INTRODUCTION
External pressures force quick-paced change in global agriculture. Socio-economic trends including population growth, urbanization and dietary shifts change demand for agricultural products with consequences for rural livelihoods. Climate change and consumers’ expectations around sustainability change the context of agricultural production. Adapting agriculture to this new reality is not a one-time effort. For one thing, climates will remain unstable long after atmospheric CO2 peaks. These accelerated changes will interact in multiple dimensions and play out in different contexts in ways that are difficult to predict. A common strategy to scaling in agricultural innovation has been to focus on interventions that are expected to be beneficial to very large groups of beneficiaries. Due to variation, complexity and instability in ecological and socio-economic conditions, this strategy is unlikely to address the challenge of global change. Agriculture can only cope through a quick-paced process of constant, massive discovery of locally appropriate solutions incorporating relevant environmental and socio-economic information (options by context). It is far from clear if this increased demand for context-specific innovation can be addressed by current agricultural research and development (R&D) capacity.
One important way to accelerate agricultural R&D would be to build on collaboration with the direct users of research products, farmers. It is well-known fact that farmers are competent innovators, often trying new seeds and practises (Johnson, Reference Johnson1972; Richards, Reference Richards1985; Sumberg and Okali, Reference Sumberg and Okali1997). Client-oriented or participatory approaches have become important elements in current R&D (Witcombe et al., Reference Witcombe, Joshi, Gyawali, Musa, Johansen, Virk and Sthapit2005). But in spite of the successes of farmer-participatory approaches, these are still not fully integrated into many R&D programmes (Ceccarelli et al., Reference Ceccarelli, Guimarães and Weltzien2009). Modern information and communication technologies (ICT) have created new possibilities to engage rural households in an active way in R&D processes, whilst reducing some of the costs of communication. The human capacity to innovate can be further enhanced by connecting the innovators into an ICT-powered network. The technological connectivity needed for such social networks is now often present as mobile telephone coverage expands rapidly in rural areas around the globe.
This paper discusses a new approach to mobilize the innovative capacity of farmers on a large scale, inspired by recent developments in citizen science and crowdsourcing. Online citizen science takes advantage of the ubiquity of internet connectivity to mobilize volunteers to do different tasks (Hand, Reference Hand2010). Van Etten (Reference Van Etten2011) proposed to extend the citizen science to agricultural experimentation. This has led to the development of new approach. The first objective of this paper is to present this new approach, distinguishing between elements that have already been validated in farmer-participatory research and elements that are novel and that require further testing.
Testing of different elements was done in 2013–2015 in order to determine the feasibility of the new approach and to make further improvements. We have done a number of partial studies and pilots on the approach, which are being published elsewhere (Beza et al., Reference Beza, Steinke, Van Etten, Reidsma, Fadda, Mittra, Mathur and Kooistra2016; Steinke, Reference Steinke2015; Steinke et al., Reference Steinke, Van Etten and Mejía Zelan2016; Van Duijvendijk, Reference Van Duijvendijk2015). The second objective of this paper is to bring some of these first findings together in a single paper, to facilitate early feedback and discussion.
BACKGROUND
Crowdsourcing and citizen science concepts
The idea behind recent citizen science approaches is that large tasks can be accomplished by distributing small tasks to many volunteers, and combining the results. Activities include observations (often using smartphones), image classification, but also more complex tasks. For example of citizen science projects see zooniverse.org or citizenscience.org. This distributed way of working corresponds to a wider trend in other sectors of ‘crowdsourcing’ (a wordplay that contracts ‘outsource’ and ‘crowd’), e.g. employing many persons to do tasks using online platforms. An example is the mTurk platform (www.mturk.com), an Amazon-owned platform, which in its own words ‘gives employers and businesses access to an on-demand, scalable workforce’.
Crowdsourcing involves creating a mechanism to break a large task into ‘microtasks’, which are tasks that can be done by one individual in a short time span, and creating mechanisms to retrieve and combine the results to accomplish the original large task. Control over individual tasks is weak in crowdsourcing approaches. The massive nature of the task makes it impossible to check every single contribution. Usually, crowdsourcing approaches have built-in redundancy, so that each or some tasks are executed more than once by several participants. Results from different participants are compared and filtered for quality by checking the consistency of answers. In this context, an important idea is the ‘Wisdom of Crowds’ which explains how under certain conditions correct choices emerge from crowd behaviour (Surowiecki, Reference Surowiecki2005). From this idea, it follows that high-quality information can be retrieved and compiled from noisy individual contributions as long as the contributions are independent, decentralized and diverse, and are aggregated with a robust mechanism.
Citizen science often makes use of the crowdsourcing techniques and the ‘Wisdom of Crowds’ principle. But at the same time, many forms of citizen science give an active, engaged role to participants. The objective of citizen science often goes beyond data collection or the execution of simple tasks and also involves educational and awareness-raising objectives (Dickinson and Bonney, Reference Dickinson and Bonney2012). This matches with the empowerment agenda behind the focus on participatory approaches in agricultural science.
Approaches to farmer selection and diffusion of varieties
Even though the approach presented in this paper is applicable to a wide range of farm technologies, our experiences with the approach to date are focussed on crop improvement. This is still the main area of focus of international agricultural research, several decades after the Green Revolution. Crop varietal change remains important, including as a response to climate change (Porter et al., Reference Porter, Xie, Challinor, Cochrane, Howden, Iqbal, Lobell, Travasso, Field, Barros, Dokken, Mach, Mastrandrea, Bilir, Chatterjee, Ebi, Estrada, Genova, Girma, Kissel, Levy, MacCracken, Mastrandrea and White2014). Also, this has traditionally been an area in which much innovation has happened regarding farmer participation in research. In marginal areas characterized by large numbers of smallholders, studies demonstrate the effectiveness of farmer-participatory approaches to crop improvement, which address farmers’ needs and preferences more effectively than conventional approaches (Ceccarelli et al., Reference Ceccarelli, Guimarães and Weltzien2009).
Participatory Variety Selection (PVS) involves growing a number of crop varieties (either on-station or on-farm) and having farmers evaluate the crop varieties (for a review, see Witcombe, Reference Witcombe, Joshi, Gyawali, Musa, Johansen, Virk and Sthapit2005). A range of PVS formats exist. In certain formats of PVS, farmers come together to a common plot to observe and rate the varieties on various aspects, including vegetative characteristics and characteristics of the final product, including yield and consumption-related traits. The sessions allow for interaction between farmers and researchers. A strong reliance of farmer groups in PVS has limitations. Misiko (Reference Misiko2013) analysed data of participation of PVS trials in East Africa and found out that participation is very uneven during the growing season, due to free-riding problems. One consequence was that observations during pre-harvest stages, when most of the work needs to be done, were limited. As a result, farmers take pre-harvest traits less into account when making a choice for a variety.
To overcome some of the limitations of designs around common plots, a number of formats involve smaller trials. The Mother–Baby Trial (MBTs) is a format that is sometimes considered the ‘gold standard’ for farmer-participatory selection of varieties and other agricultural technologies. The idea was first put into practice in work on soil management (Snapp 2002). MBTs combine larger PVS trials done on-station or on-farm (mother trial), with smaller on-farm farmer-managed trials of 1–3 technologies (baby trial). In some cases, each farmer selects the technologies to test in the baby trial on the basis of their evaluation of the technologies on display in the mother trial. In other cases, scientists assign the technologies to baby plots as part of the trial design (Atlin et al., Reference Atlin, Paris, Courtois, Bellon and Reeves2002). Statistically, results from mother and babies in the MBT can be combined if measurements are done consistently. One limitation of MBTs is that they are relatively expensive to set up with many farmers. The mother trials still require tightly timed visits during the cropping cycle.
A number of more hands-off approaches to PVS exist as well. IRD (informal R&D) involves giving farmers only one crop variety each, testing a limited set of varieties. Farmers each compare a new variety to their own variety (Joshi and Witcombe, Reference Joshi and Witcombe2002). Feedback is collected sometime after the harvest about the acceptance of each new variety and the reasons for acceptance or rejection. ‘Diversity kits’, is an approach that involves the distribution of packages with experimental quantities of 2–3 varieties directly to farmers, who test these varieties under their own conditions. This has been highly effective in diffusing varieties, but does not involve a feedback mechanism. Diversity kits in Nepal led to an adoption rate of 37% of selected rice varieties (Joshi et al., Reference Joshi, Subedi, Rana, Kadayat and Sthapit1997). Both IRD and diversity kits are highly cost-effective solutions compared to approaches that involve mother trials, farmer groups and farm visits throughout the crop cycle.
Ethnobiological approaches to crop diversity knowledge
Ethnobiological approaches propose causal models of farmers’ knowledge of crop diversity, as well as quantitative methods to analyse this knowledge. Boster (Reference Boster1986) explains variations in farmers’ individual categorization of cassava varieties as a result of individual competence, which depends on experience and social connections that underpin knowledge exchange and learning. Boster (Reference Boster1986) uses quantitative methods developed in ethnobiology to analyse the large individual differences in farmers’ knowledge (Romney et al., Reference Romney, Weller and Batchelder1986, cf. Van Etten Reference Van Etten2006). Also, Boster (Reference Boster1985) shows that categorization of cassava varieties depends mainly on the visual distinctiveness of different varieties. Likewise, Bentley (Reference Bentley1989) explains differences in farmers’ competence across different domains relevant to crop performance (i.e. plants, pests, diseases) based on the observability of biological phenomena. In contrast, Zimmerer (Reference Zimmerer1991) argues that farmers’ perception of varietal characteristics does not fully explain varietal categorization. Crop ‘use categories’, which are in turn embedded in farmers’ interactions with agricultural systems and landscapes, as well as the culinary and commercial use of crop products, also play an important role.
Environmental analysis of trials
An ‘options by context’ analysis depends on the environmental analysis of trial results. There is a growing literature on geospatial analysis around crop breeding, including refinements in the characterization of ‘target population environments’ (Hyman et al., Reference Hyman, Hodson and Jones2013). More relevant in our context is the direct use of geospatial methods in combination with empirical plant performance data in order to guide plant selection. Environmental variables can be used directly as covariates to analyse genotype by environment interactions using statistical techniques like factorial regression analysis (Voltas et al., Reference Voltas, Lopez-Corcoles and Borras2005) or redundancy analysis (van Eeuwijk, Reference Van Eeuwijk1992). Post-hoc environmental analysis of trial data has been demonstrated to produce useful results to draw conclusions about climatic stresses (Lobell et al., Reference Lobell, Banziger, Magorokosho and Vivek2011). The use of these similar techniques can be expected to increase due to the increased interest in environmental analysis in crop science (Xu, Reference Xu2016).
Designing a new approach
The new approach presented here, a further elaboration of the proposal by Van Etten (Reference Van Etten2011), aims to incorporate crowdsourcing ideas into farmer-participatory approaches. In previous work, we have referred to this approach as ‘crowdsourcing crop improvement’. We have realized we need a more neutral and specific term to distinguish it from other participatory approachesFootnote 1. We refer to it as the tricot approach, in which tricot stands for triadic comparisons of technologies. (Tricot is also a French commune and a knitting technique; neither interferes with the meaning we give it here.)
Selection of participatory methods
The tricot approach recombines validated methods in crop variety research that have been listed above, selectively incorporating features of the different approaches that make it possible to follow a crowdsourcing approach. The more hands-off approaches, IRD and diversity kits, match the ‘unsupervised’ character of crowdsourcing best. The tricot approach resembles the IRD approach in being hands-off and involving a feedback mechanism. But tricot is different from IRD in that it does not distribute one variety to each farmer but a kit of three varieties, following the diversity kits methodology. In the tricot methodology, each kit contains a combination of three varieties.
Varieties are assigned to farmers based on a trial design created by the researcher. Participants do not select the varieties to be tested (cf. Atlin et al., Reference Atlin, Paris, Courtois, Bellon and Reeves2002). Following a statistical design and having more than one variety per farmer makes it possible to gain more statistical power from the farmer trials, compared to trials of farmer-selected options. This ensures the information value of the data as limited supervision may lead to higher data losses. Randomly assigning the varieties to each farmer may help avoid cognitive biases that may be present when the only comparison is the local variety (e.g. the risk of endowment bias in relation to the local variety). Also, it will be a robust approach in the case the local variety would not work well as a check; for example, when deficient physiological seed quality is a problem or when many local varieties are present.
Observations are done on-farm by individual farmers. This ensures that observations are made during the entire crop cycle (cf. Misiko, Reference Misiko2013), and follows the rationale of IRD, diversity kits and baby trials in MBT. Similar to other participatory trials, about 6–8 variables are recorded, including agronomic traits, yield, consumption value, market value and the ‘overall performance’ or whether farmers would plant this variety again, which takes together all traits.
Farmers use ranking techniques to evaluate the varieties (Coe, Reference Coe, Bellon and Reeves2002). Farmers do not score the varieties, but rank them for different aspects of performance, including agronomic traits, yield, consumption value and overall performance (taking all aspects together). The idea behind this choice is that ranking avoids any complication arising from the need to explain rating scales and precise yield measurements. This limits the need for training farmers to bring all to the same level of skills. For example, yield comparisons can be done manually in the absence of scales. The classic experiments of Ernst Weber show that most people can correctly distinguish objects that differ 6% or more in weight (Weber Reference Weber, Ross, Murray and Weber1834).
Ranking is limited to three options, e.g. the ranking reduces to triadic comparisons. Diversity kits have worked well with three varieties (Joshi et al., Reference Joshi, Subedi, Rana, Kadayat and Sthapit1997). The choice for three options was part of the original proposed approach (Van Etten, Reference Van Etten2011), justified by a reference to Martin (Reference Martin2004), who claims triadic comparisons are successfully used in ethnobiological research. This proposal, however, led to considerable discussion with collaborators implementing the approach, who suggested a local check should be included as a fourth variety. Below, we discuss the lessons learned with these variations on the general approach.
Organized group meetings take place before and after the cropping cycle, but only one or none takes place during the cropping cycle. Farmers are asked to grow the seeds individually on their own farm. This follows the IRD approach. The individual approach reduces the free-rider phenomenon identified by Misiko (Reference Misiko2013) in group-based PVS. Spontaneous farmer-to-farmer visits comparing trials are still possible in this approach. Also, the approach can be used in the context of organized farmer groups, who may organize their own meetings and mutual visits to the trial plots.
Field agents collect data, but do not do any direct field measurements. This contributes to a significant cost reduction, as staff time of field agents is an important cost driver. Also, skilled field workers are often the most limited resource of field organizations. Large cost savings were demonstrated for IRD, which similarly involves a reduction in field visits (Joshi and Witcombe, Reference Joshi and Witcombe2002).
Novel aspects of the approach
The tricot approach follows the crowdsourcing idea in that the large task of variety evaluation is divided in many microtasks. Each microtask consists in evaluating a set of three varieties. In the case of the tricot approach, the microtask consists of evaluating three crop varieties. This builds on existing PVS approaches, as explained above. To further make the tricot approach follow the crowdsourcing principles, it incorporates the following features, which are not found in the current approaches in farmer-participatory variety evaluation.
1. Variety trials are blind, e.g. farmers do not get to know the names of the varieties until they have completed the cropping cycle. In the new approach, farmers get the varieties with codes that do not reveal the identity of the varieties (1, 2, 3 or A, B and C) and make it difficult to know for certain which varieties are identical across plots. The reason for this choice was that blind trials might reduce biases induced by known varieties and increase farmers’ motivation to complete the experimental cycle in order to get to know the names of the tested varieties. The individualistic approach of tricot makes it desirable to have additional elements that stimulate farmers’ motivation.
2. Data are preferably collected through self-reporting by farmers using digital channels. Digital data collection is essential to crowdsourcing. Using digital formats and applications eliminates errors from digitalization of data collected on paper and makes it easier to enforce standardization in data collection. Digital data collection also makes it possible to speed-up data analysis.
3. Data analysis is done using the Bradley–Terry model for ranking data (Bradley and Terry, Reference Bradley and Terry1952). This model makes it possible to aggregate crowdsourced ranking data in a robust way. The Bradley–Terry model has not frequently been used in agriculture, although it has been increasingly applied by researchers of the World Agroforestry Centre. Coe (Reference Coe, Bellon and Reeves2002) discusses its application in agricultural research. Box 1 provides a short explanation of the model.
A fourth novel feature attempts to take advantage of the geographically distributed nature of tricot trials.
4. Trial data analysis makes use of complementary environmental data. Since (part of) the data are digitally collected, it is possible to geolocate the trial points or points near the plots. This makes it possible to combine the trial data with geospatial data from other sources. An important advantage is that many environments are being sampled. By measuring the characteristics of these environments and using this information fully in the statistical analysis, the tricot approach could produce important information about genotype × environment interactions.
Data are pooled and analysed directly after the trial, in combination with any other data, including characteristics of the participants and their households and environmental data. In the meeting after the cropping cycle, farmers receive information based on a statistical analysis of the data. In the tricot approach, we create individualized info sheets for each farmer. We also prepare an automatically generated report from the data as an input into the meetings that take place shortly after the cropping cycle.
Data exploration and analysis is further deepened in a last step. Whilst the automatic report serves the need to provide feedback in a time frame constrained by agricultural cycles, further visualization and analysis of the data and comparisons between different trials should lead to deeper insights. These analyses can be done in different formats, including participatory exercises with local experts, farmers, seed producers.
Box 1. Short explanation of the Bradley–Terry model
The Bradley–Terry model is one of several models developed for ranking data (Bradley and Terry, Reference Bradley and Terry1952). As use of these models is still not common in agricultural research, we provide a short explanation.
To simplify matters, suppose we have two varieties, A and B. Variety A yields more than variety B. Farmers observe this yield, compare the two varieties and decide which yields more. They observe the yield with some degree of error, so even though we know the exact yields we cannot predict with certainty what farmers will decide, we can estimate the probability that A wins. The Bradley–Terry model assumes that A and B have a Gumbel standard distribution (which resembles a normal distribution and has a fixed standard deviation).
So, if we know the yield difference between A and B and the standard deviation of the yield, we can calculate the estimated number of wins of A over B. The Bradley–Terry model inverts this procedure. If from experimental data we know that variety A wins 75 out of 100 times from B, it makes it possible to work back to the underlying data.
The Bradley–Terry model assumes that P(A > B) = eλa/(eλa + eλb) = 75/100. In this case, lambda is an index of yield. This is an equation with two unknown variables, so it cannot be resolved. The Bradley–Terry model makes the additional assumption that λA and λB sum to one or alternatively sets one of the values to zero. If we set λA to zero, we get
λA = 0,
0.75 = e0 / (e0 + eλB),
λB = −0.29.
The obtained values are relative indices called abilities. Even though abilities are relative values and may be negative, they have a linear relationship with the underlying yields (see Coe, Reference Coe, Bellon and Reeves2002). When more varieties are analysed, the Bradley–Terry model estimates the abilities from multiple comparisons between varieties using a logistic regression model and provides additional statistics, such as the standard errors and confidence intervals of the estimated abilities.
Pilot goals and methodology
The pilots were designed to put the tricot approach in practice and generate insights in the following aspects of the approach:
1. Formal parts of the tricot methodology, including the data collection formats and statistical design.
2. Capacity of the tricot approach to generate relevant and high-quality data.
3. Feasibility to collect weather data in a geographically distributed way as part of a tricot trial.
4. Reception of the approach by farmers and farmers’ motivation to participate.
5. Gender and social inclusion of the tricot approach.
Two different types of pilots were done: Crop trial pilots and a one trial specifically looking at the feasibility of collecting weather data. On some of these aspects, we have published gray literature and we are about to publish peer-review articles, which will report in more detail on these trials (these studies are cited throughout this section). The goal here is to bring together these partial evaluations in a single text to provide early feedback. The results will therefore be reported with brevity.
We did crop trial pilots of the tricot approach in India, East Africa (Kenya, Tanzania, Ethiopia), and Central America (Honduras, Guatemala, Nicaragua). In these pilots, Bioversity worked with local partner organizations with a considerable trajectory in farmer participatory research. In all cases, the focus was on annual crops that were locally important: barley and durum wheat in Ethiopia, sorghum in Kenya and Tanzania, and common bean in Central America. On the basis of previous farmer-participatory research in each area, a selection of varieties or landraces was made that were likely to be acceptable to farmers. The selected varieties were distributed to farmers using the tricot approach.
In the first iterations, data recording was initially done on paper only, as these were still small trials with a few dozens of farmers. In subsequent rounds, data collection was digitized, but still done by field agents through visits. Reports were created in an ad hoc way using a tailor-made script in R (R Core Team 2015).
The different pilots varied in the intensity of supervision of farmers and rewards offered for participation (in some cases, seed of the preferred variety was promised before the trial and given after the trial). In some trials, variations of the approach distributing four varieties were tested. Also, variations were made in statistical design and data collection formats through an iterative learning process. Lessons generated from these pilots address points 1 (methodological lessons) and 2 (data quality) above.
In order to further address point 2, we tested how accurate farmers’ observations are in the tricot approach through a more specific test in one of the pilots in Honduras. We compared farmer rankings of varieties with the rankings of experts on the same plots (see Steinke, Reference Steinke2015; Steinke et al., Reference Steinke, Van Etten and Mejía Zelan2016 for details). In this case study, farmers evaluated the following variables: plant vigour, pest resistance, disease resistance and plant architecture.
A second type of pilot focussed exclusively on point 3 above, the collection of weather data. In order to collect environmental data, we have trialled a cheap, robust weather sensor + logger called iButton (Mittra et al., Reference Mittra, van Etten and Tito2013). The cost of the iButton is ~25 US$ for an iButton with only a thermal sensor and ~60 US$ for an iButton with sensors that measure both temperature and the relative air humidity. We have included iButton sensors in our pilots of the tricot approach. We have also created a large network of these sensors on a large coffee estate in Costa Rica in order to explore its accuracy (see Van Duijvendijk, Reference Van Duijvendijk2015 for details).
For point 4 (reception/motivation), we registered farmers’ willingness to participate in further cycles as an indicator of their perception of the approach. We have also done an evaluation of farmers’ motivations across the three continents where the pilots have taken place, using a standard questionnaire (see Beza et al., submitted for details).
To address point 5 (gender), we report on women's participation in the trials and discuss ways tested to increase women's participation. We investigated if gender influenced variety preferences. Also, we did specific interviews with women's groups (see Steinke, Reference Steinke2015 for details).
The evaluation of these five aspects will feed into a discussion of the overall feasibility of the approach and elements that are still missing to develop the tricot approach into a full crowdsourced citizen science approach.
RESULTS
Methodological lessons: data collection formats
Our first versions of the data collection formats did often assume too much about the capacity of farmers to read and to follow procedures that resulted rather complex. Our initial iterations in several countries tried a variation of the approach with three varieties but explicitly comparing these three varieties with the farmer's local variety in all steps. This made the format exceedingly complex. Farmers had to put the codes of the varieties plus the local variety in order from best to worst. This often proved too complex to do. The problem was compounded by the fact that the codes on the packages were given as 1, 2 and 3. This resulted in confusion in the field. ‘Number 3 is the second-best variety’ can easily be confused with ‘number 2 is the third-best variety’. Most farmers could fill in the form only with help from the field agents. Therefore, filling in the form was frequently done after the full crop cycle was over.
In a second iteration, several modifications were introduced. Farmers now ranked only three options. Varieties were coded with the letters A, B and C, instead of numbers. Farmers answered two questions for each evaluated aspect, for example, Which variety has the highest yield? Which variety has the lowest yield? Farmers answered indicating one of the three varieties (A, B or C) for each of the two questions, with the only restriction that the best variety cannot also be the worst variety. This format proved to be much more user friendly. Also, this format is more amenable to telephone interviews (which were not part of the pilots reported here).
But in subsequent evaluations, we discovered that for participants with low literacy, the length of the written questions made the form more difficult than needed. In a third iteration, the questions were converted in ultra-short statements (‘Highest yield’, ‘Lowest yield’). We also added pictures for each of the aspects, to make the format easier to use for users with low levels of literacy. Another modification was to make visual distinctions between different steps in the observation process and the time each step should be executed in order to encourage observations throughout the crop cycle.
< tex − math/ > A sample of the final format is presented in Figure 1. Comparisons with the local variety are made in two different ways. One or more local varieties are included in the pool of varieties and distributed in the same way as the other varieties. This attempts to provide a true ceteris paribus condition, controlling the physiological quality of seed and reducing cognitive biases that favour the local variety (e.g. endowment bias). Favouring the local variety cannot always be avoided as farmers recognize their local variety if it comes in their seed package. The latest format includes pairwise comparisons between the local variety of the farmer and each of the three varieties in the package but only for ‘overall performance’. This last addition has not been evaluated yet at the time of writing.
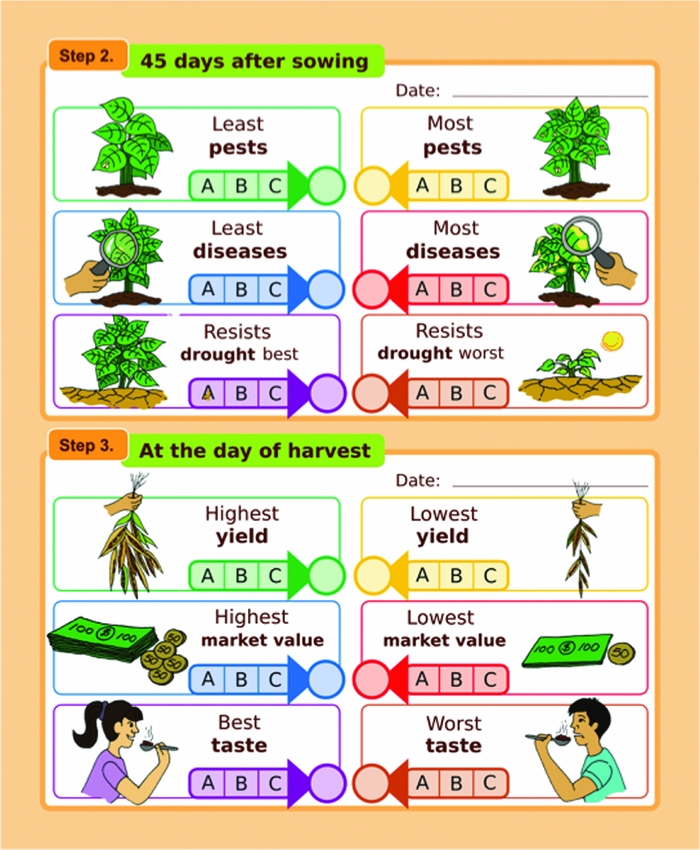
Figure 1. Excerpt from the data collection format. The format given to farmers is full colour. The idea is that farmers write the selected letter in the circle.
Methodological lessons: statistical design
The statistical design, based on simple randomization, generated several problems. Some participants dropped out when learning more about the approach or do not show up when seeds are being distributed. In some cases, we discovered that membership lists of collaborating organizations were not totally up to date and households no longer existed or had moved to another community. When incomplete blocks from an alpha design (or a suitable alternative design) were allocated randomly to participating farmers, this often led to imbalance at the level of different communities; as a result of the randomization, some varieties were present in much higher proportions and others may be rare or even absent in a single community.
To address these issues, we assign varieties through a procedure that produces a ‘sequentially balanced’ designFootnote 2. This means that if we distribute consecutively numbered seed packages to participants, the packages within each series contain a nearly balanced set of varieties. For example, if we distribute packages 1–10 in the first local community, 11–25 in the second and 26–31 in the third, the frequencies with each variety occurs will be nearly equal within each community. This gives considerable flexibility with different numbers of participants and high attrition rates during seed distribution. If farmers in the first community accept only 9 packages instead of 10, distribution in the second community can simply start with package 10. The order of the varieties is also important, as farmers may use the default order (A, B, C) to rank the varieties when in doubt. The procedure randomizes the order in which the varieties are presented, e.g. whether they are A, B or C in each setFootnote 3.
Relevance and quality of data
In all trials, the results from trials indicate statistically robust differences between varieties for a number of traits and the overall performance of the varieties, except in a few cases where only 50–70 farmers participated. Effect sizes cannot be estimated without direct measurements of underlying phenomena. Nevertheless, when results are significant, they provide useful information about the order of the varieties in terms of performance.
Figure 2 provides an example for bean variety evaluation in Nicaragua. An interesting result from this graph is the good performance of the local variety (Variedad Local) and its very small confidence interval. The local variety refers to the different local varieties that farmers grow themselves to which they compare the three introduced varieties. The small variation is remarkable because the local variety is different for each farmer. It was generally judged to be better than the introduced varieties, although three varieties can compete with it. There may be a bias in this judgement, because INTA Rojo is a commonly grown variety in this area, and was included in the trial as an additional check. By having this variety in the trial, we have two ways to assess the performance of these varieties against a check. An alternative interpretation is that farmers use different varieties for different complementary uses, which makes it difficult to indicate the best variety without indicating for what use it is best (home consumption or selling in the market). This information can only be teased out by looking at the other evaluation aspects. The worst variety was INTA Ferroso, a high-iron biofortified variety. The fact that farmers judged it as the worst variety is potentially worrying, as its diffusion will rely more on its overall performance than on the (invisible) higher iron content of the grain. This is highly relevant information in this case. In summary, these results, together with the results of the individual traits, give rich, nuanced information that may have some relation with the likelihood of adoption of each of the varieties.

Figure 2. Results for overall performance of the varieties from the ‘postrera’ season 2015 in central Nicaragua (157 participants). Bars indicate 95% confidence intervals.
When we compared the ranking of experts with the consensus ranking of Honduran farmers they invariably matched. The variation in farmers’ assessments differed across different traits, but the consensus always pointed to the correct answer. Farmer assessments of early vigour showed the highest internal agreement, whilst pest resistance assessment showed the lowest.
Another validation consists in comparing the tricot results with data collected through group-based PVS that were organized in parallel in a number pilots to reduce the number of varieties to be distributed to farmers from a larger panel, e.g. in East Africa and India. Overall, a high level of agreement was found.
What is lacking from the information gathered from participants is an indication whether certain values are acceptable or are below the threshold of acceptability. In the final workshop sessions, however, these notions become clear. In these meetings, the results are discussed with farmers and (in many cases) farmers can order extra seed and therefore indicate their final preference after considering the results from other farmers. These data provide additional insights into which varieties are acceptable to farmers.
Environmental data
It was found that it is possible to characterize the landscape with iButton sensors with an accuracy that is relevant for the trials, especially to characterize thermal stress and provide input data for phenological models (see Figure 3).

Figure 3. Interpolated mean temperature data of the Figure 3. Aquiares farm in Turrialba, Costa Rica, using universal kriging (left). The interpolation is based on 80 sensors distributed across the area (right). From Van Duijvendijk (Reference Van Duijvendijk2015).
Sensors were often stolen or displaced. When field agents had to manage the sensors they were often not correctly georeferenced. Also, data can only be retrieved from iButtons with a laptop computer, not with a mobile or tablet. This precludes (near) real-time use of the data.
Reception and motivation of participating farmers
In India, previous PVS trials had raised the expectations of farmers as some of the introduced varieties were very well received, so farmers were very eager to participate. In some communities, where seeds arrived late, farmers did their best to find a spot for the small trial, diminishing the representativeness of environments, but clearly showing the eagerness of farmers to participate and the flexibility that the small size of the trial gives. In Honduras, many farmers had previous experience as members of local agricultural investigation committees (CIAL), and knew some of the varieties. Farmers showed much curiosity in getting to know the names of the varieties in the final workshop. They had intensive discussions when they had the individual information sheets, comparing results.
Across all trials, we had positive feedback on overall satisfaction with the trial from farmers, expressing their desire to participate in new rounds of trials. In November 2014, 96% of participating farmers in India stated wishing to continue participation in subsequent research cycles, and this share was 100% of participants in the Ethiopian and Honduran pilots.
The main finding of our study is that farmers have multiple and diverse motivations to participate in the trials, including learning, social interaction and to some extent complying with expectations from field agents. One important motivation is to have contact with the field agents in order to receive crop production information and training.
Gender inclusion aspects
Through our interviews in Honduras, found that women are empowered by participation in agricultural research, especially by gaining agronomic knowledge. The tricot approach is a very low-threshold way of participating in agricultural research, so the approach could provide a stepping-stone to other forms of participation in participatory research and related activities. In Honduras, where the CIAL methodology has a long tradition and has had an explicit gender focus, women's participation was 42% of all participants. Interestingly, many women participants were members of CIAL groups; more men than women were recruited outside these groups.
In India, however, initial participation rates of women were very low. Women's participation was increased by actively involving organized self-help groups of women. Agriculture is highly feminized in the areas of Bihar where the initial trials took place. Men are often migrant workers and absent during parts of the year. Both in India and Honduras, working with women's groups to carry out a tricot trial together has proven the most direct way to increase women's rate of participation.
Our results remarkably show no statistically significant differences in preferences between men and women in any of the trials.
DISCUSSION
Methodological lessons
The pilots allowed a number of important methodological improvements in data collection formats and statistical design. Further testing of data collection formats is needed to evaluate if farmers use the forms more frequently to record observations and whether the formats facilitate farmers’ understanding of the process. Redesign of formats may be speeded-up by not waiting a full crop cycle to evaluate the results but by doing quick iterations with different versions.
The current statistical design should be robust in most circumstances. However, for more hypothesis-led trials, in which experimental factors are known (environments, farm characteristics), more sophisticated statistical designs could considerably increase the statistical power of tricot trials.
Relevance and quality of data
Our pilots demonstrate that it is possible to generate useful, high-quality data with our trials. This was to be expected as the tricot method relies to a large extent on validated participatory methods.
Our observations in in Honduras match the observations of Bentley (Reference Bentley1989), who found that Honduran farmers had precise knowledge about plants, knew less about insects, and least about plant pathology. Bentley attributes the different levels of knowledge to the different levels of visibility or salience of the biological phenomena; farmers have more difficulty to observe disease vectors than varietal differences, for example. Experiments with other crops and variables are needed to generalize our findings about the accuracy of farmers’ assessments. Even so, the results from Honduras clearly bear out the central tenet of the ‘Wisdom of Crowds’ principle that the average value from a sufficiently large collection of noisy but independent measurements tends to give the correct answer.
In the current approach, the questions farmers answer deal mainly with the qualities of the varieties per se, but less with the overall context in which categorization takes place (see section ‘Ethnobiological approaches to crop diversity knowledge’). What should be tested is adding an additional question about whether the varieties add something valuable to the existing portfolio of varieties of the farmer, following the focus on ‘use categories’ proposed by Zimmerer (Reference Zimmerer1991). This may provide useful additional information.
Environmental data
At this moment, the tested technology for weather data collection is not yet appropriate for use at a large scale in tricot trials. Placing the sensors on the plots where the trials take place is possible, but very unlikely to be cost-effective, given the number of sensors lost and the efforts needed to retrieve the data. A different solution is needed. Future trials could focus on a sensor or weather station that makes data available in real-time. Using the sensors for a range of applications will be important to justify their cost and create a strong motivation for farmers to maintain these sensors in the field. Also, they need to be placed in safe places. Important efforts are underway to create a range of robust, cheap sensors and weather stations that could be tested in the future.
Another solution is to integrate existing data into the analysis process. For example, dataset with much potential is CHIRPS, the Climate Hazards Group InfraRed Precipitation with Station data (Funk et al., Reference Funk, Peterson, Landsfeld, Pedreros, Verdin, Rowland, Romero, Husak, Michaelsen and Verdin2014). This dataset contains gridded daily rainfall data at ~5 × 5 km resolution obtained from remote sensing products combined with weather station data. It is updated regularly and available for free.
Motivation
A limitation of the current pilots is that many farmers had been exposed to the crop varieties through PVS or had general experience in participatory research. In future studies, we will expand to communities without any experience in participating in participatory variety trials in order to evaluate their reception of the approach.
Across all pilots, access to relevant information about agriculture is an important motivation for farmers to participate. Therefore, combining the two short sessions in the tricot approach with information provision about broader issues of crop production will likely increase farmers’ motivation to participate.
In some crowdsourcing citizen science schemes in environmental research, incentives are provided in the form of different types of non-material incentives to recognize efforts, learning from game design to increase intrinsic motivation to participate. Some incentives involve social recognition or accessing certain roles and rights in the crowdsourcing scheme. Having a certain differentiation of participant roles could be interesting for the tricot approach, for example to identify farmer-leaders who could recruit others, support farmers who are new to the approach or have less skills. As our results show that the motivation of farmers to participate is highly diverse, it is likely that farmers for each of these roles can be found.
Gender
The pilots show that bringing women's participation to equal levels as men's participation requires special recruitment efforts. Another issue may also be the technologies chosen for the evaluation, e.g. varieties of the main staple crops. If different technologies were to be tried that are linked more specifically to domains that women tend to manage (food processing, kitchen garden crops), it may be expected that their participation will increase. Targeting of tricot trials will need to be done in a gender-sensitive and needs-oriented way.
In other studies, gender differences in varietal preferences have been found, in contrast with our studies. One hypothesis to investigate is whether group-based methodologies, such as PVS or MBT, reveal apparent gender-based differences in preferences because they mask inter-individual variation, provoke social interactions during evaluations that generate gender differences in the levels of attentiveness for different aspects or forge consensus in the opinions about the varieties.
CONCLUSIONS
We have outlined a new approach to crowdsourcing in agricultural science, triadic comparisons of technologies (tricot), applied to PVS. Several pilots have provided feedback to improve the approach. The pilots demonstrate that it is feasible to use the tricot approach with farmers having low levels of literacy to produce high-quality data. Farmers are motivated to participate. Complementary environmental data collection is still challenging, but a number of alternative data sources are available. Gender diversity can be ensured by specific measures, whilst greater emphasis on gender-specific targeting is needed.
Even though further development of the approach is needed, in its current version, the tricot approach already offers a number of features that facilitate scaling.
Low skills requirement
Scientific researchers and professional staff of local organizations is only required during the design and training phases. Field agents with basic skill sets can execute the tasks required in the field. Trained agronomists can visit trial plots and attend meetings in a selective way, optimizing their use of time, but their availability does not limit how many farmers can participate in the trials.
Automatization and elimination of tasks
Passing data from paper to spreadsheets is no longer needed. Data cleaning requires much less time as input formats in the field restrict the options using closed questions and check the inputs before they are submitted. Info sheets and reports are created automatically based on the digital data.
Cost reduction
Staff time is a major driver of cost. Materials needed and even the seeds that are handed out to farmers constitute only a fraction of the cost and the unit costs tend to drop as more farmers join the trials.
Next steps
A number of features are still lacking to realize the full potential of crowdsourcing in the tricot approach.
User-friendly software is needed to support the implementation of the tricot approach to be able to easily integrate the approach into plant breeding programmes without substantial training and additional funding for extra staff time. On the basis of our experiences to date, we are creating an online platform with digital tools to support the tricot approach. This platform, ClimMob, will be publicly launched in 2016. The platform and instructional materials can be accessed at www.climmob.net.
Even more intensive use of digital tools can make the approach even more attractive. We are currently investigating the use of Interactive Voice Response systems to achieve this, facilitated by the ease of giving feedback using the keypad of mobile telephones (Press 1 if you think variety A is the best). Where mobile coverage is problematic, field visits remain necessary but data entry is done using tablets or smartphone.
When going to even more ‘hands-off’ crowdsourcing approaches with even less formal supervision (e.g. providing the tricot seed packages through agro-input dealers and collecting feedback through automatic calls), there will be a trade-off with data quality. Ethnobiological research shows high variation in farmers’ individual competence in crop diversity categorization (see section ‘Ethnobiological approaches to crop diversity knowledge’ above). Crowdsourcing techniques anticipate deficient data quality by filtering out contributions from participants that consistently give low-quality answers (e.g. with consistently low consensus with other participants). Filtering is easily done for crowdsourcing tasks that are executed many times in a very short time, but more difficult for tasks that are less repetitive. A number of questions that are easily verifiable could be added to provide similar checks in the tricot approach to filter out low-quality answers.
The potential of gamification, an approach used in other citizen science applications, could be further explored. Gamification is the application of game design elements to non-game situations. Gamification can bring higher levels of engagement, but can also lead to unintended behaviours. Some authors have emphasized that a degree of autonomy is an important ingredient of enjoyment, so adding an extra layer of rules on top of the experience is likely to be detrimental (Deterding, Reference Deterding2015). Next to nothing is known about the effects of gamification in the context of farmer-participatory research and more research is needed to investigate its potential.
The tricot approach should be applied to farm technologies beyond crop varieties, to products such as fertilizers, biofertilizers, soil amendments and crop protection products or in post-harvest management, consumption and nutrition. We have indicated above that from a gender perspective, better targeting is necessary. The tricot approach could be complemented with targeting exercises.
Careful evaluation of projects using the tricot approach is needed to evaluate if it can increase the human capacity to exchange knowledge and exploit environmental variation in the landscape for climate adaptation. For example, Mwongera (Reference Mwongera, Boyard-Micheau, Baron and Leclerc2014) shows that differences in social exchange of knowledge and seeds explain differences in the climate adaptation lag of different farming communities on Mount Kenya. It would be anticipated that the tricot approach stimulates learning through direct observation as well as exchange of empirical information. The tricot approach needs to be evaluated from the lens of the recent critical literature on participation and technological citizenship (cf. Cooke and Kothari, Reference Cooke and Kothari2001; Richards, Reference Richards2007). This literature criticizes instrumental approaches to participation that may stifle rather than stimulate emancipation. We hypothesize that the methodological individualism of the tricot approach can provide interesting alternative social spaces with different power dynamics: a meritocracy of local experts.
Sustainable institutional and business models are needed to support the tricot approach. The tricot approach creates valuable information that is useful to both farmers and seed and input suppliers. This could be seen as a so-called two-sided market (Eisenmann et al., Reference Eisenmann, Parker and Van Alstyne2006). More research is needed to develop the tricot approach into a financially sustainable platform that serves the two-sided market for agricultural technology targeting. A mix of public and private funding could maintain such a platform.
Tricot is a promising approach and its benefits are already evident. It is clear that the approach deserves more research effort to reach its full potential.
Acknowledgements
This article is a contribution to the CGIAR Research Program on Climate Change, Agriculture, and Food Security, which is a partnership of CGIAR and Future Earth. Further funding was provided by USAID Development Innovation Ventures. The views expressed in this document cannot be taken to reflect the official opinions of CGIAR, Future Earth or USAID.
The authors are grateful to the large number of farmers and field technicians who have executed tricot experiments over the last few years.