Implications
Resistance, tolerance and resilience are components of overall robustness, reflecting the host’s adaptation to environmental stressors and its interaction with disease mediated factors. Increasing environmental challenges, for example, due to global climate change, lead to a higher infection pressure by various pathogens. Metabolic stress, especially in high yielding cows, is a major reason for increased incidence rates for non-infectious diseases. Environmental alterations can impair the bovine immune system, having a direct or indirect effect on disease susceptibility. There is an urgent need to identify suitable phenotypes and novel markers related to immunological mechanisms, to improve overall immune response (IR) via optimized breeding strategies.
Introduction
Breeding on robustness via selection for enhanced disease resistance is of increasing importance in the dairy livestock industry worldwide (Calus et al., Reference Calus, Berry, Banos, de Haas and Veerkamp2013). Classical breeding goals focussed on improving milk or protein yield, but simultaneously neglected functional traits or health (Egger-Danner et al., Reference Egger-Danner, Cole, Pryce, Gengler, Heringstad, Bradley and Stock2015). As an antagonistic effect, long-term selection on increased productivity was associated with physiological and immunological imbalances, especially in the early lactation period. Immunological imbalances might stimulate the susceptibility to environmental influences, thus causing a decline in robustness (Rauw and Gomez-Raya, Reference Rauw and Gomez-Raja2015).
Negative side effects due to intensive selection on milk yield, the increasing value of animal welfare, ethical aspects and profit maximization via reduced medical treatments (e.g. antibiotics, anthelmintics) are the main arguments supporting breeding strategies on improved disease resistance. Moreover, environmental challenges, especially the heterogeneity of climatic impact, are major driven forces towards breeding of robust dairy cows. Breeding on robustness implies the identification of animals with a quite high and constant production level for a wide range of climatic conditions and production systems, accompanied with a high level of animal welfare (Rauw and Gomez-Raja, Reference Rauw and Gomez-Raja2015). The inclusion of functional traits into overall dairy cattle breeding goals was a first step towards breeding on robustness (Martin et al., Reference Martin, Barkema, Brito, Narayana and Miglior2018), implying rapid advances in genetic evaluations for traits being linked to disease resistance or tolerance.
The first section of this review addresses classical breeding approaches in dairy cattle in order to improve robustness via modelling reactions to environmental alterations. Relevant genotype by environment (G × E) interactions in dairy cattle (e.g. environmental sensitivity, reaction norms, phenotypic plasticity) will be discussed, considering new characteristics of disease associated factors (e.g. herd prevalence, pathogen load) as environmental descriptors. The inclusion of binary subjectively recorded producer health traits into overall breeding goals is a next step to improve disease resistance. Third, suitable novel biomarkers for resistance, tolerance and resilience-associated traits from laboratory analyses (e.g. enzymes, milk fatty acids (MFA)), will be reviewed (e.g. Pryce et al., Reference Pryce, Gaddis, Koeck, Bastin, Adessayed, Gengler, Miglior, Heringstad, Egger-Danner and Stock2016; Pieper et al., Reference Pieper, Staufenbiel, Christ, Panicke, Müller and Brockmann2016). Advances in laboratory analysis also allow deeper insights into immune genetics, aiming on the identification of suitable and practicable IR traits (e.g. Thompson-Crispi et al., Reference Thompson-Crispi, Sewalem, Miglior and Mallard2012). In this context, we will infer associations between IR traits and relevant production and health traits, in order to bridging the gap between immunity and selection for robustness.
Breeding approaches to improve robustness in dairy cows
On a genetic basis, robustness in dairy cows refers to the adaptation ability to a wide range of environmental conditions, that is, implying selection of genotypes with quite stable genetic values in different environments. Hence, those animals are robust against the impact of possible G × E interactions (Rauw and Gomez-Raja, Reference Rauw and Gomez-Raja2015). The concept of G × E interactions applied to production traits in terms of reduced environmental sensitivity contributed to robustness in dairy cows, without extending recording schemes for novel functional traits. Environmental sensitivity is associated with disease susceptibility, and changes of IR mechanisms. In order to improve disease resistance via direct breeding strategies, health traits on the basis of producer-recorded data were included gradually into overall dairy cattle breeding goals over a period of ∼20 years.
The classical approach: detection and use of genotype by environment interactions for conventional traits
Gause et al. (Reference Gause1947) introduced the term environmental sensitivity or phenotypic plasticity, which describes the rate of phenotypic alterations in response to changes in the environment. As outlined by de Jong and Bijma (Reference De Jong and Bijma2002), robust genotypes express quite constant phenotypes across environments. Oppositely, genotypes with high variable phenotypes are more sensitive to environmental impact. Plasticity in protein turnover, antibody production, immune cell response or in stress-response neuroendocrine axis function is related to robustness, due to the strong effects on a wide range of physiological processes (e.g. reproduction, metabolism, IR, combating infections) (Mormède et al., Reference Morméde, Foury, Terenina and Knap2011; Magombedze et al., Reference Magombedze, Reddy, Eda and Ganusov2013).
Phenotypic plasticity also explains G × E interactions. Differences in trait reactions between genotypes in different environments indicate possible G × E interactions. Methodological concepts for the detection of G × E interactions based on reactions of ‘simple’ production or fertility traits in dependency of an environmental descriptor (e.g. temperature, disease exposure, feeding regime). As a simple approach, multiple trait animal or sire models were applied, implying the definition of discrete environmental classes (e.g. Nauta et al., Reference Nauta, Veerkamp, Brascamp and Bovenhuis2006; Haile-Mariam et al., Reference Haile-Mariam, Carrick and Goddard2008). In multiple trait models, the trait of interest was defined as a separate trait in different environments. The proof for possible G × E interactions bases on genetic correlations lower than 0.80 between same traits from different environments (Robertson, Reference Robertson1959).
G × E interactions for production and fertility traits were detected when stratifying data according to geographic differences within countries (e.g. König et al., Reference König, Dietl, Raeder and Swalve2005; Haile-Mariam et al., Reference Haile-Mariam, Carrick and Goddard2008), but also across country borders (e.g. Montaldo et al., Reference Montaldo, Pelcastre-Cruz, Castillo-Juárez, Ruiz-López and Miglior2017). König et al. (Reference König, Dietl, Raeder and Swalve2005) only identified indications for G × E interactions when considering several environmental descriptors simultaneously, for example, herd location and herd size. Such findings were motivation for a ‘borderless clustering’ approach in international genetic evaluations (Weigel and Rekaya, Reference Weigel and Rekaya2000). Furthermore, genetic correlations were generally smaller for low heritability functional traits compared to production traits. For example, Boettcher et al. (Reference Boettcher, Fatehi and Schutz2003) created the discrete production environments ‘grazing’ and ‘conventional’. The genetic correlation for calving interval was 0.64, but close to 1 for milk yield. One explanation for obvious G × E of fertility traits addresses pronounced environmental sensitivity in physiological hormone levels and immune functions, compared to the stable hormonal impact on milk production traits (Chagas et al., Reference Chagas, Bass, Blache, Burke, Kay, Lindsay, Lucy, Martin, Meier, Rhodes, Roche, Thatcher and Webb2007). Nauta et al. (Reference Nauta, Veerkamp, Brascamp and Bovenhuis2006) reported genetic correlations slightly below or larger than 0.80 between organic and conventional protein yield. On the other hand, for different production systems in Australia, the genetic correlation for low heritability pregnancy rates was only 0.37 (Haile-Mariam et al., Reference Haile-Mariam, Carrick and Goddard2008). For low genetic correlations across environments, an appropriate breeding objective for a given environment should contain the trait-associated environment with appropriate index weights. This is already current practice in many other species’ breeding where selection focusses on field traits, but many data, however, coming from station data (Cardoso and Tempelmann, Reference Cardoso and Tempelmann2012).
An alternative way to detect and to use G × E is the application of random regression or reaction norm models to longitudinal data, which enables estimations of genetic (co)variance components and estimated breeding values (EBV) for a broad grid of continuous environmental variables (e.g. Calus and Veerkamp, Reference Calus and Veerkamp2003). Utilized continuous environmental descriptors reflected the herd management (e.g. average herd milk production, body condition score (BCS), persistency), or climate characteristics (e.g. temperature–humidity index (THI)) (Calus and Veerkamp, Reference Calus and Veerkamp2003; Fikse et al., Reference Fikse, Rekaya and Weigel2003; Haile-Mariam et al., Reference Haile-Mariam, Carrick and Goddard2008). Body condition score as a continuous herd descriptor reflects the dairy cow energy balance, being strongly related to disease resistance and tolerance (Calus and Veerkamp, Reference Calus and Veerkamp2003; Fikse et al., Reference Fikse, Rekaya and Weigel2003). For example, a cow with constantly low BCS scores has no defense mechanisms against invading pathogens. In this regard, Calus and Veerkamp (Reference Calus and Veerkamp2003) estimated sire breeding values for milk production traits of Dutch dairy cows in dependency of continuous herd BCS. Moreover, lactation persistency was defined as an environmental descriptor reflecting herd management (Calus and Veerkmap, Reference Calus and Veerkamp2003; Fikse et al., Reference Fikse, Rekaya and Weigel2003), and refers to the rate of decline in the lactation curve after the cows lactation peak. Positive genetic correlations in the range from 0.15 to 0.84 between persistency and relative peak milk yield were estimated, suggesting a higher relative peak milk yield in high persistency herds (e.g. Fikse et al., Reference Fikse, Rekaya and Weigel2003). Low persistency was strongly associated with increased susceptibility to stress, explaining improved host defense against infectious diseases in persistent herds (Calus et al., Reference Calus, Berry, Banos, de Haas and Veerkamp2013). Twomey et al. (Reference Twomey, Graham, Doherty, Blom and Berry2018a) identified re-rankings of sires for fertility traits across environments differing in endoparasite load, that is, using herd prevalences for the liver fluke Fasciola hepatica to measure genetic variability in resilience. In this regard and from a breeding perspective, resilience is defined as the ability of an animal to maintain performance during any environmental perturbation, including high pathogen loads (Bishop, Reference Bishop2012; Colditz and Hine, Reference Colditz and Hine2016). No G × E interaction was detected for milk production traits, reflecting that fertility is more influenced by F. hepatica infections compared to production traits (Twomey et al., Reference Twomey, Graham, Doherty, Blom and Berry2018a). This was also shown in own studies for different Black and White dairy cattle selection lines being infected with F. hepatica (May et al., Reference May, Brügemann, König and Strube2018).
Tolerance to heat stress is a major component of robustness, reflecting the cow’s ability to maintain performance during challenging climatic conditions. Possible G × E interactions were evaluated across levels of THI (e.g. Brügemann et al., Reference Brügemann, Gernand, von Borstel and König2013). As reviewed by Carabaño et al. (Reference Carabaño, Ramón, Díaz, Molina, Pérez-Guzmán and Serradilla2017), genetic correlations between productivity of Holstein cattle kept in cold climatic conditions and productivity under heat stress ranged from 0.40 to 0.98. However, for the functional traits conception rate and somatic cell score (SCS), a substantial decline of genetic correlations was observed, especially when correlating measurements from THI in great distance (Brügemann et al., Reference Brügemann, Gernand, von Borstel and König2013). On a genetic basis, variations of additive-genetic (co)variance components by THI might indicate that different genes are ‘switched on or off’ under certain environmental conditions. Reaction norm models are suitable to analyse G × E, because of the modelling of phenotypic variation as a function of an environmental descriptor. In such perspective, environmental sensitivity describes the first derivative (i.e. the slope) of the defined reaction norm function. A steep slope indicates high environmental sensitivity (e.g. Streit et al., Reference Streit, Reinhardt, Thaller and Bennewitz2012). In a random regression approach, breeding values for the regression coefficient depend on the value of the regression variables. Therefore, breeding objectives, but alo index traits, could be defined by simply fixing the desired target regression value. Another advantage of this type of models is their capacity to accommodate and to use all response data along the trajectory of the regression variables.
The next step: inclusion of producer health data in breeding objectives
Genetic evaluations for health traits are mostly based on producer-recorded data. Most commonly, diagnosis keys or recording guidelines depict hierarchical entry systems for the overall disease categories claw disorders, mastitis, fertility disorders and metabolic disorders, with possibilities for single-disease trait specifications. In most studies, heritabilities for binary-defined producer-recorded disease traits were quite low. Zwald et al. (Reference Zwald, Weigel, Chang, Welper and Clay2004) estimated heritabilities for producer-recorded binary health data (presence or absence of the respective disease) in the range from 0.07 and 0.18 for the economic relevant disorders mastitis, lameness, ketosis and cystic ovaries. Heritabilities are in line with estimates by Neuenschwander et al. (Reference Neuenschwander, Miglior, Jamrozik, Berke, Kelton and Schaeffer2012), reporting heritabilities from 0.02 to 0.21 for producer health data from Canada. Low disease incidences and poor data quality, because of subjective trait recording, makes genetic evaluations for health traits to a challenge (Neuenschwander et al., Reference Neuenschwander, Miglior, Jamrozik, Berke, Kelton and Schaeffer2012). Advances for developing a routine genetic evaluation for metabolic disease traits were made in Canada by combining producer health data with disease indicator measurements from the laboratory, for example, β-hydroxybutyrate (BHB) (Pryce et al., Reference Pryce, Gaddis, Koeck, Bastin, Adessayed, Gengler, Miglior, Heringstad, Egger-Danner and Stock2016). Heritabilities for objective laboratory measurements were larger compared to subjective binary producer scores, suggesting additional selection response when including BHB into overall breeding indices.
Recently, Martin et al. (Reference Martin, Barkema, Brito, Narayana and Miglior2018) reviewed breeding strategies to improve mastitis resistance. In Scandinavia, resistance to mastitis (based on a veterinarian recording scheme for unspecific clinical mastitis (CM)) was included into overall breeding goals since the 1960s. In Canada, a national genetic evaluation for producer-recorded CM was implemented in 2014. Heritability estimates for producer-recorded CM were quite small, in the range from 0.01 and 0.10 (Carlén et al., Reference Carlén, Strandberg and Roth2004; Zwald et al., Reference Zwald, Weigel, Chang, Welper and Clay2004). Simple recording of CM (presence/absence) does not cover intensities of infection and udder pathogen burden. Thus, the estimation of genetic parameters for major specific pathogens was proposed as a pre-requisite for the development of udder health indices with different economic weights for different pathogens. However, also heritabilities for different major pathogens causing CM were quite small, and the specification of pathogens via bacteriological analyses is currently too expensive for commercial breeding applications (Sorensen et al., Reference Sorensen, Mark, Madsen and Lund2009).
Resistance to claw disorders considers the simple trait lameness (presence/absence), or individual claw disorders with high incidences (e.g. dermatitis digitalis (DD)). Again, also for producer-recorded claw disorders, heritabilities were small, in a narrow range from 0.03 to 0.08 (Zwald et al., Reference Zwald, Weigel, Chang, Welper and Clay2004; Neuenschwander et al., Reference Neuenschwander, Miglior, Jamrozik, Berke, Kelton and Schaeffer2012). For claw disorders, the application of detailed scoring systems improved phenotype quality, being a better data basis to infer the genetic background of the respective disease. As elaborated by Schöpke et al. (Reference Schöpke, Gomez, Dunbar, Swalve and Döpfer2015), heritabilities for DD increased when applying a more precise scoring system, considering the variety of disease stages.
Potential of (novel) biomarkers to improve robustness to environmental stressors and disease
Biomarkers are objectively measurable indicators to infer biological processes, physiological conditions (e.g. reaction to environmental stressors) or giving a prognostic or diagnostic information value in the face of infectious or non-infectious diseases (for definition, see Biomarkers Definitions Working Group, 2001). Potential biomarkers include enzymes, serum metabolites, hormone levels or characteristics of body fluids. New technologies such as mid-infrared spectrometry (MIR) in milk can be used to monitor cow health. Mid-infrared spectrometry is a ‘by-product’ from routine milk analysis and provides suitable biomarkers (e.g. MFA) for monitoring diseases (e.g. mastitis, ketosis) (Bastin et al., Reference Bastin, Soyeurt and Gengler2013; Pryce et al., Reference Pryce, Gaddis, Koeck, Bastin, Adessayed, Gengler, Miglior, Heringstad, Egger-Danner and Stock2016). Novel biomarkers as actually used in quantitative-genetic studies are listed in Table 1, all offering potential for the identification of genetically robust dairy cattle.
Table 1 Heritability estimates in HF dairy cows for novel biomarkers (somatic cell count and fat-to-protein ratio not included) as indicators of resistance-, tolerance- and resilience-associated traits in studies as from 2000 (ordered chronologically for each biomarker)Footnote 1

MIR = assessed by mid-infrared spectrometry; RRM = random regression model; AMS = automatic milking system; DIM = Days in milk; HF = Holstein Friesian; L = lactation.
1 The list of references for Table 1 is given in Supplementary Material S1.
Biomarkers for udder health
Regarding resistance to udder infections, test-day SCS and lactation mean SCS are reliable biomarkers, with heritabilities in a range from 0.05 to 0.19 (Martin et al., Reference Martin, Barkema, Brito, Narayana and Miglior2018). Large positive genetic correlations between SCS and CM were motivation for the inclusion of SCS as an indicator trait into selection indexes, allowing the consideration of mastitis resistance into breeding goals (Martin et al., Reference Martin, Barkema, Brito, Narayana and Miglior2018). Further alternative SCS trait definitions, for example, SCS only from early lactation, or SD of SCS, explained a larger proportion of breeding value variations for mastitis resistance, compared to lactation SCS (Martin et al., Reference Martin, Barkema, Brito, Narayana and Miglior2018).
Minerals (e.g. Ca, K, Mg, Zn, Se, P) or mineral content measured via MIR in bovine milk can be used as potential biomarkers to improve mastitis resistance (Egger-Danner et al., Reference Egger-Danner, Cole, Pryce, Gengler, Heringstad, Bradley and Stock2015). As shown in Table 1, heritabilities for minerals in milk ranged from 0.20 for selenium to 0.62 for phosphorus. Moreover, milk glycoprotein lactoferrin (LTF) was proposed as a reliable biomarker for mastitis. Lactoferrin as an antibacterial and antifungal molecule plays a crucial role in host defense mechanisms, and reacted better for some type of mastitis pathogens compared to SCS (Farnaud and Evans, Reference Farnaud and Evans2003). Regarding studies from the past 20 years, heritabilities for LTF measured via MIR ranged from 0.20 to 0.22 (Table 1). Lactic acid was suggested as a potential biomarker for clinical mastitits in early lactation, but heritability estimates were close to 0 in Danish Holstein cows (Table 1).
During the past years, electrical conductivity (EC) in milk was considered as a novel biomarker in selection indices for improved udder health. Heritabilities for test-day EC records ranged between 0.22 and 0.39 (Table 1). Large genetic correlations (0.75) between test-day EC records and mastitis indicate genetic improvements for mastitis resistance through selection on reduced EC (Norberg et al., Reference Norberg, Rogers, Ødegård, Cooper and Madsen2006). For EC generated from automatic milking systems, larger heritability estimates between 0.37 and 0.51 were reported (Table 1). Generally, automatic milking systems are a new technical opportunity to generate a longitudinal data structure for objectively recorded health indicator traits (Santos et al., Reference Santos, Brügemann, Ebinghaus and König2018).
Biomarkers for metabolic health disorders
Biomarkers to measure energy balances and metabolic diseases include fat–protein ratio (FPR), ketone bodies (e.g. BHB, acetone), non-esterified free fatty acids (NEFA) or phospholipids, glucose and insulin growth factor 1 (IGF-1), which can be measured in blood, urine and in milk. Currently, the FPR generated from routine milk recording data, is a selection criterion to improve metabolic stability (Koeck et al., Reference Koeck, Jamrozik, Schenkel, Moore, Lefebvre, Kelton and Miglior2014). A FPR above 1.5 indicates abnormally high lipolysis, resulting in energy deficiency and metabolic stress in early lactation. Positive genetic correlations from 0.30 to 0.63 between FPR and metabolic diseases (e.g. ketosis, acidosis) were reported (Pryce et al., Reference Pryce, Gaddis, Koeck, Bastin, Adessayed, Gengler, Miglior, Heringstad, Egger-Danner and Stock2016), suggesting utilization of FPR as a ‘low-cost indicator’ for the metabolic disease status. However, FPR is highly affected by feeding regime and BCS. Hence, from a genetic perspective, BHB might be a better biomarker to predict ketosis. Ketosis is associated with an increase of the metabolite BHB in blood (gold standard for diagnosis) or in milk. Heritabilities for BHB were in a range from 0.04 to 0.40 (Table 1), depending on lactation stage. Thus, BHB was used as a biomarker in genetic or genomic approaches to breed cows with decreased susceptibility to ketosis (Pryce et al., Reference Pryce, Gaddis, Koeck, Bastin, Adessayed, Gengler, Miglior, Heringstad, Egger-Danner and Stock2016). Genetic correlations between BHB with FPR ranged from 0.12 to 0.49, and between BHB with clinical ketosis from 0.37 to 0.75 (Koeck et al., Reference Koeck, Jamrozik, Schenkel, Moore, Lefebvre, Kelton and Miglior2014; Pryce et al., Reference Pryce, Gaddis, Koeck, Bastin, Adessayed, Gengler, Miglior, Heringstad, Egger-Danner and Stock2016). Heritabilities for acetone concentration ranged from 0.01 to 0.29 in early lactation (Table 1). Moreover, NEFA in plasma as a product of body fat mobilization might be suitable indicators for the overall metabolic health status. Heritabilities for NEFA from random regression model were in the range from 0.08 to 0.35. Non-esterified free fatty acids peaked in week 1 of lactation and provides a substrate for BHB, resulting in the highest levels of BHB in the 2nd week of lactation (Klein et al., Reference Klein, May, Scheper and König2018). In this regard, a higher accuracy of predicting ketosis can be achieved when considering more than one biomarker (BHB together with NEFA or FPR) from MIR (Grelet et al., Reference Grelet, Bastin, Gelé, Davière, Johan, Werner, Reding, Fernandez Pierna, Colinet, Dardenne, Gengler, Soyeurt and Dehareng2016).
The consideration of MFA as biomarkers to detect hyperketonaemia and periods of negative energy balance is of growing interest. Van Haelst et al. (Reference Van Haelst, Beeckman, van Knegsel and Fievez2008) observed a significant higher secretion of long-chain fatty acid C18:1 cis-9 in milk in subclinical ketosis diagnosed cows. Moreover, an increase in C18:1 cis-9 was identified as a biomarker for a decrease in energy balance due to reduced feed intake and nutrient absorption, especially under hot conditions (Hammami et al., Reference Hammami, Vandenplas, Vanrobays, Rekik, Bastin and Gengler2015). Bastin et al. (Reference Bastin, Soyeurt and Gengler2013) estimated heritabilities for a broad range of longitudinal MFA (e.g. butanoic acid (C4:0), caprylic acid (C8:0)). As shown in Table 1, heritabilities for short- and medium-chain MFA ranged from 0.35 to 0.59, depending on the lactation stage. Heritabilities for long-chain fatty acids were generally lower (0.18).
Tetens et al. (Reference Tetens, Heuer, Heyer, Klein, Gronwald, Junge, Oefner, Thaller and Krattenmacher2015) showed that phospholipids as fatty acids in milk (e.g. phosphatidylcholine (PC), glycerophosphocholine (GPC) and the ratio of both metabolites) are suitable prognostic biomarker for ketosis. A heritability of 0.48 for the metabolite GPC was estimated in a population of Danish Holstein cows (Table 1). Hence, selection of cows with a high GPC level or a high GPC/PC ratio is associated with improved ketosis resistance in early lactation. Currently, intensive recording of phospholipids is very expensive and logistics are difficult to implement in practice, thus, also hampering breeding efforts (Tetens et al., Reference Tetens, Heuer, Heyer, Klein, Gronwald, Junge, Oefner, Thaller and Krattenmacher2015). Further approaches suggested consideration of parameters from intravenious glucose tolerance test for breeding aspects (Pieper et al., Reference Pieper, Staufenbiel, Christ, Panicke, Müller and Brockmann2016). Heritabilities in Holstein Friesian (HF) bulls ranged from 0.12 to 0.43 for different blood glucose parameters (e.g. fasting glucose concentration, glucose half-life period) (Pieper et al., Reference Pieper, Staufenbiel, Christ, Panicke, Müller and Brockmann2016).
A negative energy balance during the early lactation period is associated with major alterations in the growth hormone IGF-1. Piechotta et al. (Reference Piechotta, Sander, Kastelic, Wilde, Heppelmann, Rudolph, Schuberth, Bollwein and Kaske2012) showed that prepartum plasma IGF-1 concentrations were lower in cows with postpartum diseases, revealing the potential of IGF-1 in genetic and genomic approaches. Genetic variation for IGF-1 was detected in dairy cows in Poland (Table 1), with a moderate heritability of 0.35.
Rumen microbiome composition as a biomarker
Recently, biomarkers from the rumen microbiome were suggested to study metabolic performances and disease susceptibilities in dairy cattle (Jewell et al., Reference Jewell, McCormick, Odt, Weimer and Suen2015). There is evidence for the existence of inter-individual differences in microbiome compositions (Lin et al., Reference Lin, Raskin and Stahl1997), hypothesizing that the host genome has substantial influence on the rumen microbiome composition. In addition, variations in feed efficiency were associated with differences in rumen microbial community compositions, microbiome–host interactions or both (Jewell et al., Reference Jewell, McCormick, Odt, Weimer and Suen2015). Hernandez-Sanabria et al. (Reference Hernandez-Sanabria, Goonewardene, Wang, Mi, Moore and Guan2013) showed that variations in particular microbial phylotypes due to host sire effects influenced rumen microbial metabolic processes, and ultimately determined residual feed intake (RFI). Thus, interactions between host genotypes and environmental factors (e.g. diet) regulate presence or absence of particular microbes, as well as RFI. In addition, interactions between an animal’s genome and rumen microbiome composition have impact on the occurrence and resistance to a number of infectious and non-infectious diseases. In consequence, cows with a specific ruminal composition might be more resistant against specific infectious and non-infectious diseases. Variability and specificity of MFA was associated with the cow energy status and rumen health, indicating cow robustness (Bastin et al., Reference Bastin, Soyeurt and Gengler2013). Heritabilities for MFA ranged between 0.03 and 0.59 (Table 1). However, because of the novelty of this approach, genetic parameters for direct rumen health or microbial composition traits are lacking. Only Sasson et al. (Reference Sasson, Ben-Shabat, Seroussi, Doron-Faigenboim, Shterzer, Yaacoby, Berg Miller, White, Halperin and Mizrahi2017) analysed relationships between host genetic architectures with phylogenetic and functional compositions of the rumen microbiome. They identified several microbial groups being heritable and linked to rumen metabolic parameters and productivity in dairy cows. Hence, there is evidence that host genetic variation is associated with specific microbes, and that the bovine rumen microbiome includes heritable components, encouraging further studies on a molecular genetics scale. So far, molecular host response mechanisms determining variation in the microbial populations, are unknown.
Biomarkers for heat stress
Hot temperatures and humidity directly affect physiology (e.g. increase in respiration or heart rate), feed intake, endocrine mechanisms and the effective IR in dairy cattle, leading to enhanced disease susceptibility (Das et al., Reference Das, Sailo, Verma, Bharti, Saikia, Imtiwati and Kumar2016). Sanders et al. (Reference Sanders, Shearer and De Vries2009) observed increased incidence rates for claw disorders with increased air temperature. Increasing THI often causes high reproduction rates for a broad range of pathogens (e.g. bacterials, parasites). Thus, the risk for mastitis and endoparasite infections increases, leading to measurable changes in disease-related biomarkers (e.g. SCS). Heat stress response is regulated via endocrine, metabolic, physiological and cellular mechanisms, resulting in up- and downregulations for a number of metabolites (Hammami et al., Reference Hammami, Vandenplas, Vanrobays, Rekik, Bastin and Gengler2015; Al-Kanaan et al., Reference Al-Kanaan2016). Under heat stress, plasma levels of urea and insulin increases, while plasma glucose decreases (Wheelock et al., Reference Wheelock, Rhoads, VanBaale, Sanders and Baumgard2010). Alterations in these biomarkers are directly linked to cows energy balance and might increase susceptibility to metabolic disorders (e.g. ketosis) (West, Reference West2003). In consequence, biomarkers responding in the course of a metabolic disease (e.g. creatine, acetoacetate, arachidonic acid, BHB), are very sensitive in heat stressed lactating dairy cows (Carabaño et al., Reference Carabaño, Ramón, Díaz, Molina, Pérez-Guzmán and Serradilla2017). Hammami et al. (Reference Hammami, Vandenplas, Vanrobays, Rekik, Bastin and Gengler2015) measured fatty acids in milk via MIR, and identified C18:1 cis-9 as the most sensitive biomarker for heat stress in HF cows. A possible explanation might be that composition of rumen microbiome was significant different in various temperatures (20°C v. 28°C v. 33°C) (Tajima et al., Reference Tajima, Nonaka, Higuchi, Takusari, Kurihara, Takenaka, Mitsumori, Kailkawa and Aminov2007), implying a change of nutrient composition in the rumen and fatty acids in milk. In addition, milk protein fractions such as caseins were utilized as suitable biomarkers for heat stress (Carabaño et al., Reference Carabaño, Ramón, Díaz, Molina, Pérez-Guzmán and Serradilla2017). In recent years, heat shock proteins (HSP) as an expression of cellular stress during heat stress impact have been carefully evaluated. Heat shock protein 70 (HSP70) concentration in plasma and plasma HSP72 were suggested as biomarkers for chronic heat stress, environmental stress and for disease resistance in HF dairy cattle (Carabaño et al., Reference Carabaño, Ramón, Díaz, Molina, Pérez-Guzmán and Serradilla2017).
Al-Kanaan (Reference Al-Kanaan2016) analysed different bull semen characteristics and body fluids on a quantitative-genetic scale in dependency of THI. They identified a strong detrimental temperature × humidity – effect on bull semen parameters, and alterations of bull semen breeding values, beyond THI 60. Thus, selection of sires according to THI-specific breeding values for specific semen traits and body fluids might be a further strategy to identify sires with improved resistance to hot climatic conditions. Further physiological traits as indicators for resilience to heat stress include measurements of body temperature (rectal, vaginal, skin), pulse and respiration rate (Table 1). Reported heritabilities for body temperature traits ranged from 0.02 to 0.17, while heritabilities for pulse and respiration rate were in a range from 0.05 to 0.07 (Table 1).
Biomarker for endoparasite infections
In pasture-based production systems, endoparasite infections caused by gastrointestinal nematodes (GIN) and liver flukes (F. hepatica) are of increasing economic importance, because of strong associations with impaired dairy cow health. Biomarkers for host resistance (i.e. ability to control pathogen burden) or tolerance (i.e. ability to limit the impact of a given pathogen burden on performance) to parasitic infections include biochemical (e.g. serum pepsinogen or albumin level), immunological (serum or milk antibodies) and parasitological (e.g. faecal egg count, worm parameters, helminth-specific antigen, DNA) markers. Serum or milk antibodies (different isotypes of immunoglobulins) and FEC are the most common used indicators to measure resistance. For GIN infections in dairy cattle, heritabilities ranged from 0.05 to 0.21 for FEC, but the heritability for antibody levels measured via ELISA was only 0.07 (Table 1). In literature, negative genetic correlations were reported between FEC and antibody levels (IgG1, IgG2, IgA, IgM) for GIN infections in cattle and small ruminants. Thus, breeding for enhanced resistance to endoparasite infections is possible via selection for lower FEC, or via higher antibody titres. May et al. (Reference May, Brügemann, Yin, Scheper, Strube and König2017) estimated negative genetic correlations up to −0.40 between GIN infections detected by FEC with fat percentage and with protein percentage throughout lactation. In their study, genetic correlations between FEC and milk yield were close to 0. Accordingly, genetic correlations for the antibody response to the GIN Ostertagia ostertagi with milk production traits were close to 0 (Twomey et al., Reference Twomey, Carroll, Doherty, Byrne, Graham, Sayers, Blom and Berry2018b).
For liver fluke infections, Twomey et al. (Reference Twomey, Carroll, Doherty, Byrne, Graham, Sayers, Blom and Berry2018b) used the biomarker ‘IgG antibody titres against F. hepatica’ in Irish dairy cows, and estimated a heritability of 0.13 (Table 1). A heritability of 0.33 was estimated for the biomarker ‘FEC of F. hepatica’ in German Holstein dairy cows (May et al., Reference May, Brügemann, Yin, Scheper, Strube and König2017; see Table 1). Twomey et al. (Reference Twomey, Carroll, Doherty, Byrne, Graham, Sayers, Blom and Berry2018b) estimated negative genetic correlations in a low range (−0.04 to −0.14) between IgG antibody titres against F. hepatica and milk production traits. May et al. (Reference May, Brügemann, Yin, Scheper, Strube and König2017) found negative correlations up to −0.50 between FEC of F. hepatica and protein %. Currently, utilization of FEC or antibody levels to predict resistance or tolerance to endoparasite infections in dairy cows is not feasible, due to the costs and difficulties of measuring phenotypes in a commercial production environment. However, the application of suitable traits measuring overall IR to pathogenic agents will play a greater role in future selection strategies, instead a selection focus on specific infectious diseases.
Inclusion of immunity traits into breeding objectives
The immune system includes two components: The innate (non-specific) and the adaptive (specific) immune system. During the last years, breed-dependent differences and suitable IR traits were investigated for both host defense mechanisms (i.e. the innate and the adaptive immune system), suggesting the potential of IR traits as useful selection markers, in order to achieve enhanced disease resistance. Immune response mechanisms are primarily linked to resistance against infectious diseases. Nevertheless, also the interplay between the immune status and non-infectious diseases (e.g. metabolic health) was investigated in dairy cattle (e.g. Thompson-Crispi et al., Reference Thompson-Crispi, Miglior and Mallard2013). An increase in incidences of various diseases (e.g. mastitis, ketosis) around calving and during the postpartum period was explained trough alterations in response of important IR mediators.
Immune response traits
The genetic background of IR traits has been studied during the past 30 years in dairy cows. In Norwegian Red dairy cattle, heritabilities for serum proteins (complement, conglutinin, lysozyme) and immunoglobulins (Ig) were larger than 0.50 (Lie, Reference Lie1979). Detilleux et al. (Reference Detilleux, Koehler, Freeman, Kehrli and Kelley1994) investigated the genetic variation of serum Ig concentrations and neutrophil functions in HF cows for different immunosuppression stages (day 35 prepartum to day 35 postpartum). Moderate-to-large heritabilities up to 0.84 were estimated for different Ig isotypes (IgG1, IgG2, IgM), depending on the immunosuppression stage. The lactation stage had significant impact on Ig levels, and heritabilities were larger in the periparturient period (Mazengera et al., Reference Mazengera, Kennedy, Burnside, Wilkie and Burton1985; Detilleux et al., Reference Detilleux, Koehler, Freeman, Kehrli and Kelley1994; Wagter et al., Reference Wagter, Mallard, Wilkie, Leslie, Boettcher and Dekkers2000). In dairy cattle, the neutrophil function plays a decisive role as the first defense against udder infections. Heritabilities for specified IR traits being related to mastitis (e.g. neutrophil functions) differed from 0.20 to 0.70 in periparturient Holstein cows (Detilleux et al., Reference Detilleux, Koehler, Freeman, Kehrli and Kelley1994).
Abdel-Azim et al. (Reference Abdel-Azim, Freeman, Kehrli, Kelm, Burton, Kuck and Schnell2005) used a combination of immunologically controlled infectious diseases induced by pathogens (e.g. uterine infections, respiratory diseases) to introduce the overall immune trait ‘generalized immunity (GI)’ in US HF cows. The heritability in this study for GI was 0.20. Further advances have been made in exploring humoral or antibody-mediated immune response (AMIR), and cellular-mediated immune response (CMIR) of the bovine immune system. Immunoglobulins (antibodies) are the mediators of AMIR, produced from B cells as a reaction in the presence of extracellular pathogens. Antigen-presenting cells and cytotoxic T cells stimulate CMIR, being responsible for the protection against intracellular pathogens. A Canadian research group demonstrated selective breeding possibilities for high responders (HR), average responders (AR) or low responders (LR), based on AMIR and on CMIR (Guelph’s patented High Immune Response technology, patent number: CA2255423A1). In this regard, a standardized immunization or IR-testing protocol is used to capture CMIR and AMIR (e.g. Thompson-Crispi et al., Reference Thompson-Crispi, Sewalem, Miglior and Mallard2012 and Reference Thompson-Crispi, Miglior and Mallard2013). In this protocol, hen-egg white lysosyme or ovalbumin are the antigens to measure induced antibody (mostly IgG1 and IgG2) response for AMIR at different days after immunization. Delayed-type hypersensitivity to the yeast fungi Candida albicans, or to the intracellular bacterium Mycobacterium avium subsp. paratuberculosis, induced CMIR.
Heritabilities for CMIR ranged from 0.19 to 0.54 (Thompson-Crispi et al., Reference Thompson-Crispi, Sewalem, Miglior and Mallard2012; Heriazon et al., Reference Heriazon, Quinton, Miglior, Leslie, Sears and Mallard2013). Heritabilities for AMIR were in a broader range from 0.13 to 0.88, depending on the antigen isotype (Wagter et al., Reference Wagter, Mallard, Wilkie, Leslie, Boettcher and Dekkers2000; Thompson-Crispi et al., Reference Thompson-Crispi, Sewalem, Miglior and Mallard2012; Heriazon et al., Reference Heriazon, Quinton, Miglior, Leslie, Sears and Mallard2013). In most cases, genetic correlations between CMIR and AMIR were negative (e.g. Hernandez Reference Hernandez, Quinton, Miglior and Mallard2006; Thompson-Crispi et al., Reference Thompson-Crispi, Sewalem, Miglior and Mallard2012; Heriazon et al., Reference Heriazon, Quinton, Miglior, Leslie, Sears and Mallard2013), indicating that selection of cows with increased resistance to extracellular pathogens (e.g. helminths) might be associated with increasing susceptibility to intracellular pathogens (e.g. bacteria, viruses, protozoa), and vice versa. For example, Twomey et al. (Reference Twomey, Carroll, Doherty, Byrne, Graham, Sayers, Blom and Berry2018b) identified antagonistic genetic relationships between antibody response to the protozoan parasite Neospora caninum with the antibody response to the helminths F. hepatica (−0.29) and O. ostertagi (−0.67) in Irish dairy cattle.
Relationships between immune response traits with production and reproduction traits
A more balanced and robust immune system may be associated with decreased productivity, because a cow allocates more resources (e.g. nutrients) to the immune system. However, Thompson-Crispi et al. (Reference Thompson-Crispi, Sewalem, Miglior and Mallard2012) showed that selection on high IR improved milk yield in dairy cattle. Herizazon et al. (Reference Heriazon, Quinton, Miglior, Leslie, Sears and Mallard2013) reported only low phenotypic correlations from 0.01 to 0.18 between CMIR or AMIR with milk production traits. On a genetic scale, the correlation was 0.05 between AMIR and protein percentage, and 0.18 between AMIR and fat percentage (Heriazon et al., Reference Heriazon, Quinton, Miglior, Leslie, Sears and Mallard2013). Interestingly, the same study identified negative genetic correlations between CMIR and fat or protein percentage (−0.11 to −0.15). Samoré et al. (Reference Samoré, Rizzi, Rossoni and Banato2010) estimated positive genetic correlations between fat percentage and functional longevity. Hence, continuous breeding on effective and high AMIR reflecting best adaptation to a wide range of extracellular pathogens, positively influences longevity. Thompson-Crispi et al. (Reference Thompson-Crispi, Sewalem, Miglior and Mallard2012) reported a positive genetic correlation of 0.16 between CMIR and milk yield in first parity cows. Mazengera et al. (Reference Mazengera, Kennedy, Burnside, Wilkie and Burton1985) estimated positive genetic correlations in the range from 0.13 to 0.63 between specific IR traits (IgG2 and IgA) with 305-day milk and fat percentage, but genetic correlations between IgM with 305-day milk and fat percentage were negative (−0.83 and −0.34, respectively). A negative genetic correlation of −0.18 between IgG isotype and daily milk yield was estimated (Table 2). A complete overview including genetic correlations between IR traits and production and fertility traits is given in Table 2.
Table 2 Literature overview for genetic correlations between objectively measurable immune response traits and production traits in dairy cattleFootnote 1
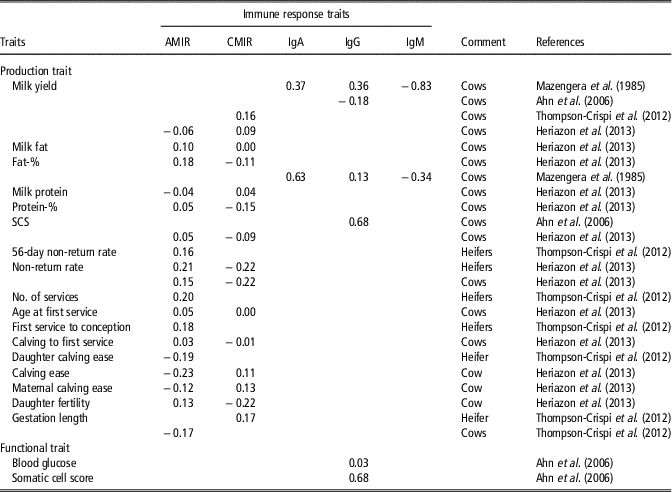
AMIR = antibody-mediated immune response; CMIR = cellular-mediated immune response; IgA = Immunoglobulin A; IgG; Immunoglobulin G; IgM = Immunoglobulin M; SCS = somatic cell score.
1 The list of references for Table 2 is given in Supplementary Material S1.
Improved IR had beneficial effects on reproductive traits (Thompson-Crispi et al., Reference Thompson-Crispi, Sewalem, Miglior and Mallard2012). Phenotypically, correlations between IR traits AMIR and CMIR with days to first service were lower than −0.11 (Heriazon et al., Reference Heriazon, Quinton, Miglior, Leslie, Sears and Mallard2013). Low, but positive genetic correlations in the range from 0.16 to 0.20 between non-return rates and IR traits (AMIR, CMIR) were estimated in Canadian heifers (Thompson-Crispi et al., Reference Thompson-Crispi, Sewalem, Miglior and Mallard2012). The genetic correlation between gestation length and AMIR was favourable (−0.17) (Thompson-Crispi et al., Reference Thompson-Crispi, Sewalem, Miglior and Mallard2012).
Relationships between immune response traits and health traits
Sires with high IR improved colostrum quality and enhanced resistance to non-infectious and infectious diseases in their daughters (e.g. Wagter et al., Reference Wagter, Mallard, Wilkie, Leslie, Boettcher and Dekkers2000). Abdel-Azim et al. (Reference Abdel-Azim, Freeman, Kehrli, Kelm, Burton, Kuck and Schnell2005) estimated correlations among predicted sire transmitting abilities for clinical disease traits and IR traits. Correlations between GI (simply defined as a combination of infectious diseases) and non-infectious diseases were lower (0.01 to 0.20) compared with correlations between GI and infectious diseases (0.56 to 0.79).
A low genetic correlation (0.03) between IgG and blood glucose was reported in Korean Holstein cows (Table 2). Blood glucose is a biomarker for non-infectious diseases or metabolic health. In contrast, the genetic correlation between IgG and SCS (biomarker for mastitis) induced by pathogens was 0.68 (Table 2). Such results indicate that resistance to infectious diseases is primarily regulated by IR genotypes, while resistance to non-infectious diseases strongly depends on environmental factors. Thompson-Crispi et al. (Reference Thompson-Crispi, Miglior and Mallard2013) compared the CM incidence rates between HR-, AR- and LR-cows for AMIR and CMIR. In this study, a significant higher incidence rate for CM induced by Staphylococcus aureus, Escherichia coli, Streptococcus spp. and further bacterial pathogens was identified in LR-AMIR cows compared to HR-AMIR cows. Kelm et al. (Reference Kelm, Dettilleux, Freeman, Kehrli, Dietz, Fox, Butler, Kasckovics and Kelley1997) estimated negative correlations between EBV for specific IR traits (e.g. Ig isotypes, neutrophil function) with EBV for SCS. Negative correlations indicate that cows with low EBV for SCS tend to have neutrophils with a greater functional ability at maximal immunosuppression. Moreover, regarding neutrophil functions, significant progeny group differences were detected (Kelm et al., Reference Kelm, Dettilleux, Freeman, Kehrli, Dietz, Fox, Butler, Kasckovics and Kelley1997). Bannerman et al. (Reference Bannerman, Springer, Paape, Kauf and Goff2008) identified breed-dependent differences for IR traits (e.g. neutrophil cell count, T lymphocytes), which are involved in host immune mechanisms against mastitis, induced by S. aureus. In this study, HF cows showed stronger neutrophil response to udder infections than Jersey cows. Banos et al. (Reference Banos, Wall, Coffey, Banall, Gillespie, Russell and McNeilly2013) identified significant variations for cellular immune traits (e.g. percentage of neutrophils or T lymphocytes in blood) in different genetic lines of Scottish HF dairy cows. Next to breed-specific variations in IR, higher variations in immunoglobulin combinatorial diversity were detected in Aubrac compared with HF or German Black Pied cattle (Walther et al., Reference Walther, Tietze, Czerny, König and Diesterbeck2016). Immunoglobulin diversity reflects the cow’s adaptation to antigens under certain environmental conditions. Thus, phenotypic differences for same genotypes in different environments regarding antibody diversity can be expected.
Conclusions
From a conventional and novel breeding perspective, improving robustness implies (i) to select animals with high and stable breeding values for production or fertility traits in the course of environmental alterations (concept of G × E interactions), (ii) to include producer-recorded health data in future breeding objectives, (iii) to focus on objectively measurable indicator traits from routine milk recording schemes and/or on novel biomarkers. Generally, as outlined in the present review, more accurate and objective phenotyping strategies allow more accurate genetic parameter estimations, and larger heritabilities. In such perspective, it is imperative to evaluate a broad pattern of biomarkers being linked to environmental response and disease resistance. A quite large number of biomarkers being linked to environmental sensitivity and disease resistance have been detected (e.g. C18:1 cis-9 MFA, BHB). Rapid progress in breeding advances for overall robustness (i.e. those animals being best adapted to environmental stressors and less susceptible to disease) suggest the inclusion of biomarkers being sensitive for more than one trait into selection indices. In dairy cattle, an obvious interaction between adaptation to changes in environment, composition of rumen microbiome, metabolism and IR mechanisms exist, affecting individual’s resistance to disease. In consequence, new approaches aiming on enhanced disease resistance focus on IR breeding strategies. Generally, genetic correlations between IR traits and performance and health traits were low to moderate, but breed-specific differences in IR reflect variability for IR traits under identical environmental conditions. Nevertheless, the optimal breeding strategy remains unclear, that is, utilization of adaptive IR, or using specific disease traits or appropriate biomarkers. Hence, it is imperative in ongoing studies to develop correct weightings and appropriate methods to accommodate all phenotypic sources simultaneously via selection index methodology.
Acknowledgements
This review is based on an invited presentation at the 68th Annual Meeting of the European Association for Animal Production held in Tallinn, Estonia, August 2017. Parts of this study were conducted in the collaborative EU-project ‘2-ORG-COWS’ and in the collaborative Research Unit FOR2432/1. Hence, the authors acknowledge the financial support for this project provided by transnational funding bodies, being partners of the FP7 ERA-net project, CORE Organic Plus, and the cofound from the European Commission, and financial support provided by the German National Science Foundation, DFG, through grant number KO 3520/5-1.
Declaration of interest
There is no potential conflicts of interest.
Ethics statement
None.
Software and data repository resources
None.
Supplementary material
To view supplementary material for this article, please visit https://doi.org/10.1017/S1751731118003208