There is accumulating evidence that diet affects the prevalence of asthma, for example via the protective effects of dietary antioxidants(Reference Shaheen, Sterne and Thompson1) and n-3 PUFA in oily fish(Reference Hodge, Salome and Peat2). Interpreting the evidence from observational nutritional studies is difficult, however, because of the wide potential for confounding and effect modification. The results of trials are often inconclusive or contradictory(Reference Devereux and Seaton3). Statistical analysis can deal with questions of confounding and interaction of dietary exposures to some extent, but the sheer number of features of diet that can be measured will often defeat comprehensive investigation. An alternative approach is to extract a small number of dietary ‘patterns’ using data-analytic methods, such as principal components analysis (PCA)(Reference Newby and Tucker4). This approach is now routinely applied to FFQ data, though applications in respiratory epidemiology have so far been scarce. Prospective cohort studies of US men and women have found that a diet rich in refined grains, cured and red meats, desserts and French fries is positively associated with risk of chronic obstructive pulmonary disease, and a diet rich in fruits, vegetables and fish is negatively associated(Reference Varraso, Fung and Hu5, Reference Varraso, Fung and Barr6). Dietary patterns were not associated with adult-onset asthma in these cohorts. A study of Chinese Singaporeans found that a diet rich in meats, Na and refined carbohydrates was positively associated with habitual cough and phlegm(Reference Butler, Koh and Lee7), while a study of Japanese female students found that a butter and rape-seed oil dietary pattern, and a fast-food, soft drink and juice dietary pattern were both positively associated with wheeze(Reference Takaoka and Norback8). In France, a study of female teachers found that a pastry, processed meat and dessert pattern was positively associated with reporting frequent asthma attacks, and a nut and wine pattern was negatively associated(Reference Varraso, Kauffmann and Leynaert9). One problem with the PCA approach is that it does not lend itself well to the synthesis of evidence from different studies. Indeed, there is no a priori guarantee that the same patterns will be seen in different countries with differing culinary traditions and sources for foods.
In the present study, we looked at dietary patterns in three countries participating in the European Community Respiratory Health Survey (ECRHS) and used these to investigate relationships between diet and asthma in adults. We derived the dietary patterns using a meta-analytic approach to PCA which, to our knowledge, has not previously been used in the analysis of FFQ data.
Material and methods
Study design and population
The design of ECRHS has been described in detail elsewhere(10). ECRHS-I ran from 1990 to 1995. At each centre, a random sample of at least 3000 adults aged 20–44 years was selected using a local sampling frame. From those who responded, a random sample of at least 600 adults was selected to undergo a detailed clinical examination. Eight to ten years later, these subjects were contacted to take part in a follow-up study (ECRHS-II) and invited to a local clinic for further assessments, including an interviewer-administered questionnaire. The sample size at each centre was sufficient for comparing changes in prevalence of wheezing, asthma and atopy between centres(10).
Dietary assessments were included in ECRHS-II at some centres, though the method and protocol differed between countries. In the present paper, we report results from five centres in three countries in ECRHS-II, where FFQ were administered: Hamburg and Erfurt in Germany; Ipswich and Norwich in the UK; Bergen in Norway. Three thousand three hundred and eighty-seven adults at these centres were contacted to take part in ECRHS-II.
Respiratory outcomes
Questions on asthma and respiratory symptoms were taken from the bronchial symptoms questions of the International Union against Tuberculosis and Lung Disease questionnaire(10). Current asthma was defined as an attack of asthma or being woken by an attack of shortness of breath in the last 12 months or currently taking any medicines for asthma. Five questions on symptoms in the last 12 months (breathless when wheezing, woken with tightness in chest, shortness of breath while at rest, shortness of breath after exercise, woken by shortness of breath) were used to construct an asthma symptom score on a five-point scale(Reference Sunyer, Pekkanen and Garcia-Esteban11).
Bronchial responsiveness (BHR) was assessed using a methacholine challenge. For safety reasons, the challenge was not carried out on participants whose forced expiratory volume in 1 s (FEV1) was < 70 % of their predicted value or < 1·5 litres. The outcome referred to here as BHR ‘slope’ is 100/(log slope+10), where log slope is calculated by regressing percentage fall in FEV1 on log10 dose(Reference Chinn, Arossa and Jarvis12). A low ‘slope’ is indicative of high BHR. To help to assess the impact of having missing slope for low FEV1 values, FEV1 was also analysed as a separate outcome.
FFQ
The German FFQ was developed for use in the German part of the European Prospective Investigation into Cancer and Nutrition (EPIC-Heidelberg)(Reference Bohlscheid-Thomas, Hoting and Boeing13). It recorded a consumption of 158 different foods over the last 12 months as frequencies from never to five portions a day or more. Portion size was selected from a multiple choice, sometimes with reference photos. Supplementary questions covered aspects of diet, such as the preparation and fat content of foods. The FFQ was distributed after the clinical and questionnaire assessments, and the participants were asked to return the completed FFQ by mail.
The UK FFQ was adapted from one developed for EPIC-UK. It recorded a consumption of 198 different foods over the last 12 months as frequencies (from never to 7 d a week) and number of portions consumed on each of these days (portions being defined on the questionnaire). As on the German FFQ, there were also supplementary questions. The Norwegian FFQ was a translation of the UK FFQ, but contained six additional foods not appearing on the UK questionnaire. In Norway, the FFQ was administered at the same clinic visit as the other assessments in ECRHS-II; while in the UK, participants were invited to attend the clinic on a separate occasion to complete the FFQ. In each case, the FFQ was self-completed and checked in the clinic by one of the local research team to cut down on missing data.
Food frequencies were converted to intakes in g/d. In the UK and Norway, this used portion weights from the standard UK reference(14), while in Germany portion sizes were those used with the EPIC FFQ(Reference Bohlscheid-Thomas, Hoting and Boeing13). For a few foods, such as butter, intake was calculated both from the reported food frequency and the supplementary questions on food preparation and cooking.
Nutrient intakes
Nutrient intakes were calculated in each country from FFQ data and supplementary questions, using local food tables(15–17). Because the Norwegian FFQ was originally translated from the UK FFQ, it contained a number of foods not commonly eaten in Norway, hence not included in the Norwegian food tables; UK references were used for these foods where they did occur.
Exclusions of dietary data
On the UK FFQ and Norwegian FFQ, respondents sometimes left individual items blank. This was assumed to denote zero intakes of these foods unless more than 20 % of items were blank, in which case the FFQ was considered incomplete, and the subject was excluded from analyses. Subjects in each country were also excluded if they had extreme values of total energy intake which might suggest an unrealistic response: we calculated expected BMR with given age, weight and sex(18), and excluded subjects with a ratio of energy intake to expected BMR below the 0·5th sample centile or above the 99·5th sample centile for their country(Reference Welch, Luben and Khaw19).
Validity and repeatability of FFQ
Validity and repeatability of the German FFQ were assessed in 104 men and women aged 35–64 years as part of a pilot for the EPIC study(Reference Bohlscheid-Thomas, Hoting and Boeing13). The FFQ was administered on two occasions in the interval of 6 months. Twelve 24-h dietary recalls applied at monthly intervals served as the reference for the validity of the second FFQ assessment.
Repeatability of the UK FFQ was assessed in eighty-two adults (sixty-six from the sample described in the present paper and sixteen others with asthma symptoms), using two assessments separated by an interval of 5–23 months. Validity of the UK FFQ was assessed in 263 adults (206 from the sample described in the present paper and 57 others with asthma symptoms), using a single 24-h dietary recall. The Norwegian FFQ was not assessed for repeatability or validity.
Validity and repeatability are summarised in Table 1.
Table 1 Energy and macronutrient intakes estimated from FFQ: estimates of repeatability and validity and observed distributions
(Median and interquartile range values)

FFQ1, 1st FFQ; FFQ2, 2nd FFQ.
* Figures are Pearson correlations, calculated from the logarithms of total energy and macronutrient intakes.
† Responders are those with complete clinical and questionnaire assessments who also completed the FFQ and had a realistic energy intake.
‡ Based on twelve 24-h dietary recalls. Multiple assessments mean that the estimate of validity can allow for within-person variation in 24-h dietary recall.
§ Based on a single 24-h dietary recall.
Dietary patterns
Some aggregation of food items on each country's FFQ was necessary to allow intakes to be matched across questionnaires, for example, on the UK FFQ and Norwegian FFQ fried egg was aggregated with omelette or scrambled egg to allow comparison with the German FFQ, which simply recorded intake of fried egg, omelette or scrambled egg. We performed the minimum aggregation necessary to allow foods to be matched. This process led to a list of seventy-four foods or food groups whose intake in g/d was available in all three countries (see the Appendix). Aggregation of food items into broader, a priori groupings (often fewer than we have used) is commonplace in the analysis of FFQ(Reference Varraso, Fung and Hu5, Reference Varraso, Fung and Barr6, Reference McCann, Marshall and Brasure20).
In each centre, we evaluated the correlation matrix of foods on our list and pooled the correlations from different centres using the method of Rosenthal(Reference Rosenthal21). Specifically, each correlation coefficient was transformed using a Fisher transformation (0·5 log((1+r)/(1 − r))) to give it an approximately normal sampling distribution with variance 1/(n − 3), where n is the sample size for that centre. A weighted average of these values was then calculated, in which each value was given a weight proportional to the inverse of its variance (analogous to pooling estimates of a mean, say, from subsamples of different sizes). An inverse Fisher transformation was then applied to give a pooled correlation coefficient. PCA was applied to the matrix of pooled correlation coefficients, giving us dietary patterns (linear combinations of standardised food intakes), which could be used in all the five centres. This meta-analytic approach to PCA has previously been applied in the field of psychiatry(Reference Smith, Mar and Turoff22, Reference Grube, Bilder and Goldman23).
Data analysis
We looked at whether characteristics of participants influenced rates of responding to the FFQ using logistic regression, with responding as the outcome, adjusting for centre. Social class was based on occupation using International Standard Classification of Occupations-88 codes(24). Subjects were categorised as never, ex- or current smokers based on questionnaire responses and were divided into four groups according to reported frequency of physical exercise (‘How often do you usually exercise so much that you get out of breath or sweat?’): never; less than once a week; one to three times a week; or more than three times a week.
We used multivariable regression to investigate associations between the dietary patterns (in quintile groups) and respiratory outcomes at each centre. Logistic regression was used for asthma, negative binomial regression for symptom score and linear regression for BHR slope and FEV1. Analyses were adjusted for age, sex, social class, smoking status, exercise, BMI and quintiles of total energy intake. The effects of the dietary patterns were also adjusted for each other, because although principal components are uncorrelated, rotations (even orthogonal ones) can introduce correlations between the dietary patterns.
Regression results were pooled across centres using random effects meta-analysis, with a test for heterogeneity of regression coefficients(Reference DerSimonian and Laird25). Heterogeneity was summarised using the I 2 statistic(Reference Higgins, Thompson and Deeks26).
Statistical software
Some analyses of the German data were carried out locally using SAS (SAS Institute, Cary, NC, USA). All other analyses were done with Stata 10 (Stata Corporation, College Station, TX, USA).
The present study was conducted according to the guidelines laid down in the Declaration of Helsinki and all procedures involving human participants were approved by the Bavarian General Medical Council in Germany, the Ipswich Hospital and Norfolk and Norwich Hospital ethics committees in the UK and the Regional Committee of Medical Research Ethics at the University of Bergen in Norway. Written informed consent was obtained from all the participants.
Results
Fig. 1 shows the numbers of people taking part, by centre. Clinical and questionnaire assessments were available for 1740 subjects. Complete FFQ data were provided by 1182 of these, but eight were excluded because they were at the extremes of the distribution of ratio of energy intake to expected BMR in their country (in Germany, there was one under-reporter (ratio < 0·59) and one over-reporter (>5·5), in the UK one under-reporter ( < 0·44) and one over-reporter(>3·6), and in Norway two under-reporter ( < 0·50) and two over-reporter (>5·2)). The 1174 people who responded to the FFQ with a realistic energy intake (35 % of those contacted) ranged in age from 29 to 55 years. Those in a higher social class were more likely to be responders (P < 0·001), as were people who exercised more frequently (P = 0·012). Current smokers were less likely than ex- or never-smokers to respond (P = 0·001). There was no evidence that age, sex or BMI were associated with responding. Of the 1174 responders, reported asthma was available for 1173, asthma score for 1160 and BHR slope for 906. Table 1 summarises total energy and macro-nutrient intakes in responders.
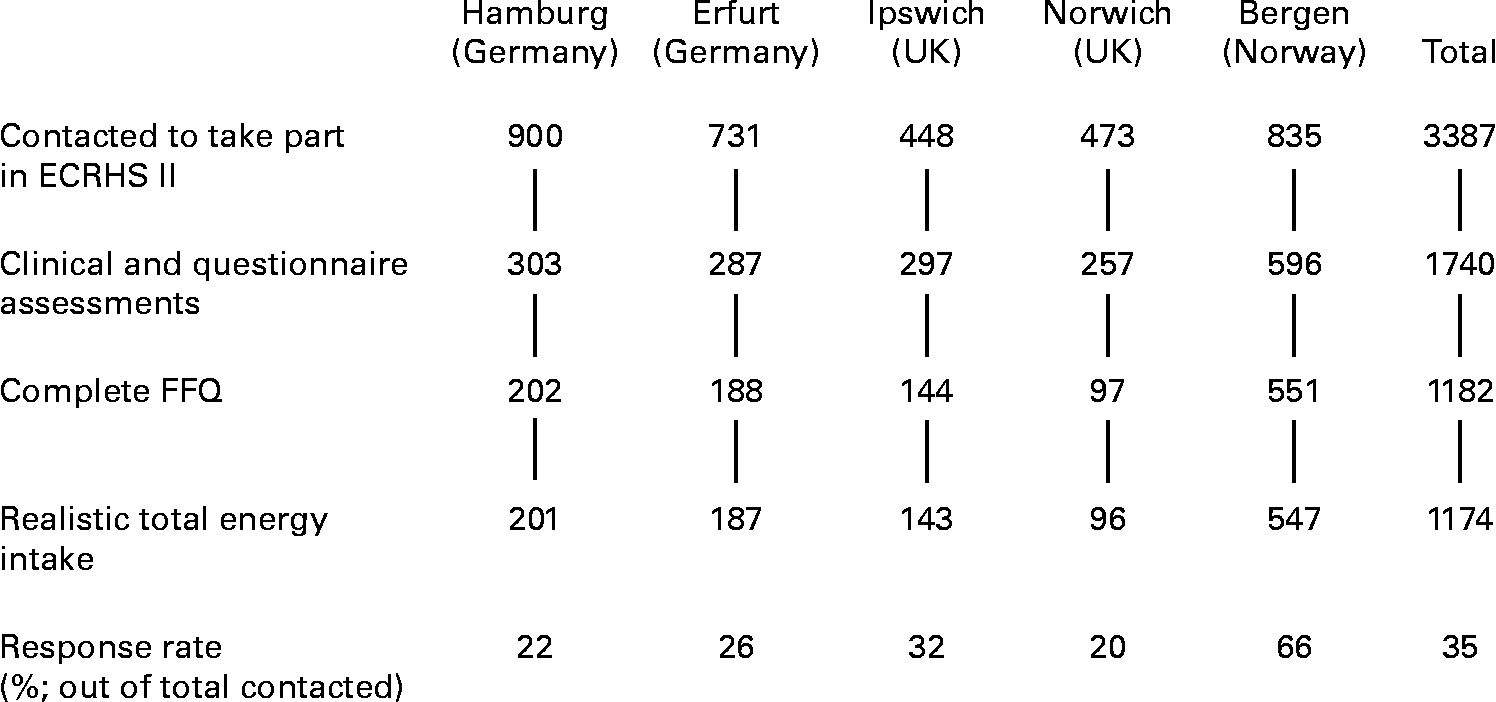
Fig. 1 Flow chart showing numbers of subjects in the study. ECRHS, European Community Respiratory Health Survey.
Principal components analysis
The scree plot from the PCA showed a clear break in the curve after two components, with no natural choice of any larger but still parsimonious number of components to extract. These two dietary patterns explained 11·2 % of the variance in the FFQ data. Table 2 shows how individual foods were correlated with each of these patterns at the five centres. Although a pattern score is defined as the same weighted sum of standardised food intakes at each centre, correlations with individual foods may differ between the centres because of differences in local diets, and this table is a good way to judge the heterogeneity or homogeneity of dietary patterns. In our case, there was a close match between the centres in the foods which characterised each pattern. The first pattern was closely associated with sliced meat, beef, pork, bacon, sausage and fried egg/scrambled egg/omelette intake at all the centres and also with intake of potato or chips. Depending on the centre, it also correlated closely with bread, butter, biscuits and cakes. This pattern is referred to here, for conciseness, as the ‘meat and potato’ pattern. The second pattern was closely associated with intakes of several fruits at all the centres and less consistently with intakes of a number of vegetables and fish. This pattern is referred to here as the ‘fish, fruits and vegetables’ pattern.
Table 2 How correlates of dietary patterns vary between centres*

G1, Hamburg, Germany; G2, Erfurt, Germany; UK1, Ipswich, UK; UK2, Norwich, UK; N, Bergen, Norway.
* Values are Pearson correlation coefficients. For clarity, only values>0·30 or < − 0·30 are shown.
† Foods made with soya protein: soya cheese; tofu; textured vegetable protein.
Dietary patterns and respiratory outcomes
Fig. 2 shows the results of meta-analyses as forest plots. There was no overall evidence that the meat and potato pattern was associated with asthma (P = 0·90), symptom score (P = 0·58), BHR slope (P = 0·39) or FEV1 (P = 0·74), and similarly no evidence that the fish, fruits and vegetables pattern was associated with asthma (P = 0·26), symptom score (P = 0·16), BHR slope (P = 0·89) or FEV1 (P = 0·19).
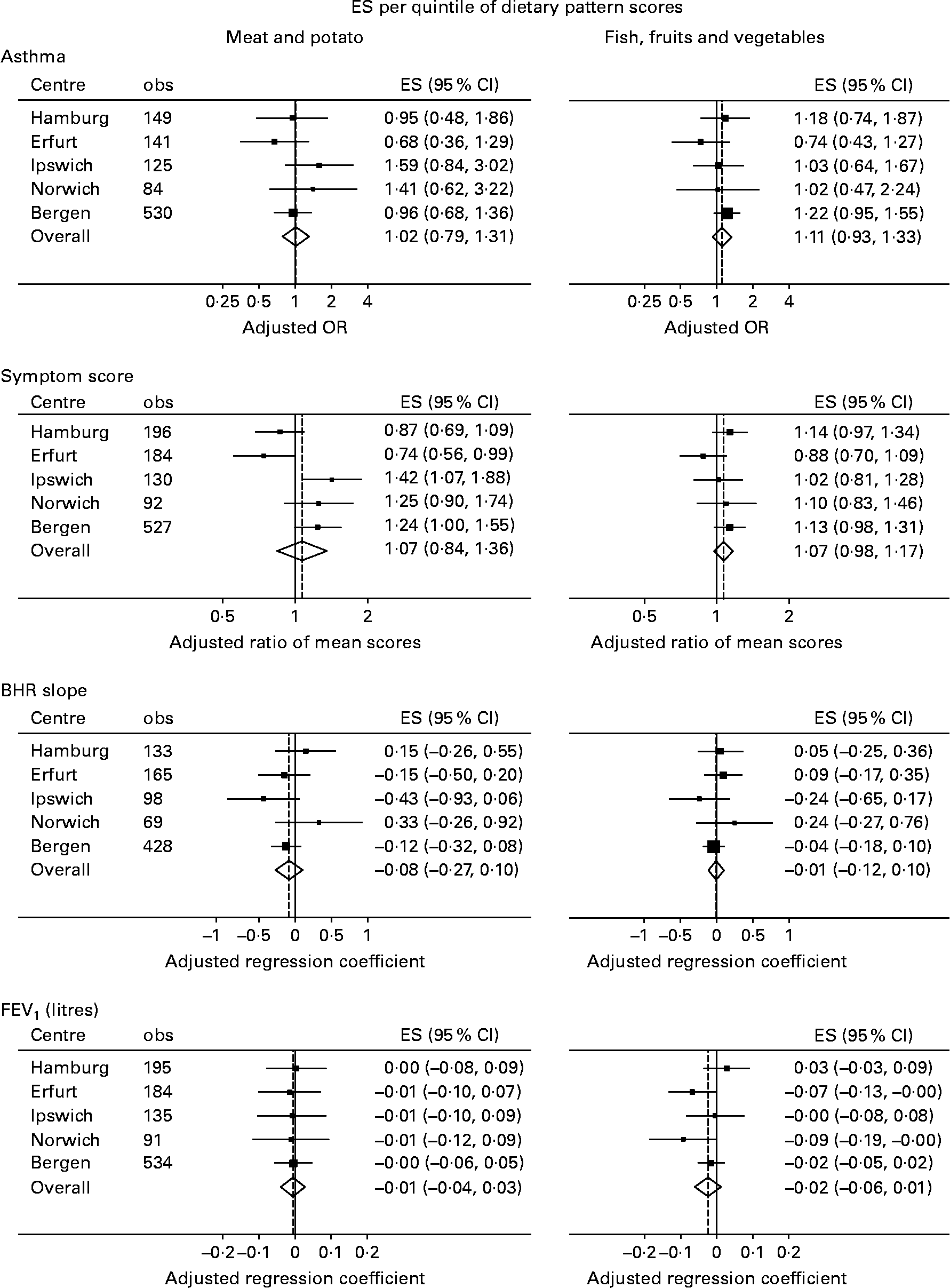
Fig. 2 Associations between the two dietary patterns and respiratory outcomes: results of meta-analyses. ES, effect size; BHR, bronchial responsiveness; FEV1, forced expiratory volume in 1 s.
There was no evidence of heterogeneity across the centres in any of these effects, except in the case of the association between meat and potato pattern score and symptom score (I 2 = 75 %; P = 0·003). In this case, it was evident from a visual inspection that the two German centres had qualitatively different results to the others; in fact, when countries were (meta-) analysed separately, increased meat and potato intake was associated with a decrease in symptoms in Germany (ratio of mean number of symptoms per quintile = 0·81; 95 % CI 0·68, 0·97; P = 0·025) but with an increase in symptoms in the UK (ratio per quintile = 1·34; 95 % CI 1·09, 1·67; P = 0·007) and Norway (ratio per quintile = 1·24; 95 % CI 1·00, 1·55; P = 0·051).
Discussion
We could not confirm any harmful or beneficial effects of fish, fruits and vegetable intake on asthma, though CI ruled out a reduction in the odds of current asthma of more than 7 % per quintile of fish, fruits and vegetable consumption or a reduction in the mean number of asthma symptoms of more than 2 % per quintile. Fish, fruits and vegetables are essential components of a Mediterranean diet, which other recent work has found to be associated with improved asthma control in adults(Reference Barros, Moreira and Fonseca27), lower risk of current severe asthma in girls aged 6–7 years(Reference Garcia-Marcos, Canflanca and Garrido28) and lower risk of allergic rhinitis in children aged 7–18 years(Reference Chatzi, Apostolaki and Bibakis29). Fish in the diet is a source of n-3 fatty acids, which may compete with the pro-inflammatory properties of n-6 fatty acids(Reference Black and Sharpe30, Reference Sausenthaler, Koletzko and Heinrich31). Recent results from the Respiratory Health in Northern Europe study indicated that infrequent fish intake was associated with increased asthma symptoms(Reference Laerum, Wentzel-Larsen and Gulsvik32), but experimental support for this as a major influence on clinical disease is still weak(Reference Thien, Woods and De Luca33). Dietary antioxidants, of which fruits and vegetables should be a rich source, have also been hypothesised to be important in protecting against atopic disease(Reference Seaton, Godden and Brown34). Vitamin E, in particular, has been associated in some studies with a reduction in atopy(Reference Devereux and Seaton3), though a trial of vitamin E supplementation in asthma failed to show an effect(Reference Pearson, Lewis and Britton35).
The heterogeneous effect of the meat and potato pattern is not easy to explain. Patterns similar to this have often been labelled ‘western’ and are likely to represent a mixture of dietary components which may independently contribute to asthma risk(Reference Varraso, Fung and Hu5, Reference Varraso, Fung and Barr6). Heterogeneity in multi-centre studies can also suggest alternative explanations for apparent effects of diet observed in single centres, such as uncontrolled confounding, and would make us cautious of progressing to a trial(Reference Burney, Potts and Makowska36). Our study was cross-sectional, and a further possibility is reverse causation, people with asthma may alter their diet in a systematic way at a given centre, for example, to be more ‘healthy’.
Note that our analysis gives us common dietary patterns (weighted sums of standardised food intakes) that we can investigate at every centre. However, if a dietary pattern is acting as a proxy for individual foods associated with asthma, then some heterogeneity in its effect might be due to heterogeneity in its associations with these foods. The greatest variation in the correlations of individual foods with the meat and potato pattern was for beef burger (high in Hamburg, low in Bergen), bacon (high in Norwich, low in Erfurt) and poultry (high in Hamburg, low in Norwich).
FFQ are widely used in epidemiological studies to investigate dietary intake of individuals and its association with diseases. FFQ are cost-effective tools to assess the usual current and past patterns of food intake over an extended period of time(Reference Cade, Burley and Warm37). Estimates of the validity of our FFQ data, for Germany at least, were good, being in the same range as those reviewed by Willett(Reference Willett38). In the UK, validity was based on just a single 24-h dietary recall, but correlations were still notably high at 0·25–0·31, and we might reasonably expect that with multiple 24-h dietary recalls and adjustment for within-person variability, we would have observed correlations as good as those seen in Germany. ‘True’ validity may, in general, be worse than implied by studies using 24-h recall, because of correlated errors. This was the case for the EPIC-Norfolk FFQ, from which our FFQ was derived, for which estimates of Na and nitrogen intake correlated poorly with repeated 24-h urine measurements(Reference Day, McKeown and Wong39). In Norway, we used a translation of the UK FFQ, but this included all the foods from the Norwegian EPIC FFQ(Reference Engeset, Alsaker and Ciampi40, 41), so we believe it is unlikely to have missed much in the Norwegian diet (we note that the total energy intake calculated from the FFQ in Norway was, if anything, higher than in the UK (Table 1)). Using a direct translation of the UK FFQ had the great advantage of simplifying the pooling of data from Norway and the UK.
The poor response rates in our study were partly due to the time that had expired between the end of ECRHS-I and the recruitment of cohort members into ECRHS-II. Differences between response rates in different countries were also partly explained by differences in procedures. FFQ assessments in the UK were implemented much later than other assessments because the protocol had to be developed and approved separately, and the interval between the two clinic visits was up to 23 months (inter-quartile range 10–16 months) helping to explain the low rate of FFQ completion. In Norway, assessments were done in a single clinic visit. In Germany, participants were asked to complete the FFQ at home and return it by mail to reduce the burden placed on them in attending the clinic.
Methods for identifying dietary patterns, such as PCA, have proved a popular way to explore complex diet data in the last decade(Reference Newby and Tucker4). A meta-analytic approach to deriving dietary patterns across a number of centres has not, to our knowledge, been investigated before. This is, no doubt, partly because FFQ are often specific to a site, making it difficult to pool data. More work is needed to develop FFQ that allow directly comparable data to be collected in different countries. We have shown that the method can be successful in identifying common dietary patterns, as well as evidence for heterogeneity in the effects of those patterns. Heterogeneity in observational studies of diet can sometimes argue against progressing to trials.
In conclusion, we found no firm, consistent evidence for an association of diet with asthma, though CI appeared to rule out large beneficial effects of fish, fruits and vegetable consumption on current asthma or asthma symptoms. However, there are likely to be important, unmeasured confounders associated with dietary choices and observational studies of diet, particularly if they are cross-sectional, must be interpreted with caution.
Acknowledgements
The co-ordination of ECRHS-II was funded by the European Union (grant number FP5-QLK4-CT-1999-01 237). The fieldwork in the UK was supported by a grant from Asthma UK (formerly known as the National Asthma Campaign); that in Germany by Deutsche Forschungsgemeinschaft; that in Norway by the Norwegian Research Council, the Norwegian Asthma and Allergy Association, GlaxoWellcome AS and the Norway Research Fund. R. H. and V. G.-L. are supported by the Department of Health, UK (grant number DHTBX-P05909). In addition, we acknowledge the contribution of the ECRHS-II steering committee, without whom the study would not be possible: Ursula Ackermann-Liebrich; Josep Antó; P. B.; Isa Cerveri; Sue Chinn; Roberto de Marco; Thorarinn Gislason; J. H.; Christer Janson; Deborah Jarvis; Jill Knox; Nino Künzli; Bénédicte Leyneart; Christina Luczynska; Françoise Neukirch; Jan Schouten; Jordi Sunyer; Cecilie Svanes; Paul Vermeire; Matthias Wjst. Contributions of the co-authors of this manuscript were as follows: J. H., E. O., S. S. and P. B. were involved in acquiring and managing the data; R. H. and I. B. conducted the data analysis; S. S. and V. G.-L. advised on nutritional analyses; R. H. wrote the first draft, and all the authors contributed to the final version. None of the authors has any conflicts of interest (personal, commercial, political, academic or financial).
Appendix: Food items in each of the food groups analysed
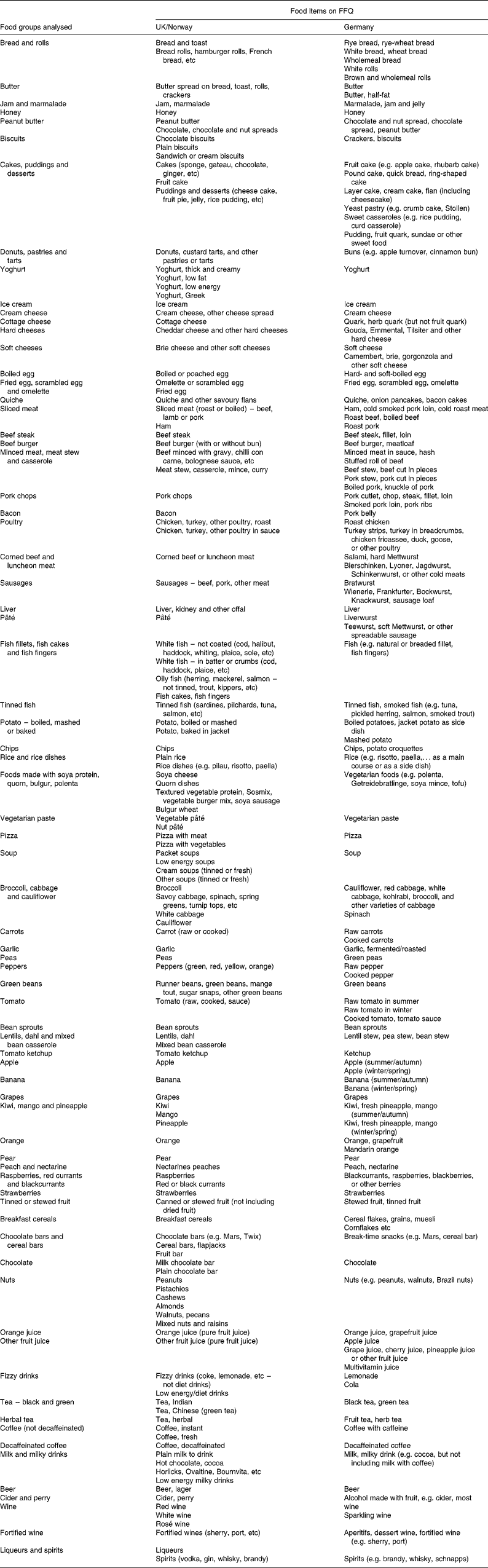