The beginning of this century was marked by the introduction of the Bologna reforms (Adelman Reference Adelman2009). In this context, ‘Diplom’ degree courses were restructured into bachelor’s and master’s degree courses. With this reform, the European Union (EU) aimed to make its higher education system more competitive compared with the well-established Anglo-Saxon system and to simplify cross-national transitions within the EU (European Commission/EACEA/Eurydice 2018). The uniform system was meant to contribute to more transparency and flexibility for students across the EU and other countries and thus to the globalization of the EU higher education space and greater student mobility. Expanded student exchange programmes as well as increasing migration (including refugee migration) have contributed both to higher numbers of prospective students in the EU as well as to an extreme heterogeneity when it comes to the students’ preconditions when entering university (Zlatkin-Troitschanskaia et al. Reference Zlatkin-Troitschanskaia, Happ, Nell-Müller, Deribo, Reinhardt and Toepper2018a; OECD 2018).
In the past decade, higher education stakeholders as well as practitioners have increasingly criticized that the Bologna reforms have hardly contributed to the transparency and comparability of degrees (Söll Reference Söll2017; Crosier and Parveva Reference Crosier and Parveva2013). On the contrary, degrees and grades are hardly comparable both nationally and internationally. Studies by the Organization for Economic Co-Operation and Development (OECD) assessing the knowledge and skills of students in different countries (e.g., AHELO, OECD 2012, 2013a, 2013b) confirm the observed deficits. Particularly in mass study programmes such as Business and Economics (B&E), practitioners criticize the extreme heterogeneity of students’ preconditions upon entering higher education (Klaus et al. Reference Klaus, Rauch, Winkler and Zlatkin-Troitschanskaia2016). Their lack of previous knowledge relevant to the respective degree courses and generic skills such as language skills and self-regulation are considered a particular challenge (Zlatkin-Troitschanskaia et al. Reference Zlatkin-Troitschanskaia, Pant, Lautenbach, Molerov, Toepper and Brückner2017; Berthold et al. Reference Berthold, Leichsenring, Kirst and Voegelin2009; KMK 2009). As a consequence, many degree programmes are characterized by extremely high dropout rates (Heublein et al. Reference Heublein, Hutzsch, Schreiber, Sommer and Besuch2010, Heublein Reference Heublein2014; Federal Statistical Office 2017; OECD 2010, 2018).
Due to the practical challenges, there have been several efforts in the past decade to develop instruments for the valid and reliable assessment of the entry requirements of students (for an overview, see Zlatkin-Troitschanskaia et al. Reference Zlatkin-Troitschanskaia, Pant, Lautenbach, Molerov, Toepper and Brückner2017; Zlatkin-Troitschanskaia et al. Reference Zlatkin-Troitschanskaia, Shavelson, Pant, Secolsky and Denison2018b). Additionally, numerous support programmes for beginning students have been implemented at German universities (Klaus et al. Reference Klaus, Rauch, Winkler and Zlatkin-Troitschanskaia2016). However, both practical experience and numerous studies show that such programmes have not been sufficiently effective so far and that a stronger internal differentiation with regard to the individual needs of the students is necessary (Zlatkin-Troitschanskaia et al. Reference Zlatkin-Troitschanskaia, Pant, Lautenbach, Molerov, Toepper and Brückner2017; Jaeger et al. Reference Jaeger, Woisch, Hauschildt and Ortenburger2014). An elaborate diagnosis of learning preconditions at the beginning of a course of study is a necessary prerequisite for providing effective and individualized offers to the students on this basis (Hell et al. Reference Hell, Linsner, Kurz and Rentschler2008; Heinze Reference Heinze2018).
The approach of Goldman and Pellegrino (Reference Goldman and Pellegrino2015) describes the connected Triad of Curriculum-Instruction-Assessment and states that curricular and instructional measures are to be developed on the basis of valid assessments that reliably measure study results (in the form of student learning outcomes), and curricula and instruction should then be aligned accordingly. However, evidence suggests that this alignment is currently deficient in educational practice. A particular challenge in higher education as described by Shepard and colleagues (Reference Shepard, Penuel and Pellegrino2018) is that the programme designs, instructional activities, and assessment strategies commonly used in higher education do not lead to the desired student learning outcomes. This was one of the focal points of the Germany-wide large-scale research program Modelling and Measuring Competencies in Higher Education (KoKoHs), which was established by the German Federal Ministry of Education and Research in 2011 (for an overview, see Zlatkin-Troitschanskaia et al. Reference Zlatkin-Troitschanskaia, Pant, Lautenbach, Molerov, Toepper and Brückner2017; Zlatkin-Troitschanskaia et al. Reference Zlatkin-Troitschanskaia, Pant, Toepper and Lautenbach2020). In this research programme, the focus has been on the challenge of developing valid assessments for student learning outcomes for the past 10 years, and on the following superordinate research questions (RQs):
(1) What levels of study-related knowledge and skills do students have when they enter higher education? (RQ 1)
(2) How do a student’s domain-specific knowledge and skills change over the course of studies? (RQ 2)
(3) Which factors influence the students’ learning and their competence development in higher education? (RQ 3)
In this contribution, an overview of the projects and research work conducted in the KoKoHs programme will be presented with a particular focus on the study entry diagnostics and therefore on RQ 1. In addition, we present findings regarding RQ 2, which also show to what extent the acquisition of knowledge and skills over the first year of study is influenced by students’ preconditions upon entry into higher education (RQ 3). The presented findings from the Germany-wide entry diagnostics provide important evidence-based insights into students’ preconditions and lead to far-reaching practical implications for successful transitions between secondary and tertiary education. This includes recommendations for the development of mechanisms to support access to tertiary education and to prevent high dropout rates.
Entry Assessments in Higher Education in the Context of the KoKoHs Programme
To draw conclusions about the development of students’ competencies over the course of higher education studies, their level of study-related knowledge and skills must be validly assessed upon entry into higher education. Many national and international studies have shown that grades and degrees in secondary school education do not provide valid and reliable information about the knowledge and skills students actually acquire (e.g. OECD 2012, 2013a, 2013b, 2016). As a consequence, stakeholders in higher education do not have sufficient, reliable information about the students’ study-related preconditions (Berthold et al. Reference Berthold, Leichsenring, Kirst and Voegelin2009; KMK 2009). To allow for a valid and reliable measurement of students’ competencies and skills from the beginning to the end of their studies, theoretical–conceptual models and corresponding test instruments are necessary to describe the knowledge and skills of students with adequate precision and complexity, and to measure them reliably and validly (Mislevy Reference Mislevy2018).
For this reason, in 2011, the German Federal Ministry of Education and Research launched a new KoKoHs research programme to develop, validate, and test newly developed models and instruments in practice. The KoKoHs projects follow an evidence-based assessment design (Riconscente et al. Reference Riconscente, Mislevy, Corrigan, Lane, Raymond and Haladyna2015; Mislevy Reference Mislevy2018). In the first phase of the programme, which ran from 2011 to 2015, models to describe and operationalize these competencies were developed. The modelling included the (e.g. multidimensional, content-related, cognitive) structure, taxonomy (e.g. difficulty levels), and development of (generic or domain-specific) competencies (for examples, see Kuhn et al. Reference Kuhn, Alonzo and Zlatkin-Troitschanskaia2016; Förster et al. Reference Förster, Brückner and Zlatkin-Troitschanskaia2015a) that
(1) are based on cognitive psychology, teaching–learning theory, domain-specificity and didactics, and
(2) serve as a basis for the development of suitable measurement instruments for valid competency assessment.
Based on these elaborated competency models, the KoKoHs projects then developed corresponding test instruments for the valid and reliable assessment of different facets and levels of students’ competencies and tested them comprehensively using suitable psychometric models (for examples, see Zlatkin-Troitschanskaia et al. Reference Zlatkin-Troitschanskaia, Pant, Lautenbach, Molerov, Toepper and Brückner2017, Reference Zlatkin-Troitschanskaia, Pant, Toepper and Lautenbach2020).
In summary, in the 85 subordinate KoKoHs projects, more than 80 theoretical competency models were developed to describe student learning preconditions and outcomes, which were then validated comprehensively in accordance with the standards for psychological and educational testing (AERA, APA and NCME 2014). All of these different models follow the assumption that students’ competencies are complex, multifaceted constructs (for a definition, see Weinert Reference Weinert, Rychen and Salganik2001). Depending on which competence facets and levels the individual KoKoHs projects focused on, for example, domain-specific knowledge or generic skills of beginning or advanced students, different appropriate measurement approaches were developed and tested in practice.
Following the model developed by Blömeke et al. (Reference Blömeke, Gustafsson and Shavelson2015), students’ competencies can be described across the continuum: dispositions – skills – (observed) performance. Cognitive or affective-motivational dispositions (e.g. previous domain-specific knowledge or academic self-efficiency), which are considered the latent traits of students, are often measured using multiple-choice tasks and items or self-report scales and are modelled in accordance with Item Response Theory. Situation-specific skills (e.g. teachers’ instructional skills) are typically measured using a situative approach (social-contextual roots) and, for instance, problem-based scenarios (e.g. assessments with video vignettes; see, for example, Kuhn et al. Reference Kuhn, Zlatkin-Troitschanskaia, Brückner and Saas2018). Finally, performance is usually assessed using concrete, realistic, hands-on tasks, sampled from criterion-related situations and analysed using holistic approaches such as generalizability theory models (e.g. Shavelson et al. Reference Shavelson, Zlatkin-Troitschanskaia, Beck, Schmidt and Marino2019).
In general, all KoKoHs projects follow the Evidence-Centred Model for Instantiating Assessment Arguments by Riconscente and colleagues (Reference Riconscente, Mislevy, Corrigan, Lane, Raymond and Haladyna2015). According to this model, a valid assessment allows for a psychometric connection between the construct that is to be measured (e.g. previous knowledge) and the developed tasks (e.g. subject-specific multiple-choice items). When assessing constructs such as students’ competencies, the focus lies on the question of which capabilities the KoKoHs projects want to assess. Developing an appropriate task follows the question: in what kinds of situations a person would demonstrate these capabilities. Each KoKoHs project focuses on a different construct, i.e. a different facet of student competencies and learning outcomes (e.g. domain-specific problem-solving, self-regulation, etc.) and develops tasks that are suitable for the valid and reliable assessment of this facet (for an overview, see Zlatkin-Troitschanskaia et al. Reference Zlatkin-Troitschanskaia, Pant, Lautenbach, Molerov, Toepper and Brückner2017, Reference Zlatkin-Troitschanskaia, Pant, Toepper and Lautenbach2020). The instruments developed in KoKoHs are supposed to assess various competence facets at different levels, including domain-specific and generic knowledge and skills as well as student performance, for example, during teaching internships. In total, more than 200 test instruments of different assessment formats (e.g. paper–pencil, computer-based) were developed, and a few already available international tests were adapted for use in Germany.
Additionally, some of the test instruments have already been translated and adapted for use in other countries (more than 20 countries across four continents) and have already been used in many international studies (e.g. Yang et al. Reference Yang, Kaiser, König and Blömeke2019; Brückner et al. Reference Brückner, Förster, Zlatkin-Troitschanskaia, Happ, Walstad, Yamaoka and Tadayoshi2015; Krüger et al. Reference Krüger, Hartmann, Nordmeier, Upmeier zu Belzen, Zlatkin-Troitschanskaia, Pant, Toepper and Lautenbach2020).
Even though the KoKoHs research programme is a large-scale collaboration, it was not possible to develop instruments for all of the various domains and disciplines in higher education. Therefore, the KoKoHs projects focused on four popular study domains and one domain-independent cluster: B&E, medicine, sociology, teacher education, and interdisciplinary generic skills. Overall, the teams of the KoKoHs projects assessed more than 85,000 students at over 350 higher education institutions across Germany; approximately one third of the test takers were beginning students in the study domains of business, economic and social sciences (Zlatkin-Troitschanskaia et al. Reference Zlatkin-Troitschanskaia, Pant, Toepper and Lautenbach2020).
Study Entry Diagnostics
An Example from the Study Domains of Economics and Social Sciences
To demonstrate the potentials of entrance testing of student learning preconditions in higher education, the RQs are examined on the basis of one of the KoKoHs projects: the WiWiKom II study (for an overview of all assessments conducted in this project, see Zlatkin-Troitschanskaia et al. Reference Zlatkin-Troitschanskaia, Jitomirski, Happ, Molerov, Schlax, Kühling-Thees, Pant, Förster and Brückner2019a). In this Germany-wide longitudinal study (after the pre-tests), a test to assess previous subject-related knowledge in economics and social sciences was used at the beginning of the winter term 2016/2017 (T1) at more than 50 universities across Germany, assessing more than 9000 students of B&E (N = 7679) and social sciences (N = 1347). The assessment took place during preparatory courses or introductory lectures in the first weeks of the term. The sample is on average 20.53 (± 2.753) years old, the gender distribution is approximately equal (52.28% male), about 30% have at least one parent who was born in a country other than Germany, and only 3.19% stated that they prefer a communication language other than German. The average school leaving grade was 2.35 (± 0.577). About one third of the sample had attended an advanced course in B&E at school. Exchange students were excluded because of deficient comparability in terms of education background.
The students were surveyed using a paper–pencil test of approximately 30 minutes, which comprised not only questions about sociodemographic and study-related variables but also a validated domain-specific knowledge test consisting of 15 items on (socio-)economic basic knowledge (adapted from the fourth version of the Test of Economic literacy, TEL IV; Walstad et al. Reference Walstad, Rebeck and Butters2013; for the adaptation process, see Förster et al. Reference Förster, Zlatkin-Troitschanskaia and Happ2015b) and ten items on deeper economic content (adapted from the fourth version of the Test of Understanding in College Economics, TUCE IV; Walstad et al. Reference Walstad, Watts and Rebeck2007; for the adaptation process, see Zlatkin-Troitschanskaia et al. Reference Zlatkin-Troitschanskaia, Förster, Brückner, Happ and Coates2014) as well as a scale of fluid intelligence (extracted from the Berlin test of fluid and crystallized intelligence for grades 11+, BEFKI; Schipolowski et al. Reference Schipolowski, Wilhelm and Schroeders2017). Missing answers in the knowledge test were interpreted as a lack of knowledge and coded as wrong answers, with more than 12 missing answers being assumed to indicate insufficient test motivation. The latter were not included in the analyses.
At the second measurement point (T2), one year later, 1867 students of B&E and 356 students of social sciences at 27 universities were assessed using the domain-specific knowledge test in courses that are scheduled in the curricula for third-semester students. Seven-hundred and fourteen of the students of business and economics and 155 of the social sciences students could be identified via a pseudonymized panel code as repeatedly assessed in both measurements (T1 and T2).
The following results are based on analyses using Stata 15 (StataCorp 2017). The metric level of measurement invariance (χ 2(1169) = 1331.98, p = 0.0006; RMSEA = 0.014, WRMR = 0.998, CFI = 0.976, TLI = 0.975) was investigated using MPlus (Muthén and Muthén Reference Muthén and Muthén1998–2011).
Results
With regard to the level of study-related knowledge at the beginning of a bachelor’s degree course (RQ 1), only the first-semester students were examined (N = 7986, N B&E = 6870, N S = 1116). On average, the students solved 13.35 of 25 tasks (SD = 4.329) correctly, which showed a middle level of prior knowledge at the beginning of their bachelor’s studies. Out of the basic knowledge questions (from the TEL), an average of 9.21 items (SD = 2.992; 61.40%) were solved correctly, whereas out of the TUCE items, an average of 4.08 items (SD = 1.975; 40.8%) were solved correctly (after metric adjustments, t(7985) = 96.492, p>0.001). The results did not differ significantly between the study courses (e.g. for overall sum score: t(7927) = –1.016, p = 0.310, see Figure 1), so that a significant pre-selection effect cannot be assumed. However, this means that a significant part of the beginning students does not have sufficient previous knowledge of (socio-)economics fundamentals; 26.80% of the beginning students solved exactly or fewer than 10 tasks (40% of the test) correctly overall (for histogram, see Figure 2).
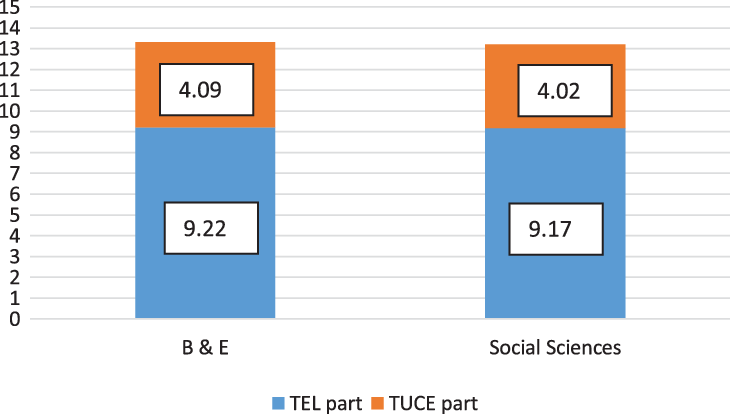
Figure 1. Average results from entry diagnostics.
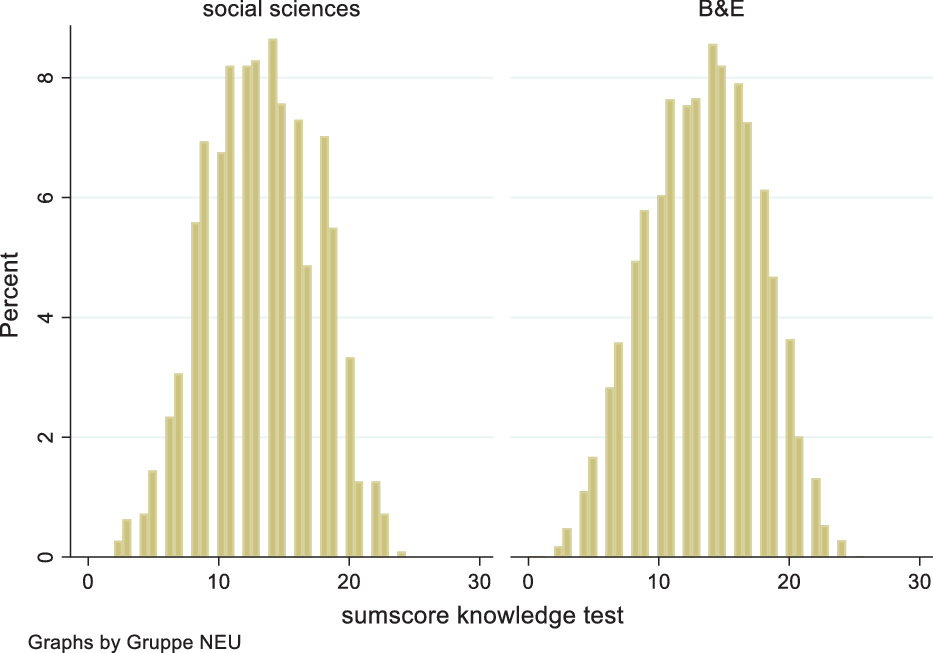
Figure 2. Histogram of sum score.
Regarding RQ 2, in a first step, we analysed the students’ level of domain-specific knowledge at the end of their first year of study. Therefore, we only considered students in their third semester (N overall = 1956, N B&E = 1646, N S = 310). The participants of the cross-section after one academic year solved an average of 13.81 (SD = 4.93) items correctly, i.e. 9.38 (SD = 3.175, 62.53%) items on the TEL part and 4.41 (SD = 2.221, 44.1%) items on the TUCE part. While the degree courses B&E and social sciences do not differ in the overall sum score (t(1949) = 0.031, p = 0.975) and not with regard to the basic content TEL part (t(1954) = 1.225, p = 0.221), the students of B&E in the third-semester cross-section show significantly better values with regard to the more in-depth contents in the TUCE part (t(1954) = –1.989, p = 0.05). This finding was in line with our expectations due to differences in the curricula for the first year of study in economics and social sciences studies.
To draw conclusions about the development of knowledge, we analysed the longitudinal sample. To examine how economics knowledge changes over the course of the first year of studies, we matched the two samples T1 and T2. Based on this matched subsample, a significant but very slight change after the first year of studies was identified. On closer examination, the significant change relates primarily to the TUCE part of the assessment. As expected, this development pattern is only evident in the group of B&E students, and not in the group of social sciences students (Table 1 and Figure 3).
Table 1. Changes in knowledge scores in the longitudinal sample.


Figure 3. Average change in sum scores between T1 and T2.
Finally, when focusing on RQ 3, we investigate the influencing factors on the knowledge level and its development. In particular, we explore whether there are any differences in economics knowledge at the beginning and at the end of the first year of studies that may be influenced by factors related to secondary school. In this study with a focus on the transition between secondary to tertiary education, indicators of the students’ secondary education, such as their school leaving grade were included. In addition, it was taken into account whether or not the students had attended advanced B&E courses in secondary school. Regression analyses show that these two indicators have a significant influence on the level of economics knowledge that beginning students have in higher education (T1, see Table 2, multi-level modelling (MLM) due to a multi-level structure regardless of a low ICC of 0.047). This applies both to the group as a whole (as illustrated in Table 2) and to the subgroups of B&E students and social sciences students.
Table 2. MLM with knowledge test score at T2 as dependent variable (group variable: 54 universities).
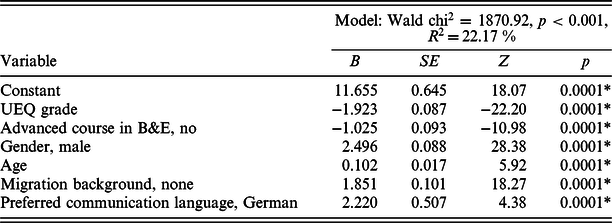
Notes. N = 7,692; UEQ = university entrance qualification; in Germany, lower grades indicate better performance, * indicates significance on a 5% level.
When analysing what influences changes in the level of economics knowledge over the first study year in the longitudinal sample, the school leaving grade from secondary education as well as the attendance of an advanced course in B&E in school show a significant impact. This finding was observed in both subgroups.
We also analysed other personal and structural factors in MLM (ICC = 0.081) that might influence student learning over the course of the first year of studies, such as overload due to media consumption and level of fluid intelligence (Table 3). Although other explanatory variables are included, the average school leaving grade continues to be a significant predictor of domain-specific knowledge even after one year in tertiary education. Attending a business-related advanced course is no longer significant, which is probably due to the inclusion of the test score for T1, which can already reflect the influence of school knowledge. The degree of fluid intelligence as well as media overload also contribute significantly to variance clarification (for more information on media use, see Maurer et al. Reference Maurer, Schemer, Zlatkin-Troitschanskaia, Jitomirski and Zlatkin-Troitschanskaia2020; Jitomirski et al. Reference Jitomirski, Zlatkin-Troitschanskaia, Schipolowski, Zlatkin-Troitschanskaia, Pant, Toepper and Lautenbach2020).
Table 3. MLM in the longitudinal sample with knowledge test score at T2 as the dependent variable (group variable: 27 universities).
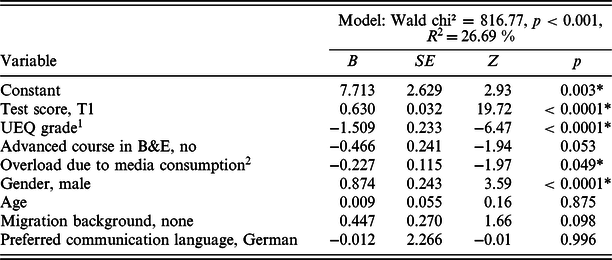
Note. N = 851; 1 UEQ = university entrance qualification; in Germany, lower grades indicate better performance; 2 lower numbers indicate more overload; * indicates significance at a 5% level.
For students of the social sciences neither of the new explanatory variables was significant in the prediction, for students of B&E the overload has no significant part in the explanation of variance, but the level of fluid intelligence does. The test value for T1 is the most significant predictor of the test value for T2 in all samples.
In this context, our study also produced an interesting additional finding: in an online survey we asked the students about the sources of learning they use when preparing for their lectures and for exams, including traditional learning media such as textbooks and lecture notes, scientific sources as well as mass and social media. This survey, differentiating between 14 different sources, showed that a significant proportion of students use online sources, such as Wikipedia, more often than textbooks when preparing for exams. Overall, it became evident that students use a huge variety of different media and sources that may comprise very diverse or even contradictory information (for details, see Maurer et al. Reference Maurer, Schemer, Zlatkin-Troitschanskaia, Jitomirski and Zlatkin-Troitschanskaia2020; Jitomirski et al. Reference Jitomirski, Zlatkin-Troitschanskaia, Schipolowski, Zlatkin-Troitschanskaia, Pant, Toepper and Lautenbach2020).
Discussion and Conclusion
As an example of the investigation of the transition from secondary to tertiary education within the framework of the KoKoHs research programme, findings from the WiWiKom II project regarding students of B&E and social sciences were presented, indicating the high heterogeneity in students’ study-related preconditions. In particular, secondary school graduates arrive at the transition to higher education with a very heterogeneous level of domain-specific knowledge, even when looking at more homogeneous subgroups (after formal selection processes when entering university) (RQ 1).
The development of domain-specific knowledge over the course of study in the domains examined here is lower than can be expected after the first year of study. Particularly in basic knowledge, no significant progress seems to have been made even though the content measured by the test items is part of the first-year curricula (RQ 2). This can partly be explained by the fact that both study domains are known for being mass study courses. Previous studies reveal only a low and often non-significant role of large-scale (introductory) lectures (Schlax et al. Reference Schlax, Zlatkin-Troitschanskaia, Kühling-Thees, Brückner, Zlatkin-Troitschanskaia, Pant, Toepper and Lautenbach2020). Alternative learning analysis approaches based on the decomposition of the overall pre-post-test score allow deeper insights into the changes in knowledge levels (Walstad and Wagner Reference Walstad and Wagner2016; Schmidt et al. Reference Schmidt, Zlatkin-Troitschanskaia, Walstad and Zlatkin-Troitschanskaia2020; Schlax et al. Reference Schlax, Zlatkin-Troitschanskaia, Schmidt, Kühling-Thees, Jitomirski and Happ2019).
With regard to influencing factors (RQ 3), school entry preconditions are significant in predicting the level of knowledge at the beginning of higher education and in predicting the level of knowledge after one academic year. Consequently, previous secondary education is not only significantly relevant for the formal transition to higher education but also has an impact on domain-specific student learning and study progress.
In this context, there is also evidence of influences from other generic interdisciplinary skills such as critical dealing with learning information. In particular, the results on the influence of overload due to media use over the course of higher education studies stress the relevance of carefully filtering out and critically dealing with different kinds of information, which are considered crucial generic skills in the twenty-first century. As many current studies indicate, university students have great deficits when it comes to critically dealing with this multitude of media and online information (Hahnel et al. Reference Hahnel, Kroehne, Goldhammer, Schoor, Mahlow and Artelt2019a; Hahnel et al. Reference Hahnel, Schoor, Kröhne, Goldhammer, Mahlow and Artelt2019b; McGrew et al. Reference McGrew, Breakstone, Ortega, Smith and Wineburg2018; Zlatkin-Troitschanskaia et al. Reference Zlatkin-Troitschanskaia, Shavelson, Schmidt and Beck2019b). This is evidently the case for beginning students when entering higher education but also for advanced bachelor’s and even master’s students (Shavelson et al. Reference Shavelson, Zlatkin-Troitschanskaia, Beck, Schmidt and Marino2019; Nagel et al. Reference Nagel, Zlatkin-Troitschanskaia, Schmidt, Beck, Zlatkin-Troitschanskaia, Pant, Toepper and Lautenbach2020).
The findings from these studies show that even though they are studying in bachelor’s or master’s degree courses, the students have great deficits when it comes to critically dealing with (online) information. The university students are much less capable of critically dealing with online information than one would expect considering their good performance in their course of studies, as evidenced by their results in the subject-specific knowledge assessment and their achieved grades. For instance, one of the studies, iPAL, focuses on measuring students’ critical handling of (online) information on a proximal basis, using a performance assessment (for details, see Zlatkin-Troitschanskaia et al. Reference Zlatkin-Troitschanskaia, Shavelson, Schmidt and Beck2019b; Shavelson et al. Reference Shavelson, Zlatkin-Troitschanskaia, Beck, Schmidt and Marino2019; Nagel et al. Reference Nagel, Zlatkin-Troitschanskaia, Schmidt, Beck, Zlatkin-Troitschanskaia, Pant, Toepper and Lautenbach2020).
In the other study, CORA (Critical Online Reasoning Assessment), an assessment framework developed by Wineburg was adapted and used to measure students’ online reasoning skills, i.e. how well the students are able to assess the trustworthiness of information sources on controversial societal issues by asking them to evaluate real websites in an open web search (Molerov et al. Reference Molerov, Zlatkin-Troitschanskaia and Schmidt2019). Although the ability to critically deal with online information is required both in curricula and as learning outcomes in higher education, as confirmed by a curricula study in 2018 (Zlatkin-Troitschanskaia et al. Reference Zlatkin-Troitschanskaia, Shavelson, Schmidt and Beck2019b) the bachelor’s and master’s students seem to have been insufficiently or ineffectively educated in this skill so far in undergraduate studies.
Limitations
Empirical longitudinal research in higher education is characterized by very specific challenges, such as natural panel mortality, in which a significant proportion of students drop out of their studies. The current dropout rates in the assessed study domains are approximately 30% (Federal Statistical Office 2017). While the sample drawn randomly in T1 is a representative sample, the sample in T2 as well as the matched sample do not rule out different selection effects and bias. This must be critically considered especially in the results for RQ 2.
While the domain-specific knowledge in T1 and T2 was assessed using the valid test, all other variables, such as school grades, are based on the self-reported information of test takers and are therefore not objective data. Due to data protection regulations, it was not possible to include school grades that had actually been achieved. Also, in the domain-specific test, bias – for instance, due to test motivation – cannot be ruled out since this was a low-stake test (e.g. Biasi et al. Reference Biasi, De Vincenzo and Patrizi2018).
Moreover, only a selection of indicators on student learning outcomes and their predicting variables were considered in the study due to the limited testing time. Even if all the variables examined here are included in the modelling, only about a quarter of the variance of domain-specific knowledge in T1 and T2 is explained. Further influencing variables and indicators of learning in higher education must therefore be sought out (e.g. Shavelson et al. Reference Shavelson, Zlatkin-Troitschanskaia, Marino, In Hazelkorn, Coates and Cormick2018).
Implications
The findings we have presented in this paper allow for the following theses as input for further research and for considerations in practice.
(1) When transitioning from secondary education to higher education, students’ learning preconditions significantly influence their acquisition of knowledge and skills in higher education.
(2) Students’ unfavourable preconditions are often not offset in higher education and may, for instance, lead to low knowledge growth.
(3) Teaching–learning settings in higher education are significantly less formalized than in secondary education and seem to have only little influence on students’ learning.
(4) Many students have severe deficits in terms of both domain-related basic knowledge and generic skills upon entering higher education; this is particularly evident with regard to critically evaluating information and online reasoning, as further studies indicate. These deficits are also rarely offset over the course of studies in higher education.
This poses great challenges for universities if they want to maximize the achievement of knowledge acquisition and professional preparation to remain competitive in the EU context. Especially in mass study subjects such as B&E, individual support in particular is extremely challenging. In this context, initial assessments are required to objectively and reliably identify students’ learning potentials and deficits, and, based on these results, to optimize the allocation to needs-based preparatory courses and tutorials.
Considering the domain-specific and generic student learning outcomes that are expected in higher education, for instance in economics, only a few of these facets seem to be explicitly fostered in higher education. As our findings indicate, we cannot expect that deficits at the end of upper secondary school are offset over the course of higher education studies. The large-scale studies indicate a great variance in the skill and knowledge levels of students entering higher education. The results stress the importance of valid entry diagnostics at the stage of transition between secondary and higher education.
About the Authors
Olga Zlatkin-Troitschanskaia, Dr. phil., is Professor for Business and Economics Education; Fellow of the International Academy of Education since 2017; Fellow of the German National Academy of Science and Engineering since 2017; and Fellow of the Gutenberg Research College (2013–2018). Her many international commitments to science and research include membership in several advisory and editorial boards, including the scientific advisory board of the European Academy of Technology and Innovation Assessment and the editorial advisory board of the journal Empirical Research in Vocational Education and Training. She has been guest editor for six national journals (e.g. German Journal of Educational Psychology) and ten international journals (e.g. British Journal of Educational Psychology); besides 300+ articles in national and international journals and volumes, she has published four monographs and co-edited 11 volumes, including Frontiers and Advances in Positive Learning in the Age of Information; Assessment of Learning Outcomes in Higher Education.
Jasmin Schlax, MSc, is research assistant at the Chair of Business and Economics Education, Johannes Gutenberg University Mainz. She has (co-)authored several publications, for example on the development of domain-specific knowledge over the course of university studies, and numerous articles in national and international journals and volumes, e.g. Influences on the Development of Economic Knowledge over the First Academic Year: Results of a Germany-Wide Longitudinal Study.