1. Introduction
South Korea has experienced economic development and democratization in a relatively short period of time. However, Koreans’ evaluation of Korean politics is negative. Koreans are particularly concerned about what they perceive as a growing polarization between liberal and conservative political parties. Indeed, this perception has been verified by previous studies, which have shown that South Korea's two major political parties are now characterized by high levels of internal ideological homogeneity and have increasingly diverged from one another over time (Ka Reference Ka2014; Park et al. Reference Park, Kim, Park, Kang and Koo2016). In this way, the South Korean situation meets the definition of political polarization, which can be defined as loss of the capacity for inter-party dialog and compromise and the recurrence of hostile confrontations and deadlock (McCarty, Poole, and Rosenthal Reference McCarty, Poole and Rosenthal2006). In this study, political polarization refers specifically to the polarization of the political elites participating in party politics (Baldassarri and Gelman Reference Baldassarri and Gelman2008). This is because political parties do not resolve but rather amplify social conflicts in Korean society (Cho and Lee Reference Cho and Lee2021; Kwon Reference Kwon and Hollingsworth2020; Lee Reference Lee2011; Lim et al. Reference Lim, Lee, Jung and Choi2019). In this way, the polarization of elites can drive polarization on a societal scale (Druckman, Peterson, and Slothuus Reference Druckman, Peterson and Slothuus2013; Robison and Mullinix Reference Robison and Mullinix2016; Banda and Cluverius Reference Banda and Cluverius2018).
Despite its important role for representative democracy, public confidence in the National Assembly is low compared to other public institutions. A 2019 survey noted that public confidence in the National Assembly (19.7 percent) is rather low compared to institutions such as South Korea's central government (38.4 percent), court system (36.8 percent), police (36.5 percent), and prosecution (32.2 percent) (Statistics Korea 2020). Some scholars have suggested that this low level of public confidence in the National Assembly is a direct result of the disappearance of compromise and coexistence within National Assembly politics, which is reflected in the confrontation and deadlock between political parties (Yoo Reference Yoo2009; Seo Reference Seo2016). As these hostile, polarizing confrontations between political parties continue and repeat, major legislation and policy issues become delayed, and law-making becomes more difficult and less effective (McCarty, Poole, and Rosenthal Reference McCarty, Poole and Rosenthal2006; Gilmour Reference Gilmour1995; Groseclose and McCarty Reference Groseclose and McCarty2001). Although it is easier for internally consistent majority ruling parties to garner the support necessary to pass legislative proposals, enacting such bills into law is more difficult when politics are polarized in this way (Krehbiel Reference Krehbiel1998; Brady and Volden Reference Brady and Volden1998). This is a key issue, because as the productivity of politics decreases, distrust in politics increases, and the meaning and purpose of representative democracy fade as a result (Hibbing and Theiss-Morse Reference Hibbing and Theiss-Morse1995, Reference Hibbing and Theiss-Morse2002; Hetherington Reference Hetherington2005; Theriault Reference Theriault2008). In short, although the expression and fierce contestation of political views is important to democracy, ideological differences between parties can widen to the point that confrontation and conflict intensify and productive debate or legislative work is impossible. Thus, it is very important for observers and policy-makers alike to determine the roots, extent, and consequences of political polarization, and to work to remedy it.
Since roll-call data were released from the second half of the 16th National Assembly, scholars have studied political polarization within the National Assembly using the Nominal Three-Step Estimation (NOMINATE) method proposed by Poole and Rosenthal (Reference Poole and Rosenthal1985) (e.g., Jeon Reference Jeon2006; Lee and Lee Reference Lee and Lee2008; Lee and Lee Reference Lee and Lee2015). However, this approach only measures the outcome of votes on bills, not how polarization arises and affects the legislative process in detail. The current study aims to present empirical evidence of the polarization of South Korean political elites by analyzing subcommittee meeting minutes that actually reflect the legislative process, rather than the result of votes on the floor. Thus, it fills some gaps in the literature.
The subcommittee meeting minutes show how politicians from across the political spectrum use language to gain advantages in debates over bills, and they thus reflect the active, competitive use of language in the legislative process (Edelman Reference Edelman1985, 16). This study focuses primarily on the second subcommittee of the Legislation and Judiciary Committee because it examines the wording of all bills that have been reviewed by other committees. This study uses a natural language processing (NLP) model that learned the political language of 20 years of whole subcommittee meeting minutes to examine the minutes of the second subcommittee of the Legislation and Judiciary Committee from the 17th National Assembly through 20th National Assembly in their entirety and quantify changes in political polarization overtime.
The classification model of the NLP technique learns sentences and words belonging to the two classes, and the trained model classifies the target text and measures its accuracy. The degree of this accuracy is calculated by measuring the polarization of political language. Its findings indicate that the degree of political polarization increased and decreased at various times over the study period but has risen sharply since the second half of 2016 and remained high through 2020. This suggests that partisan political gaps between members of the South Korean National Assembly increase substantially.
The use of neural network NLP techniques can complement previous studies and present different perspectives on analyzing political polarization. The current study also contributes to the literature by providing empirical evidence from South Korea, as most recent attempts to analyze ideology using neural network NLP techniques have been limited to Anglophone and European countries.
The remainder of the article is organized as follows. Section 2 reviews the literature on measuring political polarization and presents some theoretical discussion of language's relation to politics. Section 3 discusses this study's methodology. Section 4 discusses this study's data—that is, the subcommittee meeting minutes—in more detail. Section 5 provides the results. Section 6 discusses the findings in some detail. Section 7 discusses the implications of the findings.
2. Literature review
Political polarization is not exclusively a South Korean problem; it is a growing global phenomenon (McCarty, Poole, and Rosenthal Reference McCarty, Poole and Rosenthal2006; Singer Reference Singer2016; Pew Research Center 2017; Banda and Cluverius Reference Banda and Cluverius2018; Vachudova Reference Vachudova2019). Political polarization has been studied mainly in the context of national-level politics in the United States (Abramowitz and Saunders Reference Abramowitz and Saunders2008; Theriault Reference Theriault2008; Shor and McCarty Reference Shor and McCarty2011; Banda and Cluverius Reference Banda and Cluverius2018). According to Binder (Reference Binder1999) and Fleisher and Bond (Reference Fleisher and Bond2004), the political polarization of national-level politics in the United States has intensified since the Democratic and Republic parties took on more sharply divisive and ideological identities in the 1980s and 1990s. They also found that cross-party voting has declined, as has the number of opposition members supporting the president's agenda. Furthermore, Layman and Carsey (Reference Layman and Carsey2000, Reference Layman and Carsey2002) found that congressional candidates with increasingly ideological roll-call voting records are more likely to be elected or re-elected.
Although South Korea, unlike the United States, has a parliamentary system with multiple political parties, two major parties have occupied most seats in the South Korean National Assemblies since the 1990s (after democratization)—much like the United States, which only has two political parties. While South Korea's liberal–conservative dimension does not exactly match the liberal–conservative dimension in the United States party system, it has become very analogous to its party system in terms of the political parties’ position on economic and redistribution policy (Hix and Jun Reference Hix and Jun2009; Kang Reference Kang2018; Kwak Reference Kwak1998). In addition, Korean political parties have developed toward a loosely centralized organization, rather than a catch-all organization (Han Reference Han2021). The cohesion of the two political parties has been somewhat large, even though their cohesion has been weakened compared to when they were led by two charismatic politicians, Kim Young-sam and Kim Dae-jung, the former presidents (Han Reference Han2021; Horiuchi and Lee Reference Horiuchi and Lee2008; Jeon Reference Jeon2014; Kim Reference Kim2008). Both parties have a strong support base in that they developed based on regionalism: the Honam and Youngnam regions (Cho Reference Cho1998; Kang Reference Kang2016; Kwon Reference Kwon2004; Lee and Repkine Reference Lee and Repkine2020; Moon Reference Moon2005).
Scholarly literature on political polarization in South Korea has appeared only recently. Lee (Reference Lee2011) gave empirical grounds for examining political polarization in the Korean context by analyzing changes in lawmakers and Korean citizens’ ideological orientation. They found that polarization increased between politicians but was minimal between citizens, suggesting that polarization in Korean politics is driven by political elites. Similarly, Ka (Reference Ka2014) surveyed members of the 16th, 17th, 18th, and 19th National Assemblies and found that the ideological gaps between them widened over this period. Lee and Lee (Reference Lee and Lee2015) analyzed roll-call data from the 16th, 17th, and 18th National Assemblies and found that the state of polarization in Korean party politics is severe and has become remarkably worse since the 17th National Assembly. Kang (Reference Kang2012) examined roll-call data and found that political polarization was particularly prominent in foreign policy discussions during the 19th National Assembly. Lee (Reference Lee2015) concurred, suggesting that political polarization in South Korean politics has accelerated since the 2000s not just as a result of debates over domestic issue such as expanding universal welfare but foreign policy and trade issues such as Korea's involvement in the Iraq War, the prospects of a Korea–US free trade agreement, and importing American beef.
Such polarization likely peaked in 2016–17 (Jung Reference Jung2018). In December 2016, the National Assembly impeached President Park Geun-hye, a member of the conservative party. She was later removed from office by a unanimous decision of the Constitutional Court in March 2017 (Shin and Moon Reference Shin and Moon2017). The polarized post-impeachment atmosphere is reflected in the 20th National Assembly's bill passage rate—approximately 36 percent, the lowest in the National Assembly's history.Footnote 1 Furthermore, this period saw an increase in conservative politicians’ extra-parliamentary political activity, insofar as the liberal, public-led candlelight rallies were met by conservative, civil society-led Taegeukgi rallies involving conservative politicians (Cho and Lee Reference Cho and Lee2021; Hwang and Willis Reference Hwang and Willis2020; Min and Yun Reference Min and Yun2018; Oh Reference Oh2019; Reijven et al. Reference Reijven, Cho, Ross and Dori-Hacohen2020). These may partially explain the phenomenon of political polarization, but the bill passage rate and Taegeukgi rallies do not empirically prove that political polarization in South Korean has intensified since President Park's impeachment.
The studies aiming to prove political polarization are either based on roll-call data or survey data. Roll-call data are relatively easy to access, can be easily modified and applied, and can function as data in themselves. This makes it easy to apply them as a way to measure political polarization. Hence, many studies have used roll-call data to study polarization and ideology (e.g., Poole and Rosenthal Reference Poole and Rosenthal1997; Ansolabehere, Snyder, and Stewart Reference Ansolabehere, Snyder and Stewart2001; Jeon Reference Jeon2006; Poole Reference Poole2007; Garand Reference Garand2010; Abramowitz Reference Abramowitz2010; Shor and McCarty Reference Shor and McCarty2011; Lee and Lee Reference Lee and Lee2015; Lee Reference Lee2015). However, some studies have argued that this approach does not travel well in the parliamentary context (Schwarz, Traber, and Benoit Reference Schwarz, Traber and Benoit2017) because the voting data captured in roll-call data may reflect selection bias for politicians’ votes (Carrubba et al. Reference Carrubba, Gabel, Murrah and Clough2006; Carrubba, Gabel, and Hug Reference Carrubba, Gabel and Hug2008; Hug Reference Hug2010). Politicians’ votes may not directly reflect their ideological positions—they may reflect the influence of other factors, such as politicians’ personal interests and their relations with the ruling government (Sinclair Reference Sinclair1982, Reference Sinclair, Bond and Fleisher2000; Benedetto and Hix Reference Benedetto and Hix2007; Kam Reference Kam2009). This approach also overlooks the context of the legislative process, which is key to understand ideology (Jessee and Theriault Reference Jessee and Theriault2014). For example, by focusing on votes, these studies cannot account for deliberation which arrives at consensus or the fact that some politicians abstain from voting or are not present at the vote. Such studies would mischaracterize voting results’ relationship to ideology, perhaps especially in highly polarized contexts such as the Korean National Assembly.
Furthermore, decision-making processes at the party level can affect the individual lawmakers’ votes regardless of their personal ideological dispositions. For example, as a party becomes more conservative, its members are likely to vote more conservatively regardless of their own stances on a given issue. This is especially true in South Korea, where party leadership has a strong influence over individual lawmakers (Jeon Reference Jeon2014; Lee and Lee Reference Lee2011). In this case, the lawmaker is given a more conservative NOMINATE score than his or her ideological orientation. In other words, increases in the ideological distance between lawmakers and their political party's leadership might predict their overall ideological polarization. In NOMINATE-based analysis, it is necessary to decide which legislation and lawmakers to include in the analysis target, but there is no statistical or theoretically established criterion for this yet in literature.
Other studies (e.g. Lee Reference Lee2011; Kang Reference Kang2012; Ka Reference Ka2014, Reference Ka2016, Park et al. Reference Park, Kim, Park, Kang and Koo2016; Park et al. Reference Park, Kim, Park, Kang and Koo2016 Jung Reference Jung2018) have used National Assembly survey data to study polarization in South Korean politics. Like the roll-call data, these data are also widely available—members of the National Assembly have filled out questionnaires on their ideological orientation since 2002. However, this method also has limitations. First, lawmakers sometimes refuse to respond to these surveys. For example, only 56.91 percent members from the Democratic Party, 55.7 percent from the Saenuri Party, 42.11 percent from the People's Party, and 16.67 percent from the Justice Party responded to a survey of the 20th National Assembly (Park et al. Reference Park, Kim, Park, Kang and Koo2016, 127). Second, if the questionnaire items do not remain consistent over time, it might be more difficult to reliably measure lawmaker’ ideology over time. Finally, and above all, this method relies on lawmakers’ self-reported responses, so it is difficult to regard them as objective data—not the least because these responses do not reflect politicians’ actual actions and may be strategically adjusted for the context of a study.
Other studies have undertaken qualitative analyses of National Assembly subcommittee meeting minutes. Kwon and Lee (Reference Kwon and Lee2012) classified lawmakers’ remarks in the minutes of the standing committee of the 17th National Assembly into those that reflected partisanship, representativeness, professionalism, and compromise. Their analysis showed that partisan criticisms and personal attacks were less prominent in these minutes than the other three types; instead, they found that the minutes reflected a search for opinions based on expert knowledge and the identification of causal relationships. Ka et al. (Reference Ka, Cho, Choi and Sohn2008) analyzed the same meeting minutes in order to study National Assembly members’ participation in the meeting and the subcommittee's decision-making processes. They found that the standing committee's decision-making method was closer to a consensus system than a majority system by showing that proposals for the chairman's resolutions were more common than votes. However, these qualitative analyses have two problems. First, the researchers’ subjective selection of data makes it difficult for other researchers to reproduce their results. Second, it is difficult to extract sufficiently meaningful information from this kind of data, especially given the large (and increasing) volume of meeting minute data. Below, in the methodology section, this study discusses why current study chose to use an NLP model to overcome the limitations with previous studies described above and describe the use of the model.
3. Methodology
NLP techniques
Unlike the roll-call-based approach, an NLP approach to meeting minutes analyzes the discussion process rather than voting results. Unlike the survey-based approach, an NLP approach analyzes the degree to which politicians’ political polarization reflects their actual actions (in this case, behavior in subcommittee meetings). This approach can analyze vast amounts of meeting minutes using algorithms, which can be used by other researchers, making the results highly reproducible (see Appendix A for more details about NLP and see Appendix B for NLP code).
Other studies in political science and the social sciences have used NLP techniques to measure political polarization. These studies value these techniques because they allow the use of large amounts of text data using supervised or unsupervised learning approaches (Haselmayer and Jenny Reference Haselmayer and Jenny2017; Wilkerson and Casas Reference Wilkerson and Casas2017; Goet Reference Goet2019; Pozen, Talley, and Nyarko Reference Pozen, Talley and Nyarko2019; Gentzkow, Shapiro, and Taddy Reference Gentzkow, Shapiro and Taddy2019; Proksch et al. Reference Proksch, Lowe, Wackerle and Soroka2019; Chang and Masterson Reference Chang and Masterson2019; Hausladen, Schubert, and Ash Reference Hausladen, Schubert and Ash2020). This study trains a model to learn political language from meeting minutes (see below) and then classifies text data to quantify political polarization.
If political parties maintain disparate positions as a result of their ideological characteristics and these parties’ members use language accordingly, NLP model should be able to classify text by learning the respective languages of liberal and conservative parties and then identifying classification elements. Figure 1 summarizes the data analysis process: the current study built NLP model using 20 years’ worth of subcommittee text data, excluding that of the Legislation and Judiciary Committee, and then classified the text from the second subcommittee of the Legislation and Judiciary Committee as the target text.

Figure 1. Political polarization estimate process using NLP
The logic of text classification is as follows. Text classification refers to the process of receiving a sentence (or word) as input and classifying where the sentence belongs between pre-trained (defined) classes. That is, in advance, by classifying the sentences or words to be learned into binary (conservative vs liberal), the NLP model learns through the learning process (training process), and the target text is input and classified. To explain it based on Figure 1, the learning process means the process of learning to which class the sentences (or words) related to a specific issue belong through the “Training, tuning, and evaluation” (corresponding to “NLP model learning code” in Appendix B). And by classifying “Target Data” through the trained model, we can estimate the polarization (corresponding to “Test code” in Appendix B).
The logic of quantification of polarization is as follows. If the classification model can accurately distinguish between the language of given political parties, it will be considered to indicate significant ideological polarization between those factions. If the trained model cannot do so, then the degree of polarization between these parties’ ideological positions will be considered to be small or insignificant. The classification model that learned the language of each party for 20 years classifies each meeting minute of the second subcommittee of the Legislation and Judiciary Committee. We can convert the accuracy (degree of polarization) of each into time-series data.
This study uses bidirectional encoder representations from transformers (BERT), a neural networks model that dynamically generates word vectors utilizing contexts and learning them in both directions according to context (see Appendix A for more details). BERT is a semi-supervised learning model that builds a general-purpose language understanding model using unsupervised learning of a large corpus. It fine-tunes this model using supervised learning and applies it to other work (Devlin et al., Reference Devlin, Chang, Lee and Toutanova2019). Simply put, BERT uses pre-learning within a text corpus to create a model that understands the basic patterns of language, and transfer learning is then performed by applying this model to new tasks. In this way, BERT combines the advantages of unsupervised and supervised learning approaches. Unsupervised methods come with significant post hoc validation costs, as the researcher “must combine experimental, substantive, and statistical evidence to demonstrate that the measures areas conceptually valid as measures from an equivalent supervised model” (Grimmer and Stewart Reference Grimmer and Stewart2013, 271). However, as described above, BERT performs a classification task through supervised learning by transferring a model learned through unsupervised learning. Therefore, current study compared the results of Generative Pre-trained Transformer 2 (GPT-2) (Ethayarajh Reference Ethayarajh2019), another transformer-based learning model, to validate the results.
This study utilized Google Colab Python 3 and Pytorch as analytic tools. This study also applied BERT with the Hugging Face transformer model installed. Hugging Face is a useful Pytorch interface designed for utilization with BERT. The library includes pre-build modifications of the model, which enables specific tasks, such as classification. When fine-tuning BERT model, this study established the following: batch size of 32, learning rate (Adam) of 2e-5, and 3 epochs. In dividing the data into training, validation, and testing data in the model-building process, this study first set 30 percent of the total data as test data and then 10 percent of the training data as validation data (see Appendix B for seeing the process of building BERT NLP model, additionally GPT-2 NLP model).
4. Data
Data that best reflect the functions of politicians’ political language are necessary to measure political polarization. This study proposes an approach in which the model learns the entire minutes of the Standing Committee subcommittees except for the Legislation and Judiciary subcommittee and then classifies the minutes of the second subcommittee of the Legislation and Judiciary Committee.
Figure 2 shows the South Korean legislative process. The subcommittee is responsible for practical legislation and government budget review within National Assembly committees. Standing committees in different fields, including education, diplomacy, national defense, labor, and the environment, include three to six subcommittees each with narrower roles. If a bill's contents are simple or uncontentious, it is not referred to subcommittees. If they are, the relevant committee refers the bill to a subcommittee for review after a general discussion. The final resolutions of bills are done in plenary sessions of the National Assembly after they have been reviewed by each standing committee. It is common for bills to be passed during plenary sessions without discussion. Not all bills and budgets are reviewed by the standing committee; in such cases, subcommittees conduct a practical review of the given bills.

Figure 2. The process of enacting and amending a law in South Korea
Data from: The National Assembly of the Republic of Korea (https://korea.assembly.go.kr:447/int/act_01.jsp).
All bills passed by each standing committee are examined by the second subcommittee of the Legislation and Judiciary Committee (see Figure 2). This subcommittee performs the final review before a bill is transferred to the plenary session; it thus effectively serves a role similar to that of the United States Senate. The second subcommittee reviews whether the bill conflicts with existing bills or violates the constitution and refines the wording of bills. It is during this process that each party's positions on the bill become clear. Thus, political language is actively used in the standing committee subcommittees and in the second subcommittee of the Legislation and Judiciary Committee in particular.
Article 50, Clause 1 of the South Korean Constitution states that the National Assembly must disclose the bill review process to the public, with the exception of bills that concern national security (Article 50, Clause 1 of the Constitution; Article 75 of the National Assembly Act) and meetings of the intelligence committee (Article 54-2 of the National Assembly Act). Thus, sample data are quite comprehensive, not limited to certain subcommittees. They contain meeting minutes from all standing committee subcommittee meetings between July 2000 and May 2020. Current research gave the Democratic Party of Korea (the leading liberal party) a value of zero and the People's Power Party (the leading conservative party) a value of one when pre-processing the minute data for training. These two leading parties have won most of the seats in South Korean parliament since the 1990s; this study refers to them by their current names (as of 2021) for convenience. This study also classified data which represent the actions of lawmakers from minor parties within the National Assembly in a binary manner, accounting for their political inclination to form coalitions with either of the two major parties. This study acknowledges that the classification of minor parties cannot but be subjective (see Appendix C for more details).
When pre-processing the subcommittee data, this study noted that documents from the 16th and 17th National Assemblies presented the names of assembly members in Chinese characters instead of Korean letters. Thus, this study had to translate their names into Korean. This study excluded unnecessary or irrelevant information, such as descriptions of the documents and remarks by other persons, from the analysis. It is important to pre-process the data so that we can better analyze relevant dialog and exchanges among relevant parties.
5. Results
Figure 3 shows the results of BERT model of 17 years’ worth of subcommittee meeting data. For comparison, the red line marking a value of 0.7 is the benchmark for a high degree of polarization, and the blue dotted line marking a value of 0.5 is the average accuracy result of evaluating the test data in the process of building the BERT classification model. The green dotted line represents the degree of polarization, and the orange line represents the trend. The X-axis marks the years 2004–2020 (from the 17th to the 20th National Assembly) and the administration in each period.

Figure 3. Political polarization, 2004–2020
Figure 3 shows that there is a trend toward increasing political polarization. Although polarization remained relatively steady between 2004 and the early 2010s and even decreased between 2011 and 2014, it rebounded during the first half of 2014 and has risen sharply and remained high since late 2016. Figure 4 displays changes in political polarization between 2004 and 2020, by National Assembly.
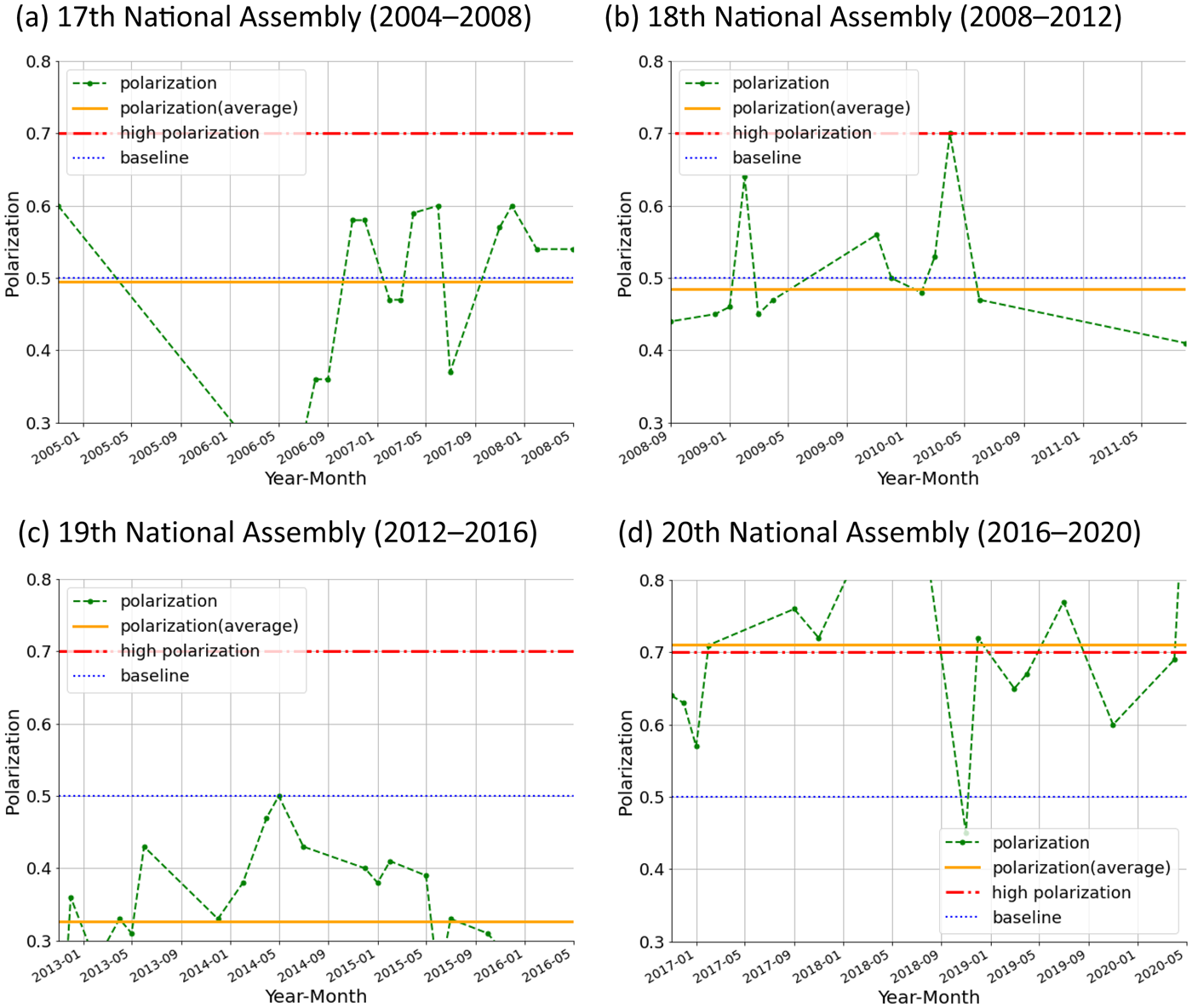
Figure 4. Political polarization, 2004–2020, by National Assembly
The figure indicates that the average degree of polarization was similar in the 17th (0.4953) and 18th (0.485) National Assemblies. Polarization waxed and waned during the 17th National Assembly, rose sharply in April 2010, and then trended downward for the rest of the 18th National Assembly. The average degree of polarization during the 19th National Assembly was 0.3265; it rose during April and May 2014 and then began to decline. Polarization was most severe during the 20th National Assembly, in which the average degree of polarization was 0.7043. The data indicate that polarization rapidly escalated in February and March 2009, April 2010, June 2013, May 2014, and November 2016. Although it usually decreased after these escalations, polarization has remained very high since 2016–17. From a data-intuitive perspective, it may rise and fall due to noise in the data. However, remaining high should be interpreted in a different context. This finding implies that polarization is becoming and remaining more intense over time rather than returning to normal or exhibiting the wax/wane pattern of previous National Assemblies. Given that this model captures wider political differences beyond particular legislation, this study interprets pre-2016 cycles in polarization as reflecting regular politics and debate and the high level of post-2016 polarization as capturing a growing and more enduring set of ideological differences between ruling and opposition parties. Figure 5 compares the results of the BERT and GPT-2 models. They exhibit similar trends in polarization, especially after 2016, thus validating the findings.

Figure 5. Comparing the BERT and GPT-2 models
6. Discussion
The above section described the process and the finding that increasingly polarized political language indicates a serious, more enduring, and deepening polarization between political elites in South Korea. This deepening tension leads each of the parties to use different political language and stoke division on particular issues. This study also found that the more polarizing the language used in meeting minutes, the more polarized politics becomes (e.g. the growth in partisan language after 2016). These conflicts have obvious consequences for the legislative process.
There may be several reasons behind the increase in polarization post-2016, but the impeachment of President Park undeniably looms largest among them. After Moon Jae-in was elected following the impeachment, his administration promoted investigations which framed the previous two conservative administrations (Park Geun-hye administration and Lee Myung-bak administration) as corrupt and untrustworthy (B. Kim Reference Kim2019; Kirk Reference Kirk2020; Lee Reference Lee2018). These included the creation of a special committee to confiscate the illegitimate proceeds earned by former president Park Geun-hye and her longtime friend Choi Soon-sil. The Moon Jae-in administration's core and public focus on correcting the mistakes and injustices of previous governments formed by the opposition party has had a lingering and polarizing effect on Korean political discourse. Although these policies nominally aimed to restore and improve South Korean democracy, they have instead made Korean politics so polarized that party politics is nearly impossible. There have been no joint efforts between the two parties to create an inclusive, good-faith, post-impeachment regime in the public interest, and this has led to a culture of slander and personal attacks.
Unfortunately, the politicians who made up a large part of the conservative party opposed or denied the impeachment, and ironically disparaged and rejected the democratically constituted Moon Jae-in administration (Kim Reference Kim2020). On the other hand, the faction leading the impeachment regarded the opposition faction only as an object of reform and did not accept it as an object of cooperation, driving parliamentary politics into a hostile confrontation. Following the impeachment, Park's party—the People's Power Party—conducted political activities outside of parliament, such as the Taegeukgi street rallies (Cho and Lee Reference Cho and Lee2021; T.-H. Kim Reference Kim2019). These rallies turned violent and, in turn, damaged the legislative process and the prospect for effective parliamentary politics (Cho and Lee Reference Cho and Lee2021; Kwon Reference Kwon and Hollingsworth2020). Conflicts between two factions persisted throughout the 20th National Assembly, and the findings of this study can be interpreted as reflecting the language of conflict in the process of legislation (in terms of bill passage rate, the passage rate of bills proposed by lawmakers was particularly low in the 20th National Assembly. see Appendix D for details).
7. Conclusions
This study's findings indicate that political polarization has waxed and waned in South Korea's National Assembly since 2004, but increased sharply in late 2016 and has remained at a high level since. This indicates that South Korea, similar to many other countries, is affected by the widening and deepening phenomenon of political polarization. I conclude this study by discussing some implications of the findings.
The findings have important implications for the National Assembly moving forward. The analysis indicates that persistent use of polarizing political language stokes polarization in general. This is likely because it removes opportunities for politicians to find (or seek) common ground from which to build a compromise. These findings imply that bills whose contents are closely related to people's everyday lives and give the parties their few opportunities to make ideological gains may not be properly reviewed, and that those which have a lot of ideological content often face dead lock and/or last-resort negotiations. These findings also imply that polarization can undermine other important functions of the National Assembly, such as conducting confirmation hearings. Furthermore, an increasingly polarized environment pushes political parties to seek ideological gains rather than governance—they support or maintain policies which only appeal to their supporters, cast all political issues as dichotomous, and perpetuate a picture of their political opponents as enemies rather than parliamentary colleagues. Members of the 21st National Assembly, which opened in June 2020, should seek to avoid increasingly polarizing language and aim to resolve polarization and create cross-party dialog.
The current study has some shortcomings, but it contributes to the development of the analysis of political polarization based on NLP, analyzes the polarization of South Korean politics, complements previous studies with the new approach and gathers data that can be of use for further studies. Above all, this study opens up new areas of analysis of political phenomena using neural network algorithms.
The limitations of this study and suggestions for follow-up studies are as follows. First, this study's proposed causal link between language and political polarization is tentative and begs further exploration. This study's empirical findings are only suggestive; although it helps us understand the trends and timing of the escalation of polarization in South Korean politics since 2004, it does not suggest causes for this phenomenon or specify which social issues exacerbated polarization. Furthermore, it is difficult to determine clearly whether the polarization of political language is influenced by a particular political phase, represents a position on a particular bill, or is caused by an interaction between the two.
When measuring polarization in elite politics, there is no data directly representing polarization in the real world. Therefore, this study utilized the tool of the language of the space of the National Assembly, which is considered to reflect the political polarization. In terms of language as a proxy variable, the possibility of a certain level of error in its measurement is open. In a follow-up study, it is necessary to compare it with the result of analyzing the roll-call data of the 20th National Assembly in order to analyze the political polarization in more depth.
Second, polarization has multiple meanings—it can refer to gaps between parties’ ideological tendencies or their to polarizing behavior (e.g., basing political positions on factional logic and the need to score ideological wins rather than focusing on governance). While both beget confrontation and conflict, the former presupposes a polarization of opinion and the latter does not. This study's approach makes it difficult to clearly determine whether the conflict between liberals and conservatives in South Korea is attributable to differences in their ideological orientation or politicians voting by party lines or logic.
Third, as mentioned above, previous studies have found that polarization is more prominent in certain fields, such as foreign policy (Kang Reference Kang2012; Park et al. Reference Park, Kim, Park, Kang and Koo2016). However, this study cannot contribute to this argument because this study analyzed meeting minutes in general without specifying a field of focus. Although polarization is a broad phenomenon with many disparate effects, it is important for future researchers to determine which dimensions of politics are most polarized or polarizing so that we can better understand and overcome polarization.
Fourth and finally, the polarization of South Korean parliamentary politics reported in this study does not necessarily reflect the overall polarization of South Korean society or political discourse. Political elites may be polarized without the masses being similarly polarized. Future studies should analyze the polarization of the masses in more detail.
Acknowledgement
I thank the anonymous reviewers and the editor for their thoughtful reviews and helpful comments on the previous drafts. Their comments greatly contributed to the improvement of the quality of this manuscript.
Conflict of Interest
The author declares none.
Funding
The author received no financial support for the research, authorship, and/or publication of this article.
Appendix A
In natural language processing (NLP), “distribution” refers to a set of neighboring words or contexts that appear simultaneously within a window, that is, within a specific range. The distribution of individual words depends mainly on where within sentences the words appear and which words appear frequently in neighboring positions (Harris Reference Harris1954; McDonald and Ramscar Reference McDonald and Ramscar2001; Sahlgren Reference Sahlgren2008). That is, NLP is premised on a distributional hypothesis (Pantel Reference Pantel2005): if a word pair appears frequently in a similar context, the meaning of the words will also be similar.
Conventional statistics-based language models are trained by counting the frequency of words; in contrast, neural network learning enables flexible capture of the relationship between input and output and can function as a probability model in itself (Goldberg Reference Goldberg2017). A neural network, which belongs to machine learning, is a series of algorithms that endeavors to recognize underlying relationships in a set of data through a process that mimics the way the human brain operates. In this sense, neural networks refer to systems of neurons, either organic or artificial in nature. Among NLP models using neural networks, this study applies BERT, a transformer-based transfer learning model that uses contextual information embedded at the sentence level and combines supervised and unsupervised learning with bidirectional characteristics that accounts for both forward and reverse directional contexts (Devlin et al. Reference Devlin, Chang, Lee and Toutanova2019). This model was published by Google in 2018.
The reason why this study uses BERT as a text classification model lies in its embedding and tokenization methods. First is dynamic embedding (Peters et al. Reference Peters, Neumann, Iyyer, Gardner, Clark, Lee and Zettlemoyer2018; Devlin et al. Reference Devlin, Chang, Lee and Toutanova2019). Ideal expressions of words must contain the syntactic and semantic characteristics of the words and must capture meanings that can vary by context. Context-free models such as Word2Vec and GloVe, which embed at word level, have been limited in expressing words that shift by context, because in these models all words have static vectors (Mikolov et al. Reference Mikolov, Chen, Corrado and Dean2013; Pennington, Socher, and Manning Reference Pennington, Socher and Manning2014), and certain words always carry the same expression regardless of the situation.
A feature of BERT that most prominently distinguishes it from the conventional Word2Vec and GloVe is that one word may entail different embeddings depending on the shape and position of the characters, thereby removing ambiguity. Because BERT dynamically generates word vectors using contexts, it is possible to create different word expressions depending on context. Another characteristic of BERT that this study notes is the tokenization method. As the Korean language is an agglutinative language, the role of morphemes is more prominent than in English, for example, and that of words less so. Hence, conventional Korean NLP has generally been used to cut and tokenize Korean language data into morphemes (Lim Reference Lim2019). Thus there exist methods to utilize external morpheme analyzers that provide favorable performance, but this approach is not only affected by the variable performance of morpheme analyzers but also prone to multiple out-of-vocabulary (OOV) occurrences in the Korean language, in which word forms vary extensively. Accordingly, this study examines alternative tokenization methods independent of morpheme analyzers.
BERT uses a tokenization algorithm called Word Piece that tokenizes without relying on external morpheme analyzers. Word Piece creates a token by collecting meaningful units frequently appearing in a word and expresses a word as a combination of sub words, enabling detailed expressions of its meaning (Wu et al. Reference Wu, Schuster, Chen, Le, Norouzi, Macherey, Krikun, Cao, Gao, Macherey, Klingner, Shah, Johnson, Liu, Kaiser, Gouws, Kato, Kudo, Kazawa, Stevens, Kurian, Patil, Wang, Young, Smith, Riesa, Rudnick, Vinyals, Corrado, Hughes and Dean2016; Devlin et al. Reference Devlin, Chang, Lee and Toutanova2019). This approach is useful for processing words not found in the dictionary, such as neologisms. The application of BERT Word Piece may be useful because the minutes of the subcommittee of the standing committee of the National Assembly, which this study analyzes, reflects various issues in diverse areas of real society.
In terms of performance, favorable performance of a model indicates that it captures the grammatical and semantic relationships of natural languages. Sentence embedding techniques such as BERT are useful in that they more effectively capture these relationships than word-level embedding techniques such as Word2Vec and GloVe. Furthermore, among models using sentence embedding techniques (ELMo, GPT), BERT actively utilizes the role of context, bidirectionally examining forward and reverse-directional contexts (Devlin et al. Reference Devlin, Chang, Lee and Toutanova2019; Tenny, Das, and Pavlick Reference Tenny, Das and Pavlick2019). Similarly, Generative Pre-trained Transformer 2 (GPT-2) is an open-source artificial intelligence created by OpenAI in 2019, which is another transformer-based learning model (Ethayarajh Reference Ethayarajh2019).
Appendix B
B1 BERT NLP model learning code (Google Colab Python 3)
!pip install transformers
-------------------------------------------------------------------
import tensorflow as tf
import torch
from transformers import BertTokenizer
from transformers import BertForSequenceClassification, AdamW, BertConfig
from transformers import get_linear_schedule_with_warmup
from torch.utils.data import TensorDataset, DataLoader, RandomSampler, SequentialSampler
from keras.preprocessing.sequence import pad_sequences
from sklearn.model_selection import train_test_split
from sklearn.metrics import classification_report
import pandas as pd
import numpy as np
import random
import time
import datetime
-------------------------------------------------------------------
Train = pd.read_csv(′/content/train.csv′)
sentences = train[′text′]
sentences[:5]
labels sentences = ["[CLS] " + str(sentence) + " [SEP]" for sentence in sentences]
sentences[:5]
-------------------------------------------------------------------
labels = train['label'].values
MAX_LEN = 128
input_ids = [tokenizer.convert_tokens_to_ids(x) for x in tokenized_texts]
input_ids = pad_sequences(input_ids, maxlen=MAX_LEN, dtype="long", truncating="post", padding="post")
input_ids[0]
-------------------------------------------------------------------
attention_masks = []
for seq in input_ids:
seq_mask = [float(i>0) for i in seq]
attention_masks.append(seq_mask)
print(attention_masks[0])
--------------------------------------------------------------------
train_inputs, validation_inputs, train_labels, validation_labels = train_test_split(input_ids,labels,random_state=2018, test_size=0.1)
train_masks, validation_masks, _, _ = train_test_split(attention_masks, input_ids,random_state=2018, test_size=0.1)
train_inputs = torch.tensor(train_inputs)
train_labels = torch.tensor(train_labels)
train_masks = torch.tensor(train_masks)
validation_inputs = torch.tensor(validation_inputs)
validation_labels = torch.tensor(validation_labels)
validation_masks = torch.tensor(validation_masks)
print(train_inputs[0])
print(train_labels[0])
print(train_masks[0])
print(validation_inputs[0])
print(validation_labels[0])
print(validation_masks[0])
--------------------------------------------------------------------
batch_size = 32
train_data = TensorDataset(train_inputs, train_masks, train_labels)
train_sampler = RandomSampler(train_data)
train_dataloader = DataLoader(train_data, sampler=train_sampler, batch_size=batch_size)
validation_data = TensorDataset(validation_inputs, validation_masks, validation_labels)
validation_sampler = SequentialSampler(validation_data)
validation_dataloader = DataLoader(validation_data, sampler=validation_sampler, batch_size=batch_size)
-------------------------------------------------------------------
device_name = tf.test.gpu_device_name()
if device_name == '/device:GPU:0':
print('Found GPU at: {}'.format(device_name))
else:
raise SystemError('GPU device not found')
--------------------------------------------------------------------
if torch.cuda.is_available():
device = torch.device("cuda")
print('There are %d GPU(s) available.' % torch.cuda.device_count())
print('We will use the GPU:', torch.cuda.get_device_name(0))
else:
device = torch.device("cpu")
print('No GPU available, using the CPU instead.')
-------------------------------------------------------------------
model = BertForSequenceClassification.from_pretrained("bert-base-multilingual-cased", num_labels=2)
model.cuda()
optimizer = AdamW(model.parameters(),lr = 2e-5, eps = 1e-8)
epochs = 3
total_steps = len(train_dataloader) * epochs
scheduler = get_linear_schedule_with_warmup(optimizer, num_warmup_steps = 0, num_training_steps = total_steps)
--------------------------------------------------------------------
def flat_accuracy(preds, labels):
pred_flat = np.argmax(preds, axis=1).flatten()
labels_flat = labels.flatten()
return np.sum(pred_flat == labels_flat) / len(labels_flat)
def format_time(elapsed):
elapsed_rounded = int(round((elapsed)))
return str(datetime.timedelta(seconds=elapsed_rounded))
--------------------------------------------------------------------
seed_val = 42
random.seed(seed_val)
np.random.seed(seed_val)
torch.manual_seed(seed_val)
torch.cuda.manual_seed_all(seed_val)
model.zero_grad()
for epoch_i in range(0, epochs):
# ========================================
# Training
# ========================================
print("")
print('======== Epoch {:} / {:} ========'.format(epoch_i + 1, epochs))
print('Training…')
t0 = time.time()
total_loss = 0
model.train()
for step, batch in enumerate(train_dataloader):
if step % 500 = = 0 and not step == 0:
elapsed = format_time(time.time() - t0)
print(' Batch {:>5,} of {:>5,}. Elapsed: {:}.'.format(step, len(train_dataloader), elapsed))
batch = tuple(t.to(device) for t in batch)
b_input_ids, b_input_mask, b_labels = batch
outputs = model(b_input_ids,
token_type_ids=None,
attention_mask=b_input_mask,
labels=b_labels)
loss = outputs[0]
total_loss += loss.item()
loss.backward()
torch.nn.utils.clip_grad_norm_(model.parameters(), 1.0)
optimizer.step()
scheduler.step()
model.zero_grad()
avg_train_loss = total_loss / len(train_dataloader)
print("")
print(" Average training loss: {0:.2f}".format(avg_train_loss))
print(" Training epcoh took: {:}".format(format_time(time.time() - t0)))
# ========================================
# Validation
# ========================================
print("")
print("Running Validation…")
t0 = time.time()
model.eval()
eval_loss, eval_accuracy = 0, 0
nb_eval_steps, nb_eval_examples = 0, 0
for batch in validation_dataloader:
batch = tuple(t.to(device) for t in batch)
b_input_ids, b_input_mask, b_labels = batch
with torch.no_grad():
outputs = model(b_input_ids,
token_type_ids=None,
attention_mask=b_input_mask)
logits = outputs[0]
logits = logits.detach().cpu().numpy()
label_ids = b_labels.to('cpu').numpy()
tmp_eval_accuracy = flat_accuracy(logits, label_ids)
eval_accuracy += tmp_eval_accuracy
nb_eval_steps += 1
print(" Accuracy: {0:.2f}".format(eval_accuracy/nb_eval_steps))
print(" Validation took: {:}".format(format_time(time.time() - t0)))
print("")
print("Training complete!")
--------------------------------------------------------------------
* The code above was programmed by the author for the analysis.
** The code above may change a little according to changes in the Google Colab environment.
*** A rigorous text preprocessing and labeling should be preceded prior to the implementation of the above process.
B2 BERT Test code (Google Colab Python 3)
test_text = pd.read_csv('/content/test_text.csv')
---------------------------------------------------------------------
sentences = test_text['text']
sentences[:5]
---------------------------------------------------------------------
sentences = ["[CLS] " + str(sentence) + " [SEP]" for sentence in sentences]
sentences[:5]
-------------------------------------------------------------------
test_labels = pd.read_csv('/content/test_labels.csv')
---------------------------------------------------------------------
labels = test_labels['label'].values
labels
---------------------------------------------------------------------
tokenizer = BertTokenizer.from_pretrained('bert-base-multilingual-cased', do_lower_case=False)
tokenized_texts = [tokenizer.tokenize(sent) for sent in sentences]
print (sentences[0])
print (tokenized_texts[0])
-------------------------------------------------------------------
MAX_LEN = 128
input_ids = [tokenizer.convert_tokens_to_ids(x) for x in tokenized_texts]
input_ids = pad_sequences(input_ids, maxlen=MAX_LEN, dtype="long", truncating="post", padding="post")
input_ids[0]
--------------------------------------------------------------------
attention_masks = []
for seq in input_ids:
seq_mask = [float(i>0) for i in seq]
attention_masks.append(seq_mask)
print(attention_masks[0])
test_inputs = torch.tensor(input_ids)
test_labels = torch.tensor(labels)
test_masks = torch.tensor(attention_masks)
print(test_inputs[0])
print(test_labels[0])
print(test_masks[0])
--------------------------------------------------------------------
batch_size = 32
test_data = TensorDataset(test_inputs, test_masks, test_labels)
test_sampler = RandomSampler(test_data)
test_dataloader = DataLoader(test_data, sampler=test_sampler, batch_size=batch_size)
-------------------------------------------------------------------
t0 = time.time()
model.eval()
eval_loss, eval_accuracy = 0, 0
nb_eval_steps, nb_eval_examples = 0, 0
for step, batch in enumerate(test_dataloader):
if step % 100 == 0 and not step == 0:
elapsed = format_time(time.time() - t0)
print(' Batch {:>5,} of {:>5,}. Elapsed: {:}.'.format(step, len(test_dataloader), elapse))
batch = tuple(t.to(device) for t in batch)
b_input_ids, b_input_mask, b_labels = batch
with torch.no_grad():
outputs = model(b_input_ids,
token_type_ids=None,
attention_mask=b_input_mask)
logits = outputs[0]
logits = logits.detach().cpu().numpy()
label_ids = b_labels.to('cpu').numpy()
tmp_eval_accuracy = flat_accuracy(logits, label_ids)
eval_accuracy += tmp_eval_accuracy
nb_eval_steps += 1
print("")
print("Accuracy: {0:.4f}".format(eval_accuracy/nb_eval_steps))
print("Test took: {:}".format(format_time(time.time() - t0)))
--------------------------------------------------------------------
* The code above was programmed by the author for the analysis.
** The code above may change a little according to changes in the Google Colab environment.
*** A rigorous text preprocessing and labeling should be preceded prior to the implementation of the above process.
B3 GPT-2 NLP model learning code (Google Colab Python 3)
!wget https://raw.githubusercontent.com/NLP-kr/tensorflow-ml-nlp-tf2/master/requirements.txt -O requirements.txt
!pip install -r requirements.txt
!pip install tensorflow==2.2.0
-------------------------------------------------------------------
import os
import tensorflow as tf
from transformers import TFGPT2Model
from tensorflow.keras.preprocessing.sequence import pad_sequences
from tensorflow.keras.callbacks import EarlyStopping, ModelCheckpoint
import gluonnlp as nlp
from gluonnlp.data import SentencepieceTokenizer
import pandas as pd
import matplotlib.pyplot as plt
import numpy as np
import re
--------------------------------------------------------------------
train_data = pd.read_csv('/content/train.csv')
train_data = train_data.dropna()
train_data.head()
---------------------------------------------------------------------
!wget https://www.dropbox.com/s/nzfa9xpzm4edp6o/gpt_ckpt.zip -O gpt_ckpt.zip
!unzip -o gpt_ckpt.zip
-------------------------------------------------------------------
def plot_graphs(history, string):
plt.plot(history.history[string])
plt.plot(history.history['val_'+string], '')
plt.xlabel("Epochs")
plt.ylabel(string)
plt.legend([string, 'val_'+string])
plt.show()
---------------------------------------------------------------------
SEED_NUM = 1234
tf.random.set_seed(SEED_NUM)
np.random.seed(SEED_NUM)
--------------------------------------------------------------------
TOKENIZER_PATH = './gpt_ckpt/gpt2_kor_tokenizer.spiece'
tokenizer = SentencepieceTokenizer(TOKENIZER_PATH)
vocab = nlp.vocab.BERTVocab.from_sentencepiece(TOKENIZER_PATH,
mask_token=None,
sep_token='<unused0>',
cls_token=None,
unknown_token='<unk>',
padding_token='<pad>',
bos_token='<s>',
eos_token='</s>')
-------------------------------------------------------------------
BATCH_SIZE = 32
NUM_EPOCHS = 3
VALID_SPLIT = 0.1
SENT_MAX_LEN = 39
def clean_text(sent):
sent_clean = re.sub("[̂가-힣ㄱ-ㅎㅏ-ㅣ\\s]", "", sent)
return sent_clean
---------------------------------------------------------------------
train_data_sents = []
train_data_labels = []
for train_sent, train_label in train_data[['text', 'label']].values:
train_tokenized_text = vocab[tokenizer(clean_text(train_sent))]
tokens = [vocab[vocab.bos_token]]
tokens += pad_sequences([train_tokenized_text],
SENT_MAX_LEN,
value=vocab[vocab.padding_token],
padding='post').tolist()[0]
tokens += [vocab[vocab.eos_token]]
train_data_sents.append(tokens)
train_data_labels.append(train_label)
train_data_sents = np.array(train_data_sents, dtype=np.int64)
train_data_labels = np.array(train_data_labels, dtype=np.int64)
--------------------------------------------------------------------
class TFGPT2Classifier(tf.keras.Model):
def __init__(self, dir_path, num_class):
super(TFGPT2Classifier, self).__init__()
self.gpt2 = TFGPT2Model.from_pretrained(dir_path)
self.num_class = num_class
self.dropout = tf.keras.layers.Dropout(self.gpt2.config.summary_first_dropout)
self.classifier = tf.keras.layers.Dense(self.num_class,
kernel_initializer=tf.keras.initializers.TruncatedNormal(stddev=self.gpt2.config.initializer_range),name="classifier")
def call(self, inputs):
outputs = self.gpt2(inputs)
pooled_output = outputs[0][:, -1]
pooled_output = self.dropout(pooled_output)
logits = self.classifier(pooled_output)
return logits
------------------------------------------------------------------
BASE_MODEL_PATH = './gpt_ckpt'
cls_model = TFGPT2Classifier(dir_path=BASE_MODEL_PATH, num_class=2)
--------------------------------------------------------------------
optimizer = tf.keras.optimizers.Adam(learning_rate=6.25e-5)
loss = tf.keras.losses.SparseCategoricalCrossentropy(from_logits=True)
metric = tf.keras.metrics.SparseCategoricalAccuracy('accuracy')
cls_model.compile(optimizer=optimizer, loss=loss, metrics=[metric])
--------------------------------------------------------------------
model_name = "tf2_gpt2_political polarization"
earlystop_callback = EarlyStopping(monitor='val_accuracy', min_delta=0.0001, patience=2)
history = cls_model.fit(train_data_sents, train_data_labels,
epochs=NUM_EPOCHS,
batch_size=BATCH_SIZE,
validation_split=VALID_SPLIT,
callbacks=[earlystop_callback])
---------------------------------------------------------------------
* The code above was programmed by the author for the analysis.
** The code above may change a little according to changes in the Google Colab environment.
*** A rigorous text preprocessing and labeling should be preceded prior to the implementation of the above process.
B4 GPT-2 Test code (Google Colab Python 3)
test_data = pd.read_csv('/content/test.csv')
test_data = test_data.dropna()
test_data.head()
--------------------------------------------------------------------
test_data_sents = []
test_data_labels = []
for test_sent, test_label in test_data[['text','label']].values:
test_tokenized_text = vocab[tokenizer(clean_text(test_sent))]
tokens = [vocab[vocab.bos_token]]
tokens += pad_sequences([test_tokenized_text],
SENT_MAX_LEN,
value=vocab[vocab.padding_token],
padding='post').tolist()[0]
tokens += [vocab[vocab.eos_token]]
test_data_sents.append(tokens)
test_data_labels.append(test_label)
test_data_sents = np.array(test_data_sents, dtype=np.int64)
test_data_labels = np.array(test_data_labels, dtype=np.int64)
-------------------------------------------------------------------------------------------------------------------------------------------------------------------------------
print("num sents, labels {}, {}".format(len(test_data_sents), len(test_data_labels)))
--------------------------------------------------------------------
results = cls_model.evaluate(test_data_sents, test_data_labels, batch_size=1024)
print("test loss, test acc: ", results)
---------------------------------------------------------------------
* The code above was programmed by the author for the analysis.
** The code above may change a little according to changes in the Google Colab environment.
*** A rigorous text preprocessing and labeling should be preceded prior to the implementation of the above process.
Appendix C
Table C1 16th National Assembly (May 30, 2000–May 29, 2004)

Table C2 17th National Assembly (May 30, 2004–May 29, 2008)

Table C3 18th National Assembly (May 30, 2008–May 29, 2012)

Table C4 19th National Assembly (May 30, 2012–May 29, 2016)

Table C5 20th National Assembly (May 30, 2016–May 29, 2020)

Table C6 21st National Assembly (May 30, 2020–May 29, 2024)

* Party classification is based on the second half of each National Assembly, excluding independent politicians. The boldface refers to the two major parties and the numbers in brackets refers to the number of seats.
Appendix D

Figure D1. Bill passage rate, 15th National Assembly to 20th National Assembly
Data from: Bill Information (https://likms.assembly.go.kr/bill/stat/statFinishBillSearch.do)

Figure D2. Bill passage rate (proposed by administrations), 15th National Assembly to 20th National Assembly
Data from: Bill Information (https://likms.assembly.go.kr/bill/stat/statFinishBillSearch.do)

Figure D3. Bill repeal rate (proposed by administrations), 15th National Assembly to 20th National Assembly
Data from: Bill Information (https://likms.assembly.go.kr/bill/stat/statFinishBillSearch.do)

Figure D4. Bill passage rate (proposed by lawmakers), 15th National Assembly to 20th National Assembly
Data from: Bill Information (https://likms.assembly.go.kr/bill/stat/statFinishBillSearch.do)

Figure D5. Bill repeal rate (proposed by lawmakers), 15th National Assembly to 20th National Assembly
Data from: Bill Information (https://likms.assembly.go.kr/bill/stat/statFinishBillSearch.do)
Seungwoo Han (seungwoo.han@rutgers.edu) is a Ph.D. candidate at Rutgers University, The State University of New Jersey. His research interests include comparative political economy, inequality, political behavior, social polarization, and welfare state. For the analysis, he is specialized in quantitative methods such as econometrics, Big Data, machine learning, neural networks, natural language processing and computer vision.