10.1 Introduction
The “sharing economy” has been lauded for decentralizing commercial transactions, allowing individuals to directly transfer products and services. Less often discussed is how it has precipitated a parallel geographic decentralization. Whereas historically the industries being disrupted by these platforms have been concentrated in downtown centers and business districts, the same transactions are now spreading throughout cities and towns. Uber and Lyft drivers make many more pick-ups and drop-offs in residential areas than traditional taxis(Lam & Liu, Reference Lam and Liu2017). Airbnb listings offer short-term lodging in residential neighborhoods that have never had hotels. When this is discussed, it is often done in terms of supply and demand. First, some describe it as a boon to both the seller and the buyer as it expands the opportunity for each to deliver and receive services in locations that are more convenient to them. Second, there is sometimes concern, especially in the case of Airbnb, that these new transactions are removing products (for example, housing) from existing markets, shifting their balance. This market-oriented perspective, however, fails to engage the more fundamental implication of sharing economy platforms for neighborhoods: By shifting the geographic distribution of commercial transactions, they have the potential to alter who is in a neighborhood at a given time and what they do – whether residents or visitors – while they are there. This could lead to pervasive impacts to the social and behavioral dynamics, going beyond questions of supply and demand.
Here we present a generalized theory of how sharing economy platforms can and do impact neighborhoods. Our rhetorical focus is on urban neighborhoods, and we draw heavily from literature on cities, but the argument is relevant to suburban and even rural areas as well. After articulating this model more fully, we apply it to Airbnb, treating its incursion into the neighborhoods of Boston, MA as a test case. Along the way, we discuss some of the conceptual challenges that must be taken into account in such analyses.
10.2 A Framework for How Sharing Economy Platforms Can Impact Neighborhoods
The sharing economy has enabled private citizens to directly exchange services and products. This “peer- to-peer” construction has also changed the places where such transactions are likely or even possible. We propose a three-step framework for how these shifts in the commercial landscape of the city can affect the social and behavioral dynamics of neighborhoods (see Figure 10.1). Throughout, we use Airbnb and ride-sharing services (Uber and Lyft) as illustrative examples as they are the most common sharing economy platforms. That said, we go deeper into the implications of the model for Airbnb in the next section.
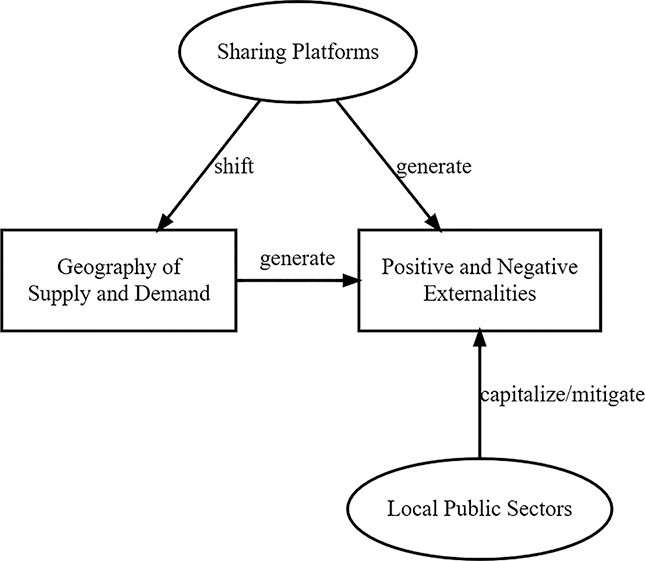
Figure 10.1 Three-step model of the sharing economy.
First, the sharing economy alters the traditional geographic distribution of service supply. Many services have historically been concentrated in business districts because of the incentives of agglomeration and access to consumers and the relatively high cost of serving areas with low expected demand. Sharing economy platforms have disrupted these mechanisms by lowering the transaction cost of offering services, thus enabling producers and consumers to find each other in less densely populated areas. This can be seen in both Airbnb and ride-hailing services. In terms of the former, researchers examining New York City, Boston, San Francisco, and Barcelona have found that Airbnb listings are common in neighborhoods that did not previously have hotels (Benítez-Aurioles, Reference Benítez-Aurioles2017; Horn & Merante, Reference Horn and Merante2017; Koster, van Ommeren, & Volkhausen, Reference Koster, van Ommeren and Volkhausen2021). Likewise, much has been written about how ride-sharing has increased access to taxis in residential neighborhoods, especially in New York City, where taxis notoriously concentrate in Manhattan and rarely venture out to the other four boroughs (Correa Diego, 2017).
Second, these geographic shifts in supply and demand can generate unexpected positive and negative externalities for communities. Oftentimes the impacts of the sharing economy are articulated in terms of city- or region-wide industry patterns, like drops in overall hotel revenue (Dogru, Mody, & Suess, Reference Dogru, Mody and Suess2017; Zervas, Proserpio, & Byers, Reference Zervas, Proserpio and Byers2017). We are concerned here with more local impacts at the neighborhood level as the introduction of services brings in people and activities that were not there previously. These impacts might be economic in nature, but they also could arise from the social interplay between these visitors, the services themselves, and the local community. Such effects might be positive. For example, a sharing economy platform can stimulate economic development by increasing access to flexible jobs and foot traffic by outsiders who will frequent local establishments. For instance, one study in New York City found that neighborhoods with more Airbnb listings saw an increase in business, as reflected by expanded staff hiring (Alyakoob & Rahman, Reference Alyakoob and Rahman2019). On the other hand, the increased activity might bring nuisances, like unpleasant guests or burdensome traffic, or, as has been seen, lower the supply and in turn raise prices for rental units in the community. We find the example of Airbnb leading to additional crime intriguing, in fact, as it might implicate either of two mechanisms instigated by a density of short-term rentals. As tourists begin to spend more time in the neighborhood, they might commit crimes, create disorder, or be targets for those who might do so themselves (Biagi & Detotto, Reference Biagi and Detotto2014; Brunt, Mawby, & Hambly, Reference Brunt, Mawby and Hambly2000; De Albuquerque & McElroy, Reference De Albuquerque and McElroy1999; Harper, Reference Harper2001; Ryan, Reference Ryan1993; Schiebler, Crofts, & Hollinger, Reference Schiebler, Crotts, Hollinger, Pizam and Mansfield1996; Stults & Hasbrouck, Reference Stults and Hasbrouck2015). Alternatively, the replacement of stable (or at least semi-stable) renter households with short-term rental units can create gaps in local social networks, potentially undermining a community’s natural ability to prevent crime (Kawachi, Kennedy, & Wilkinson, Reference Kawachi, Kennedy and Wilkinson1999; Sampson, Reference Sampson2012; Sampson & Groves, Reference Sampson and Groves1989).
Third, local governments are tasked with designing policies and regulations that capitalize on the positive externalities of the sharing economy while minimizing the negative externalities. This third component of the model is a response to the first two factors we have described, they also will create a feedback loop, reshaping the distribution and consequences of both the sharing economy activity and its consequences. For instance, regulatory and legislative approaches to Airbnb and ridesharing have often focused on specific (and, at times, anecdotal) concerns about the impacts of these platforms on neighborhoods. In the case of New York City’s negotiations with Uber and Lyft, the emphasis was primarily on increased traffic. For Airbnb in Boston, it was gentrification and displacement of the incumbent community. It is not to say that these considerations were incorrect, but that a more extensive science of the kinds of externalities we suggest here will be critical to developing fully informed policy. In the work we present here, our goal is not necessarily to critique current policy strategies for regulating the sharing economy, but to recommend a scientifically informed process for designing such regulations. That said, it is likely that in many cases the latter will give rise to the former.
Our three-part framework lays the groundwork for various research questions and practical implications for the sharing economy. In terms of the first component of the model, to what extent do sharing economy platforms shift the geographic distribution of a given service? Which neighborhoods see the greatest change? Are these changes correlated with demographic factors? As we progress to the second part of the model, what are the externalities arising from the geographic shifts resulting from a given sharing economy platform? To what extent are they consequential? Do they affect certain communities more than others? Critically, answers to these questions make it possible for policymakers to take action at the third stage of the model. They will be better equipped to determine which policy tools are most effective in managing the externalities surrounding the sharing economy. How do we design them to best effect and which neighborhoods most need attention? We thus encourage researchers and policymakers to partner under the perspective that these three components – the geography of supply and demand, localized social and behavioral dynamics, and public policy – are deeply intertwined in determining how the sharing economy contributes to the ongoing evolution of neighborhoods. With luck, such partnerships will provide the depth of knowledge necessary to design and advance “next-generation” regulatory approaches that move past traditional policy tools.
10.3 Airbnb and Urban Neighborhoods
Airbnb and other peer-to-peer rental platforms make an ideal test case for our framework on how sharing economy platforms can impact neighborhoods. Per the first component of our model, travelers are no longer limited to districts with hotels, which are often concentrated around downtown neighborhoods with commercial and industrial zoning. Instead, they are now able to stay in apartments, houses, and condominiums in residential neighborhoods. This newly introduced commercial activity and the visitors it brings with it can have a variety of externalities, as stated in component two of our model. These can be both positive and negative, thereby impacting the character and trajectory of a neighborhood. An understanding of these externalities should in turn influence the regulation of short-term rentals, which have been the subject of much public discourse and policy debates in cities around the world. This is the culmination and third piece of our model.
To date, most research on the impact of Airbnb rentals on communities has centered on two main outcomes, each related to patterns of supply and demand – the revenue of the hotel industry and the price of housing. The former line of inquiry has found that Airbnb draws potential customers away from hotels and thus lowers their revenue, especially for middle- and low-end hotels (Dogru et al., Reference Dogru, Mody and Suess2017; Zervas et al., Reference Zervas, Proserpio and Byers2017). This work, however, has been more about the way that Airbnb disrupts the hotel industry in a city or broader region, and not about how Airbnb impacts neighborhoods. Meanwhile, research on the relationship between Airbnb and housing values works off the assumption that many Airbnb listings are for units that would otherwise be owner-occupied or rental properties. By lowering this supply, Airbnb in turn leads to increases in housing value and rental prices, a result that has been found in multiple cities in North America and Europe (Ayouba et al., Reference Ayouba, Breuillé, Grivault and Le Gallo2019; Barron, Kung, & Proserpio, Reference Barron, Kung and Proserpio2018; Horn & Merante, Reference Horn and Merante2017; Garcia-López et al., Reference Garcia-López, Jofre-Monseny, Martínez-Mazza and Segú2020; Sheppard & Udell, Reference Sheppard and Udell2016).
Less attention has been paid, however, to the ways that the prevalence of Airbnb in a neighborhood might impact other aspects of a neighborhood. This is important scientifically and practically, as it allows us to better understand how short-term rentals interact with local social dynamics and can provide guidance for policies and regulations that balance their costs and benefits for local residents and businesses. In order to enumerate these possibilities, we must first consider what it means to have tourists in a residential neighborhood. First, tourists tend to frequent local establishments, especially for food and drink, which could increase economic activity in “main street” districts that serve local communities. For example, Alyakoob & Rahman (Reference Alyakoob and Rahman2019) found that New York City neighborhoods with a greater presence of Airbnb listings experienced more growth in restaurant employment. They attributed this effect to visitors using Yelp reviews data. Likewise, Schild (Reference Schild2019) identified a positive impact of Airbnb usage on the number of firms in the entertainment sector.
Although they might contribute to the local economy, Airbnb users might not have an exclusively positive effect on neighborhoods. Tourists can potentially be disruptive by keeping different hours than locals and possibly partaking more avidly in alcohol or other disruptive activities. They also are more vulnerable to property crime than locals as they lack protective relationships and have money and valuables with them. Criminologists have regularly found that neighborhoods with more tourists tend to have greater crime (Boakye, Reference Boakye2010; Harper, Reference Harper2001; Schiebler et al., Reference Schiebler, Crotts, Hollinger, Pizam and Mansfield1996). No one has yet directly tested, however, whether the presence of Airbnb in a neighborhood leads to rises in crime and disorder via this mechanism.
Additionally, as we stated earlier, there is also the possibility that the tourists themselves are not responsible for rises in crime, but that the diminished number of stable households in the neighborhood interrupts the function of local social networks, which are known to be crucial in the management of crime and disorder (Sampson & Groves, Reference Sampson and Groves1989). To illustrate, if a sufficient number of units throughout a community have been converted to short-term rentals – the most transient form of occupancy possible – fewer neighbors are present to sustain a strong social network, also referred to as the social organization (Sampson, Reference Sampson2012), and establish the “social capital,” including trust, reciprocity, and social cooperation, known to be crucial to communities (Coleman, Reference Coleman1988). Regardless of the specific terminologies used, researchers have found these processes to be active in diminishing crime (Kennedy et al., Reference Kennedy, Kawachi, Prothrow-Stith, Lochner and Gupta1998; Sampson, Reference Sampson2012). There is also evidence in the network science literature that demonstrates the positive effect of enhancing the community structure of social networks on their collective prosocial behavior. The strength of the community structure in network science is often measured by network modularity, which measures the ratio of intensity of ties within and across a set of nodes that are identified as a community. Emergence of prosocial norms can then be studied by allowing nodes on a network to play some strategic games with their network neighbors and update their behaviors by occasionally mimicking their successful neighbors. The final status of the collective behavior is then related to the structural features of the network, such as modularity, degree distribution, and other standard network measures. The games are chosen in such a way to model various social dilemmas (for example, prisoner’s dilemma or ultimatum game), in which players can either act selfishly for short-term gains, or take prosocial actions that have long-term benefits for them and the network as a whole. Several studies using this framework have demonstrated that how players resolve such dilemmas depends on the structure of the social network and have shown a strong impact of network modularity – that measures the intensity of community structure based on ratio of link density within and across communities – on population-level attributes such as cooperation, fairness, and stability (Gianetto & Heydari, Reference Gianetto and Heydari2015, Reference Gianetto and Heydari2016; Heydari, Heydari, & Mosleh, Reference Heydari, Heydari and Mosleh2019; Mosleh & Heydari, Reference Mosleh and Heydari2017).
A corollary question is how the presence of Airbnb and tourists might impact the behaviors of local property owners. As listings increase in a neighborhood, they act as a tangible representation of the attractiveness and marketability of housing there. This might then inspire property owners to invest in their buildings, potentially accelerating increases in property values, rental prices, and more generalized gentrification.
Here we leverage our three-component model of sharing economy and neighborhoods to examine outcomes in Boston, MA, which has been the site for early work on how Airbnb impacts property value. It has also been one of the cities that has led the way in developing policy interventions that seek to limit the negative impacts of Airbnb on neighborhoods. Specifically, we analyze how the longitudinal growth of Airbnb across the neighborhoods of Boston, MA is associated with: (1) the increase in food establishments; (2) levels of crime and disorder; and (3) the tendency of property owners to invest in parcels across the city.
10.4 Airbnb’s Presence in Boston
To examine the impact of Airbnb on Boston neighborhoods, we downloaded listings and reviews from InsideAirbnb.com, an independent, noncommercial website that scrapes and publishes data sets of Airbnb listings for cities across the world. InsideAribnb.com has published these data annually since 2015, but Airbnb entered Boston in 2009. In order to overcome this limitation, we leveraged the “host since” field, which indicates the date a property became an Airbnb listing, to estimate which Airbnb listings were present in each year 2010–2014. Koster et al. (Reference Koster, van Ommeren and Volkhausen2021) took a similar approach using the date of a listing’s first review, but we found that the “host since” variable more consistently had a value and would be more precise in any case.
As shown in Figure 10.2, Airbnb had limited presence in Boston in the early years, with a negligible number of listings and reviews. There was rapid growth, however, between 2014 and 2018, over which time the number of listings more than doubled from 2,558 to 6,014. There were also nearly 80,000 total reviews by 2018. Our focus here, though, is not the city as whole, but how the increased prevalence of Airbnb was distributed across neighborhoods. We examine this by joining each listing to the containing census tract, which we use to approximate neighborhood (avg. population = 4,000; 168 with meaningful population in Boston). A major challenge, though, is determining how best to measure Airbnb’s presence in a neighborhood, particularly in terms of how we would expect that presence to have impact on local behavioral and social dynamics.

Figure 10.2 Airbnb’s expansion in Boston: 2009–2018.
Airbnb listings are a “naturally occurring” data set, meaning they were not originally constructed for research purposes. The upshot is that it is incumbent upon researchers to determine how to operationalize the measures of interest (O’Brien, Reference O’Brien2018). As such, previous studies have proposed several ways of measuring Airbnb’s presence in a community. Some have focused on the number of listings, either as the percentage of total housing units in a census tract (Wegmann & Jiao, Reference Wegmann and Jiao2017) or relative to the number of buildings, being that many buildings contain multiple apartments of condos (Koster et al., Reference Koster, van Ommeren and Volkhausen2021). Others have focused on the number of reviews, either as a pure count (Schild, Reference Schild2019) or relative to the number of households in the neighborhood (Alyakoob & Rahman, Reference Alyakoob and Rahman2019), arguing that reviews are a better reflection of the number of tourists brought to the neighborhood by the platform. There is no consensus as to whether one of these is superior to others, however, for which reason we use three measures of Airbnb presence here. First, we measured the density of Airbnb as the number of Airbnb listings in a neighborhood divided by the number of housing units (as accessed from American Community Survey 2011–2017 estimates). Second, we measured the penetration of Airbnb as the number of unique addresses with listings divided by the number of parcels (lots that contain one or more units, per the City of Boston’s Assessing Department) in the census tract, thereby approximating the share of buildings with at least one Airbnb listing. Third, we measured usage of Airbnb as the number of reviews divided by housing units in a census tract. For the sake of brevity, we use these three terms – density, penetration, and usage – throughout.
The reason for adopting three measures of Airbnb’s presence is because none of them captures the whole picture of Airbnb’s impacts on neighborhood on its own. For example, if most listings in a neighborhood are concentrated in a few condo buildings, the potential positive or negative effects generated by Airbnb-related activities might be limited to the immediately surrounding areas, leaving the rest of the community unaffected. On the other hand, if the census tract is a sprawled suburban neighborhood filled with single family houses, the same number of Airbnb listings would be spread more evenly, having a wider impact across the neighborhood. In addition, per the advice of Schild (Reference Schild2019), Airbnb reviews more directly reflect the volume of usage by tourists as a higher density or penetration of Airbnb listings is not always accompanied by more visitors. There is of course, though, the potential for substantial measurement error in this last measure as leaving reviews for hosts is not a mandatory policy of Airbnb, hence many visitors choose not to do so. Also, a party with multiple visitors can only leave one review for a listing, inevitably fewer than the people who were present. As a consequence, this measure underestimates the total volume of tourists produced by Airbnb, and analyses using it must assume that these undercounts are consistent across neighborhoods; that is, the tendency to undercount is not exaggerated in some neighborhoods more than others. Another benefit of using these three different measures is the ability to potentially differentiate between those impacts caused by the sheer number of tourists (quantified with usage), versus those created by listings (quantified with penetration and density). As we noted earlier, this is specifically relevant to our discussion of Airbnb might lead to elevated crime in a neighborhood: Tourists could attract or perpetrate crime, or the conversion of units to short-term rentals could lead to weaker ties and relationships within the community.
Our first task is to determine whether we are justified in treating each of these three measures as a separate indication of the presence of Airbnb in a neighborhood. While they are semantically and computationally distinct, it is completely possible that they are too strongly correlated to be analyzed independently. Figure 10.3 presents the correlations among the three measurements for census tracts, showing that they are substantially correlated (r = .47 – .76), but not so much to suggest that they cannot be analyzed separately. The strongest correlation is between density and usage, which is unsurprising being that they are calculated using the same denominator. Meanwhile, the weakest correlation is between penetration and usage. As one can see in the scatter plot at the bottom left corner of Figure 10.3, there are numerous neighborhoods with a high level of Airbnb usage (that is, many reviews per housing unit) but a low level of Airbnb penetration (that is, number of parcels with at least one listing); meanwhile there are others with low Airbnb usage but high penetration. Given this, we move forward analyzing all three measures.

Figure 10.3 Correlations between three measures of Airbnb’s presence in Boston.
In Figure 10.4 we can see that Airbnb density, penetration, and usage all increased over time and across census tracts in Boston. That is not to say that this growth was uniform. Certain census tracts were the first to have a measurable presence of Airbnb and then proceeded to have high levels of Airbnb by all three measures. The highest were tracts with as many as 8 % of units and 40 % of buildings featuring at least one listing. Even more striking, our measure of Airbnb usage is as high as 1.5 reviews per housing unit in the tract. In contrast, in many other tracts the presence of Airbnb was limited and even absent throughout the study period. Meanwhile a handful of tracts started with very low Airbnb presence and then witnessed rapid growth of Airbnb-related activities.

Figure 10.4 Airbnb’s presence in Boston.
Note: Each row represents a census tract from 2010 to 2018. The darker the color, the higher the Airbnb presence. Tracts are in the same position in each panel, meaning we can compare panels to confirm that most tracts with high level of presence on one measure scored similarly on the other measures.
Before proceeding, it is worth noting which neighborhoods have high and low presence of Airbnb. Figures 10.5–10.7 map the spatial distributions of the three measures over time, demonstrating that the measures indeed tell different stories about Airbnb’s expansion in Boston. In Figure 10.5, we notice that as the number of listings increases, Airbnb penetrations were most significant in census tracts that are located in the downtown urban center (in the northeast of the city). However, in Figure 10.6, although census tracts in urban centers still show relatively high Airbnb presence measured by Airbnb density, the tracts with highest level of Airbnb density are scattered surrounding the downtown areas. This divergence can be explained by population density in different urban neighborhoods. Urban centers usually have more people and less land while surrounding neighborhoods are somewhat less dense. Hence, Airbnb penetration might be high in an urban center, but its density is dwarfed by the immense number of housing units there. Owing to their strong correlation, Airbnb usage displayed a similar pattern to density (see Figure 10.7). Whereas the downtown and the first ring of neighborhoods around it share the distinction of highest presence of Airbnb depending on the measure used, neighborhoods located further out are consistently low in all three measurements. In the next section, we estimate Airbnb’s impacts on neighborhood’s investment and quality of life using the three measurements.

Figure 10.5 Airbnb penetration.

Figure 10.6 Airbnb density.

Figure 10.7 Airbnb usage.
10.5 Evaluating Airbnb’s Impacts on Boston Neighborhood
10.5.1 Methods
To estimate Airbnb’s impacts on Boston neighborhoods, we return to the three hypotheses from above: (1) Airbnb might encourage property owners to invest in their buildings; (2) the presence of tourists will support expansion of the local restaurant industry; and (3) the increased prevalence of tourists will lead to elevated disorder and crime. In order to test each of these hypotheses, we draw from indicators collected or developed by the Boston Area Research Initiative (BARI). First, BARI releases measures of investment and growth annually based on building permits approved by the City of Boston (O’Brien & Montgomery, Reference O’Brien and Montgomery2015; O’Brien et al., Reference O’Brien, Montgomery, de Benedictis-Kessner and Sheini2019). The most relevant such indicator is alteration to existing buildings, calculated as the percentage of parcels in a census tract undergoing an addition or renovation in a year (excluding newly constructed buildings).Footnote 1 Second, we calculated the number of new food establishments from the records of new food establishment licenses approved by the Health Division of the Department of Inspectional Services (ISD).Footnote 2 Third, we obtained three indicators of social disorder and crime based on 911 dispatches (Ciomek & O’Brien, Reference Ciomek and O’Brien2019): Public social disorder, such as panhandlers, drunks, and loud disturbances; private conflict arising from personal relationships (for example, domestic violence); and public violence that did not involve a gun (for example, fight). Each of these measures was based on a combination of case types in the 911 system, divided by the population of the census tract (to form a rate). We also access demographic measures from the American Community Survey as control variables, particularly measures of socioeconomic status (for example, income, poverty rate), as they are closely related to many of the variables here. Table 10.1 provides a summary of the variables.
Table 10.1 Description of variables
mean | sd | min | max | Observations | |
---|---|---|---|---|---|
Permits for Property Alteration (%) | 0.19 | 0.09 | 0.04 | 0.61 | 1,176 |
Number of Licenses for Food Establishment | 1.03 | 2.32 | 0.00 | 24.00 | 1,344 |
Airbnb Density (%) | 0.01 | 0.01 | 0.00 | 0.08 | 1,344 |
Airbnb Penetration (%) | 0.04 | 0.06 | 0.00 | 0.41 | 1,344 |
Airbnb Usage (%) | 0.12 | 0.20 | 0.00 | 1.44 | 1,344 |
Events of Private Conflict (per 1,000) | 11.13 | 6.18 | 0.00 | 32.97 | 1,344 |
Events of Guns (per 1,000) | 4.21 | 4.52 | 0.00 | 25.79 | 1,344 |
Events of Violence (per 1,000) | 28.10 | 21.84 | 1.33 | 172.54 | 1,344 |
Events of Social Disorder (per 1,000) | 7.51 | 8.67 | 0.00 | 75.82 | 1,344 |
Each of the variables was measured annually throughout the study period, making for a panel design. Thus, we analyze the impacts of Airbnb on a neighborhood using a set of generalized difference-in-difference (DID) fixed-effects models with multiple periods and continuous treatments (Lechner, Reference Lechner2011):

where i represents the census tract, t represents the year. Yi,t are the dependent variables: alteration to existing structures, growth in food establishment licenses, and indicators of social disorder and crime. g is the estimated causal effect of Airbnb presence.Footnote 3 The key independent variables are lagged for one year to avoid reverse causal effects. h and b are the tract and year fixed effects, respectively, capturing both time-invariant characteristics of tracts and spatially invariant characteristics of years (for example, a city-wide increase in Airbnb prevalence or property investment). Ci,t is the vector of control variables including log of median home values, median household income, and population density, among others.Footnote 4 Note that we run the models three times for each outcome variable, independently testing the impact of each aspect of Airbnb prevalence.
10.5.2 Results
The results from the DID models supported one of our three hypotheses of how Airbnb can impact neighborhoods. As shown in Table 10.2, increases in the prevalence of Airbnb predicted increased property investment in the following year. This was true whether we measured Airbnb in terms of density (b = 0.004, p < 0.01), penetration (b = 0.001, p < 0.001), or usage (b = 0.000, p < 0.01). The coefficients indicate that a one percentage-point increase in Airbnb density was associated with a 0.4 percent increase in property alteration and that a 10 percentage-point increase in Airbnb penetration was associated with 1 percent increase in building permits qualified as housing investments. This effect seems practically small but presents a reasonable ratio of 10:1 between properties listing on Airbnb and properties pulling building permits for improvements. It is worth noting that these are not necessarily the same properties, but it would seem reasonable to assume there is at least some overlap. Further, a 1 percent increase in property alteration is nontrivial, considering that the average census tract has just below 10 percent of properties apply for permits in a given year. In summary, Table 10.2 provides evidence that the prevalence of an Airbnb market in a neighborhood contributes to a greater percent of property investment. This joins existing evidence that Airbnb presence leads to higher housing property value, but we found no evidence to suggest that residential property investment is the intermediate factor through which Airbnb density affects housing values.
Table 10.2 Fixed-effects models on property alterations
(1) | (2) | (3) | |
---|---|---|---|
Airbnb Density (%) | 0.386∗∗ | ||
Airbnb Penetration (%) | (0.162) | 0.108∗∗∗ | |
Airbnb Usage (%) | (0.039) | 0.017∗∗ | |
(0.008) | |||
Tract FE | Yes | Yes | Yes |
Year FE | Yes | Yes | Yes |
Controls | Yes | Yes | Yes |
Observations | 1,126 | 1,126 | 1,126 |
F | 6.742 | 6.417 | 6.841 |
R2 | 0.940 | 0.941 | 0.940 |
Note: clustered standard errors are displayed in parenthesis. All independent variables are lagged for one year. Control variables include median home value (log) and events of violence, social disorder, guns, and private conflicts
Significance levels: * p<0.00; ** p<0.01; *** p<0.001.
Table 10.3 shows no clear relationship between Airbnb prevalence in Boston neighborhoods and the expansion of food establishment licenses, regardless of which measure of Airbnb prevalence we used. Table 10.4, however, shows that neighborhoods with a higher level of Airbnb penetration saw rises in violent crime in the following year (b = 0.546, p < 0.001), and notably to a greater extent than the concurrent measure of penetration. There was still no corresponding effect on public social disorder or private conflict, however. Airbnb density in the previous year was also associated with higher levels of violent crime, albeit at a lower significance, and thus magnitude, relative to penetration (b = 1.407, p < 0.05). Airbnb usage had no effect on any of the three measures in the following year. We also performed a two-year lagged analysis, and the results are in general agreement with those with one-year lag in terms of the impact of Airbnb penetration on events of violence. There, Airbnb penetration not only predicted increased violence at this time scale, but also showed a moderate impact on events of private conflict, an effect that was not present in the one-year lagged analysis. The effects of Airbnb usage and density also concurred with the one-year lagged analysis (Ke et al., Reference Ke, O’Brien and Heydari2021).
Table 10.3 Fixed-effects Poisson regressions on new food establishment licenses
(1) | (2) | (3) | |
---|---|---|---|
Airbnb Density (%) | –0.040 | ||
(0.047) | |||
Airbnb Penetration (%) | –0.012 | ||
(0.008) | |||
Airbnb Usage (%) | –0.003 | ||
(0.003) | |||
Tract FE | Yes | Yes | Yes |
Year FE | Yes | Yes | Yes |
Controls | Yes | Yes | Yes |
Observations | 1,196 | 1,196 | 1,196 |
Chi2 | 63.089 | 64.386 | 63.863 |
Note: clustered standard errors are displayed in parenthesis. All independent variables are lagged for one year. Control variables include median home value (log) and events of violence, social disorder, guns, and private conflicts.
Significance levels: * p<0.00; ** p<0.01; *** p<0.001.
Table 10.4 One-year lagged independent variables
Events of Private Conflict | Events of Social Disorder | Events of Violence | |||||||
---|---|---|---|---|---|---|---|---|---|
(1) | (2) | (3) | (4) | (5) | (6) | (7) | (8) | (9) | |
Airbnb Penetration (lag 1) | 0.041 | –0.115 | 0.546*** | ||||||
(0.039) | (0.118) | (0.133) | |||||||
Airbnb Density (lag 1) | –0.112 | –0.426 | 1.407* | ||||||
(0.227) | (0.293) | (0.614) | |||||||
Airbnb Usage (lag 1) | 0.001 | –0.011 | 0.037 | ||||||
(0.009) | (0.016) | (0.021) | |||||||
Tract FE | Yes | Yes | Yes | Yes | Yes | Yes | Yes | Yes | Yes |
Year FE | Yes | Yes | Yes | Yes | Yes | Yes | Yes | Yes | Yes |
Controls | Yes | Yes | Yes | Yes | Yes | Yes | Yes | Yes | Yes |
Observations | 1,004 | 1,004 | 1,004 | 1,004 | 1,004 | 1,004 | 1,004 | 1,004 | 1,004 |
F | 0.62 | 0.16 | 0.04 | 0.8 | 1.32 | 0.79 | 8.7 | 2.69 | 1.56 |
Note: clustered standard errors are displayed in parenthesis. Control variable is median household income. Significance levels: * p<0.05; ** p<0.01; *** p<0.001.
10.6 Discussion
We have used the expansion of Airbnb across the neighborhoods of Boston, MA to illustrate an overarching framework for considering how sharing economy platforms can impact neighborhoods. Airbnb has epitomized the way that such platforms have changed the geographic locus of certain commercial transactions, often by expanding them from business districts into more residential communities. In turn, cities like Boston have seen Airbnb listings direct more and more tourists to residential areas. Many have expressed concerns at the externalities that this unexpected influx of visitors might create. Here we articulated and tested three specific hypotheses for what these might be: (1) encouraging greater investment in property; (2) expanding the local hospitality industry; and (3) increasing crime and social disorder.
The empirical test provided support for our first hypothesis as well as some suggestive evidence for our third. Neighborhoods with increasing prevalence of Airbnb listings saw more investment in property, as measured through building permit applications approved by the City of Boston. Meanwhile, we saw mixed evidence for the other two hypotheses. Part of this might be limitations from the outcome measures we used. In particular, counting new restaurants is a constrained approach that requires that the hospitality industry expanded sufficiently to support entire new businesses. Researchers using the more nuanced measure of restaurant employment in New York City did find that Airbnb led to localized growth in the industry (Alyakoob & Rahman, Reference Alyakoob and Rahman2019). Meanwhile, we see some initial evidence that Airbnb listings in a neighborhood are associated with increases in public violence in a neighborhood.
The suggestion that Airbnb might be associated with increases in public violence is intriguing, if preliminary. Admittedly, we sought here to illustrate a generalized framework for pursuing hypotheses on the externalities of sharing economy platforms. As such, this analysis and finding does not fully engage the more nuanced set of relationships between Airbnb and crime that we proposed earlier. To reiterate, Airbnb might impact crime through either of two pathways. First, tourists could directly raise crime, either by perpetrating crimes or acting as attractive targets for criminals. Alternatively, a density of Airbnb listings may undermine local social dynamics that mitigate and prevent crime. A seminal concept in criminology known as social disorganization theory posits that communities with high residential turnover will have difficulty creating and sustaining the relationships and norms necessary for limiting crime (Sampson, Reference Sampson2012). Consistent with this model, Airbnb is the most transient type of household possible, literally “turning over” every few days. To this end, the listings may leave gaps in the social fabric off the neighborhood that could permit crime to increase. Adjudicating between these two hypotheses requires a targeted conceptual approach and methods that attend to the nuanced differences between them. We conduct such a test in a recently published study in a separate venue (Ke et al., Reference Ke, O’Brien and Heydari2021). We do indeed find evidence that Airbnb listings lead to increased crime in neighborhoods by undermining the local social organization – not because they attract tourists, as one might assume. By undermining a neighborhood’s social organization, higher Airbnb penetration diminishes the natural ability of a neighborhood to counteract and discourage crime, specifically violent crime.
Our goal in presenting this study is to highlight three important considerations, one conceptual, one methodological, and one practical. Taking the conceptual first, the framework here provides a guide for examining how other sharing economy platforms might be impacting neighborhoods. The study of each will first need to determine if and how the platform in question is in fact shifting the geographic distribution of commerce. It then will need to proceed to reasoning how those transactions might be altering existing behavioral or economic dynamics. In the current case, we reasoned that Airbnb highlights the value of local housing stock, thereby stimulating investment by property owners. We also discussed how the introduction of tourists might lead to more patrons at local restaurants, but also more disorder and crime. Similar logics would need to be developed for each sharing economy platform and might reveal that certain platforms would be more likely than others to have tangible impacts on communities.
Working through the first two steps of our conceptual process raises a crucial methodological consideration. How does one quantify the presence of a sharing economy platform in a neighborhood? In order to maintain brevity and avoid ambiguity, most scientific studies present and justify a single measure for a quantity of interest. This is a truism for science in general, and has become pronounced in recent years with the use of naturally occurring data sets, including but not limited to the data generated by sharing economy platforms (O’Brien, Sampson, & Winship, Reference O’Brien, Sampson and Winship2015). In contrast, we have taken the opportunity here to detail our own struggle to identify a single measure for the presence of Airbnb in a neighborhood, revealing that multiple measures are possible and that they are neither statistically nor conceptually equivalent. This highlights the challenge faced by researchers in this space and makes clear that the measures themselves need to be developed with care. Further, researchers need to be clear on the interpretation of the measure or measures they select. To illustrate this latter point, there are clear differences between the meaning of our three measures. Whereas high density could be created by a few large buildings with many units listed, penetration reflects a more general distribution of listings throughout a neighborhood. The latter might better reflect the exposure of many parts of a neighborhood and their residents to Airbnb. Meanwhile, the usage measure, which estimates the total number of visitors to the neighborhood, might be most relevant for hypotheses regarding the volume of impact of individual tourists.
Last, it is important to consider how this framework might be a useful tool as local governments look to manage and regulate the sharing economy. Presumably, the goal of such regulations is to maximize the benefits generated by the sharing economy and minimize the negative impacts. Research of the sort presented here will inform policymakers to the specific positive and negative externalities that should be taken into account when designing such regulations. Here we see that Airbnb is leading to more investment in neighborhood properties, which might be argued as a positive outcome. It joins, however, a wealth of evidence that the expansion of Airbnb listings can lead to increases in housing and rental costs and gentrification more generally. Municipal leaders need to weigh these costs and benefits alongside each other when determining how a regulation will impact neighborhoods.
Meanwhile, combining the results here with our further analysis elsewhere (Ke et al., Reference Ke, O’Brien and Heydari2021), we see that Airbnb can also lead to elevated levels crime and disorder in a neighborhood. But the crucial question of measurement returns, this time highlighting how intervention requires not only an understanding of the externalities of the sharing economy, but also of the mechanisms by which these externalities occur. Comparing different ways of measuring the presence of Airbnb in a neighborhood, the increase in crime appears to have been instigated not by the arrival of tourists, as many have assumed. Instead, the listings themselves create a transience that can undermine local social networks and the community’s ability to prevent crime. There have been arguments that these sorts of effects are nonlinear and are only visible as they reach certain thresholds (Clear et al., Reference Clear, Rose, Waring and Scully2003). Fittingly, the same has been demonstrated for gentrification (Hwang & Sampson, Reference Hwang and Sampson2014), which is the potential concern arising from increases in building permits.
With information like that provided by our analysis, cities might be able to develop more informed regulations. Complete bans of Airbnb listings are a blunt tool that foregoes any of the possible benefits they might offer, like stimulating local businesses. Cities like Boston, MA, should be applauded for pursuing a middle ground, but the question is whether they were designed to best support communities. Boston, similar to other locales, has placed the focus on limiting property owners to renting out only one unit in an owner-occupied building at a time. This does limit the overall number of listings, but it has its weaknesses. At the extreme, it could lower density of short-term rentals in the neighborhood while permitting maximal penetration across its parcels. The results in studies based on the suggested framework here (Ke et al., Reference Ke, O’Brien and Heydari2021), would suggest that the negative externalities of the listings could still take hold in such a situation. Policymakers might instead consider quotas of parcels within a neighborhood that can have one or more short-term rentals. In conclusion, this framework can support well-crafted empirical research that then can be translated into well-designed policies. This is critical as it becomes increasingly clear that sharing economy platforms will be a major component of the commercial landscape for the foreseeable future.