Suicide is a severe public health problem, with approximately 800 000 people dying by suicide each year globally.1 The World Health Organization estimates that the global suicide rate is 10.6 per 100 000 person-years.2 The immediate time after hospital discharge for a mental health condition is a critical period during which suicide risk is high.Reference Chung, Ryan, Hadzi-Pavlovic, Singh, Stanton and Large3,Reference Chung, Hadzi-Pavlovic, Wang, Swaraj, Olfson and Large4 The rate of suicide in the first month after psychiatric hospital discharge is reported to be 2060 per 100 000 person-years.Reference Chung, Hadzi-Pavlovic, Wang, Swaraj, Olfson and Large4 The suicide rate among persons in the first month after discharge from a psychiatric hospital is over 190 times the global suicide rate.2,Reference Chung, Hadzi-Pavlovic, Wang, Swaraj, Olfson and Large4 The immediate period after discharge represents a unique opportunity for prevention to reduce suicide deaths among this vulnerable population.
Suicide prediction
Our understanding of the factors that predict heightened risk of suicide after psychiatric hospital discharge remains limited. Previous studies documented increased postdischarge suicide risk among persons who have a history of self-harm, affective disorders, relationship problems, job loss and are living alone.Reference Qin and Nordentoft5,Reference Olfson, Wall, Wang, Crystal, Liu and Gerhard6 Although many risk factors for suicide have been documented, a 2017 meta-analysis of the past 50 years of research on suicidal thoughts and behaviours found that we still cannot accurately predict who will die by suicide.Reference Franklin, Ribeiro, Fox, Bentley, Kleiman and Huang7 Most studies examined a small number of risk factors, but accurate suicide prediction likely requires examination of hundreds of risk factors and their interactions. However, conventional parametric statistical techniques are not designed to examine large, highly correlated sets of predictors, or to detect interactions among predictors without a priori specification. Machine learning methods can detect complex patterns and return useful algorithms for predicting suicide, thus enabling the development of novel suicide risk profiles that include constellations of predictors. Furthermore, the development of prediction models in high-risk groups, such as persons who have been admitted to psychiatric hospital, is likely to improve relevance and acceptability to clinicians who work with this population.Reference Fazel and O'Reilly8
Kessler and colleagues developed algorithms to estimate suicide risk among USA army soldiers after a stay in hospital, and in the USA Veterans Health Administration system.Reference Kessler, Warner, Ivany, Petukhova, Rose and Bromet9,Reference Kessler, Bauer, Bishop, Demler, Dobscha and Gildea10 However, despite this contribution, several gaps remain. First, few studies have focused on predicting suicide in the relatively short 30-day window of interest to clinicians.Reference Franklin, Ribeiro, Fox, Bentley, Kleiman and Huang7 One reason for this is the lack of an adequate sample size, given that suicide is rare. Second, findings from USA army members and veterans may not be generalisable to the broader population of people who are admitted to a psychiatric hospital. Third, little is known if risk profiles of suicide after a psychiatric hospital admission differ among men and women. Previous research predicting postdischarge suicides by using machine learning did not examine gender differences, which is likely because of the smaller proportion of women in the military than men. However, there may be different risk profiles of suicide in men and women, since there are well-established gender differencesReference Weissman, Bland, Canino, Greenwald, Hwu and Joyce11 in suicide risk and risk factor distributions.Reference Miranda-Mendizabal, Castellví, Parés-Badell, Alayo, Almenara and Alonso12
Aims of study
The purpose of this study was to identify novel interactions and variables that predict suicide in the 30 days after discharge from a psychiatric hospital, in men and women. We leveraged Danish registry data captured over a 20-year period, encapsulating all diagnoses, surgeries, medication prescriptions and demographic/social register information. We used machine learning methods, including classification trees and random forests, to achieve our dual goals of characterising interactions between variables and elucidating novel predictors of suicide after discharge from a psychiatric hospital.
Method
Study sample
The source population was all individuals born or residing in Denmark as of 1 January 1995. The start of the study period coincides with the switch from the ICD-8 to the ICD-10 in 1994, and the start of reporting of all hospital out-patient clinic visits to the Danish National Patient Registry, covering all Danish hospitals in 1995.Reference Schmidt, Schmidt, Sandegaard, Ehrenstein, Pedersen and Sørensen13 We implemented a case–cohort design because it is an efficient approach for studying rare outcomes.Reference Barlow, Ichikawa, Rosner and Izumi14 We did not match cases and subcohort members, to allow for maximum variability in the predictors in the analysis. Cases were all individuals who died by suicide and had been hospitalised for a psychiatric disorder within 30 days before their death between 1 January 1995 and 31 December 2015 in Denmark (n = 1205). The comparison subcohort was a 5% random sample of individuals in Denmark on 1 January 1995, and who had a first hospital admission for a psychiatric disorder between 1 January 1995 and 31 December 2015 in Denmark (n = 24 559). We included persons who were hospitalised for the following disorders, as recorded by two-digit ICD-10 codes from the Danish Psychiatric Central Research RegisterReference Mors, Perto and Mortensen15 and Danish National Patient Registry:Reference Schmidt, Schmidt, Sandegaard, Ehrenstein, Pedersen and Sørensen13 mental disorders due to known physiological conditions (F01–F09); substance use disorders (F10–F19); schizophrenia (F20–F29); mood disorders (F30–F39); anxiety, dissociative, stress-related and somatoform disorders (F40–F48); behavioural syndromes associated with physiological disturbances and physical factors (F50–F59); personality and behaviour disorders (F60–F69); behavioural and emotional disorders with onset usually occurring in childhood and adolescence (F90–F98) and unspecified mental disorders (F99). We used central personal registry numbers that are unique, individual-level identifiers assigned to all Danish residents to link data across Danish administrative and medical registries.Reference Schmidt, Pedersen and Sørensen16 We used the Danish Civil Registration System to randomly select comparison subcohort members.Reference Pedersen17
Outcome
We obtained suicide cases by ICD-10 codes X60–X84, as recorded in the Danish Cause of Death Registry.Reference Helweg-Larsen18 This registry records data on age of death, manner of death (e.g. natural, suicide), place of death and autopsy results.Reference Helweg-Larsen18 A validation study confirmed suicide as the cause of death for 92% of the deaths recorded as suicides.Reference Tøllefsen, Helweg-Larsen, Thiblin, Hem, Kastrup and Nyberg19
Predictors
We examined the following variables as predictors in the machine learning models: age, marital status, immigration status, citizenship, family suicide history (parent or spouse), employment, income, mental disorders, physical health disorders, surgeries, prescription drugs and psychotherapy. We used the Danish Civil Registration SystemReference Pedersen17 to obtain data on age, marital status, immigration status, generation of citizenship and family suicide history. We used the Integrated Database for Labor Market ResearchReference Timmermans20 and Income Statistics Register to obtain baseline data on employment and income.Reference Baadsgaard and Quitzau21 We ascertained psychiatric disorder diagnoses by using two-digit ICD-10 codes from the Danish Psychiatric Central Research RegisterReference Mors, Perto and Mortensen15 and Danish National Patient Registry.Reference Schmidt, Schmidt, Sandegaard, Ehrenstein, Pedersen and Sørensen13 We also used the Danish National Patient Registry to obtain physical health diagnoses, as recorded by second-level ICD-10 groupings. Surgery procedure codes from the Danish National Patient Registry were examined according to the body system. We obtained data on prescription drugs from the Danish National Prescription Registry.Reference Kildemoes, Sørensen and Hallas22 Prescription drugs for this study were coded according to level three Anatomical Therapeutic Classification codes. All codes analysed are in the Supplementary Appendix, available at https://doi.org/10.1192/bjp.2021.19.
Statistical analyses
The time-varying predictors for both cases and the comparison subcohort members were defined 30 days after discharge from psychiatric hospital. Cases were persons who died by suicide in the 30 days after discharge. For cases, we dummy-coded variables to create time-varying predictors with intervals of 0–6, 0–12, 0–24 and 0–48 months before the date of suicide. To compute the prevalence of each predictor in the person-time that gave rise to cases for the comparison subcohort, we used the date 30 days after discharge to calculate the prevalence of predictors in the 0–6, 0–12, 0–24 and 0–48 months before that date. For example, for a member of the comparison subcohort who was discharged from a psychiatric hospital on 1 December 2010, we calculated the prevalence of predictors 0–6, 0–12, 0–24 and 0–48 months before 31 December 2010 (i.e. 31 December 2010 is 30 days after the discharge date). Time intervals were chosen to be consistent with intervals used in previous research that used machine learning for suicide prediction.Reference Kessler, Warner, Ivany, Petukhova, Rose and Bromet9,Reference McCarthy, Bossarte, Katz, Thompson, Kemp and Hannemann23–Reference Gradus, Rosellini, Horváth-Puhó, Street, Galatzer-Levy and Jiang25 Age, immigration status, employment and income at baseline were not coded as time-varying predictors. Predictors from all periods were evaluated simultaneously in the models.
We conducted data reduction to avoid overfitting. Overfitting arises when a model finds patterns that are unique to a specific data-set, but are not generalisable to external samples.Reference Hawkins26 We performed data reduction for men and women separately, by removing rare predictors that had fewer than ten observations in any cell of a 2 × 2 contingency table of the predictor and suicide,Reference Kessler, Warner, Ivany, Petukhova, Rose and Bromet9 and removing predictors with negligible associations with suicide (unadjusted odds ratio between 0.9 and 1.1). We removed emergency department diagnoses because of their low positive predictive values.Reference Lühdorf, Overvad, Schmidt, Johnsen and Bach27,Reference Tuckuviene, Kristensen, Helgestad, Christensen and Johnsen28 The initial analytic data-set contained 2563 predictors. After data reduction, the final number of included predictors was 509 for men and 422 for women. The Supplementary Appendix provides the considered and retained predictors.
Given our interests in identifying novel predictors and interactions that accurately predict suicide after discharge from psychiatric hospital, we used recursive partitioning methods that automatise detection of interactions and provide metrics of predictor importance.Reference Strobl, Malley and Tutz29 First, we used classification trees, which are a nonparametric method that builds a decision tree based on predictors and their combinations that result in the highest probability of differentiating cases from non-cases. Classification trees can elucidate interactions among large sets of predictors without a priori specification, and provide a visual depiction of risk factor constellations that predict suicide. However, classification trees are more vulnerable to overfitting than random forests.Reference James, Witten, Hastie and Tibshirani30 To decrease the risk of overfitting, we used ten-fold cross-validation of classification trees. To increase visual interpretability, we set the maximum tree depth and minimum number of observations in any node to five. To address class imbalance, we used equal priors.Reference Kuhn, Johnson, Kuhn and Johnson31 The risk of suicide was calculated for each identified combination of predictors. We used the R package rpart Reference Therneau, Atkinson and Ripley32 to implement classification trees.
Second, we implemented random forests, which are a recursive partitioning method that comprises a set of decision trees generated with bootstrapped samples of the data. Each forest was built with 1000 trees, and a minimum of ten observations were needed to attempt a split. The number of variables sampled as split candidates at each node were 23 for men and 21 for women (i.e. square root of the total number of predictors for men and women; R package randomForest default). To address class imbalance, each individual tree was built using all suicide observations and an equally sized number of randomly selected non-suicide observations, using the sampsize tuning parameter. We used two-fold cross-validation to generate individual-level random forests predicted values. We calculated the mean decrease in accuracy of each variable, which represents the reduction in accuracy if a predictor were permuted.Reference Strobl, Malley and Tutz29 The larger the mean decrease in accuracy of a predictor, the more important it is for accurate prediction of suicide. We used the R package randomForest.Reference Liaw and Wiener33 Although random forests provide metrics of predictor importance, they do not provide a visualisation of interactions between variables as classification trees do. Thus, we leveraged the strengths of both classification trees and random forests to best serve our dual interests in identifying novel predictors and interactions that predict suicide after discharge from psychiatric hospital.
We evaluated prediction accuracy by receiver operating characteristics curve analysis conducted in 1000 bootstrap replicates, to estimate the area under the curve (AUC) and its 95% confidence interval. We also examined the sensitivity and specificity of the classification trees and random forests in detecting suicide. The analyses were conducted separately for men and women. We conducted gender-stratified analyses instead of including gender as a predictor in an analysis of the entire sample, because the latter approach would not reveal gender differences in random forest variable importance, and in classification trees, it would only display separate patterns of risk in men and women at the point that gender is chosen as a splitting variable, but not earlier in the tree. Analyses were conducted in SAS, version 9.4 (SAS Institute) for Windows34 and R, version 3.5.2 in Windows (R Core Team, Vienna Austria, https://www.R-project.org/).35 This study was determined to be exempt from review by the Boston University Institutional Review Board, approved by the Danish Data Protection Agency (record number 2015-57-0002). Use of data from Danish registries does not require informed consent according to Danish law.
Results
Table 1 shows the descriptive characteristics of the study sample. Among men, the mean ages were similar in the suicide cases and the comparison subcohort. Among women, suicide cases were, on average, younger than the comparison subcohort members (mean 52 [s.d. 16] v. 57 [s.d. 24]). Across sexes, suicide cases and subcohort members had similar proportions of immigrant status and persons in a married or registered partnership. Suicide cases were less likely to be in the lowest income quartile compared with subcohort members.
Table 1 Characteristics of the suicide cases and the subcohort, Denmark, 1 January 1995
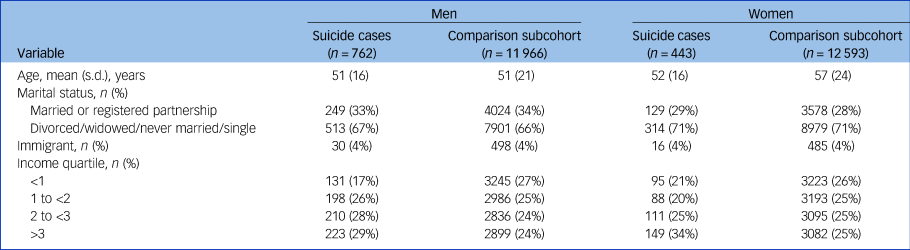
Classification trees
The highest risk of suicide was among men who were not prescribed antidepressants in the 48 months preceding admission to psychiatric hospital, were diagnosed with alcohol-related disorders in the preceding 6 months, were prescribed hypnotics and sedatives, had a poisoning diagnosis (poisoning by, adverse effect of and underdosing of drugs, medications and biological substances) in the preceding 48 months and were prescribed anxiolytics in the preceding 12 months (n = 20; suicide risk 91%). However, men with the same risk profile (i.e. same combination of variables) who were not prescribed anxiolytics in the preceding 12 months (n = 14) had a 0% risk of suicide. This result suggests that in this subgroup, there is an important interaction between this combination of variables and anxiolytics prescriptions. Another stark contrast in suicide risk is among men who were prescribed antidepressants in the prior 48 months and hypnotics and sedatives in the preceding 24 months, were diagnosed with cerebrovascular diseases in the preceding 12 months, and not diagnosed with poisoning in the preceding 48 months. Among men with this risk profile, those who were prescribed drugs used in addictive disorders in the preceding 6 months (n = 14) had a 72% risk of suicide. However, men with the same risk profile, but who were not prescribed drugs used in addictive disorders in the preceding 6 months (n = 112) had a 0% risk of suicide. Figure 1 shows other combinations of predictors that conferred elevated risk of suicide in men (AUC = 0.80, 95% CI 0.78–0.81).

Fig. 1 Classification tree depicting suicide predictors among male patients hospitalised for psychiatric disorders in Denmark, 1995–2015. Each shaded rectangular bin at the bottom (terminal node) represents the group of people with the characteristic profile in the branches above. Within the rectangular bins, n indicates the number of people who had the characteristic profile, and risk indicates the proportion of people in that bin who died by suicide. aPoisoning by, adverse effect of and underdosing of drugs, medications and biological substances.
Among women who were hospitalised for psychiatric disorders, the highest risk of suicide was in women who were prescribed antipsychotics and had a poisoning diagnosis in the preceding 48 months (n = 313, suicide risk 93%). The second highest risk group was women who were prescribed antipsychotics and anxiolytics and diagnosed with a specific personality disorder, but were not diagnosed with poisoning, in the preceding 48 months (n = 172, risk 91%). Another interesting combination of variables was among women who had a poisoning diagnosis in the preceding 48 months, were not prescribed antipsychotics in the preceding 48 months or anxiolytics in the preceding 6 months, and were not in the highest income quartile. Among women with this risk profile, those who had a recurrent major depressive disorder diagnosis in the preceding 6 months (n = 22) had an 86% risk of postdischarge suicide, whereas those who did not have recurrent depression (n = 254) had a 10% risk of postdischarge suicide. Figure 2 shows other combinations of predictors and their associated suicide risks among women (AUC = 0.83, 95% CI 0.80–0.86).

Fig. 2 Classification tree depicting suicide predictors among female patients hospitalised for psychiatric disorders in Denmark, 1995–2015. Each shaded rectangular bin at the bottom (terminal node) represents the group of people with the characteristic profile in the branches above. Within the rectangular bins, n indicates the number of people who had the characteristic profile, and risk indicates the proportion of people in that bin who died by suicide. aPoisoning by, adverse effect of and underdosing of drugs, medications and biological substances.
Random forests
Among men who were hospitalised for a psychiatric disorder, 64–67% (fold one to fold two) of the predictors had a mean decrease in accuracy above zero (mean 3.8, s.d. 3.4). Fifteen predictors were among the top 30 most important predictors in both folds (Fig. 3). The most important variables for predicting suicide included age >30 years, alcohol-related disorders, nicotine dependence, major depressive disorder, unspecified dementia, antidepressants, and reaction to severe stress and adjustment disorders. The AUC for the random forest across folds was 0.82 (95% CI 0.80–0.83).

Fig. 3 Variable importance of suicide predictors among male patients hospitalised for psychiatric disorders in Denmark from split sample cross-validation, 1995–2015. The dark blue dots represent the mean decrease in accuracy (MDA) value in fold one, and the light blue dots represent the MDA value in fold two. The vertical line represents the average of the MDA values of all predictors with nonzero MDA values in folds one and two (3.8). The predictors shown in bold were in the top 30 predictors in folds 1 and 2 for men.
Among women who were hospitalised for a psychiatric disorder, 62–64% (fold one to fold two) of the predictors had a mean decrease in accuracy above zero (mean 2.9, s.d. 2.2). Twelve predictors were among the top 30 most important predictors in both folds (Fig. 4). The most important predictors of suicide among female patients hospitalised for psychiatric disorders included progestogens and oestrogens in combinations, poisoning, age >60 years, receiving a state pension, antipsychotics, bipolar disorder and major depressive disorder. The AUC for the random forest across folds was 0.85 (95% CI 0.83–0.87).

Fig. 4 Variable importance of suicide predictors among female patients hospitalised for psychiatric disorders in Denmark from split sample cross-validation, 1995–2015. The dark blue dots represent the mean decrease in accuracy (MDA) value in fold one, and the light blue dots represent the MDA value in fold two. The vertical line represents the average of the MDA values of all predictors with nonzero MDA values in folds one and two (2.9). The predictors shown in bold were in the top 30 predictors in folds 1 and 2 for women.
Operating characteristics of high-risk thresholds
The predicted probabilities from cross-validated random forests were rank-ordered, and operating characteristics were computed among individuals in the top quintile of the predicted risk distribution. Men in the top 5%, 10% and 20% of predicted risk accounted for 23%, 38% and 59% of all suicide cases among men, respectively (specificity of 96%, 92% and 82%). Women in the top 5%, 10% and 20% of predicted risk accounted for 38%, 52% and 71% of all suicide cases among women, respectively (specificity of 96%, 92% and 82%).
Discussion
This study demonstrates the complexity of the patient profiles that carry a high risk of suicide in the 30 days after discharge from psychiatric hospital, among men and women in a full population sample. Our findings build upon previous work demonstrating that accurate suicide prediction requires a complex combination of a large number of psychological, physical and social factors, many of which are time-varying.
For men, there were important interactions between specific risk profiles by anxiolytics and drugs used in addictive disorders. In women, we found an interaction between poisoning and recurrent major depressive disorder that elevates the risk of suicide after discharge from a psychiatric hospital. Poisonings may be capturing some non-fatal suicide attempts in these data, so these results may suggest that women with recurrent major depression and a history of non-fatal suicide attempts are at high risk for postdischarge suicide. These novel interactions should be replicated in future studies and examined in conventional designs aimed at quantifying the causal joint effects of these variables.
A surprising predictor of postdischarge suicide among men in the random forests was nicotine dependence. Nicotine dependence may serve as a proxy for smoking and health problems that are linked with chronic hypoxia and risk-taking, which in turn are associated with an increased risk of suicide.Reference Young36,Reference Riblet, Gottlieb, Hoyt, Watts and Shiner37 Furthermore, smoking may be a form of self-medication for emotional distress, which in itself is a risk factor for suicide.Reference Orlando, Ellickson and Jinnett38 Nicotine dependence may represent a potentially transdiagnostic suicide prevention factor because it appeared among the top most important predictors of postdischarge suicide above many psychiatric disorders. This finding is worthy of additional research to quantify its effect on suicide risk. It is also noteworthy that alcohol-related disorders were important for accurate prediction of suicide in men, but less so in women, according to the random forests and classification trees. Alcohol-related disorders improved prediction accuracy to a small extent in women, but they were not among the top 30 predictors in both folds in cross-validation of the random forests and they did not appear in the classification tree for women. Previous work suggests that male suicide decedents may be more likely to have had alcohol use disorders than female suicide decedents, which may explain our findings.Reference McGirr, Séguin, Renaud, Benkelfat, Alda and Turecki39 A novel predictor identified among women was prescriptions for progestogens and oestrogens in combination. An earlier study found lower suicide mortality in those receiving oestrogen compared with those who were not receiving oestrogen.Reference Petitti, Perlman and Sidney40 However, this association may be explained by the selective prescription of postmenopausal oestrogens to healthier women.Reference Schairer, Adami, Hoover and Persson41 It is noteworthy that these predictors should be interpreted as risk markers and not causal risk factors, given that our analyses were not intended to quantify the causal effect of any of these predictors, but rather to examine their contribution to accurate prediction of postdischarge suicide.
Our random forests’ operating characteristics are comparable with those of a previous study that used machine learning to predict postdischarge suicide among veterans,Reference Kessler, Bauer, Bishop, Demler, Dobscha and Gildea10 which found that the 5% of patients with the highest predicted risk accounted for 32% of suicides in the 1 month after psychiatric hospital discharge. In our study, persons in the highest 5% of predicted suicide risk accounted for 23% of all suicide deaths among men and 38% among women 1 month postdischarge. This suggests that a prevention program delivered to only 5% of hospitalised patients with the highest predicted risk could capture a large proportion of patients who would otherwise die by suicide.Reference Kessler, Bauer, Bishop, Demler, Dobscha and Gildea10
This study has several limitations. We were unable to observe more detailed social data. For many patients, the hospital may represent a respite from intolerably stressful situations, but upon discharge, patients are re-exposed to the same stressors that preceded their hospital stay, such as social isolation, financial difficulties, problematic relationships, dependent care responsibilities and other stressors.Reference Owen-Smith, Bennewith, Donovan, Evans, Hawton and Kapur42 We lacked data on important suicide risk factors, such as trauma exposure, sexual minority status and homelessness. Adequate representation of social conditions and acute emotional states in registry data remains an ongoing challenge. A second limitation is that there may be measurement error of variables, which may decrease model performance and distort variable importance in random forests.Reference Jiang, Gradus, Lash and Fox43 For example, a diagnosis of poisoning by, adverse effect of and underdosing of drugs, medications and biological substances that may include non-fatal drug overdose, including accidental, intentional self-harm, assault or undetermined intent, as well as poisoning owing to adverse effects or underdosing. The broadness of this indicator makes it susceptible to measurement error. We were unable to conduct probabilistic quantitative bias analyses to examine the effect of measurement error because of the computational capacity limitations of the analytic server. A third limitation is that although we were able to perform ten-fold cross-validation for the classification trees, we were unable to do so for random forests. We instead performed two-fold cross-validation of the random forests because the analytic server was unable to conduct ten-fold cross-validation of 1000 trees. The generalisability of these results to the USA remains unclear, but our results are generally consistent with existing USA-based suicide findings.
Our results illustrate the complexity of the interactions between risk factors that elevate suicide risk in the immediate period after psychiatric hospital discharge, and the ways that they differ between men and women. We also highlight surprising, novel factors that emerged as important predictors for accurate classification of postdischarge suicide that are worthy of additional research.
Supplementary material
To view supplementary material for this article, please visit https://doi.org/10.1192/bjp.2021.19.
Data availability
The data used for this study contain sensitive personal information and therefore cannot be made publicly available according to Danish regulations. Requests for data can be made to the Department of Clinical Epidemiology at Aarhus University Hospital.
Author contributions
J.L.G., H.T.S., T.L.L. and E.H.-P. contributed to the acquisition of the data. T.J., J.L.G., A.J.R., B.S. and A.E.S. made substantial contributions to the conception or design of the work. T.J., A.J.R., E.H.-P. and J.L.G. were involved in the analysis. All authors were involved in the interpretation of data, drafting the work or revising it critically for important intellectual content, and provided final approval of the version to be published.
Funding
This work was supported by National Institute of Mental Health grants R01MH109507 (Principal Investigator J.L.G.) and 1R01MH110453-01A1 (Principal Investigator J.L.G.), and the Lundbeck Foundation (grant R248-2017-521, Principal Investigator H.T.S.).The funding source had no role in the design and conduct of the study; collection, management, analysis and interpretation of the data; preparation, review or approval of the manuscript; and decision to submit the manuscript for publication. The authors do not have any conflicts of interest to disclose.
Declaration of interest
None.
eLetters
No eLetters have been published for this article.