Prediabetes is an intermediate glycaemic state between normoglycaemia and diabetes. Prediabetes is characterised by impaired glucose tolerance, impaired fasting glucose or elevated glycosylated HbA1c levels(1). The International Diabetes Federation reported that the global prevalence in adults with prediabetes which is defined as impaired glucose tolerance was approximately 7·5 % in 2019 and will increase to 8·0 % by 2030(2). In China, a nationally representative survey conducted in 2013 showed that 35·7 % of Chinese adults had prediabetes, which is defined as having an HbA1c level of 5·7–6·4 %, a fasting plasma glucose level of 5·6–6·9 mmol/l or a 2-h plasma glucose level of 7·8–11·0 mmol/l(Reference Wang, Gao and Zhang3). Individuals with prediabetes have a 4–19 % (varied based on different abnormal parameters) annual risk of developing type 2 diabetes (T2D)(Reference Gerstein, Santaguida and Raina4,Reference Bansal5) . Furthermore, prediabetes has been associated with an increased risk of structural brain abnormalities, CVD and all-cause mortality(Reference van Agtmaal, Houben and de Wit6,Reference Cai, Zhang and Li7) . Moreover, the control of prediabetes may play an important role in the occurrence and progression of T2D, CVD and death(Reference Cai, Zhang and Li7,Reference Evert, Dennison and Gardner8) . Therefore, the identification of prediabetes risk factors is crucial for the early prevention and control of these complications.
Dietary fibre is an important component of a daily diet. It can be divided into soluble and insoluble fibre types(Reference Weickert and Pfeiffer9). Generally, soluble fibre is viscous, gel-forming and highly fermentable, while insoluble fibre is non-viscous, not gel-forming and moderately fermentable(Reference Weickert and Pfeiffer9). Gut microbes can ferment some types of dietary fibre (primarily soluble fibre) to produce SCFA(Reference Weickert and Pfeiffer9,Reference Koh, De Vadder and Kovatcheva-Datchary10) . Research has suggested that SCFA are the endogenous ligands for two G protein-coupled receptors (GPR41 and GPR43)(Reference Brown, Goldsworthy and Barnes11), which contribute to the regulation of insulin secretion through activating these receptors(Reference Pingitore, Gonzalez-Abuin and Ruz-Maldonado12,Reference Kimura, Ozawa and Inoue13) . Diet-derived SCFA propionate could improve β-cell function in vivo, and in vitro results have demonstrated that this beneficial effect may occur through stimulation of insulin secretion and inhibition of apoptosis(Reference Pingitore, Chambers and Hill14). Dietary soluble fibre may also slow the absorption of macronutrients, thereby reducing the rate of postprandial blood glucose absorption and blood insulin level(Reference Lattimer and Haub15). Taken together, the prior evidence suggests that dietary fibre intake may have potential protective effects on glucose metabolism disorders. Moreover, the main food sources of dietary fibre are cereals, fruits, vegetables and beans, which typically provide a distinctive mixture of both soluble and insoluble dietary fibres(Reference Weickert and Pfeiffer9). Different food sources for fibre showed different health benefits(Reference Partula, Deschasaux and Druesne-Pecollo16). We hypothesised that different dietary fibre types and different sources of dietary fibre were differentially associated with the risk of prediabetes.
Recent reviews(Reference Weickert and Pfeiffer9,Reference Gowd, Xie and Zheng17) and meta-analyses(Reference Yao, Fang and Xu18,Reference Wang, Fang and Gao19) investigated the association between dietary fibre intake, including total dietary fibre, soluble fibre, insoluble fibre, cereal fibre, fruit fibre, vegetable fibre and bean fibre, and the risk of T2D, and these studies have yielded mixed results. In addition, these studies mainly consisted of American and European populations, and the findings might not be generalisable to the Chinese population with different sources of dietary fibre intake, different lifestyles, metabolic characteristics and genetic predisposition in relation to T2D(Reference Chan, Malik and Jia20). To date, few studies have focused on the association between dietary fibre and prediabetes in the general adult population. To fill this knowledge gap, we investigated whether dietary fibre intake and different food fibre sources were prospectively associated with the risk of prediabetes in a general Chinese population of adults.
Methods
Study population
The Tianjin Chronic Low-grade Systemic Inflammation and Health Cohort Study is an ongoing, dynamic prospective observational study with men and women aged 18–90 years, living in Tianjin, China. Details of the cohort have been published elsewhere(Reference Hu, Gu and Lu21,Reference Zhang, Kumari and Gu22) . Briefly, the cohort study was established in May 2013 and is ongoing. At baseline, participants received comprehensive health examinations, which included administering a questionnaire to assess diet and lifestyle factors. Follow-up visits were repeated annually. Written informed consent was provided by all participants. Ethical approval for this study was provided by the Institutional Review Board of Tianjin Medical University (approval number: TMUhMEC 201430).
From May 2013 to December 2019, a total of 32 702 participants in a rolling recruitment process were included in this study. Participants were excluded if they had missing data of dietary fibre or reported extreme total energy intake (defined as < 2·5 % or > 97·5 % percentiles of intake). Furthermore, we excluded participants who had self-reported CVD, self-reported cancer, physician-diagnosed diabetes or prediabetes at baseline. Participants were also excluded if they were lost to follow-up before December 2019 (lost to follow-up rate: 11·0 %). Finally, 18 085 participants (mean age: 38·5 (sd 10·4) years; follow-up rate: 89·0 %) were included in the analysis (Fig. 1).
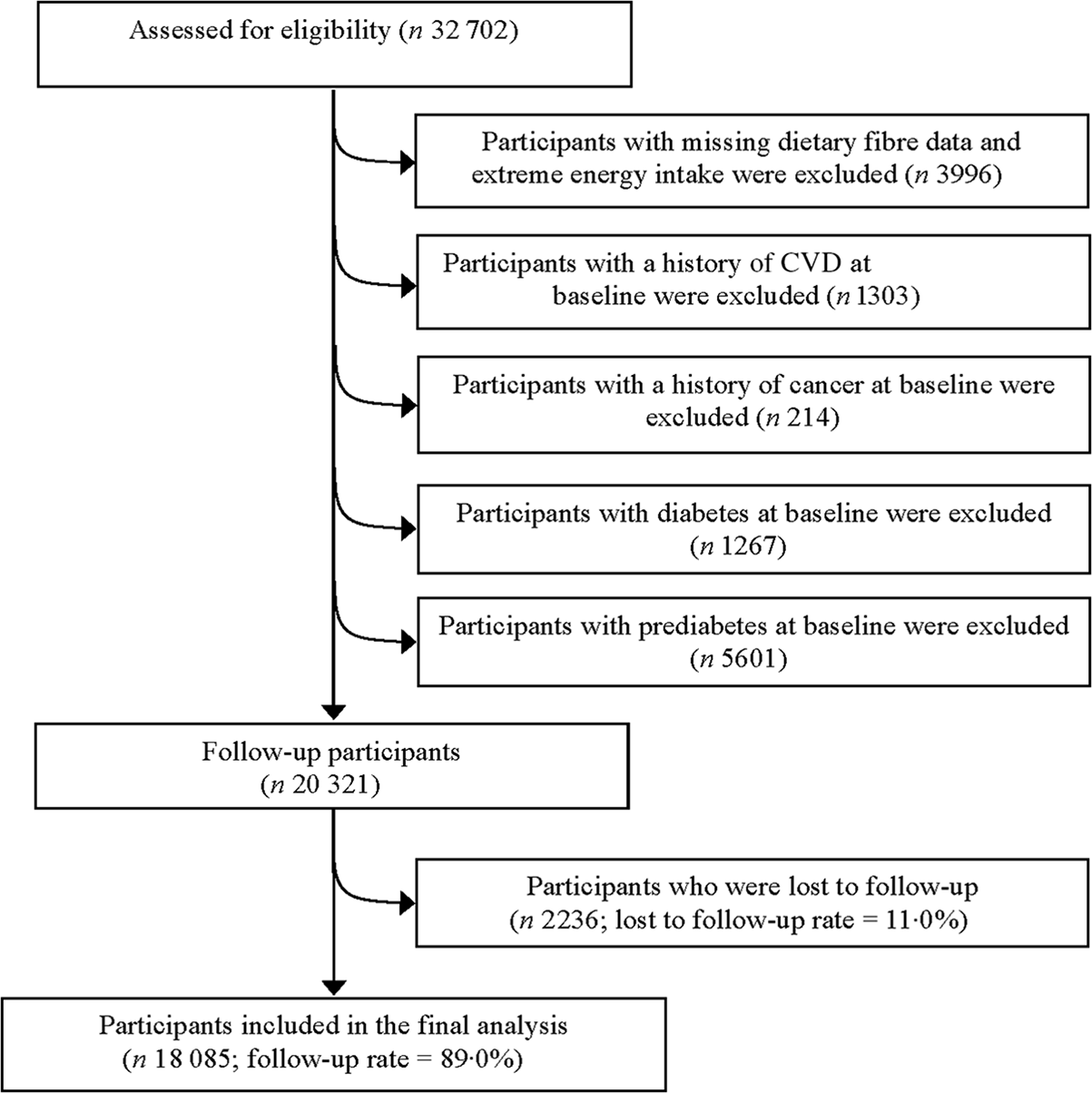
Fig. 1. Participant flow chart of the study.
Diagnosis of prediabetes
During annual health examinations, fasting plasma glucose was measured by the glucose hexokinase method using a blood sample after 12-h overnight fasting. Impaired glucose tolerance was determined by the 75 g oral glucose tolerance test, which was completed after an overnight fasting. HbA1c was measured using the HLC-723 G8 automated HPLC analyser (Tosoh). Prediabetes was defined as fasting plasma glucose of 5·6–6·9 mmol/l, and/or 2-h plasma glucose of 7·8–11·0 mmol/l in the oral glucose tolerance test, and/or an HbA1c of 5·7–6·4 % (36–46 mmol/mol) without a history of diabetes according to the American Diabetes Association criteria(1).
Assessment of dietary intake
Dietary information was collected using a self-administered semi-quantitative FFQ. The FFQ assessed the usual intake of 100 food and beverage items over the last month and provided seven frequency categories for foods ranging from ‘never or hardly ever’ to ‘two or more times a day’ and eight frequency categories for beverages ranging from ‘almost never drink’ to ‘four or more times a day’. A typical portion size was prespecified for each food item (e.g. the standard serving size of mushrooms was 50 g for men and 30 g for women(Reference Zhang, Gu and Lu23)). Daily energy and nutrient intakes were estimated from the food items consumed according to the 2009 Chinese Food Composition Table(Reference Yang24). Due to the very low trans-fatty acid intake in our study population and lack of data on trans-fatty acid intake in the Chinese Food Composition Table, trans-fat intake was not included in the calculation of total fat intake. The FFQ has been validated using four 4-d weighed diet records (covering three non-consecutive weekdays and one weekend day) among 150 participants from the Tianjin Chronic Low-grade Systemic Inflammation and Health study(Reference Zhang, Kumari and Gu25). The Spearman correlation coefficients between FFQ and diet records were 0·49 for energy intake and 0·35–0·54 for nutrients. The energy-adjusted correlation coefficients between estimates from the FFQ and diet records ranged from 0·49 to 0·70 for dietary fibre (total fibre, soluble fibre, insoluble fibre, cereal fibre, bean fibre, vegetable fibre and fruit fibre). To correct for potential measurement error, each nutrient was expressed as intake per 4184 KJ (1000 kcal) by the nutrient density method(Reference Walter26).
Assessment of covariates
Information on age, sex, smoking status (current smoker, ex-smoker and non-smoker), alcohol drinking status (everyday drinker, sometime drinker, ex-drinker and non-drinker), educational level, occupation, household income/month, personal and family medical history was collected from a structured questionnaire at baseline. Physical activity was assessed using the short International Physical Activity Questionnaire form(Reference Craig, Marshall and Sjostrom27). The short International Physical Activity Questionnaire form data were then used to estimate total weekly hours of metabolic equivalents (MET-h/week). Body height and weight of participants were measured while wearing light clothing and no shoes. BMI was calculated as body weight (kg) divided by height squared (m2). Waist circumference (WC) was measured to the nearest 0·1 cm at the umbilical line with participants standing and breathing normally.
Venous blood samples were collected after an overnight fast. Total cholesterol, TAG, HDL-cholesterol and LDL-cholesterol concentrations were analysed using an automatic biochemistry analyser (Roche Cobas 8000 modular analyser). Blood pressure was measured three times using the TM-2655 device (A&D Company Ltd) after at least 5 min of rest in a quiet room. The metabolic syndrome was defined following the criteria of the American Heart Association scientific statements of 2009(Reference Alberti, Eckel and Grundy28). Information about long-term use of medications (more than 6 months) was collected at baseline using structured questionnaires.
Statistical analysis
Normality was assessed for each continuous variable by the one-sample Kolmogorov–Smirnov test (n ≥ 2000). Because the distributions of all continuous variables were non-normal, the natural logarithmic transformation was applied to normalise the data before statistical analysis. Baseline characteristics of the study participants were described using geometric means (95 % CI) for continuous variables and percentages for categorical variables. Differences in baseline characteristics between those with incident prediabetes and those without incident prediabetes were tested using ANOVA for continuous variables and logistic regression analysis for categorical variables. In addition, we compared the differences between those excluded from the study and the final study population.
The follow-up time for each participant was calculated from the date of the baseline dietary survey until the date of the first prediabetes diagnosis, the date of loss to follow-up or the last time of follow-up (31 December 2019), whichever occurred first. Cox proportional hazards regression models were used to estimate hazard ratios (HR) and 95 % CI for the associations of dietary fibre intake (total fibre, soluble fibre and insoluble fibre) quartiles with the risk of prediabetes. The proportional hazards assumption was assessed using Schoenfeld residuals, and no violation was found. We fitted four statistical models. Model 1 was adjusted for age, sex and baseline BMI. Model 2 was further adjusted for smoking status, alcohol drinking status, educational level, occupation, household income per month, physical activity, family history of disease (including CVD, hypertension, hyperlipidaemia and diabetes), the metabolic syndrome, long-term use of medications and total energy intake. Model 3 was additionally adjusted for intakes of total protein, total fat, refined grains and added sugars. Moreover, analyses for soluble and insoluble fibres were mutually adjusted in model 3. Model 4 was additionally adjusted for total fruit intake. When analysing the association of sources and prediabetes, different fibre sources (cereal fibre, bean fibre, vegetable fibre and fruit fibre; modelled as quartiles) were also adjusted for each other in model 3. Tests for trend were carried out by assigning the median value to each category of fibre intake and modelling this value as a continuous variable.
As a secondary analysis, we examined the effect of substituting fruit fibre for cereal fibre, bean fibre or vegetable fibre by including all these fibres as continuous variables (5 g/1000 kcal per d) in the same multivariable model. The difference in the coefficients and variances from this multivariate model was used to estimate the HR and 95 % CI for the substitution effect(Reference Halton, Willett and Liu29,Reference Song and Giovannucci30) .
We examined the interactions between dietary fibre intake and age, sex, BMI, smoking status, alcohol drinking status, education, occupation, income, physical activity, the metabolic syndrome and long-term medication use by including the corresponding cross-product terms in the most adjusted models and assessing the statistical significance of the log-likelihood ratios. Furthermore, multicollinearity among covariates was evaluated using the variance inflation factor.
We ran sensitivity analyses by further adjusting for WC or adjusting for WC instead of BMI. All statistical analyses were performed using SAS version 9.4 (SAS Institute Inc.). A two-sided P < 0·05 was considered statistically significant.
Results
Table 1 shows the baseline characteristics of participants according to incident prediabetes status. Participants with incident prediabetes were more likely to be older and men, had higher BMI, WC, total cholesterol, TAG, LDL-cholesterol, blood pressure, fasting plasma glucose and lower HDL-cholesterol and tended to be more physically active. They were also more likely to have the metabolic syndrome and a family history of CVD, hypertension and diabetes. The comparison between included and excluded participants was provided in online Supplementary Table S1. Compared with those excluded, participants included in the analytical sample were younger, were more likely to be women, were more often educated and had healthier lifestyles, as well as had a better metabolic profile.
Table 1. Age- and sex-adjusted baseline characteristics of the participants by incident prediabetes status (Means and 95 % confidence intervals, n 18 085)*
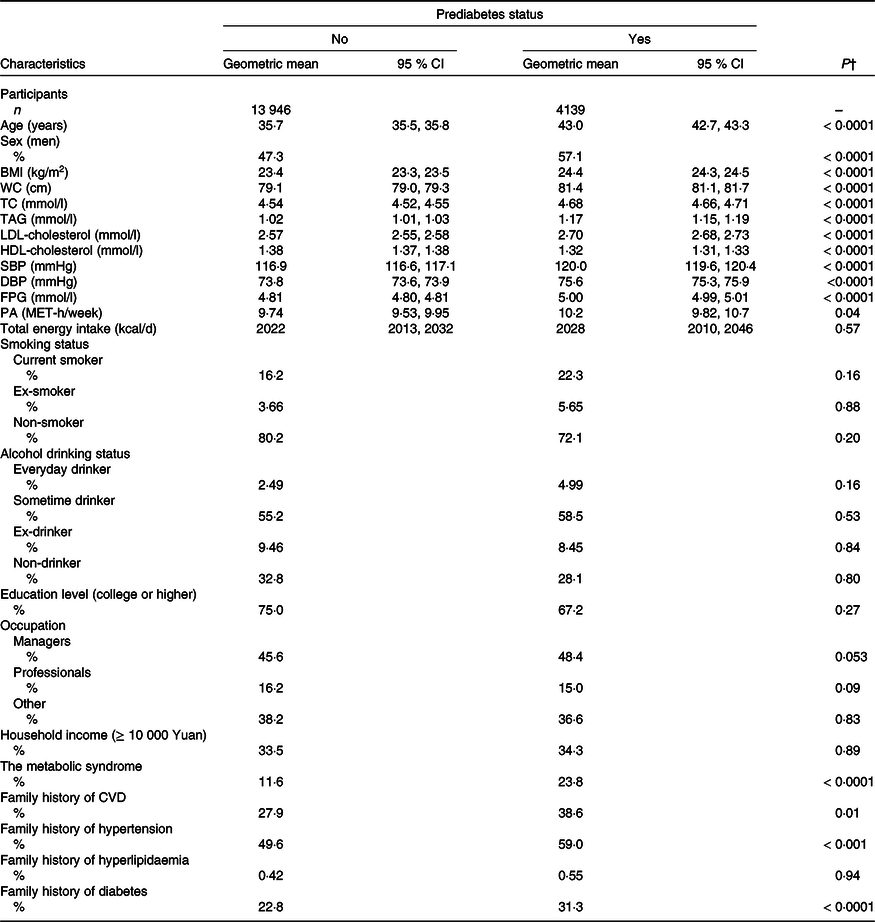
DBP, diastolic blood pressure; FPG, fasting plasma glucose; MET, metabolic equivalent; PA, physical activity; SBP, systolic blood pressure; TC, total cholesterol; WC, waist circumference.
* Continuous variables are expressed as geometric means (95 % CI) and categorical variables are expressed as percentages.
† ANOVA or logistic regression analysis adjusted for age and sex where appropriate.
Table 2 presents the association between dietary fibre intake (total, soluble and insoluble) and the risk of prediabetes. Higher intakes of total and soluble fibre, but not insoluble fibre, were significantly associated with a lower risk of prediabetes in all four of the multivariable models. In the most adjusted models, the multivariable HR of prediabetes across quartiles of total dietary fibre intake were 1·00 (reference) for 1st quartile, 0·93 (95 % CI 0·85, 1·02) for 2nd quartile, 0·91 (95 % CI 0·82, 1·01) for 3rd quartile and 0·85 (95 % CI 0·75, 0·98) for 4th quartile, respectively (P for trend = 0·02). Participants in the highest quartile of soluble fibre intake had a lower risk of prediabetes compared with those in the lowest quartile (HRQ4v.Q1 = 0·84, 95 % CI 0·74, 0·95; P for trend < 0·01). No significant association was observed between insoluble fibre intake and risk of prediabetes (HRQ4v.Q1 = 1·05, 95 % CI 0·93, 1·19; P for trend = 0·38).
Table 2. Association between dietary fibre intake and risk of prediabetes (Hazard ratios and 95 % confidence intervals, n 18 085)*
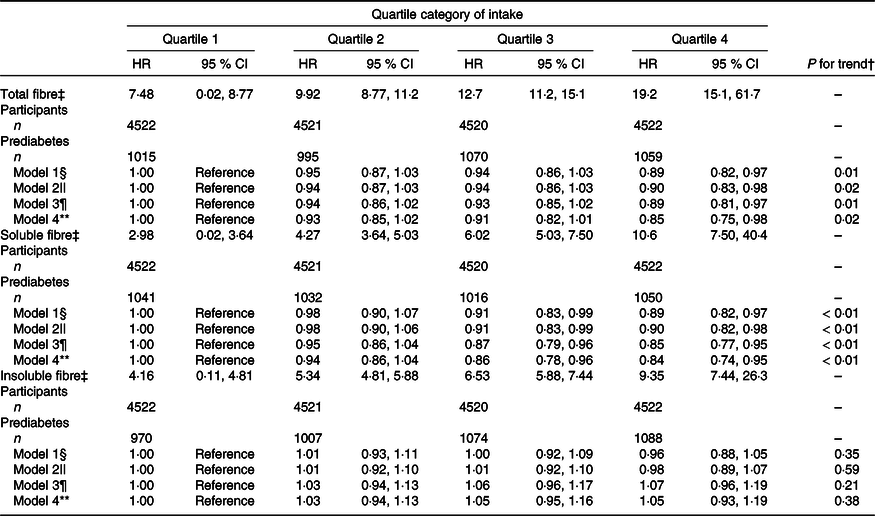
* Values are hazard ratios (95 % CI) unless otherwise indicated.
† Test for trend based on variable containing median value for each quartile.
‡ Median (range) intake, g/1000 kcal per d.
§ Model 1: adjusted for age (continuous; years), sex (categorical; men or women) and baseline BMI (continuous; kg/m2).
|| Model 2: additionally adjusted for smoking status (categorical; current smoker, ex-smoker or non-smoker), alcohol drinking status (categorical; everyday drinker, sometime drinker, ex-drinker or non-drinker), educational level (categorical: < or ≥ college graduate), occupation (categorical; managers, professionals and other), household income per month (categorical: < or ≥ 10 000 Yuan), physical activity (continuous; MET-h/week), the metabolic syndrome (yes or no), family history of disease (including CVD, hypertension, hyperlipidaemia and diabetes (each yes or no)), long-term use of medications (yes or no) and total energy intake (quartiles).
¶ Model 3: additionally adjusted for total protein intake (quartiles), total fat intake (quartiles), refined grain intake (quartiles) and added sugar intake (quartiles). For soluble and insoluble fibres, mutual adjustment was conducted in the model.
** Model 4: additionally adjusted for total fruit intake (quartiles).
The mean intake of fibre from fruit, vegetable, bean and cereal sources was 12·2, 4·87, 2·42 and 2·86 g/d, respectively. Associations between dietary fibre intake from different food sources and prediabetes are presented in Fig. 2. Among the different dietary fibre sources, only fibre from fruits was significantly associated with a lower risk of prediabetes (HRQ4v.Q1 = 0·89, 95 % CI 0·81, 0·97; P for trend < 0·01). In contrast, no significant association with the risk of prediabetes was observed between fibre from vegetables (HRQ4v.Q1 = 1·03, 95 % CI 0·93, 1·13), beans (HRQ4v.Q1 = 0·97, 95 % CI 0·88, 1·07) and cereals (HRQ4v.Q1 = 0·94, 95 % CI 0·85, 1·04).
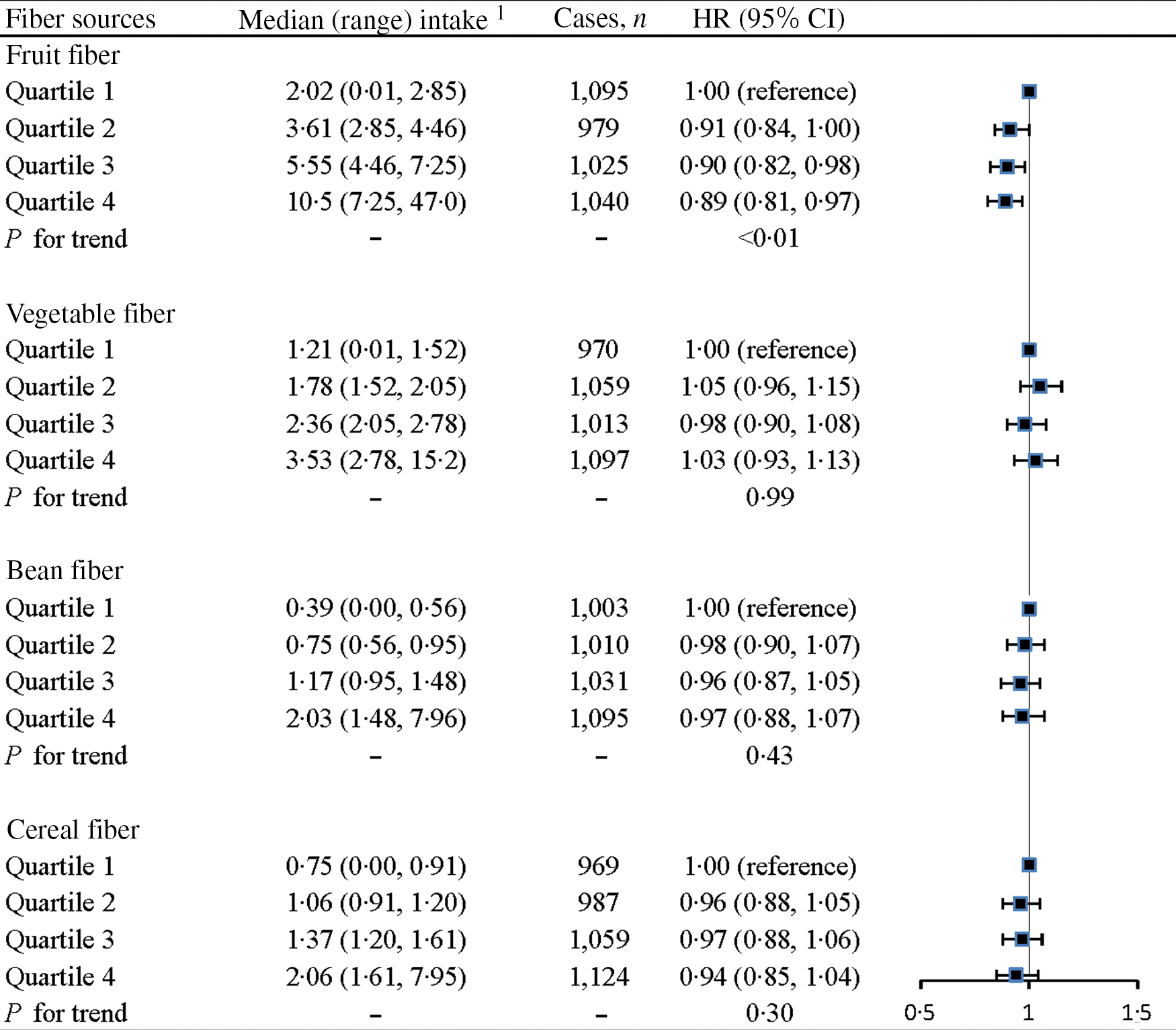
Fig. 2. Associations between dietary fibres from different food sources and the risk of prediabetes. Adjusted for age (continuous; years), sex (categorical; men or women), baseline BMI (continuous; kg/m2), smoking status (categorical; current smoker, ex-smoker or non-smoker), alcohol drinking status (categorical; everyday drinker, sometime drinker, ex-drinker or non-drinker), educational level (categorical: < or ≥ college graduate), occupation (categorical; managers, professionals and other), household income per month (categorical: < or ≥ 10 000 Yuan), physical activity (continuous; MET-h/week), the metabolic syndrome (yes or no), family history of disease (including CVD, hypertension, hyperlipidaemia and diabetes (each yes or no)), long-term use of medications (yes or no), total energy intake (quartiles), total protein intake (quartiles), total fat intake (quartiles), refined grain intake (quartiles), added sugar intake (quartiles) and intake (quartiles) of the other fibre sources (fruit fibre, vegetable fibre, bean fibre or cereal fibre). 1Presented as energy-adjusted intake amounts (g/1000 kcal per d) using the nutrient density method.
In the substitution model, the HR of substituting fruit fibre (5 g/1000 kcal per d) for 5 g/1000 kcal per d of cereal fibre, bean fibre and vegetable fibre were 0·93 (95 % CI 0·75, 0·99), 0·98 (95 % CI 0·95, 1·10) and 0·95 (95 % CI 0·86, 1·06), respectively.
No significant interactions were observed between total fibre intake and age, sex, BMI, smoking status, alcohol drinking status, education, occupation, income, physical activity, the metabolic syndrome and long-term medication use (all P for interaction > 0·10). In addition, all variance inflation factors of the covariates were less than 10, indicating that low collinearity was accepted.
When adjusting for WC + BMI or WC, the results from sensitivity analyses were comparable to those of the primary analyses (online Supplementary Table S2).
Discussion
In this study, we conducted a prospective assessment of the association between dietary fibre intake and the risk of prediabetes in the general population. Our results showed that total fibre and soluble fibre intakes were inversely associated with the risk of prediabetes. There was no observed association of insoluble fibre and prediabetes. Regarding food sources, only fibre from fruits was inversely associated with a lower risk of prediabetes.
To the best of our knowledge, this was the first prospective cohort study from China on this topic. Two previous meta-analyses of prospective cohort studies have indicated an inverse association between total dietary fibre and fruit fibre intake and T2D(Reference Yao, Fang and Xu18,Reference Wang, Fang and Gao19) . In addition, recent findings from the NutriNet-Santé study showed that total dietary fibre intake, soluble fibre intake and fibre from fruits, but not fibre from vegetables, beans and cereals, were significantly associated with a lower risk of T2D(Reference Partula, Deschasaux and Druesne-Pecollo16). These results are similar to those presented here. However, our results are in contrast to a previous meta-analysis that reported an inverse association between insoluble fibre, but not soluble fibre, and the risk of T2D(Reference Yao, Fang and Xu18). This discrepancy may be caused by differences in study populations. In the prior meta-analysis(Reference Yao, Fang and Xu18), most of the studies included were conducted with American and European populations with different dietary patterns and sources of fibre compared with Chinese population. Furthermore, results from the present study were supported by the previous evidence that soluble fibre could delay gastric emptying and slow absorption of nutrients, thereby lowering postprandial glucose responses(Reference Weickert and Pfeiffer9,Reference Lattimer and Haub15) . Indeed, a cross-sectional study conducted in 552 adult non-diabetic relatives of patients with T2D found soluble fibre intake was inversely associated with insulin resistance(Reference Ylonen, Saloranta and Kronberg-Kippila31). Soluble fibre is mainly from fruits in our study population (Spearman’s correlation coefficient = 0·79), and we found that only fibre from fruit sources was inversely associated with the risk of prediabetes. Also, the association between soluble fibre intake and prediabetes remained statistically significant after further adjustment for total fruit intake, indicating that this relation was independent of total fruit intake. This also supports the idea that fibre rather than another component of fruit is responsible for the association. Moreover, a recent study conducted in China found that dietary fruit intake, but not vegetable intake, was prospectively associated with gut microbiota β-diversity and thirty-one microbial features (belonging to Faecalibacterium prausnitzii, Akkermansia muciniphila, Ruminococcaceae, Clostridiales, Acidaminococcus, Prevotella stercorea, Prevotella copri, Fusobacterium and Enterobacteriaceae)(Reference Jiang, Sun and He32). In that study, the fruit-related gut microbiota index (created from the thirty-one identified microbial features) was positively associated with fruit intake and inversely associated with T2D risk(Reference Jiang, Sun and He32).
A recent review documented a health benefit of high dietary fibre (especially cereal fibre) intake in the prevention of diabetes(Reference McRae33). However, we found no significant inverse association between cereal fibre and prediabetes. One possible explanation for the null association is that the main source of cereal fibre in our study was refined grains, which means high consumption of grains but a very low intake of total cereal fibre. The mean intake of cereal fibre in our cohort was 2·86 g/d, lower than that of American adults (mean intake > 6 g/d)(Reference AlEssa, Cohen and Malik34,Reference Ma, Nguyen and Song35) . In addition to the amount of cereal fibre, the primary grain consumed in our cohort was different than that of American and European cohorts. In China, cereal fibre is obtained primarily from refined rice and refined wheat flour(Reference Yu, Zhao and Zhao36). A previous study has shown that a higher intake of refined grains was associated with impaired glucose homoeostasis(Reference Song, Lee and Song37). Therefore, in our study, the possible harmful effect of refined grains on prediabetes may have offset the possible protective effect of cereal fibre on prediabetes.
In the present study, no significant association between vegetable fibre intake and prediabetes was observed, which is in line with a previous meta-analysis of seventeen prospective cohort studies on T2D(Reference Yao, Fang and Xu18). Likewise, another prospective cohort study of French adults (median follow-up: 5·0 years) showed that vegetable fibre intake was not associated with the risk of T2D(Reference Partula, Deschasaux and Druesne-Pecollo16). However, a previous meta-analysis of prospective cohort studies mostly conducted in the USA found that vegetable fibre intake was inversely associated with the risk of T2D only in studies with a follow-up period of 10 years or more(Reference Wang, Fang and Gao19). Therefore, in our study, this null association between vegetable fibre intake and prediabetes could be partly attributed to a relatively short follow-up period. Moreover, the dietary fibre content varies considerably among vegetable varieties and species, and thus differences in diets between China and the USA may explain this discrepancy. In addition, our results are supported by the findings from Chinese adults showed that vegetable intake was not associated with T2D(Reference Jiang, Sun and He32).
Furthermore, we did not observe a significant association between bean fibre and prediabetes. This might be related to the insufficient intake of bean fibre in our study cohort (mean: 2·42 g/d) as well as the relatively moderate effect of bean fibre on prediabetes. A prospective cohort study in French adults, whose mean intake of bean fibre was 0·7 g/d, indicated that there is no significant association between bean fibre and T2D(Reference Partula, Deschasaux and Druesne-Pecollo16). Although bean fibre intake was relatively high in our study cohort compared with those of Western cohorts, bean consumption appears to be falling in China.
Several potential mechanisms may explain the inverse associations between soluble, bean, and fruit fibres and prediabetes. First, fermentation of soluble dietary fibre by gut microbiota could produce SCFA, thereby improving HbA1c levels partly via increased glucagon-like peptide-1 production(Reference Zhao, Zhang and Ding38). Especially, viscous soluble fibre (e.g. pectin contained in fruits) may be more quickly fermented into SCFA and is recognised for their blood glucose-lowering effects(Reference Stephen, Champ and Cloran39). Moreover, in vitro studies have shown that SCFA could stimulate insulin secretion through activating free fatty acid receptor 2 (GPR43)(Reference Pingitore, Gonzalez-Abuin and Ruz-Maldonado12). Second, soluble fibre (can form a viscous solution in the small intestine) has been shown to increase digestion time, delay gastric emptying and enhance satiety, thereby improving insulin sensitivity and postprandial glucose(Reference Lattimer and Haub15,Reference Bernstein, Titgemeier and Kirkpatrick40) . Finally, dietary fibre-induced SCFA could reduce pro-inflammatory cytokine expression by inhibiting NF-κB activation and enhancing anti-microbial peptide secretion(Reference McNabney and Henagan41), whereas inflammation plays a pivotal role in the pathogenesis of prediabetes(Reference Akash, Rehman and Chen42).
There are several strengths to this study. First, this is the first large prospective cohort study among all the populations to investigate the association of dietary fibre intake and the risk of prediabetes. Second, detailed data on potential confounders allowed us to minimise potential bias. Third, there is a paucity of evidence from China associating dietary fibre and prediabetes. Our study provides an opportunity to investigate the effects of diet on human health in China, extending our understanding of the association between dietary fibre and prediabetes in China. Nonetheless, our study has potential limitations. First, diet information was self-reported, which could result in measurement errors. However, the measurement errors are most likely to be random and might bias true associations towards the null(Reference Walter26). Further, diet information was collected once at baseline. It is likely that dietary habits might have changed during follow-up. Therefore, further studies should examine the associations between changes in dietary fibre intake and subsequent prediabetes risk. Second, although we carefully adjusted for potential confounders, residual confounding could not be completely ruled out(Reference Agoritsas, Merglen and Shah43). For example, information on trans-fat intake was not included in the study. However, there is a very low intake of trans-fat in the Chinese population(Reference Jiang, Shen and Zhao44), and our findings were less likely to be influenced by the lack of trans-fatty acid intake. Third, this is an observational study, thus the causality of the observed associations cannot be inferred. Fourth, participants included in the present study were younger and tended to be healthier than those excluded, similar to the NutriNet-Santé cohort(Reference Partula, Deschasaux and Druesne-Pecollo16) and the Whitehall II prospective cohort(Reference Barbiellini Amidei, Fayosse and Dumurgier45). While representative samples are necessary for estimating prevalence and incidence of disease, this is not the case when the study aims to examine the association between an exposure and an outcome(Reference Rothman, Gallacher and Hatch46), as shown by the association between dietary fibre intake and risk of prediabetes in the present study. Finally, our participants are exclusively Chinese, which limits the generalisability of the findings to other populations.
Conclusions
In conclusion, we found that higher intakes of total dietary fibre, soluble fibre and fibre from fruits were associated with a lower risk of prediabetes in Chinese adults. The results of this study suggest that high dietary fibre intake may protect against the development of prediabetes.
Acknowledgements
The authors gratefully acknowledge all the people that have made this study.
This study was supported by grants from the National Natural Science Foundation of China (No. 81872611 and 81941024), Tianjin Major Public Health Science and Technology Project (No. 21ZXGWSY00090), National Health Commission of China (No. SPSYYC 2020015), Food Science and Technology Foundation of Chinese Institute of Food Science and Technology (No. 2019-12), 2014 and 2016 Chinese Nutrition Society (CNS) Nutrition Research Foundation—DSM Research Fund (Nos. 2016-046, 2014-071 and 2016-023), China.
S. Z. analysed the data and wrote the paper. S. Z., G. M., Q. Z., L. L., Z. Y., H. W., Y. G., Y. W., T. Z., X. W., J. Z., S. S., X. W., M. Z., Q. J. and K. S. conducted the research. L. Q. and K. N. designed the research and had primary responsibility for the final content. All authors had full access to all the data in the study and read and approved the final manuscript.
The authors declared they have no conflicts of interest.
Supplementary material
For supplementary materials referred to in this article, please visit https://doi.org/10.1017/S0007114521003779