Introduction
More diagnostic effort is recommended by the Alzheimer’s society because early recognition of dementia allows for timely interventions and better quality of life for the patients (Borson et al., Reference Borson2013). However, the (clinical) reality has its limitations.
The diagnosis of patients with suspected mild dementia (MD) is challenging, and the number of patients continues to rise.
It is difficult to differentiate who has MD based on anamnesis alone. Subjective complaints and reports from informants often do not correspond to objective impairments (Schouws et al., Reference Schouws2012; Pendlebury et al., Reference Pendlebury2015; Ryu et al., Reference Ryu2020).
Specialized diagnostic facilities are needed but will become overloaded by the number of referred patients in the near future. Most countries already have diagnostic challenges (Alzheimer’s Disease International, 2018), including a lack of financial or staff resources for a time-consuming comprehensive neuropsychological assessment (NPA). An accurate short screening test to identify patients with a (high) risk of MD, i.e. those in need of an NPA, is therefore necessary. A difficulty is who is to be considered at risk as definitions for disease (e.g. MD) do not always define health at the same time and thereby create subthreshold disorders (Helmchen and Linden, Reference Helmchen and Linden2000). Cognitive functioning is a state on a continuum with dementia on one end and no cognitive impairment (NoCI) on the other end of the extremes. Classifications define these states, therefore creating double thresholds. In-between, there is an area in which the patient is in an intermediate state and at risk, e.g. mild cognitive impairment (MCI), of which approximately 40% worsens, 40% stabilizes, and 20% recovers (Gauthier et al., Reference Gauthier2006; Julayanont et al., Reference Julayanont2014; Canevelli et al., Reference Canevelli2016).
Given the wide range of outcomes of MCI and the large numbers involved, it is essential to be able to differentiate patients with MCI from those with MD and NoCI (Gauthier et al., Reference Gauthier2006). In particular, in an old-age psychiatry setting, there is a high correlation between psychiatric conditions (American Psychiatric Association, 2013) (including psychotropic medication and substance abuse) and MCI that does not necessarily worsen over time (Julayanont et al., Reference Julayanont2014). These MCI cases deserve their own policy. An elaborate diagnostic route (including biomarkers/MRI) is often not yet necessary, but they should not be discharged either. An NPA comes to mind as a compromise. However, limited resources warrant the restraint of false-positive (FP) referrals for an NPA to avoid potential harm due to unnecessary emotional and financial burden (Borson et al., Reference Borson2013; Burn et al., Reference Burn2018; Davis et al., Reference Davis2015). Although early identification of neurocognitive disorders is advocated spending resources wisely is as important, giving the ones the most in need priority (Alzheimer’s Disease International 2018 ; Borson et al., Reference Borson2013). This implies that patients at highest risk (taking into account the age or speed of onset in combination with the degree of impairment) should be referred for an elaborate specialized diagnostic route. The cognitive functioning of patients considered to be at lower risk (e.g. with psychiatric disorders) should be assessed in the best available way, depending on the resources. When the scarce and time-consuming gold standard, i.e. an NPA, is less available the assessment of the cognitive functions could be done with a short, validated test. In our opinion, this should include reassessment with this test, as it is easy to perform and takes limited time to administer, i.e. active monitoring. Therefore, it is important to use screening instruments that can detect both MD and MCI.
The Montreal Cognitive Assessment (MoCA) was developed as a short screening tool for MCI and MD (Nasreddine et al., Reference Nasreddine2005) and validated in at least 35 different languages and even more settings. Most of these studies can be found on the MoCA-test website (mocatest.org). At the original proposed cutoff of <26, the sensitivity for correctly screening patients with MCI (90%) and MD (100%) is very good (Nasreddine et al., Reference Nasreddine2005). Although it has been repeatedly shown to be superior to the MMSE in identifying MCI (Folstein, et al., Reference Folstein, Folstein and McHugh1975; Pinto et al., Reference Pinto2019), the MoCA still has its limitations as a triaging tool. Its ability to identify people with NoCI (specificity) is criticised in clinical practice because specificity varies due to clinical and demographic reasons (Davis et al., Reference Davis2015). Frequently reported examples are age, education, rural environment, ethnic or cultural background (including race in some countries), substance abuse, and psychiatric diseases (mocatest.org). It is repeatedly suggested to lower the cutoff with higher specificity as a result (O’Driscoll and Shaikh, Reference O’Driscoll and Shaikh2017; Carson et al. Reference Carson, Leach and Murphy2018; Dautzenberg et al., Reference Dautzenberg, Lijmer and Beekman2020). Nevertheless, the MoCA with a lower cutoff is still not suitable for identifying MD as a stand-alone assessment of referred patients to an old-age psychiatric clinic (Dautzenberg et al. Reference Dautzenberg, Lijmer and Beekman2020; Korsnes, Reference Korsnes2020), or as an assessment of referred patients to its memory clinic (Smith et al. Reference Smith, Gildeh and Holmes2007; Dautzenberg et al., Reference Dautzenberg, Lijmer and Beekman2021) because the positive predictive value (PPV) is never sufficient (Carson, et al., Reference Carson, Leach and Murphy2018). Its high sensitivity makes it a good screener, finding most MD patients. The high negative predictive value (NPV) for appropriately discharging NoCI patients, is promising, although for triaging those who need a scarce NPA, moderate specificity gives too many FPs.
Double cutoffs are reported in the literature as a solution by using one threshold for health and one for disease (Batelaan et al., Reference Batelaan2007; Swartz et al., Reference Swartz2016; Landsheer, Reference Landsheer2020; Thomann et al., Reference Thomann2020). Especially where classifications create subthreshold disorders, regardless of whether these are disorders in their own right or are merely (minor) forms of major disorders (Batelaan et al., Reference Batelaan2007). Either way MCI is not (yet) dementia.
A double-threshold MoCA offers the possibility to distinguish clinical and subclinical states according to their appropriate domain and thus to implement different policies. Previous studies have shown that almost no patients with MD or even MCI will score ≥26, the originally proposed cutoff of the MoCA, therefore indicating health (i.e. NoCI) (Nasreddine et al., Reference Nasreddine2005; Davis et al., Reference Davis2015; Carson et al., Reference Carson, Leach and Murphy2018; Dautzenberg et al., Reference Dautzenberg, Lijmer and Beekman2020, Reference Dautzenberg, Lijmer and Beekman2021; Korsnes, Reference Korsnes2020). The number of FPs below this cutoff is too large to have a full work-up. ‘Reducing the risk of FP is important (Davis et al., Reference Davis2015). In a memory clinic setting, half of the depressed patients scored below 26 (Blair et al., Reference Blair2016; Dautzenberg et al., Reference Dautzenberg, Lijmer and Beekman2021). Other studies showed that the majority of patients with affective, psychotic, or neurotic disorders scored between 20 and 26 on the MoCA, while the majority of the organic disorders scored <19 (Gierus and Mosiolek, Reference Gierus and Mosiolek2015; Dautzenberg et al., Reference Dautzenberg, Lijmer and Beekman2021; Korsnes, Reference Korsnes2020). By using a lower (second) cutoff for referral (using the highest Youden index for MD in this cohort; <21) (Dautzenberg et al., Reference Dautzenberg, Lijmer and Beekman2020), FPs will decrease, but this will also increase the FNs. This could be compensated for by actively monitoring all these patients with a score from 21 to 26, reducing unnecessary referrals (FP), but still allowing those patients at high risk of MD to be monitored (FN).
A recent study by Landsheer demonstrated that using a double threshold for the MoCA improves clinical classification and that using an uncertainty interval (21 to 26) reduces the effect of prevalence on MoCA performance (Landsheer, Reference Landsheer2020).
Other studies on double thresholds aimed to improve classification accuracy by stratifying the population based on normative data (Oren et al., Reference Oren2014; Tan et al., Reference Tan2014; Borland et al., Reference Borland2017) or stratifying the outcome, of certain or uncertain test results (Swartz et al., Reference Swartz2016; Landsheer, Reference Landsheer2020; Thomann et al., Reference Thomann2020). They do not separate the three distinct cognitive states, i.e. MD versus MCI versus NoCI.
In our study, however, we wanted to introduce three policies, matching the three diagnostic entities of cognitive functioning, to improve the MoCA’s potential as a triaging tool.
Although the use of the MoCA in this way feels intuitive, to our knowledge, no results have been presented before on the MoCA with a double threshold separating all three distinct stages and analyzing the consequences of subsequent policies.
Especially in old-age psychiatry, there are many inconclusive MoCA scores due to age (60+) and psychiatric comorbidities (including psychotropic medication), i.e. from 21 to 26. Therefore, we studied the policy of ‘active monitoring’ in this population as an intermediate option.
We used data from our cohort of an old-age psychiatric setting (Dautzenberg et al., Reference Dautzenberg, Lijmer and Beekman2020, Reference Dautzenberg, Lijmer and Beekman2021), where referrals, at least in the Netherlands, include patients with cognitive, behavioral, and psychiatric symptoms, that may result from neurodegenerative diseases, but also from other psychiatric disorders. The standard is that after an initial assessment, it is decided who could benefit from an extensive cognitive diagnostic route at our memory clinic.
We aim to demonstrate the advantages of using a double-threshold MoCA to triage patients in need of an NPA. Therefore, we compare different selection strategies, including the double threshold, to efficiently select patients in need of an NPA (i.e. MD), those who are not (NoCI), and patients who should be actively monitored (MCI). We rate the strategies according to their accuracy and the number of referrals for an NPA that result in as few FNs as possible.
The compared selection strategies for referral to an NPA are as follows: an initial assessment only (without the use of an objective test, i.e. the MoCA), the MoCA as a stand-alone (i.e. without clinical judgment), or the MoCA as an add-on after the initial assessment (i.e. as a two-stage screener). The MoCA strategies are compared when using single and double thresholds.
Methods
Patients
The cohort (n = 693) was taken from a previously reported validation study of the MoCA for patients referred to an old-age psychiatric service in Utrecht, the Netherlands, as described in detail elsewhere (Dautzenberg et al., Reference Dautzenberg, Lijmer and Beekman2020). In short, all newly referred patients to our clinic were eligible for the study if they were capable of giving written informed consent. Therefore, patients with severe dementia (Global Deterioration Scale (GDS) ≥6) (Reisberg et al., Reference Reisberg1982) and Behavioral and Psychological Symptoms of Dementia (BPSD) as a reason for referral and compulsory referrals were not eligible (n = 1337). To resemble a clinical screening population, Standards for Reporting Diagnostic Accuracy (STARD/STARDdem) (Noel-Storr et al., Reference Noel-Storr2014; Bossuyt et al., Reference Bossuyt2015) require we excluded patients with an obvious diagnosis of dementia (GDS ≥5), a recent history of substance abuse (<1 year), delirium (<6 months), or acquired brain injury including CVA or TIA (n = 174). The patients suspected of having cognitive impairment after the initial assessment were referred to our memory clinic (n = 290) (Figure 1). All of these patients underwent a comprehensive cognitive diagnostic route for cognitive impairment using a consensus-based diagnosis, following international criteria as a reference standard with an NPA and when applicable, CT/MRI- imaging and cerebrospinal fluid (CSF) analysis (CBO Geriatrie, 2014). They were classified as MD, MCI (including psychiatric etiologies), or NoCI (including subjective complaints, mostly psychiatric patients without objective cognitive impairment).

Figure 1. Flowchart and results of the different selection strategies.
MoCA: Montreal Cognitive assessment.
NPA: comprehensive NeuroPsychological Assessment.
FP: False Positive; FN: False Negative; TP: True Positive; TN: True Negative.
*FN during follow up.
For the strategic selection route comparison, we included all eligible referred patients (n = 693), including those not suspected of cognitive impairment (n = 403). The latter were followed for at least 2 years to compensate for not having an NPA to exclude conversion to any DSM IV/5 (American Psychiatric Association, 2000, 2013) cognitive diagnoses after the initial assessment. Three of them were diagnosed with cognitive impairment during follow-up and were considered inconclusive as it is not certain if these impairments manifested before or after initial assessment. To be conservative, we classified these three as FN MD.
All participants gave their informed consent. Data are available upon request.
Measurements
Initial assessment
The initial assessment was completed by old-age psychiatrists (n = 4, having at least 8 years of practical experience in 2008) and included a laboratory test, medical, and functional history from a next of kin and an investigation of Instrumental Activities of Daily Living (IADL) performed by a psychiatric nurse practitioner with a home visit. The 15-item Geriatric Depression Scale (GDS15) (Yesavage and Sheikh, Reference Yesavage and Sheikh1986) and the Global Assessment of Functioning (GAF) (American Psychiatric Association, 2000) were also administered during this time.
Diagnostic test
All of the referred participants were assessed with an MoCA as soon as possible but within 3 months of referral by a trained psychiatric nurse practitioner. This was independent of the diagnostic procedure.
The MoCA consists of one page that covers the cognitive domains of executive function and visuospatial abilities, naming, short-term memory, attention and working memory, language, concentration, verbal abstraction, and orientation. It can be administered within 10 minutes and the maximum score is 30, which indicates that no errors were made. Correction for low education effects was done, according to the instructions, by adding one point to the total of patients with 12 years of education or less. The suggested best cutoff for the diagnosis of dementia was a score of 21 (<21) and <26 for MCI, in both old-age psychiatry and memory clinic settings (Dautzenberg et al., Reference Dautzenberg, Lijmer and Beekman2020, Reference Dautzenberg, Lijmer and Beekman2021).
Reference test
The reference test was the diagnosis determined at multidisciplinary meetings, including an old-age psychiatrist, neuropsychologist, and geriatrician. The diagnosis of MD, MCI, or NoCI was supported with at least a 4-h NPA. The NPA included multiple tests in the domains of memory, attention, executive function, fluid intelligence, and language capacities (for details please see Dautzenberg et al., Reference Dautzenberg, Lijmer and Beekman2020). The diagnoses were made in consensus and in accordance with the MCI criteria as proposed by an international consortium (Winblad et al., Reference Winblad2004; Gauthier et al. Reference Gauthier2006), or the Dutch guideline on dementia (CBO Geriatrie, 2014). This guideline covers the criteria of -DSM IV/5 and the international criteria for dementia. The results of the MoCA were not used to diagnose MCI or dementia. The Dutch translation of the DSM 5 was introduced in the Netherlands at the end of the study of the cohort (2017). All patients were classified according to DSM IV for the purpose of this study.
Statistical analyses
Statistics
The demographic results (Table 1) were compared within the patients with MD, MCI, or NoCI using Statistical Package for the Social Sciences (SPSS, version 22; SPSS Inc., Chicago, IL); Chi2 test to compare sex and education. ANOVA was used to compare age, GAF, GDS15, and MoCA scores followed by a least significant difference post hoc test.
Table 1. key demographic and clinical characteristics

Groups a,b and c were compared with ANOVA, education and sex were compared with a Chi2 test.
MCI: Mild Cognitive Impairment; NoCI: No Cognitive Impairment; GAF: Global Assessment of Functioning; GDS15: Geriatric Depression Scale 15 question version; MoCA: Montreal Cognitive Assessment.
The previously reported area under the curve calculations using receiver operating characteristic analysis were used to find the best cutoff scores (Dautzenberg et al., Reference Dautzenberg, Lijmer and Beekman2020, Reference Dautzenberg, Lijmer and Beekman2021) for both settings (Table 2).
Table 2. Area Under the Curve between variations of groups and their sensitivity and specificity at cutoff scores 26 and 21, often used in literature. Stand-alone (n = 693) or add-on (n = 290)

MD: Mild Dementia; NoDem: No Dementia (MCI+NoCI); MCI: Mild Cognitive impairment; NoCI: Referred patients no Cognitive Impairment; CI; Cognitive Impairment (Dem + MCI).
Stand-alone (n = 693): all referred patients without judgement of initial assessment.
Add-on (n = 290): only those patients suspected of CI after initial assessment. All referred to memory clinic.
We reported the FPs, false negatives (FNs), true positives (TPs), and true negatives (TNs) of the different selection strategies to judge the clinical effects (Figure 1). The positive predictive value (PPV = TP/(TP+FP)), negative predictive value (NPV = TN/(TN+FN)), and accuracy (ACC=(TP+TN)/(TP+TN+FP+FN)) were calculated (Table 3). However, with these indicators, it is impossible to weigh the FN and FP rates separately, which is a disadvantage, and absolute quantities can provide more insight when diagnostic routes are compared (Glas et al., Reference Glas2003). Therefore, we also expressed the results of the selection strategies in absolute numbers (Figure 1, Tables 3 and 4) of patients who were to be referred (Figure 1; columns A, E), those who were to be observed (B, F), who were not (C, G), and the reference diagnoses (D). As the purpose of the study is to reduce the number of FP referrals without discharge a MD patient, we considered the observed MD, MCI, and NoCI as TP, TN, and TN, respectively. The calculation of the indicators for the two-stage strategy included the effects of the initial assessment (e.g. adding the 400TN and 3*FN to columns E, F, G).
Table 3. results of the selection strategies
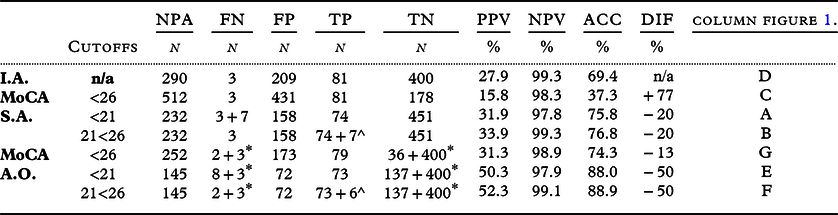
*including FN/TN of Initial Assessment.
^including the observation group.
I.A.: Initial Assessment. MoCA: Montreal Cognitive Assessment. S.A.: stand-alone. A.O.: add-on; NPA: referred for a Neuropsychological assessment. FN: False negative. FP: False Positive. TP; True Positive.TN: True Negative. PPV: Positive Predictive Value. NPV: Negative Predictive Value. ACC: Accuracy. DIF: difference in referrals compared to I.A.
Table 4. (a, b, c and d): cross tables of the different strategies

Results
Demographic findings
The main demographic and clinical characteristics of the study group are listed in Table 1.
The significant differences in age and sex are representative of the demographics of an old-age psychiatry setting and are substantiated elsewhere (Dautzenberg et al., Reference Dautzenberg, Lijmer and Beekman2020).
The GAF score was the highest in the MCI group, as expected because these patients have the least severe symptoms, given the high number of MCI patients without any other psychiatric conditions (50%), whereas almost all patients in the NoCI had one or more psychiatric diagnoses (Dautzenberg et al., Reference Dautzenberg, Lijmer and Beekman2020).
Single threshold
The initial assessment resulted in 290 referrals for NPA (Figure 1, column D). An accuracy of 69% was achieved for detecting MD (Table 3), with three FNs. Using a single MoCA threshold of <21 to select those requiring an NPA resulted in a decrease in referrals, but an increase in FNs compared to the initial assessment (columns A and E). However, this resulted in an improvement in their accuracy (Table 3). When the MoCA was used as an add-on to screen the ‘patients not suspected after initial assessment’ (n = 403: Table 4d, calculations not shown), the accuracy deteriorated to 57% by adding 87 referrals to the initial set of 290 referrals, resulting in a total of 377 referrals (including 297 FPs).
Comparing the PPVs and NPVs of the different selection strategies at a cutoff <21 for MD, we found a substantial increase in PPV and only a slight decrease in NPV when the MoCA was used as an add-on.
When the cutoff was raised to <26 for detecting MD per strategy (columns C, G), the FNs decreased but with a substantial increase in referrals, which decreased the PPV and ACC.
Double threshold
The single threshold dichotomy reported above had a binary outcome: referral for NPA or no referral. This is not consistent with clinical practice, where an intermediate strategy of ‘keeping the patient under observation’ or ‘active monitoring’ is often used.
The use of two cutoff scores: <21 invitation for NPA, 21≤ active monitoring <26; no follow-up ≥26 gave a more differentiated result (columns B, F).
The double-threshold MoCA as an add-on for suspected patients after initial assessment (column F) resulted in 5(2+3*) instead of 11 MD (column E) patients with MD not undergoing a comprehensive diagnostic route, without the increase in FP referrals. This resulted in the highest accuracy (89%), PPV (52%), and NPV (99%). However, it would mean that 107 patients need to be reassessed.
Discussion
Limited diagnostic resources and rising patient numbers present challenges in MD diagnostic procedures. It is necessary to differentiate patients to focus scarce diagnostic resources on those who need them most. Therefore, we compared different strategies, including a double-threshold MoCA. Our results confirm that an objective test can have added value. However, how and when the MoCA was used gave different results.
All the strategies we tested were able to find most of the patients with MD. Judgment by only the TP/FN is not sufficient, as the FPs differs substantially (Figure 1/Table 3). Using merely initial assessment (column D) gave the highest TP of the compared strategies, but the high amount of FPs complicates the diagnostic route: still 42% of all assessed patients were referred for a comprehensive diagnostic route. This is probably because clinicians try to avoid FNs. Especially without the assistance of an objective test, clinicians tend to refer subthreshold states earlier.
The single cutoff MoCA strategy did not solve the (sub)threshold dilemma. Although the low PPV and the very high NPV underscore that only an MoCA score above the cutoff (i.e. a negative MoCA) should be considered reliable and thus suitable to adjust clinical judgment, i.e. initial assessment (Table 4d).
Using an MoCA as a second-stage screener with a score of 21 or higher (cutoff <21) to adjust initial assessment (column E) reduced the FP referrals for an NPA by 65% (to n = 72 of 290) but increased the FN by 3.6 times to 13% of MD (n = 11).
Using a double threshold with scores <21 identifying patients suitable for an NPA and ≥26 for discharging patients, i.e. indicating patients do not need an NPA or reassessment, not only gave the best results but also achieved two goals simultaneously. Not only compensating for the increase in FNs by monitoring most of the missed MDs but also at-risk intermediate state patients (MCIs), without increasing the number of referrals.
How to classify the different strategic selection outcomes for the accuracy calculations can be debated and depends on the setting and its target disease. Introducing a double threshold together with an intermediate state raises the dilemma of what is to be considered TP/FP or TN/FN. As these cells only exist in a 2x2 classification table, in our study, we created a 3x3 table (Table 4b,c,d).
In addition to this theoretical classification problem, there is a clinical classification dilemma. There are multiple reasons to advocate (TP) or to be cautious (FP) with early MCI referrals, and the debate is ongoing. Because of limited access to NPAs in most countries or rural areas and because MCI can also consist of etiologies from which a patient can recover, we considered MCI an FP when a patient was referred for a comprehensive diagnostic route (therefore, MCI automatically became a TN for observation and discharging). Even though we understand that, with unlimited resources, one could consider (some of) the MCI-patients as TP when referred, as quality of life can improve by cognitive testing (Janssen et al., Reference Janssen2019). Identifying this intermediate state to actively monitor MCI without giving them this demanding diagnostic route is another justification for using a double threshold. Intuitively, we would consider 21≤MCI<26 as TP (for monitoring); however, technically this is not possible, as MCI is already labeled as an FP when a patient has a score <21.
For dementia, a short assessment that differentiates MD from MCI with certainty would be preferred, but such a test is still not available. This is also true for the MoCA, as our results showed that our best PPV is still too low (52%) for a conclusive classification. Selecting those patients in need without missing one MD in the best way possible, without referring too many who are not (yet) in need of a memory clinic, is essential, i.e. triaging. These requirements were translated into our evaluation criteria of low absolute referral rates while still maintaining the highest possible sensitivity, i.e. no FNs and low FPs. Therefore, we judged the strategies by these values.
Of the strategies selecting MD, the double threshold add-on not only gave the highest accuracy (89%), PPV (53%), NPV (99%), and the lowest FPs with still acceptable FNs, it also creates the opportunity to monitor MCI and seems the preferred selection route (Table 3, column F).
In addition to the accuracy calculations of our clinical example addressing the three cognitive entities as well as possible to their appropriate policy, as debated above, there are more arguments to be found in literature to use a double threshold for the MoCA.
First, a substantial number of MCI patients scoring below <21 on the MoCA are at very high risk of converting to MD, while those above this cutoff are considerably less at risk (Smith et al., Reference Smith, Gildeh and Holmes2007; Julayanont et al., Reference Julayanont2014; Dautzenberg et al., Reference Dautzenberg, Lijmer and Beekman2021).
Second, a double threshold for the MoCA also reduces the ‘uncertain test scores’ due to ‘random classification errors’. These outcomes result from the distribution of the different diagnostic groups in the middle range MoCA scores (Landsheer, Reference Landsheer2020; Thomann et al., Reference Thomann2020). By applying an uncertainty interval, as these MoCA scores are the most error prone, the PPV and NPV improve in the studied prevalence, and become less dependent on the setting. Even if their study objective was not to identify the subthreshold state, the implementation is similar; applying an (uncertainty) interval improves the accuracy of the MoCA.
Third, as mentioned in the introduction, several variables are found to be of importance in different clinical populations, and these can lead to an inflated rate of FPs particularly older age and lower education (Carson et al., Reference Carson, Leach and Murphy2018; Thomann et al., Reference Thomann2020). Lifestyle and physical activity are found to significantly influence MoCA scores even more than age and education (Ihara et al., Reference Ihara2013; Innocenti et al., Reference Innocenti2017). Although education (Wong et al., Reference Wong2015; Borland et al., Reference Borland2017; Pinto et al., Reference Pinto2018), ethnicity (Rossetti et al., Reference Rossetti2011; Tan et al., Reference Tan2014; Wong et al., Reference Wong2015), race (Goldstein et al., Reference Goldstein2014; Tan et al., Reference Tan2014; O’Driscoll and Shaikh, Reference O’Driscoll and Shaikh2017), and (rural)habitat (Goldstein et al., Reference Goldstein2014; Hilgeman et al., Reference Hilgeman2019) are known factors, others debate that these factors are better represented by ‘literacy in late life’ (Sisco et al., Reference Sisco2015). More important for our setting are the negative influence of substance abuse (Rojo-Mota et al., Reference Rojo-Mota2013; Pugh et al., Reference Pugh2018) or psychiatric diseases (Musso et al., Reference Musso2014; Gierus and Mosiolek, Reference Gierus and Mosiolek2015; Blair et al., Reference Blair2016; Srisurapanont et al., Reference Srisurapanont2016; Wu et al., Reference Wu, Dagg and Molgat2017; Korsnes, Reference Korsnes2020).
The above enumeration shows that there are many reasons for heterogeneity affecting the MoCA score. It shows that a single cutoff rarely fits a pluriform clinical practice where many covariates influence the individual MoCA score. A single cutoff is associated with substantially high rates of misclassification. Stratification was suggested for age and education as a solution (Oren et al., Reference Oren2014; Wong et al., Reference Wong2015; Borland et al., Reference Borland2017). However, stratification of patients is impracticable if one needs to take all the possible confounders into account.
Psychiatric comorbidities, such as depression or mania, only substantially influence the MoCA score in some individuals (Gierus and Mosiolek, Reference Gierus and Mosiolek2015; Blair et al., Reference Blair2016; Dautzenberg et al., Reference Dautzenberg, Lijmer and Beekman2021; Korsnes, Reference Korsnes2020), making beforehand stratification of these patients infeasible. This underscores that the MoCA should not be used as a stand-alone or overrule but should help clinical judgment as an add-on by knowing its strength (NVP, sensitivity) and weakness (PPV, specificity).
A strength of our study is that possible psychiatric causes of CI were not excluded, as (cloaked) psychiatry is the clinical reality in most settings, especially in old-age psychiatry. Another strength is that we used a clinical cohort setup by avoiding the extremes of the spectrum, such as community-based healthy controls, and severely demented including those with BPSD, following the STARDdem recommendations. Therefore, the cohort consisted of patients a clinician would consider screening for CI. Including severe dementia would give better results due to a higher dementia prevalence and lower MoCA scores, but this is not the clinical reality.
Our setting, on the other hand, is also a limitation, as referrals with BPSD and MCI caused by psychiatric etiology will be higher than those in nonpsychiatric settings and will influences the prevalence of MD. However, our previous study showed that the mean MoCA score did not differ between neurodegenerative and psychiatric etiologies of MCI (Dautzenberg et al., Reference Dautzenberg, Lijmer and Beekman2021).
A limitation of our study is the uncertainty of the number of FNs after initial assessment. The consecutive cohort design resulted in ‘unsuspected patients after initial assessment’ (n = 403) not receiving an NPA due to practicality and resource constraints. We minimized this flaw by following these patients for at least 2 years. Three out of 403 unsuspected patients progressed to CI, most likely new cases. This corresponds with the incidence of 6.6 males or 7.4 females in the 74–79 age group (Volksgezondheidenzorg.info, 2019). Nonetheless, we labeled them as if they were FN at the initial assessment. As stated before, clinicians tend to refer when in doubt to minimize their FNs, which adds to their low FNs in this study. This favors the add-on strategy, but it also mimics the clinical reality.
The acceptance of the amount of FN next to the availability of an NPA will influence where one puts the cutoff for referring to an NPA. Simply changing a single cutoff will not improve the number of classification errors (Landsheer, Reference Landsheer2020). With a second cutoff for monitoring, one can consider the pros and cons of lowering sensitivity against the gain of specificity but avoid absolute or binary decision errors. We also considered the use of a third cutoff, meaning below a certain score no referral is necessary as dementia is surely identified, i.e. diagnosed, but this is not feasible. Even with high dementia prevalence (e.g. including the severe or known demented, although not the clinical reality), the PPV of this extra cutoff would increase but never to the needed PPV of 100%.
For settings similar to ours, we recommend the use of the double threshold as described of <21 and ≥26, as these cutoffs give the best results, including the Youden index (Dautzenberg et al., Reference Dautzenberg, Lijmer and Beekman2020, Reference Dautzenberg, Lijmer and Beekman2021). This corresponds with the most error-prone scores of the MoCA (Landsheer, Reference Landsheer2020) and is consistent with another study in old-age psychiatry, in which 87% of patients with dementia scored <20 and 100% scored <23 on the MoCA (Korsnes, Reference Korsnes2020). In addition, almost all MCI patients with low MoCA scores (<20) will develop MD, whereas only half of the MCI patients above this score will convert in the near future (Julayanont et al., Reference Julayanont2014). Another study showed that 65% of their MCI patients with a score <26 did not convert to MD (Smith et al., Reference Smith, Gildeh and Holmes2007). This suggests that a double-threshold MoCA can separate low-risk MCI patients from very high-risk patients along with almost all patients with MD and benefit from a specialized diagnostic route.
Although our findings are not compatible with other settings, different settings may also benefit from a double-threshold MoCA. Whether it is to improve accuracy or because these settings have a more diverse population (and less uniformly distributed cognitive functioning). Even if one does not agree with our proposed policy because of a low prevalence of psychiatric diseases or easy access to specialized diagnostic routes in their setting. The three policies can easily be altered to fit once own setting, e.g. full memory clinic work up <21; 21< active monitoring with an NPA <26; and ≥26 watchful waiting with an MoCA.
As the MoCA can detect changes over time in MCI patients (Krishnan et al., Reference Krishnan2017) and remains stable among cognitively normal patients (Malek-Ahmadi et al., Reference Malek-Ahmadi2018), active monitoring (21<26) can be done by reassessment with a MoCA, which has three versions avoiding a learning curve (Costa et al., Reference Costa2012; Nasreddine and Patel, Reference Nasreddine and Patel2016) and has a high retest reliability (Bruijnen et al., Reference Bruijnen2020). Together with an interview on IADL, giving an improved model fit (Durant et al., Reference Durant2016) combined with an IQcode (De Jonghe, Reference De Jonghe1997), it can be administered in less than 30 minutes and could increase the overall diagnostic accuracy (Roalf et al., Reference Roalf2013). The average time of an NPA was 9 h, including processing and feedback, at a cost of (in the Netherlands) €110/h. Therefore, the MoCA can not only reduce the stressful NPA waiting list but also avoid €1000 per FP and actively monitor those at risk less expensively.
To conclude, the optimal strategy for NPA referral is a two-stage selection process using the MoCA with a double threshold as an add-on after initial assessment. By selecting who is likely to have dementia and should be assessed further (MoCA<21), who should be discharged (≥26), and who’s course should be monitored actively as they are at risk (21<26). This strategy not only gives the best results (accuracy, PPV, NPV) by referring most MD patients and reduces unnecessary FP referrals by 65%. It also identifies most MCIs whose intermediate state justifies active monitoring. By introducing a second cutoff, the clinical value of the MoCA improved.
Conflict of interest
The authors have no conflict of interest.
Description of authors’ roles
Géraud M. F. C. Dautzenberg: designed the study, collected the data, analyzing the data, and writing the paper.
Jeroen G. Lijmer: designed the study, assisted with writing the paper, supervized the data collection and statistical analyzing.
Aartjan T. F. Beekman: supervized the design and writing.
Acknowledgments
The authors thank Kasper Mens for his help to retrieve the digital data. The authors thank research assistants Andrea Hagg-Koelewijn, Ilse Geurts, Michelle Baars, Veerle van Meijl, Lara Verbeek, Sarah Rooijackers, Mariam Shanurkeyl, Wanisha Latchmansing, and Cagla Celik for their help collecting and digitalizing the test results and they also thank Jamie Nolan for his help in editing the manuscript.