends on the market both for their supply of inputs and their food security also emerged. This ‘subsistence’ type farm (G2) is the most fragile.
Analysis of the SI-based farm types and their trajectories over the decade between the two surveys led to conclude that the most viable SI pathway in this semi-arid zone is based on livestock diversification, which may differ according to the landscape, and that water and management are the driving factors. Conventional intensification based on increased use of inputs per unit may improve socio-economic viability but does not guarantee long-term environmental sustainability. Based on crop–livestock integration, the self-sufficiency model is shifting towards more diversification in non-farm or off-farm activities. However, this type of diversification does not necessarily improve long-term socio-economic viability. So, although the diversification largely embedded in the buffer or adaptive capability at the family farm level reveals a transformative capability (based on the framework of Darnhofer, Reference Darnhofer2014), this transformative capacity does not guarantee the sustainability of the systems and even less the future viability of SI.
More broadly, based on the most sustainable pathways identified in the current study, the future pathways of SI in the rainfed areas of North Africa should be sought at the interface between landscape management (referring to natural resources) and water system at the territorial level that determine livestock diversification; but also, through innovations and sound policies and measures for sustainable resource use that integrate local knowledge and practices. The results confirm recent international calls for a switch from SI to ‘agroecological’ food system approaches that represent a promising pathway by promoting economic diversification and co-creation of knowledge (FAO, 2019; HLPE, 2019), which is particularly true in North Africa where available water is scarce and soil degradation is high (Ameur et al., Reference Ameur, Amichi and Leauthaud2020). In this line of thought, as observed in study area in Tunisia, agroecological intensification provides a model for small farms that are diverse, integrated and use small quantities of inputs (Mockshell and Kamanda, Reference Mockshell and Kamanda2018). The main goal of agroecological intensification is to insure the long-term balance between food production and the sustainability of natural resources. Incorporating the principles of the agroecology in the overall food system approach will make it possible to conceive and develop other socio-economic alternatives based on diversification along the value chains (Losch, Reference Losch2016). Notably, in the study area, some common factors characterizing this diversity and complementarity (integration) emerged in the crop–livestock association and exploiting the diverse range of crop and livestock products and by-products that involve different members of the family (men or women, old or young). However, this system faces a variety of difficulties, in particular highly uncertain access to land, whether cultivated (with very variable user rights) or grazed (with fluctuating rights of access that depend on tribal or intergenerational kinships or state regulations). Along with the value chains, there is a kind of westernization of urban spheres and shrinking of value chains for local products. These factors need to be taken seriously when searching for new farming patterns at the territorial level.
Methodological insights
From a methodological point of view, using a holistic approach to investigate the transformation of the agricultural production system via the degree of diversification and intensification enabled to consider the overall sustainability of the system in both the short and long term. The results confirm the value and scope of a systemic and holistic approach to farming systems when addressing farm trajectories or transformation pathways. The holistic approach is particularly appropriate in the case of small family farms where sustainability depends on permanent adjustments to the combination of activities and assets and where the outcome of the system as a whole is more important than that of each separate component (Gibon et al., Reference Gibon, Sibbald, Flamant, Lhoste, Revilla, Rubino and Sørensen1999; van Keulen and Schiere, Reference van Keulen and Schiere2004; Darnhofer et al., Reference Darnhofer, Bellon, Dedieu and Milestad2010). These principles are even more important in mixed crop–livestock farms because of the close interactions between the units that offer plenty of room for manoeuvre to improve overall farm performance (Schiere et al., Reference Schiere, Ibrahim and Van Keulen2002; Hendrickson et al., Reference Hendrickson, Hanson, Tanaka and Sassenrath2008).
The originality of the current approach consisted in re-using farm data collected in surveys conducted 10 years apart and reconstituting longitudinal data sets. The holistic approach aimed at combining data from two distinct surveys through factorial analysis proved to be very rich in information regarding the transformation pathways and is thus highly recommended given the importance of this type of study in understanding the farm management and development strategies required to survive and adapt (Rueff et al., Reference Rueff, Choisis, Balent and Gibon2012). The approach gets round one of the weaknesses of typological analyses that only provide a snapshot of diversity and hence make it difficult to understand the dynamics of the systems. Joint analysis of the two data sets acquired at an interval of 10 years made it possible to capture dramatic changes that it would not have been possible to observe through a one-off study of diversity of system, even if some complementary qualitative data were able to capture some trends. Finally, the holistic approach linked diversification and intensification with the different time factors involved in the dynamics of change of the local family farm population, particularly concerning the short-term household viability and long-term life-cycle of natural assets and the reproducibility of the family farm.
The analysis showed that socio-economic viability and environmental sustainability depend on territorial and extraterritorial factors, notably landscape (or resources), access to water and management, that are difficult to fully address at the family farm level. In other words, sustainability pathways should be sought at different spatial and organizational scales. It is also shown that one major factor responsible for socio-economic viability in semi-arid areas is embedded not only in diversification, but also in the interaction between the different activities, including off-farm activities. This diversity is well described from an economic point of view in agropastoral and rural systems (Reardon et al., Reference Reardon, Delgado and Matlon1992; Lam Do et al., Reference Lam Do, Nguyen and Grote2017). As shown, diversity can also be sought in the family and social organization at the farm and territorial levels, as a pillar of the sustainability of the ecosystem at the interface between the human and natural resources components. Cross-analysis of data sets using factorial analysis made it possible to identify the increase in small-scale farms and is an original way to observe the transformation of agricultural systems over time.
Conclusion
The current study identified SI-based farm types over time and produced further evidence for the current large-scale trend in family farm impoverishment in semi-arid areas when farmers are unable to diversify their livestock activities due to labour constraints (both availability and preference) or have limited access to their own or collective natural resources. The holistic approach that was used made it possible to grasp the scale of the phenomenon of reduction of land ownership that concerns the majority of family farms as a result of the process of land transmission (based on inheritance rules and population dynamics) in dryland regions, with an overall reduction in the size of household flocks of sheep and goats. This reduction can be explained by the fragmentation of land leading to the departure of household members of working age. However, emigration has not compensated for the reduction in land available to family farms, resulting in a drastic reduction of the overall viability of family farms. This change explains the trend in cropping systems, with an increasing proportion of land allocated to wheat than to barley since the first survey. More generally, this rural dynamic is reflected in a highly representative farm type whose viability depends on a diversity of activities in- and off-farm. The diversification through non-agricultural activities is the most advanced on small-scale farms with very low viability performance indicators (monetary poverty or food security). These results imply that for these types of farms that represent the majority of the farm systems in the study area diversification is mainly a way to cover short-term needs. The sustainability of the farm systems between generations (based on the inheritance or transmissibility capacity of land and livestock according to the number of children in the household) of these family farms is strongly compromised by their inability to emigrate, itself linked to the need for an increase in human capability, especially through education.
So, concerning the question whether a given system is becoming more productive and sustainable over time through transformation, the current results revealed that overall sustainability depends more on the diversification of farm activities than on intensification in this semi-arid environment. However, the trend to diversification reflects the main challenges currently observed in North African rainfed areas associated with land assets. Moreover, the widely observed diversification trend through livestock integration in rural areas requires both more downstream knowledge and more investment, notably throughout the local food system. More generally, the results reveal the urgency of innovative measures, whether political or economic, to support the trajectory of these systems oriented towards the diversification of livestock products, more particularly in these semi-arid zones where water pressure and temperatures frequently compromise agricultural crops. Finally, the current case study is a representative example of many other farming systems facing the dilemma of population growth in areas of very low productivity.
Acknowledgements
The data used in the current study were collected in the framework of two collaborative research projects: the FEMISE project in 2002/03 and the Consortium Research Program on Dryland Systems (CRP-DS) in 2013/14. The authors sincerely thank all the research partners involved in the intensive data collection: ICARDA (International Center for Agricultural Research in the Dry Areas), INRAT (Institute National de Recherche Agronomique in Tunisia) and CIRAD (Centre International de Recherche Agronomique pour le Developpement). The research presented in this paper was conducted within the CRP Wheat and the CRP Livestock (2018–2021). The authors thank all donors and organizations who globally supported the work of the CGIAR Research Program on Wheat and Livestock through their contributions to the CGIAR system.
Financial support
CGIAR Research Program on Wheat and the CRP Livestock through their contributions to the CGIAR system.
Conflict of interest
None.