Introduction
Speech perception skills are crucial for second-language (L2) acquisition, not only to benefit from aural input but also to participate in conversational interaction. While listening in a native language (L1) is usually an effortless process, spoken word recognition is harder for L2 listeners due to their less accurate word segmentation, less accurate phoneme perception, and increased lexical competition arising from L1 words (Cutler, Reference Cutler2012). Given these speech processing difficulties inherent to L2 listening, it may be especially hard for L2 listeners to deal with the added complication of interspeaker variability, such as accent variation (Field, Reference Field2008). Unfamiliar accents can impair speech processing and comprehension both for L1 listeners (e.g., Adank et al., Reference Adank, Evans, Stuart-Smith and Scott2009; Clopper & Bradlow, Reference Clopper and Bradlow2008; Floccia et al., Reference Floccia, Goslin, Girard and Konopczynski2006; Munro, Reference Munro1998) and L2 listeners (e.g., Bent & Bradlow, Reference Bent and Bradlow2003; Escudero & Boersma, Reference Escudero and Boersma2004; Major et al., Reference Major, Fitzmaurice, Bunta and Balasubramanian2005; Pinet et al., Reference Pinet, Iverson and Huckvale2011). Since L2 learners often encounter multiple regional and foreign accents of their L2 in educational and professional settings abroad, the ability to adapt their perception to accommodate accent variation—what we refer to as perceptual learning—is a key communicative competency (Canagarajah, Reference Canagarajah2006; Harding, Reference Harding2014).
The present study aims to better understand the learning mechanisms that facilitate L2 perceptual learning of accents in dialogue. Interactive communication is considered to be an important locus of L2 acquisition in general (Ellis, Reference Ellis and Ellis1999, Reference Ellis2003; Long, Reference Long1980), but perceptual learning has almost never before been researched in conversational contexts, likely due to the methodological challenge of maintaining the necessary experimental control over the phonetic input. To achieve this control to study perceptual learning in conversation, we employ an innovative paradigm in which participants interact face-to-face with a confederate whose speech is entirely prerecorded (Felker et al., Reference Felker, Troncoso-Ruiz, Ernestus and Broersma2018). While briefly hiding her face behind a screen, the confederate uses a hidden keyboard to play prerecorded utterances to participants’ headphones. These utterances include task-relevant phrases and various flexible remarks to respond to any spontaneous questions, creating the convincing illusion of a live conversation. We also replicate the main experiment in a more traditional setup in which participants interact with what they believe to be a “smart computer player.” By combining the face-to-face and computer-based settings in one study, we test whether both settings tap into the same underlying processes for L2 perceptual learning.
Within these interactive settings, we investigate two factors that may facilitate perceptual learning of the interlocutor’s novel accent: (1) corrective feedback and (2) lexical guidance provided visually that constrains the interpretation of key phonemes. Perception-oriented corrective feedback and lexical guidance are two well-studied mechanisms for sound learning, but neither has been studied in the context of a two-way communicative dialogue. Moreover, they are typically studied in different disciplines, with corrective feedback featuring in research on instructed L2 learning (e.g., Brown, Reference Brown2016) and lexical guidance in research on L1 perception and psychophysics (e.g., Samuel & Kraljic, Reference Samuel and Kraljic2009). While L2 learning in general benefits more from explicit than implicit instruction (Norris & Ortega, Reference Norris and Ortega2000) and more from explicit than implicit corrective feedback (Rassaei, Reference Rassaei2013), implicit lexical guidance may be advantageous because it retunes perception automatically (McQueen et al., Reference McQueen, Norris and Cutler2006b) and because lexical information may have a less ambiguous interpretation than feedback in an interactive context. By studying corrective feedback and lexical guidance together, we aim to reconcile research from different fields and examine the extent to which both explicit and implicit information can contribute to L2 perceptual learning of accents.
Explicit perceptual learning through corrective feedback
Interactional feedback, including corrective feedback, is theorized to facilitate L2 learning because it brings learners’ errors to their conscious awareness, helping them to “notice the gap” between their own productions and target forms (e.g., Schmidt, Reference Schmidt and Robinson2001). For speech perception, explicit corrective feedback in interaction would help listeners notice the discrepancy between their interpretation of the spoken input and what their interlocutor intended to communicate. Corrective feedback in the language classroom has been shown to facilitate L2 grammar, vocabulary, and pronunciation learning (e.g., see meta-analysis of Brown, Reference Brown2016). However, research about corrective feedback for L2 speech perception primarily employs noninteractive settings, such as computer-based training programs using highly controlled phonetic input. These programs have been proven effective for learning L2 sounds that do not make a phonemic distinction in the L1 (e.g., Bradlow et al., Reference Bradlow, Akahana-Yamada, Pisoni and Tohkura1999; Iverson et al., Reference Iverson, Hazan and Bannister2005; Wang & Munro, Reference Wang and Munro2004). Furthermore, Lee and Lyster (Reference Lee and Lyster2016b) demonstrated that the type of corrective feedback matters, using forced-choice listening tests with phonological minimal pairs. Visual corrective feedback, implemented as the word “wrong” shown onscreen, was less effective than auditory feedback, which consisted of a voice saying either “No, s/he said [X],” “No, not [Y],” or “No, s/he said [X], not [Y].” The most effective feedback type was the contrastive auditory feedback that combined the target and nontarget forms. The authors reasoned that it was superior because it aurally reinforced the target form and increased learners’ awareness of phonetic differences by accentuating the gap between the intended forms and what they thought they heard.
To our knowledge, only one study has examined the effect of corrective feedback on L2 speech perception outside the context of computer-based training. Moving closer to a naturalistic, interactive setting, Lee and Lyster (Reference Lee and Lyster2016a) used classroom simulations providing form-focused instruction on L2 vowel contrasts. Learners practiced their perception with pick-a-card, bingo, and fill-in-the-blank games with minimal pairs. Whenever a learner made a perceptual error, such as by selecting the wrong word in a minimal pair, the instructor repeated the learner’s wrongly chosen word verbatim with rising intonation. If the learner did not self-repair, more explicit feedback was given: “Not [Y], but [X].” Compared to a control classroom where no feedback was given, learners in the corrective feedback classroom performed significantly better on word identification posttests. Taken together, Lee and Lyster’s (Reference Lee and Lyster2016a, Reference Lee and Lyster2016b) studies suggest that contrastive, or more explicit, corrective feedback is more effective than generic, or implicit, feedback. It remains to be seen whether corrective feedback can also facilitate the learning of a novel accent, rather than a novel L2 phonemic contrast. Moreover, as even classroom-based corrective feedback is not always interpreted by learners the way teachers intended (Mackey et al., Reference Mackey, Al-Khalil, Atanassova, Hama, Logan-Terry and Nakatsukasa2007), the interpretability of corrective feedback in a communicative dialogue merits further study. To determine whether feedback was interpreted accurately, it can be informative to examine learners’ immediate response to the feedback, or uptake (Mackey et al., Reference Mackey, Gass and McDonough2000). The present study compares the interpretability of two types of corrective feedback in dialogue—generic and contrastive feedback—to assess which better promotes uptake for L2 perceptual learning.
Implicit perceptual learning through lexical guidance
Not only does interaction provide the opportunity for explicit learning through corrective feedback, but it also creates the context for implicit learning. The most studied implicit learning mechanism for perceptual adaptation to accents is lexically guided learning (McQueen et al., Reference McQueen, Cutler and Norris2006a; Norris et al., Reference Norris, McQueen and Cutler2003): listeners use top-down lexical knowledge to constrain their interpretation of ambiguous sounds and, after exposure to those ambiguous sounds in different lexical frames, adjust their phonemic boundaries to accommodate the accent. For instance, if a speaker repeatedly pronounces /æ/ in different lexical contexts where /ε/ is expected (e.g., pronouncing “west” as /wæst/ instead of /wεst/), the listener’s perceptual /ε/ category eventually expands to allow for /æ/-like realizations. Lexical guidance provides feedback from the lexical to the prelexical level of processing (Norris et al., Reference Norris, McQueen and Cutler2003), and the resultant perceptual learning occurs automatically as a result of exposure to ambiguous sounds in lexically biased contexts (McQueen et al., Reference McQueen, Norris and Cutler2006b). The effects of lexical guidance on perceptual learning can be measured with a lexical decision task. For instance, Maye et al. (Reference Maye, Aslin and Tanenhaus2008) showed that L1 English listeners who heard systematically lowered front vowels (e.g., /ε/ lowered to /æ/) within a short story adapted their posttest auditory lexical decision judgments in accordance with the vowel shift (e.g., becoming more likely to judge /wæb/, an accented pronunciation of “web,” as being a real word). The effect of lexical guidance in perceptual adaptation is typically studied with L1 listeners and almost exclusively in noninteractive tasks due to the requirement for highly phonetically controlled stimuli (see reviews by Samuel & Kraljic, Reference Samuel and Kraljic2009; Baese-Berk, Reference Baese-Berk2018). Thus, it remains to be seen how effective lexical guidance is for perceptual learning in an interactive, L2 listening context.
In recent years, lexically guided perceptual learning has also been demonstrated in L2 listening. Mitterer and McQueen (Reference Mitterer and McQueen2009) showed that adding English-language subtitles to videos of heavily accented Australian or Scottish English speech improved Dutch listeners’ subsequent perceptual accuracy for the dialects, supporting the theory that the lexical guidance provided by the subtitles facilitated perceptual retuning of the accented sounds. Drozdova et al. (Reference Drozdova, Van Hout and Scharenborg2016) showed that Dutch listeners could adapt to an ambiguous sound between /ɹ/ and /l/ embedded into an English short story, shifting their phonemic category boundary in a different direction depending on whether they had heard the sound in /ɹ/- or /l/-biasing lexical contexts. Lexically guided learning in an L2 has been attested not only when the L2 is phonologically similar to the L1, such as with Swedish L2 listeners of German (Hanulíková & Ekström, Reference Hanulíková and Ekström2017) and German L2 listeners of Dutch (Reinisch et al., Reference Reinisch, Weber and Mitterer2013) and English (Schuhmann, Reference Schuhmann2014), but also when the L2 is phonologically unrelated to the L1, as with English L2 listeners of Mandarin (Cutler et al., Reference Cutler, Burchfield, Antoniou, Epps, Wolfe, Smith and Jones2018). However, crosslinguistic constraints on L2 perceptual learning have also been observed. For instance, Cooper and Bradlow (Reference Cooper and Bradlow2018) showed that after exposure to accented English words presented in a lexically or semantically disambiguating context, Dutch listeners exhibited perceptual adaptation for words containing the trained accent pattern, but only for deviations involving phoneme pairs that were contrastive in both the L1 and L2.
To the best of our knowledge, lexically guided perceptual learning has never before been demonstrated in conversational interaction, where the listener also has to produce speech. Leach and Samuel (Reference Leach and Samuel2007) found evidence that lexically guided perceptual adaptation involving newly learned words was severely impaired when the participants had both heard and spoken the words aloud during the word training, compared to a condition in which they had only passively listened to the words during training. Baese-Berk and Samuel (Reference Baese-Berk and Samuel2016) showed that in a feedback-based discrimination training paradigm, perceptual improvement for novel L2 sounds was disrupted when listeners had to intermittently produce speech as part of the training, possibly due to increased cognitive load. Similarly, Baese-Berk (Reference Baese-Berk2019) found that producing speech during training disrupted perceptual learning of novel sound categories in an implicit distributional learning paradigm, and she proposed that this may result from an overload in shared cognitive processing resources between the perception and production modalities. Overall, these studies suggest that more research is needed about the effectiveness of implicit perceptual learning mechanisms in cognitively demanding, interactive settings.
The present study
This study investigates the effectiveness of two types of corrective feedback and of lexical guidance on perceptual learning of a novel L2 accent in conversation. Native Dutch-speaking participants engaged in a task-based dialogue in English with an interlocutor whose accent contained an unexpected vowel shift, whereby /ε/ was pronounced as /ɪ/. These vowels were chosen for three reasons: (1) Dutch listeners should already perceive them as two different phonemes, given that they are also contrastive in Dutch (e.g., Booij, Reference Booij1999), (2) they distinguish many English minimal pairs, facilitating the creation of experimental stimuli, and (c) this vowel shift is phonologically plausible, as short front vowel raising has been observed in various Southern Hemisphere English dialects, such as New Zealand (Kiesling, Reference Kiesling, Kachru, Kachru and Nelson2006; Maclagan & Hay, Reference Maclagan and Hay2007), Australian (Cox & Palethorpe, Reference Cox and Palethorpe2008), and South African English (Bowerman, Reference Bowerman and Mesthrie2008). We restricted the accent manipulation to this single vowel shift and inserted it into an unfamiliar regional dialect (see the “Materials” section for details) to ensure that all participants would begin the experiment with no prior knowledge of the overall accent. To carefully control participants’ phonetic exposure, all of the interlocutor’s speech was prerecorded and scripted to avoid the experimental sounds outside of critical utterances.
In each round of the interactive information-gap task, named “Code Breaker,” the participant’s task was to recognize a visual pattern in a sequence of shapes on their computer screen and tell their interlocutor what shape should follow to complete the sequence. The interlocutor would then tell the participant to click on one of the four words displayed on the participant’s screen, as the interlocutor’s screen indicated that this word was linked to the participant’s shape. As the four word options always consisted of two phonological minimal pairs, the participant had to listen carefully to their interlocutor’s pronunciation to choose the right word. On critical trials, the target word was spelled with “e” and contained /ε/ in Standard English but was pronounced with /ɪ/ instead, reflecting the experimental vowel shift.
Participants played Code Breaker in one of the four conditions which differed in the mechanism available to learn the /ε/-/ɪ/ vowel shift. The Control condition contained no evidence for vowel shift. Whenever the interlocutor said a critical target word (e.g., “set” pronounced /sɪt/), both the “e”-spelled and “i”-spelled member of the relevant minimal pair (e.g., “set” and “sit”) were among the onscreen word options. Control participants never received feedback about their selection and could thus assume their interlocutor’s /ɪ/-pronounced word matched the “i”-spelled word onscreen, as it would in Standard English.
In the two corrective feedback conditions, the interlocutor responded verbally to incorrect choices. In the Generic Corrective Feedback condition, whenever the participant incorrectly selected the “i”-spelled competitor instead of the “e”-spelled target, the interlocutor simply remarked that a mistake was made (e.g., “Oh no, that’s not the one!”). In the Contrastive Corrective Feedback condition, she instead used more specific phrasing that contrasted the target with the competitor (e.g., “Oh, you wanted “set” /sɪt/, not “sit” /sit/!”, the /ɪ/ being pronounced /i/ to follow the vowel-raising pattern). In both conditions, we expected participants to become explicitly aware that the word they had originally understood did not match the word their partner was trying to communicate.
In the Lexical Guidance condition, evidence for the vowel shift was implicit and came exclusively from how the onscreen lexical options constrained the possible interpretation of the phonetic input. Crucially, the “e”-spelled target word was shown paired with a consonant competitor (e.g., target “set” and competitor “pet”), while the “i”-spelled option (e.g., “sit”) was absent. Thus, the lexical context would imply that the /ɪ/ heard was meant to represent /ε/ (e.g., /sɪt/ could only match “set”), promoting implicit lexically guided learning of the shift.
The amount of perceptual learning that occurred during the dialogue was measured in two ways. First, word identification accuracy in the critical Code Breaker trials was taken as a measure of listeners’ uptake: the degree to which they correctly interpreted recent corrective feedback or lexical guidance to accommodate to the accentFootnote 1. Second, an auditory lexical decision task following the dialogue was taken as a measure of listeners’ online processing of the vowel shift. In this task, the same interlocutor produced a series of (prerecorded) words and pseudowords, some pronounced with /ɪ/, and participants had to make speeded judgments about whether each one was a real word or not. For two critical item types, to be described later, the expected response (yes/no) would differ depending on whether or not the /ɪ/ was perceived as representing /ε/.
A final aim of this study was to investigate perceptual learning within two different interactive settings. Accordingly, one participant group completed the entire experiment while interacting face-to-face with the experimenter, who surreptitiously played the prerecorded utterances to participants’ headphones to create the illusion of a live conversation (using the “ventriloquist paradigm”; Felker et al., Reference Felker, Troncoso-Ruiz, Ernestus and Broersma2018). The other participant group completed the same experiment without the interlocutor co-present; instead, they were told they were interacting with a “smart computer player.” While the computer player setting resembles traditional speech perception experiments, the face-to-face setting resembles real-life social interaction, a more typical context for L2 acquisition.
The research questions and hypotheses are as follows:
-
RQ1: (a) Does corrective feedback about erroneous perception of a novel accent in dialogue lead to uptake during the interaction for L2 listeners? (b) If so, which is more effective: generic or contrastive corrective feedback?
-
H1: (a) We predict that corrective feedback about a dialogue partner’s novel accent will lead to uptake and improve L2 listeners’ perceptual accuracy for accented words over the course of the conversation. That is, compared to Control participants who received no evidence for the vowel shift and whose accuracy should remain close to zero, listeners in both the Generic and Contrastive Corrective Feedback conditions should show a pattern of increasing accuracy across the critical Code Breaker trials. (b) We further expect contrastive feedback to be more effective than generic feedback because the former is more explicit and interpretable and thereby better promotes noticing the gap between the word the listener perceived and the word the speaker intended.
-
RQ2: Do corrective feedback and lexical guidance about a novel accent in dialogue improve L2 listeners’ subsequent online processing of the accent?
-
H2: We predict that corrective feedback and lexical guidance will directly improve online processing of accented speech in the auditory lexical decision task. We expect to observe the most improved processing in listeners who played Code Breaker in the Lexical Guidance condition because the lexical guidance will be automatically processed and entail less room for ambiguity in interpretation than corrective feedback during the dialogue. We also expect participants who received contrastive corrective feedback to show more improved processing than those who received generic corrective feedback, again because the more explicit feedback type will be more clearly interpretable. Moreover, we expect that each individual’s Code Breaker accuracy itself, as a measure of their uptake for the accent, will predict their online processing even more robustly than the experimental condition in which they played the game.
In the lexical decision task, we define improved online processing as being faster and more likely to accept Critical Words with /ɪ/ pronunciations representing /ε/ (e.g., /bɪst/ as the pronunciation of “best”), which would sound like nonwords to naïve listeners. Accepting these words would indicate that listeners have expanded their /ε/ category boundary to include /ɪ/-like pronunciations. We also explore whether listeners learn an even stricter rule, that /ɪ/ not only can but must represent /ε/, by examining whether they become more likely and faster to reject Critical Pseudowords with /ɪ/ pronunciations representing /ε/ (e.g., /gɪft/ as the pronunciation of “geft”). This would require overriding the real-word interpretation of these items (e.g., “gift”), reflecting an even stronger form of learning.
-
RQ3: Does the amount of perceptual learning of a novel L2 accent in dialogue differ between a computer-based setting and a face-to-face setting?
-
H3: We might expect to observe more perceptual learning in the face-to-face setting than in the computer-based setting because listeners may experience stronger social resonance with a human interlocutor. Successful perceptual learning entails listeners aligning their phonological representations to their interlocutor’s, and linguistic alignment is known to be affected by social factors as well as by the perceived human or computer nature of the interlocutor (Branigan et al., Reference Branigan, Pickering, Pearson and McLean2010). On the other hand, we might find no differences in learning between the two settings, which would in any case show that results from the more traditional, computer-based setting generalize to a more naturalistic context.
Methodology
Participants
The participants were 108 native Dutch speakers, assigned to conditions on a rotating basis such that 27 people were tested in each of the four conditions (Control, Generic Corrective Feedback, Contrastive Corrective Feedback, and Lexical Guidance). Per condition, 15 participants were tested in the face-to-face setting and 12 in the computer player setting; we tested more in the face-to-face setting in case we would need to exclude participants due to technical problems arising from this more complicated experimental setup (in the end, no such problems arose). Participants were aged 18 to 30 (M = 21.7, SD = 2.6) years, and 60.2% were female. All were raised monolingually and reported that English was their most proficient L2. On average, they reported speaking English 1.6 hr per week (SD = 2.8 hr) and listening to English for 11.9 hr per week (SD = 10.6 hr). On a scale ranging from 0 (“no ability”) to 5 (“native-like ability”), participants’ mean self-rated English proficiency was 2.9 for speaking (SD = 0.8), 3.3 for listening (SD = 0.7), 3.0 for writing (SD = 0.9), and 3.7 for reading (SD = 0.7). These measures of English usage and proficiency did not differ significantly between participants across the four conditions (all p’s > .05). Participants in the four conditions also reported similar levels of prior familiarity with Australian (F(3, 104) = 0.68, p = .56) and New Zealand English (F(3, 104) = 0.33, p = .80), making it unlikely that any one group would be more familiar with the experimental vowel shift. All participants gave written informed consent and received course credit or financial compensation in exchange for participating.
Procedures
General procedures
Participants played 84 rounds of the Code Breaker game in one of the four conditions (Control, Generic Contrastive Feedback, Contrastive Corrective Feedback, or Lexical Guidance) and in one of the two settings (face-to-face or computer player). Directly afterward, they completed 96 auditory lexical decision trials in the same setting. The Code Breaker game typically lasted 15–20 min, and the lexical decision task took about 6–10 min.
Face-to-face setting. The 60 participants in the face-to-face setting interacted with an interlocutor who was actually the experimenter, pretending to be just another participant, while another researcher took charge of the session. To prevent any spoken interaction between the participant and experimenter before the start of the game (which would have revealed the mismatch between the prerecorded speech and the experimenter’s own voice), the participant received instructions for Code Breaker in a separate room before the start of the main task. The researcher in charge of the session then guided the participant into the main testing room, where the experimenter was already sitting with headphones on and pretending to be busy finishing another task, staring intently at her screen and pressing buttons. The participant was quickly instructed to take a seat behind their own monitor, across the table from the interlocutor, and to put on their headphones for the remainder of the experiment. From that point on, the experimenter communicated with the participant using a hidden keypad to play different categories of prerecorded speech, ducking her face behind her monitor whenever “speaking” (for technical implementation details, see Felker et al., Reference Felker, Troncoso-Ruiz, Ernestus and Broersma2018). The illusion that the prerecorded speech was actually being spoken in real time was supported by a cover story, explained in the previous room, that both players would be speaking into microphones that transmitted their speech into each other’s headphones.
Computer player setting. The 48 participants in the computer player setting received the same instructions for the Code Breaker and lexical decision tasks as the participants in the face-to-face setting except they were told that their interlocutor for both tasks was a smart computer player capable of recognizing their speech and talking back. In fact, the role of the computer player interlocutor was played by the experimenter, who listened to participants’ speech from outside the testing booth via headphones. As in the face-to-face setting, she used a keyboard to control the playing of the prerecorded utterances into participants’ headphones.
Code Breaker game
Each Code Breaker trial featured a set of puzzle shapes and four words consisting of two minimal pairs, which appeared on the participant’s and interlocutor’s screens as shown in Figure 1. The participant’s screen displayed the puzzle sequence above the four words, randomly positioned in four quadrants. Participants had been instructed that their partner’s screen displayed four potential answer shapes, each linked to one of the four words, and that the trial’s target word was linked to the correct answer shape for the puzzle.

Figure 1. Example screens of a single Code Breaker trial as it appeared for the participant (left) and the interlocutor (right). Here, the puzzle’s correct answer is a gray square, corresponding to the trial’s target word “better.” In the Lexical Guidance condition, the phonological distractor “bitter” would be replaced with “letter” (on both screens).
In each trial, the participant’s task was to figure out the pattern in their shape series and tell their interlocutor what shape was needed to complete the sequence (for details about the puzzles, see the Materials section). The interlocutor then responded by telling the participant which word was linked to the requested shape, playing the prerecorded target word utterance for that trial (e.g., “Okay, so you want tab,” “That’s, uh, chase”). Finally, the participant had to click on that word to complete their turn. No matter what shape the participant named, the interlocutor responded by playing that specific trial’s target word utterance. If requested to do so, the interlocutor would repeat the word up to two times, playing additional audio tokens. Once the participant clicked on a word, it was highlighted with a gray rectangle on both players’ screens, confirming the selection and ending the round. When appropriate, the interlocutor could play utterances belonging to various predetermined categories to react to the participant’s spontaneous remarks or questions; for example, she could play affirmative responses (e.g., “Uh-huh”), negative responses (e.g., “Um, no”), statements of uncertainty (e.g., “I don’t know”), reassuring remarks (e.g., “No problem!”), and backchannels to indicate listening (e.g., “Mm-hmm”). In the first few Code Breaker trials, the interlocutor would play a short affirmative utterance to indicate that she had seen the participant’s choice.
Control condition and Lexical Guidance condition. In both the Control and Lexical Guidance conditions, participants received no feedback of any kind about whether their answers were right or wrong.
Generic Corrective Feedback condition. In this condition, whenever the participant clicked on a word, the word “correct” in a green box or “incorrect” in a red box appeared in the middle of their screen as visual corrective feedback about their response (similar to Lee & Lyster, Reference Lee and Lyster2016b). If the participant had answered incorrectly, whether on a critical or filler trial, the interlocutor responded by playing a generic corrective feedback utterance (e.g., “Oh no, wrong one,” “Oh no, wasn’t that one”). If the participant acknowledged their error aloud before the feedback could be played, the interlocutor instead played a reassuring remark in order to be more socially appropriate.
Contrastive Corrective Feedback condition. This condition worked exactly as in the Generic Corrective Feedback condition except that, on critical trials, the interlocutor reacted to errors by playing the contrastive corrective feedback utterance associated with that trial’s target word and phonological competitor (e.g., “Oh, the answer was set, not sit,” “Oh no, you wanted better, not bitter”). Generic feedback utterances would have still been played in response to errors on filler trials, but fillers (in any condition) virtually never evoked errors in practice.
Auditory lexical decision task
Instructions for the lexical decision task, presented to participants as the “Word or Not?” game, appeared onscreen right after the last Code Breaker round. The participant read that the other player was going to pronounce a series of real words and non-existing words, one at a time. Based only on the interlocutor’s pronunciation, the participant had to judge whether or not each item was a real word by pressing “Y” or “N” on a button box. The audio recording of each word played automatically after a random delay of 500 to 1,500 ms following trial onset, supporting the illusion that the interlocutor was reading and pronouncing the words in real time. Once the participant responded, a blank screen flashed for 1 s before the next trial; no feedback was provided.
Materials
Code Breaker game
Minimal word pairs . The Code Breaker game featured 16 critical minimal word pairs consisting of a target word and a phonological competitor (see Appendix A). All critical target words were spelled with “e” and contained /ε/ in Standard English pronunciation (e.g., “set”). In the Control condition and both Corrective Feedback conditions, each target’s /ε/ was replaced with /ɪ/ to form the phonological competitors (e.g., target “set” paired with competitor “sit”). In the Lexical Guidance condition, the critical phonological competitor was formed by replacing one of the target words’ consonants (e.g., target “set” with competitor “pet”). All critical minimal pairs consisted of mono- and disyllabic words of medium to high frequency in the SUBTLEX-UK corpus (Van Heuven et al., Reference Van Heuven, Mandera, Keuleers and Brysbaert2014).
The game also included 64 filler minimal word pairs, comparable to the critical pairs in length and frequency, designed to draw attention away from the critical /ε/-/ɪ/ contrast. To balance out the 16 critical pairs’ “e”-spelled targets and “i”-spelled competitors, there were an additional 16 pairs with “i”-spelled targets (vs. competitors with any nonexperimental vowel, e.g., target “bike” with competitor “bake”) and 16 pairs with “e”-spelled competitors (vs. targets with any nonexperimental vowel, e.g., target “tall” vs. competitor “tell”). Importantly, the “i”-spelled items in the former group were always pronounced with /aɪ/ rather than /ɪ/ to avoid providing additional information about the /ɪ/ sound, and the “e”-spelled items in the latter group, being competitors rather than targets, were never actually pronounced. Finally, 16 filler pairs had various initial consonant contrasts (e.g., “down” vs. “town”) and 16 had final consonant contrasts (e.g., “proof” vs. “prove”).
Each Code Breaker trial included four words: one target word and its phonological competitor plus a distractor minimal pair. To form trial lists, each critical and filler minimal pair was used once as the target pair (i.e., the pair whose target word was the right answer for that trial) and once as the distractor pair. The minimal pairs were pseudo-randomly combined into trials such that no trial combined two minimal pairs of the same contrast type. The order of the main 80 trials (16 critical + 64 filler) was pseudo-randomized such that any two trials with critical target pairs were separated by at least two trials with filler target pairs. Each trial list was then prepended with a set of 4 fixed word quadruplets comprising relatively easy minimal pairs as warm-up items, yielding 84 total trials.
Prerecorded speech . All prerecorded speech was scripted to avoid any instances of /ε/, /ɪ/, or /i/ except within the target words and contrastive corrective feedback, thereby ensuring controlled exposure to the vowel shift across conditions and preventing incidental learning of the vowel shift from the carrier phrases. The utterances were recorded at 44.1 kHz with a headset microphone in a sound-attenuating booth by a young adult female native speaker of Middlesbrough English. Her accent differed from Standard British English in several ways, for example, /t/ was often glottalized, /ʌ/ was pronounced as /ʊ/, /eɪ/ was monophongized to /eː/, and /ɘʊ/ was monophongized to /ɔː/. Crucially, for the purposes of this experiment, a short front vowel shift was introduced into her accent such that she pronounced /ε/ as /ɪ/ and /ɪ/ as /i/. Thus, all critical /ε/-containing target words were pronounced with /ɪ/, and their /ɪ/-containing phonological competitors (only heard in the contrastive feedback utterances) were pronounced with /i/. This effect was achieved by replacing certain words in her script (e.g., replacing “set” with “sit” and “sit” with “seat”) and, if necessary, eliciting the desired pronunciation with pseudowords (e.g., replacing “middle” with “meedle”).
Puzzles . The Code Breaker game included 84 unique puzzles (see examples in Appendix A). Each puzzle was a sequence of five colored shapes followed by a question mark representing a missing sixth item, whose identity could be determined by a pattern in the preceding sequence (e.g., alternating colors or shapes). The puzzles varied in difficulty to keep the task engaging but were easily solvable within a few seconds. They were distributed randomly across trials so that the combinations of puzzle and target word varied in each experimental list (except the four puzzles fixed to the warm-up trials).
Auditory lexical decision task
The auditory lexical decision task consisted of 96 items recorded by the same speaker as in the Code Breaker game, none of which had appeared previously in the experiment (see Appendix B). There were two critical item types whose lexical status hinged on whether or not their stressed /ɪ/ vowel was interpreted as representing /ε/. The 12 Critical Real Words (e.g., “best”) contained /ε/ in Standard British English but were pronounced with /ɪ/ (e.g., /bɪst/) in accordance with the vowel shift, thereby sounding like nonwords (e.g., *“bist”) to a naïve listener. The 12 Critical Pseudowords (e.g., *“geft”) also contained /ε/ in Standard British English but were pronounced with /ɪ/ following the vowel shift (e.g., /gɪft/), thereby sounding like real words (e.g. “gift”).
The lexical decision task included three filler item types. To draw attention away from the many /ɪ/-pronounced items, there were 36 Filler Real Words (e.g., “game”) and 24 Filler Pseudowords (e.g., *“trup”) that did not contain the /ε/, /ɪ/, or /i/ vowels. The latter were designed with the help of Keuleers and Brysbaert’s (Reference Keuleers and Brysbaert2010) software, which generated items that obeyed English phonotactic constraints and roughly matched the real words in subsyllabic structure and segment transition frequencies. In addition, there were 12 Filler /ɪ/-Pseudowords: items pronounced with /ɪ/ that would remain nonwords regardless of whether or not the /ɪ/ was interpreted as /ε/ (e.g., /frɪp/ representing *“frep” or *“frip”). This category ensured that some of the task’s /ɪ/-pronounced items had unambiguous right answers, unlike the critical items.
Overall, the lexical decision task contained 48 real words and 48 nonwords. All critical and filler item groups contained a 7:5 ratio of monosyllabic to disyllabic items. The Critical and Filler Words were equivalent in their parts of speech and Zipf frequencies (Van Heuven et al., Reference Van Heuven, Mandera, Keuleers and Brysbaert2014). The lexical decision trial lists were ordered pseudo-randomly with two constraints: (1) at least two filler items must come between any two critical items and (2) no streaks of five or more real words or pseudowords were allowed.
Results
For all analyses, we computed linear mixed effects models combining data from the four conditions (Control, Generic Corrective Feedback, Contrastive Corrective Feedback, and Lexical Guidance) and both settings (face-to-face and computer player), using the lme4 package in R (Bates et al., Reference Bates, Maechler, Bolker and Walker2015), with p-values computed using Satterthwaite’s degrees of freedom method of the lmerTest package (Kuznetsova et al., Reference Kuznetsova, Brockhoff and Christensen2017).
RQ1: Uptake during the Code Breaker game
First, we confirmed that corrective feedback utterances were played approximately equally often in the Generic and Contrastive Corrective Feedback conditions. The average number of critical trial feedback utterances played per session was identical between the two conditions (M = 6.74 corrective feedback utterances, SD = 3.18 for Generic and 2.81 for Contrastive Corrective Feedback, t(52.22) = 0, p = 1), implying that any differences in uptake would likely be due to differences in the nature, rather than the quantity, of the feedback.
Table 1 presents descriptive statistics based on each participant’s overall Code Breaker accuracy, per condition, while Figure 2 displays the mean accuracy per condition over the course of the critical trials. These statistics confirm the expected near-zero accuracy in the Control condition (which contained no evidence for the /ε/-to-/ɪ/ vowel shift) and the near-perfect accuracy in the Lexical Guidance condition (due to the removal of “i”-spelled competitors from the answer options).
Table 1. Overall percent accuracy on critical Code Breaker trials per participant (combining both settings, N = 108)


Figure 2. Mean Accuracy on Critical Code Breaker Trials Over Time (Combining Both Settings); CF = corrective feedback.
To assess whether participants adapted to their interlocutor’s vowel shift during the course of the interaction—clicking on the “e”-spelled target words (e.g., “set”) despite hearing /ɪ/ pronunciations (e.g., /sɪt/)—we analyzed their responses across the 16 critical trials. We computed a generalized logistic mixed effects model with accuracy as the binary dependent variable. The fixed effects were condition (treatment coding with Control condition on the intercept), setting (treatment coding with a computer player on the intercept), critical trial number (continuous variable 1–16), and all possible two- and three-way interactions among these factors. The random effects were participant and word (with random intercepts only, since random slopes prevented convergence).
The full statistical model is provided in Appendix C (see Online Supplementary Materials). The only significant simple effect in the model was an effect of condition indicating that the Lexical Guidance condition had higher overall accuracy than the Control condition (β = 11.44, SE = 2.52, p < .001, 95% CI [6.51, 16.37]). In partial support of our hypotheses, the model also contained a statistically significant interaction between condition and trial number (β = 0.34, SE = 0.11, p = .002, 95% CI [0.13, 0.55]) for the Contrastive Corrective Feedback level of condition only, indicating that these participants became more accurate on critical trials as the game went on (see the third panel of Figure 2). There were no other statistically significant interactions between trial number and either condition or setting, indicating that participants otherwise maintained a similar level of accuracy on critical trials throughout the game. Furthermore, because setting showed no significant simple or interaction effects, the face-to-face and computer player settings appear to be equivalent.
To test the hypothesis that the two corrective feedback conditions differed from each other, we releveled the model with the Generic Corrective Feedback condition on the baseline. This releveled model revealed that the small numerical difference in accuracy between the Generic and Contrastive Corrective Feedback conditions was not significant (β = −0.63, SE = 1.64, p = .70, 95% CI [−3.84, 2.58]).
For the sake of completeness in reporting all significant effects, we also releveled the model to put Lexical Guidance on the intercept, which showed that this condition also had higher accuracy than the Generic Corrective Feedback condition (β = 12.81, SE = 2.57, p < .001, 95% CI [7.78, 17.84]) and the Contrastive Corrective Feedback condition (β = 13.44, SE = 2.58, p < .001, 95% CI [8.38, 18.51]).
In line with our predictions, Corrective Feedback participants thus showed learning over time. However, the fact that Generic Corrective Feedback participants performed no better than Control participants, showing almost no uptake for the accent, was not anticipated. Moreover, the high standard deviations and wide score ranges in both Corrective Feedback conditions (see Table 1) indicate substantial individual variability in how participants responded to the feedback.
RQ2: Online processing in the auditory lexical decision task
To assess listeners’ online processing of accented speech, we analyzed their responses to Critical Words and Critical Pseudowords in the auditory lexical decision task. At the outset, we removed responses with reaction times (measured from word offset) outside +/− 2 standard deviations from the mean for each item type, which amounted to 3.0% of Critical Word responses and 2.5% of Critical Pseudoword responses. In this way, we aimed to restrict the analyses to lexical decisions that were made quickly and automatically as opposed to decisions influenced by a more conscious, deliberate reasoning process.
Critical Words
Responses to Critical Words, such as “best” pronounced as /bɪst/, are summarized in Table 2 and visualized in Figure 3. Higher acceptance rates and faster reaction times to make a “yes” decision would indicate more accurate and efficient processing of the vowel shift: that listeners can (rapidly) interpret /ɪ/ as representing /ε/. With Control participants as the baseline, we expected to observe the most improved processing for Lexical Guidance participants, followed by Contrastive Corrective Feedback and finally Generic Corrective Feedback participants. Additionally, regardless of condition, we expected higher acceptance rates and faster “yes” reaction times for listeners who had exhibited greater uptake of the vowel shift, as measured by their Code Breaker accuracy.
Table 2. Responses to Critical Words in auditory lexical decision task


Figure 3. Critical Word Acceptance Rates (left) and Mean Reaction Times for “Yes” Responses (right) for Each Participant as a Function of their Code Breaker Accuracy and Condition, with Simple Regression Lines; RT = reaction time, CF = corrective feedback.
Acceptance rates. To analyze the Critical Words’ acceptance rates, we computed a generalized logistic mixed effects model with the logit link function. Response (yes/no) was the binary dependent variable. The fixed effects were condition, setting, and their interaction (all with treatment coding). The random effects were participant and item (with random intercepts only, since random slopes prevented convergence). Despite the apparent mean differences across conditions shown in Table 2, no effects proved statistically significant in the model.
We recomputed the model with condition replaced by the other predictor of interest: each participant’s Code Breaker accuracy (standardized as a z-score, continuous variable). As predicted, this model showed that higher Code Breaker accuracy led to significantly more “yes” responses (β = 0.39, SE = 0.16, p = .01, 95% CI [0.08, 0.70]). There was no significant effect of setting, nor a significant interaction between setting and Code Breaker accuracy; thus, the learning effect was equivalent in the face-to-face and computer player settings.
Reaction times. We restricted the reaction time analysis to trials on which participants responded “yes” to the Critical Words, computing a linear mixed effects model with log reaction time from word offset as the dependent variable and random intercepts for participant and item (no random slopes since these prevented convergence). The fixed effects included all theoretical variables of interest (condition, setting, and their interaction, with treatment coding) plus control variables known to influence lexical decision reaction times (trial number, log reaction time on previous trial, word duration, and word frequency based on the Zipf values from Van Heuven et al. [Reference Van Heuven, Mandera, Keuleers and Brysbaert2014], all as continuous variables). Since we observed a pattern of increasing means across conditions in terms of Code Breaker accuracy (Table 1, top row) and Critical Word acceptance rates (Table 2, top row), we applied reverse Helmert coding for the condition factor, comparing each “level” of condition to the preceding levels. We applied the backward elimination procedure provided by the lmerTest library’s step function (Kuznetsova et al., Reference Kuznetsova, Brockhoff and Christensen2017) to remove insignificant predictors, resulting in a final model structure containing the significant fixed effects of condition, log reaction time on previous trial, and trial number; the final model’s fit is shown in Table 3.
Table 3. Model predicting log reaction times to accept Critical Words in auditory lexical decision task

Note. CF = corrective feedback, SE = standard error, RT = reaction time; CI = confidence interval, * = significant.
This model shows that Lexical Guidance participants were faster than those in the other three conditions to accept the Critical Words, as expected. However, there were no significant differences among the other conditions, contrary to our prediction that corrective feedback would also improve online processing. As is typically found in lexical decision experiments, reaction times were correlated with the previous trial’s reaction time and became faster over time. There was no significant simple effect or interaction effect with setting after the model selection procedure, so the learning effect was comparable in the face-to-face and computer player settings.
Next, we repeated this analysis with Code Breaker accuracy (continuous variable) replacing the condition variable. After the backward elimination procedure to remove insignificant predictors, the resulting model contained the significant fixed effects of Code Breaker accuracy, the log of the previous trial’s reaction time, and trial number. As predicted, this model showed that reaction times were significantly faster with increasing Code Breaker accuracy (β = −0.07, SE = 0.02, p = .002, 95% CI [−0.11, −0.03]). Furthermore, reaction times were correlated with the previous trial’s reaction time (β = 0.28, SE = 0.05, p < .001, 95% CI [0.19, 0.36]) and became faster as trials went on (β = −0.0012, SE = 0.0005, p = .03, 95% CI [−0.002, −0.0002]). As the model selection procedure removed setting and its interaction with Code Breaker accuracy, it appears that reaction times in general, and perceptual learning linked to Code Breaker accuracy, were equivalent in both settings.
Critical Pseudowords
Responses to Critical Pseudowords, such as *“geft” pronounced /gɪft/, are summarized in Table 4. Recall that these pseudowords match real words in regular pronunciation (e.g., “gift”), so rejecting them requires overriding the real-word interpretation. Lower acceptance rates and faster “no” responses would indicate a very strong type of learning: that listeners (rapidly) interpret /ɪ/ as necessarily representing /ε/. If this type of learning were to occur, we expected to see the strongest effect (relative to Control participants) for Lexical Guidance participants, followed by Contrastive Corrective Feedback participants and finally Generic Corrective Feedback participants. Furthermore, we expected to observe a stronger learning effect in listeners who had exhibited more uptake for the accent via higher Code Breaker accuracy.
Table 4. Responses to Critical Words in auditory lexical decision task

Acceptance rates . As Table 4 shows, Critical Pseudowords were almost universally accepted as real words, thereby providing no support for the strong learning hypothesis. A generalized logistic mixed effects model constructed the same way as for Critical Words confirmed that no effects of condition or setting were statistically significant, nor were any effects significant when recomputing the model to replace the condition variable with Code Breaker accuracy.
Reaction times. Given the extremely high acceptance rates, there was insufficient data to analyze reaction times to “no” responses as planned. Therefore, we analyzed reaction times to “yes” responses instead, using the same model structure and selection procedure as with Critical Words. For word frequency, we used frequencies of the real words the pseudowords sounded like (e.g., the frequency of “gift” for *“geft” pronounced /gɪft/). Neither condition, setting, nor their interaction were significant predictors, leaving only control predictors in the model as shown in Table 5. When we repeated the modeling procedure with Code Breaker accuracy replacing condition, Code Breaker accuracy was also not significant, yielding an identical final model. In short, the time it took participants to accept the Critical Pseudowords was the same regardless of condition, Code Breaker accuracy, and setting.
Table 5. Model predicting log reaction times to accept Critical Pseudowords in auditory lexical decision task

Note. SE = standard error, RT = reaction time; * = significant.
Filler items
Overall, the responses were as expected, with a majority of “yes” responses to Filler Words (M = 94.4%, SD = 23.0%) and “no” responses to Filler Pseudowords and Filler /ɪ/-Pseudowords (M = 78.7%, SD = 40.9% and M = 86.9%, SD = 33.7%, respectively). Appendix D (see Online Supplementary Materials) provides descriptive statistics and supplementary analyses.
RQ3: Differences between computer-based and face-to-face settings
As described in the preceding sections, the setting (computer player vs. face-to-face) did not interact significantly with condition for predicting uptake in the Code Breaker game, nor did it interact significantly with either condition or Code Breaker accuracy for predicting online processing in the lexical decision task. Therefore, these results do not provide any evidence that the perceptual learning under study differs between the computer-based and face-to-face settings.
Discussion
The purpose of the present research was to investigate the effectiveness of two types of corrective feedback and of lexical guidance at improving the perceptual processing of an unfamiliar accent in an interactive, L2 listening context. To assess whether generic or contrastive corrective feedback would better promote uptake for the accent, we analyzed listeners’ word identification accuracy over the course of the interactive Code Breaker game. Furthermore, using an auditory lexical decision task, we examined whether listeners’ online processing of the accent differed either depending on whether they had received corrective feedback or lexical guidance or depending on how much uptake they had exhibited during the game. Finally, we examined whether perceptual learning differed between a computer-based and a face-to-face interactive setting.
Comparing uptake from generic and contrastive corrective feedback
The first research question was whether corrective feedback would promote uptake by increasing word identification accuracy over the course of the interaction and, if so, whether generic or contrastive feedback would be more effective. Results showed that listeners in the Contrastive Corrective Feedback condition, but not listeners in the Generic Corrective Feedback condition, were more accurate than those in the Control condition for later-occurring critical trials in the Code Breaker game. That is, listeners receiving contrastive corrective feedback began to accommodate their interlocutor’s accent over time, allowing her /ɪ/ pronunciation to represent /ε/ and choosing “e” -spelled words (e.g., “set”) despite hearing /ɪ/-containing pronunciations (e.g., /sɪt/). The superiority of contrastive corrective feedback matches our hypothesis and mirrors the findings of Lee and Lyster (Reference Lee and Lyster2016b), who found that the most effective feedback type for learning a non-native L2 sound contrast was one that auditorily contrasted two members of a minimal pair. One reason for the effectiveness of this feedback type could be that it drew listeners’ attention to the relevant phonological contrast, simultaneously providing positive evidence for the correct interpretation and negative evidence against the wrong interpretation. Another explanation is that the Contrastive Feedback condition is the only one that provided additional exposure to the target form with each instance of feedback. However, it seems unlikely that mere exposure to a cross-category vowel shift would induce learning by itself in the absence of some sort of disambiguating information, as the lack of learning in the Control condition attests.
Although the contrastive corrective feedback did improve word identification during the interaction, some listeners never accommodated the vowel shift despite the feedback. Also, contrary to our expectation, almost no listeners in the Generic Corrective Feedback condition demonstrated any uptake. This calls to mind Mackey et al.’s (Reference Mackey, Gass and McDonough2000) point that the way L2 learners perceive interactional feedback is not always in line with what their dialogue partner intended to communicate. In our case, listeners may not have interpreted the generic corrective feedback as reflecting their mistaken perception. Rather, they might have assumed that they heard right but that their partner had misspoken, or that they were told the wrong word because they gave the wrong answer to the puzzle. Moreover, repeated provision of generic feedback was arguably unnatural from a pragmatic standpoint because a cooperative interlocutor would make their remarks more specific over time or perhaps even adapt their own pronunciation in order to avoid repeated misunderstandings. While such ambiguities about feedback would not arise in a form-focused perception training program, they may occur often in interactive communication. Interestingly, Lee and Lyster’s classroom-based study (Reference Lee and Lyster2016a) implemented a two-step feedback protocol, first providing implicit feedback (repeating the wrong word with question intonation) and following it up when necessary (if learners did not make self-repairs) with explicit feedback similar to our study’s contrastive corrective feedback. The fact that their learners did not always seem to understand the initial implicit feedback aligns with our finding that generic feedback about speech perception may, in some contexts, be too ambiguous to learn from.
Effects of feedback and lexical guidance on lexical processing
The second research question was whether corrective feedback and lexical guidance, or uptake resulting from these factors, would contribute to faster and more accurate online processing of the accented speech, as measured by a lexical decision task. Results showed that for critical accented words (e.g., “best” pronounced as /bɪst/), online processing was faster for participants receiving lexical guidance than for participants in the other three conditions, as evidenced by faster reaction times to accept these items as real words. Additionally, lexical processing was both faster and more accurate (in terms of acceptance rates) for listeners who had exhibited greater uptake, as operationalized by their word identification accuracy during critical Code Breaker trials. These results align with those of Maye et al. (Reference Maye, Aslin and Tanenhaus2008), who found that accented words that originally sounded like nonwords came to be more often interpreted as real words after exposure to a vowel shift in a story context. In our study, the fact that the online processing of accented words was more robustly affected by prior uptake, rather than being directly affected by condition, suggests that listeners’ conscious word recognition during Code Breaker played a crucial role in automatizing their knowledge of the vowel shift. In other words, online lexical processing changed only to the extent that listeners had interpreted the accented words correctly during the previous communicative task.
Interestingly, the lexical decision task showed no significant effects of condition or uptake for Critical Pseudowords (e.g., *“geft” pronounced as /gɪft/), which were in fact nearly universally accepted as words by all participants (e.g., /gɪft/ was treated as “gift”). Thus, even if listeners had learned that /ɪ/ could represent /ε/, they did not learn that it must represent /ε/. This lack of learning effect for items that sounded like real words is also consistent with the results of Maye et al. (Reference Maye, Aslin and Tanenhaus2008). They found that items that were perceived as real words before exposure to a novel accent were still judged as real words after exposure, even when the exposure had contained evidence that the vowel was involved in a chain shift (e.g., /wɪtʃ/ or “witch” was perceived as a real word both before and after accent exposure, even though the /i/-to-/ɪ/ shift in the exposure implied that /wɪtʃ/ should correspond to the nonword “weech”). Overall, our results indicate that even if listeners did adapt to the vowel shift, they did not completely remap their vowel space but simply increased their tolerance for nonstandard pronunciations (i.e., allowing /ɪ/-like pronunciations of /ε/).
The interactive context for L2 sound learning
The third research question was whether perceptual learning would differ between the two communicative settings: interacting with a computer player (resembling traditional lab-based phonetic training studies) and interacting with a face-to-face interlocutor (resembling naturalistic interaction). Across all results, no significant differences in perceptual learning between the settings were observed. While we cannot draw strong conclusions from the lack of a difference, especially given the modest effect sizes, this does suggest that the perceptual learning mechanisms under study can be generalized to a more natural communicative context than what is traditionally studied in the field of L2 perceptual learning.
The fact that lexical guidance in interactive conversation improved online perceptual processing shows that this type of implicit perceptual learning can occur even when cognitive processing demands are relatively high. Not only did participants have to solve puzzles on every turn, they also engaged their L2 speech production system repeatedly to communicate the answers. While previous research found that alternating speaking and listening could interfere with perceptual learning (Baese-Berk, Reference Baese-Berk2019; Baese-Berk & Samuel, Reference Baese-Berk and Samuel2016; Leach & Samuel, Reference Leach and Samuel2007), the present findings show that significant learning can still take place in such interactive conditions. Our study was not specifically designed to test the effect of cognitive load on perceptual learning. However, other researchers have found that speaking with a physically co-present interlocutor involves a higher cognitive processing load than speaking in response to prerecorded utterances (Sjerps et al., Reference Sjerps, Decuyper and Meyer2020). Thus, our experiment’s face-to-face setting might well have induced a higher processing load than the computer player setting, yet still it led to equivalent perceptual learning. Finding comparable learning effects in two different interactive settings, even in a task with relatively high processing demands, supports the viewpoint that conversational interaction is a beneficial context for L2 learning (Ellis, Reference Ellis and Ellis1999, Reference Ellis2003; Long, Reference Long1980, Reference Long, Ritchie and Bhatia1996); moreover, we have now extended interactionist research to the area of L2 speech perception.
One important issue raised by our study is the role of explicit and implicit learning in perceptual adaptation to a novel L2 accent, as the contribution of these two types of learning to L2 acquisition is a question of interest to the field (Hulstijn, Reference Hulstijn2005). The present findings suggest that what matters for perceptual learning, whether it occurs explicitly or implicitly, is the extent to which the listener reaches the right interpretation of the spoken words during the learning phase. In our Lexical Guidance condition, the onscreen text was a reliable cue to the proper interpretation of the interlocutor’s word. Before the interlocutor spoke (e.g., saying /lɪft/), participants could already rule out the incorrect default interpretation (e.g. “lift”) because it was absent from their onscreen answer options; this made it easy to choose the right word (e.g. “left”) despite the accented vowel. This high word identification accuracy during the interaction, confirming that participants mapped the ambiguous pronunciations to the correct lexical items, was linked to improved online processing in the subsequent lexical decision task. As lexically guided perceptual learning is an automatic process (McQueen et al., Reference McQueen, Norris and Cutler2006b), it appears that mere exposure to vowel shift in the context of the disambiguating lexical information was enough to trigger perceptual adjustments, without interpretational difficulties playing a role. In the Corrective Feedback conditions, however, listeners were much less likely to interpret their partner’s words correctly during the interaction, even after receiving repeated negative feedback in response to their perceptual errors. Thus, the corrective feedback, especially the generic feedback, was apparently not a reliable cue to interpreting the interlocutor’s accent. This finding mirrors classroom-based studies (e.g., Mackey et al., Reference Mackey, Gass and McDonough2000, Reference Mackey, Al-Khalil, Atanassova, Hama, Logan-Terry and Nakatsukasa2007) showing that the linguistic target of corrective feedback is not always perceived by learners. Overall, our study suggests that the cues that drive implicit perceptual learning, like lexical constraints, may sometimes be more effective than the cues used in explicit perceptual learning, like corrective feedback, if the implicit cues yield a more reliable interpretation. Having to consciously process interactional feedback creates more room for ambiguity in interpretation due to any number of social and pragmatic factors, especially when the feedback is relatively generic in form.
Furthermore, our results suggest that conscious awareness is beneficial for L2 sound learning, supporting a weak version of the noticing hypothesis (Schmidt, Reference Schmidt and Robinson2001). Although listeners in the Lexical Guidance condition only had implicit cues to learn from, they very likely noticed that their interlocutor had a nonstandard accent that they needed to adapt to, as the Code Breaker game encouraged them to choose words that mismatched their default interpretation (e.g., choosing “left” when hearing /lɪft/). Moreover, for corrective feedback to be effective, it was crucial that it explicitly highlighted the gap between the speaker’s intended word and what the participant had mistakenly perceived. The positive relationship between listeners’ uptake and their subsequent online processing implies that listeners who noticed these mismatches, interpreted them as reflecting their own mistaken perception, and adjusted their responses accordingly were the ones who subsequently became faster and more accurate at processing accented words.
Future directions and conclusions
The limitations of the present study suggest several interesting avenues for future research. First, this study only examined short-term perceptual learning based on a relatively brief dialog with a single accented speaker. A single interactive session may not have been sufficient to produce robust learning, particularly since the amount of feedback that could be given was limited by pragmatic and methodological considerations. Thus, the potential for more robust learning effects from repeated or prolonged interaction merits further study. Moreover, while we limited the prerecorded speech to that of a single speaker to maximize phonetic control, it would be useful to test how well the present results generalize to other voices and accents. This study focused on learning a novel L2 accent involving familiar vowels, but future research should also examine how interaction facilitates perceptual learning of L2 phonetic contrasts not present in the L1 phonemic repertoire. Additionally, given the substantial individual variability we observed in listeners’ receptivity to corrective feedback, it would be interesting for future research to investigate whether factors such as proficiency can explain L2 learners’ variable success in perceptual learning from interaction.
In conclusion, the main finding of this study is that L2 listeners can use corrective feedback and lexical guidance in conversation to perceptually adapt to a vowel shift in an unfamiliar accent, improving both their word identification accuracy and their online processing of accented words. Specifically, L2 listeners’ word identification accuracy was shown to improve over the course of the interaction when their dialogue partner responded to perceptual errors with corrective feedback that explicitly contrasted the perceived word and the intended word. Their accuracy did not improve if they only received generic feedback, highlighting the importance of clear interpretability for interactional feedback to effectively promote uptake. The study also demonstrated that after the dialogue, L2 listeners’ online processing of accented words was faster if, during the dialogue, onscreen lexical information had implicitly constrained their interpretation of the interlocutor’s words. Moreover, individual differences in the amount of uptake for the accent during the dialogue significantly predicted both the speed and accuracy of post-dialogue lexical processing. Finally, as our phonetically controlled experimental paradigm yielded comparable learning effects in both computer-based and face-to-face interactive settings, these results can likely be generalized to a more naturalistic L2 acquisition context.
Supplementary material
To view supplementary material for this article, please visit https://doi.org/10.1017/S0142716421000205.
Acknowledgments
This research was supported by a Vidi grant from the Dutch Research Council (NWO) awarded to Mirjam Broersma.
Appendix A
Table A1. Code Breaker critical minimal pairs
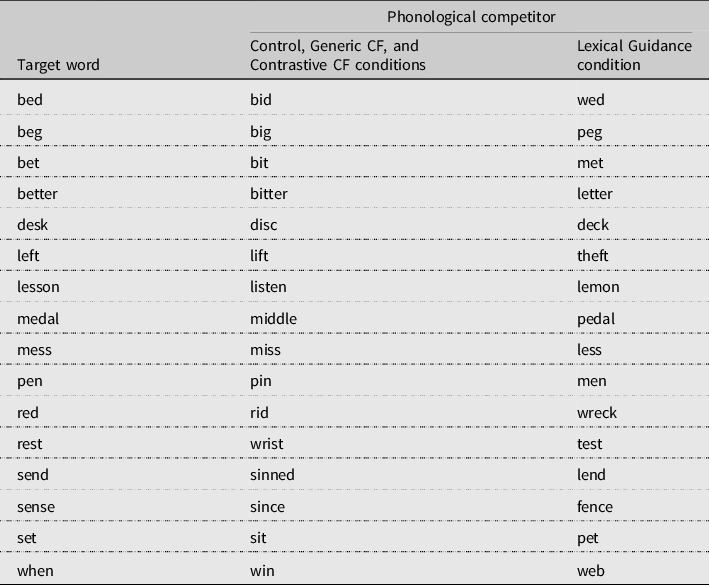
Note. CF = corrective feedback.

Figure A1. Three Sample Code Breaker Puzzles, Ranging in Difficulty from Easier (First Row) to Harder (Last Row).
Appendix B
Table B1. Auditory lexical decision task items
