Poor dietary intake is a significant contributor to an array of adverse health outcomes, such as diabetes, CVD and certain cancers( Reference He, Nowson and Lucas 1 – Reference Franz, Bantle and Beebe 10 ). The transition from adolescence to adulthood is an important age at which excess weight gain is likely to occur and long-term health habits are established( Reference Nelson, Story and Larson 11 ). As young adults face changing life circumstances, adult responsibilities and demands on their time, unhealthy dietary behaviours are not only prevalent but may increase in frequency during this life stage( Reference Nelson, Story and Larson 11 , Reference Harris, Gordon-Larsen and Chantala 12 ). National surveillance data from the National Health and Nutrition Examination Survey indicate that only 45 % of 19–30-year-old men and 30 % of similarly aged women consume five or more daily servings of fruits and vegetables( Reference Guenther, Dodd and Reedy 13 ). On average, young adults consume approximately two cups of sugar-sweetened beverages per day( Reference LaComb, Sebastian and Enns 14 ). Furthermore, findings from other nationally representative data indicate that young adults, age 18–27 years, are frequent consumers of fast foods, with reported consumption frequency averaging 2·5 times per week( Reference Niemeier, Raynor and Lloyd-Richardson 15 ).
Research indicates that there are likely numerous unhealthy diet-related behaviours that underlie poor food choices among young adults. For example, in a recent study of more than 1600 young adults (mean age: 20·5 years), the majority reported they enjoy and value eating with others, yet 35 % of males and 42 % of females reported lacking time to sit down and eat a meal( Reference Larson, Nelson and Neumark-Sztainer 16 ). Further, regularly eating dinner with others was significantly associated with numerous indicators of healthier dietary intake (i.e. greater intakes of fruits and vegetables), whereas eating on the run was associated with poorer dietary intake (i.e. greater intakes of soft drinks, fast foods and saturated fat). Other research has shown that activities such as watching television or multi-tasking during meals are prevalent among young adults and also associated with less healthy food choices( Reference Laska, Graham and Moe 17 , Reference Barr-Anderson, Larson and Nelson 18 ). Protective factors, such as preparing meals at home, have been shown to be associated with long-term dietary intake throughout the young adult years( Reference Laska, Larson and Neumark-Sztainer 19 ).
Understanding the most prominent risk and protective behaviours that underlie poor dietary outcomes during young adulthood, particularly among college students, is an important step in developing effective behavioural nutrition interventions among this age group. College students are an important group to study, especially given that nearly half of high-school graduates in the USA up to 24 years of age are enrolled in post-secondary institutions; thus these settings provide unique platforms for intervention delivery( 20 ). Important institutions to target include not only traditional four-year universities, but also two-year community and technical colleges, which serve millions of emerging adults, particularly those from lower-income and minority backgrounds( 21 , Reference Adelman 22 ).
To better understand the most prominent risk and protective behaviours underlying poor dietary behaviours at this critical age, the purpose of the present paper was to examine associations between a wide array of meal routines and practices (including food preparation patterns, meal skipping, eating on the run, media use while eating, campus food purchasing and time for meal preparation and eating) and key dietary indicators (fruit and vegetable consumption, frequency of eating fast foods and sugar-sweetened beverage intake) among young adult college students. Through identification of a set of factors that, together, represent risk and protection, we also sought to develop index measures of protective and risky meal routines and practices most strongly associated with dietary intake. Our intent was that these new index measures could be used in future research in this area as a means of data reduction and synthesis. We used data from a large and diverse sample of two- and four-year college students to address these research questions.
Methods
The Student Health and Wellness survey was conducted among public four-year university and two-year community college students in the Twin Cities metropolitan area of Minnesota in the spring of 2010. Research team members approached students on campus in high-traffic areas to invite them to participate in the survey and provided ‘pass codes’ to enter an online survey, which took approximately 30–35 min to complete. The survey assessed a wide array of nutrition- and weight-related attitudes, behaviours and related factors. Accompanying the survey were objective measurements of height, weight and body fat percentage. Students who completed the survey and measurements received a $US 50 gift card and the chance to win an Apple iPod Touch™ in a lottery drawing. The final sample included 1201 participants (two-year: n 598, four-year: n 603). All study protocols were approved by the University of Minnesota Institutional Review Board.
Dietary intake measures (dependent variables)
Fruit and vegetables
Participants self-reported dietary behaviours for the previous 30 d using a validated screener developed by the National Cancer Institute, the Five Factor Screener( 23 ). Fruit and vegetable consumption (in daily cup-equivalents, excluding French fries) was calculated using reported consumption during the past month of 100 % fruit juice, fruit, salad, potatoes, beans, vegetables, tomato sauce and salsa.
Fast foods
Participants were also asked to report how many times in the past week they ate a meal at a fast-food restaurant (like McDonald’s, Burger King, Hardees, etc.), using a standard survey item that has been used extensively in previous literature( Reference French, Story and Neumark-Sztainer 24 , Reference Bauer, Larson and Nelson 25 ). Response options were ‘never’, ‘1–2 times’, ‘3–4 times’, ‘5–6 times’ or ‘7 or more times’. Response options were set to the mid-point of each category, with ‘7 or more times’ assigned a value of 7.
Sugar-sweetened beverages
Participants were asked, ‘During the past month, how often did you consume…’: (i) ‘…regular carbonated soda, pop or soft drinks that contain sugar?’; (ii) ‘…fruit drinks?’; (iii) ‘…sports drinks?’; (iv) ‘…sugar-sweetened coffee drinks?’; and (v) ‘…other sugar-sweetened beverages?’( Reference Nelson and Lytle 26 ). Ten response options ranged from ‘never’ to ‘5 or more times per day’. Responses were scaled to reflect the number of beverages daily over the past month and were used as a continuous variable.
Meal routines and practices measures (independent variables)
The independent variables were classified into five primary constructs, including food preparation, meal routines, use of media while eating, campus food purchasing and time for meals. The variables included in each construct are described below. Survey items were adapted from those used in previous research and other large, longitudinal studies among similar age groups, such as Project EAT (Eating Among Teens)( 27 ) and the IDEA (Identifying Determinants of Eating and Activity) Study( Reference Lytle 28 ), including items that have yielded significant associations with dietary intake in previous work and/or those that have been identified as notable elements of young adults’ meal routines in our formative work in this area.
Food preparation
Food preparation was assessed using three items( Reference Larson, Story and Eisenberg 29 , Reference Larson, Perry and Story 30 ). Participants were asked to report the number of days per week they prepared a meal at home, prepared their dinner and prepared a meal with vegetables. Response options were on a scale of 0–7 d. The three food preparation variables were treated as continuous measures.
Meal routines
Meal routines included three items also adapted from Project EAT( Reference Larson, Nelson and Neumark-Sztainer 16 , Reference Nelson, Larson and Barr-Anderson 31 ). Participants were asked how many days per week they ate an evening meal and how many days per week they ate breakfast. Response options were on a scale of 0–7 d. Participants were also asked how much they agree (5-point Likert scale: ‘strongly agree’ to ‘strongly disagree’) with the following statement: ‘I tend to “eat on the run”’. The three meal routine variables were treated as continuous.
Use of media while eating
Media use while eating was assessed using three items adapted from the IDEA study( Reference Lytle 28 ). Participants were asked, ‘During the past 7 days, how many times did you…’: (i) ‘…watch TV while eating a meal or snack?’; (ii) ‘…work on the computer, read or do schoolwork while eating a meal or a snack?’; and (iii) ‘…play video/computer games while eating a meal or snack?’ Response options were ‘never’, ‘1–2 times’, ‘3–4 times’, ‘5–6 times’ and ‘7 or more times’. Response options were set to the mid-point of each category and used as continuous variables.
Campus food purchasing
Campus food purchasing was assessed with two questions adapted from the IDEA study( Reference Lytle 28 ). Participants were asked, ‘During a normal week, how many days per week do you…’: (i) ‘…buy food from the Campus Center?’ (two-year college participants only) or ‘…get food from another campus restaurant or UDS facility where you pay as you go?’ (four-year college participants only); and (ii) ‘…buy food from a vending machine on campus?’ Response options were on a scale of 0–7 d and each variable was treated as continuous.
Time for meals
Time for meals was assessed with two questions adapted from a previous study( Reference Laska, Graham and Moe 17 ). Time to prepare dinner was measured with the following question: ‘When you eat dinner in your home (or living space), how long does it usually take to prepare your food? For example, this includes from the time you take things out of the refrigerator/freezer/etc. up to the time that you are ready to eat your food’. Response options included: (i) ‘I never prepare dinner in my home or living space’; (ii) ‘About 5 minutes or less’; (iii) ‘About 10 minutes’; (iv) ‘About 20 minutes’; (v) ‘About 30 minutes’; and (vi) ‘More than 30 minutes’. The last two categories were combined.
Time to eat dinner
Time to eat dinner was measured with the following question: ‘How long does your evening meal (dinner) typically last (for example, from the time you start eating until the time you are finished with the meal?’ Response options included: (i) ‘Less than 15 minutes’; (ii) ‘15–30 minutes’; (iii) ‘30–45 minutes’; (iv) ’45 minutes–1 hour’; (v) ‘1–2 hours’; and (vi) ‘More than 2 hours’. The last two categories were combined. Both time to prepare dinner and time to eat dinner were treated as categorical variables, with the referent category being the shortest amount of time of each question.
Demographics
Participants also self-reported their gender, age, race/ethnicity and relationship status, which were used as covariates in adjusted models.
Analysis
The analysis consisted of three phases. First, descriptive characteristics were calculated using mean and frequency distributions.
Second, using a series of linear regression models (Models 1–5), the regression of each of the categories of meal routines and practices (i.e. food preparation, meal routines, using media while eating, campus food purchasing and time for meals) v. each of the dietary consumption indicators (fruit and vegetables, fast foods and sugar-sweetened beverages) was performed. Each category of meal routines and practices contained two or three variables, and thus these variables were mutually adjusted within the models. For example, in performing the regression of dietary intake v. food preparation, all three food preparation variables (prepare meals at home, prepare own dinner and prepare meals with vegetables) were entered into the model simultaneously, thus resulting in mutual adjustment for all three variables within the food preparation category. When including sugar-sweetened beverage consumption as a dependent variable in these models, this variable was transformed using a natural logarithm function, given its skewed distribution. Overall, variables that were statistically significant in each model (P<0·05) were carried forward and included in the final models in order to assess both unadjusted and adjusted associations (adjusting for gender, age, attendance at a two- or four-year college, race/ethnicity and relationship status; Models 6–7).
Third, two new index scores were created that were comprised of all of the statistically significant variables associated with fruit and vegetable, fast-food and sugar-sweetened beverage consumption (identified via final Model 7 for each dependent variable). Meal routines and practices variables that were not already on a 7-point scale (i.e. preparing meals at home and eat on the run) were transformed into a 7-point range. One index score was created called the Protective Factors Index, representing factors yielding a beneficial association with dietary intake. A second index score called the Risky Factors Index represented factors yielding an association with poorer dietary intake. Both indices were created by summing the variables. Therefore, a high score on the Protective Factors Index represents more behaviours that are supportive of a healthy diet. Alternatively, a high score on the Risky Factors Index represents more behaviours that are harmful for diet. As a final step, linear regression was used to assess the association between the Protective Factors Index and the Risky Factors Index and fruit and vegetable, fast-food and sugar-sweetened beverage consumption.
Ad hoc sensitivity analyses were also conducted by removing data collected from college students who reported residing in dormitories (n 99), in order to understand the potential for differential associations that may exist for those who were living in dormitories v. those who were not.
All analyses were conducted using the statistical software package Stata version 11·1.
Results
There were 1201 survey participants. Those with incomplete data on dietary consumption patterns or meal routines and practices variables were excluded from the analysis, leaving a final sample size of 1013. Available and complete survey data from participants who did not complete the objective height and weight measurements were included in these analyses. Table 1 provides a description of the sociodemographic characteristics of the sample, as well as a summary of key dietary factors and meal routines and practices. The sample population for the study had a mean age of 21 years. Overall, 52 % of participants were males, 47 % were students from two-year colleges and 57 % were non-white. Participants reported consuming 2·4 cup-equivalents of fruits and vegetables/d, as well as sugar-sweetened beverages 1·2 times/d and fast foods 1·5 times/week.
Table 1 Sample characteristics of young adult, two- and four-year college students (n 1013), Minneapolis/St. Paul metropolitan area, MN, USA, spring 2010
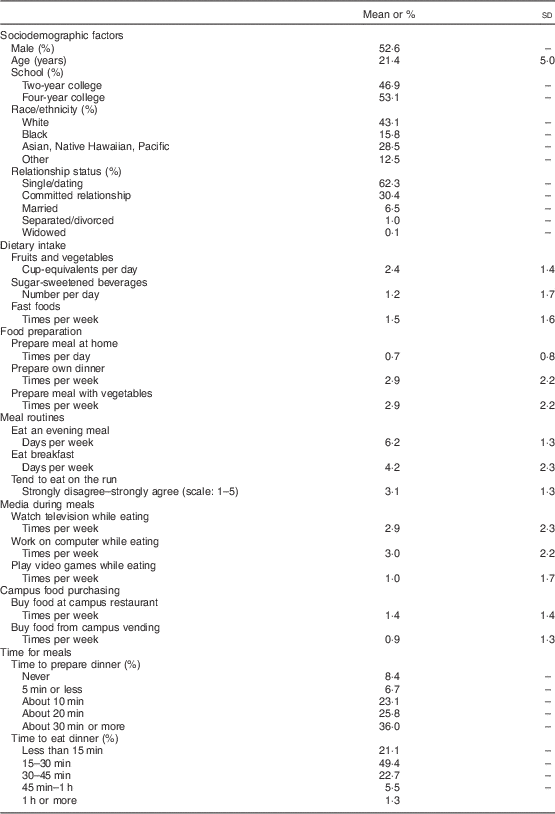
In addition, there were some differences between the study sample and overall student enrolment within each of the two schools. For example, the four-year university sample had a racial/ethnic composition that was 52 % White, 7 % African American, 36 % Asian and 9 % Other, compared with the total enrolled undergraduate population that was 70 % White, 7 % African American, 8 % Asian and 18 % Other. The two-year college study sample also included more racial/ethnic minorities (40 % White, 33 % African American, 21 % Asian, 11 % Other) than the enrolled student population (62 % White, 20 % African American, 12 % Asian, 4 % Other). Additionally, in the four-year university sample there were differences in age (11 % <19 years old, 83 % 19–24 years old, 4 % >24 years old) compared with all enrolled students (10 % <19 years old, 79 % 19–24 years old, 11 % >24 years old). There were also similar differences in age in the two-year sample (18 % <19 years old, 59 % 19–24 years old, 20 % >24 years old) compared with enrolled students overall (6 % <19 years old, 54 % 19–24 years old, 41 % >24 years old). However, there were no observed differences in gender composition (i.e. % male v. % female) when comparing the study sample to the enrolled student populations for either the two-year or four-year school. These findings have been previously reported elsewhere( Reference VanKim and Laska 32 ).
Meal routines and practices varied among participants. For example, on average, participants prepared meals at home less than once per day, although reported preparing their own dinner and preparing a dinner with vegetables approximately 3 times/week. Participants on average ate an evening meal most nights (6·2 d/week), but only ate breakfast 4·2 d/week. Average responses to the statement ‘I tend to eat on the run’ indicated neither agreement nor disagreement (averaging 3·1 on a scale of 1–5). Participants reported both eating while watching television and eating while working on the computer approximately 3 times/week, but eating while playing video games averaged once per week. Participants purchased food from campus restaurants or vending approximately 1–2 times/week. Time spent preparing dinner and/or eating dinner varied considerably.
Table 2 presents the results of the linear regression models of these meal routines and practices and fruit and vegetable consumption. All three food preparation variables were significantly (P<0·05) associated with fruit and vegetable consumption (Model 1). Preparing meals at home and meals with vegetables were positively associated with fruit and vegetable consumption, but preparing one’s own dinner was negatively associated with fruit and vegetable consumption. In the following models (Models 2–5), only eating breakfast (Model 2) and time to prepare dinner (Model 5) yielded significant associations (P<0·05) with fruit and vegetable consumption. In both the full model, unadjusted for sociodemographic characteristics (Model 6), and the fully adjusted final model (Model 7), all three facets of food preparation (more frequent meal preparation at home, preparation of one’s own dinner, preparation of meals with vegetables), as well as more frequent breakfast consumption, remained significantly associated with a greater consumption of fruits and vegetables.
Table 2 Correlates of fruit and vegetable consumption (cup-equivalents of fruit and vegetables per day) among young adult, two- and four-year college students (n 1013), Minneapolis/St. Paul metropolitan area, MN, USA, spring 2010

Coeff., coefficient; Ref., referent category.
Each category was mutually adjusted for Models 1–5 (i.e. by entering three variables assessing food preparation simultaneously into Model 1, these variables are mutually adjusted for each other). Model 6 was a model that included only significant variables from previous models. Model 7 was additionally adjusted for gender, two- or four-year school, race/ethnicity, relationship status and age.
* P<0·05.
Table 3 presents the results of the linear regression models of meal routines and patterns on frequency of fast-food consumption. As in Table 2, food preparation (home meal preparation and meal preparation that included vegetables) was inversely associated with fast-food consumption. Meal routines were also associated with fast-food consumption, with less fast-food consumption among those who ate an evening meal and breakfast, and more fast-food consumption among those who reported eating on the run. Watching television and playing video games while eating were also associated with higher fast-food consumption, as was purchasing food on campus. Compared with never preparing dinner, spending a minimal amount of time preparing dinner (i.e. 10 min or less) was associated with slightly greater fast-food consumption. Model 6 presents the results of the full unadjusted model and Model 7 presents those of the fully adjusted model. In Model 7, meal routines, watching television while eating and campus food purchasing were all significantly associated with fast-food consumption.
Table 3 Correlates of fast-food consumption (number of times fast foods eaten per week) among young adult, two- and four-year college students (n 1013), Minneapolis/St. Paul metropolitan area, MN, USA, spring 2010

Coeff., coefficient; Ref., referent category.
Each category was mutually adjusted for Models 1–5 (i.e. by entering three variables assessing food preparation simultaneously into Model 1, these variables are mutually adjusted for each other). Model 6 was a model that included only significant variables from previous models. Model 7 was additionally adjusted for gender, two- or four-year school, race/ethnicity, relationship status and age.
* P<0·05.
Table 4 presents the results of the linear regression models of meal routines and patterns on sugar-sweetened beverage consumption. Similar to fast-food consumption, preparing a meal at home or with vegetables and eating breakfast were inversely associated with sugar-sweetened beverage consumption. Watching television and playing video games while eating were associated with more sugar-sweetened beverage consumption, as was campus food purchasing. Compared with never preparing dinner, spending some time preparing dinner (about 10 min) was associated with higher consumption of sugar-sweetened beverages. In the fully adjusted final model (Model 7), preparing a meal with vegetables and eating breakfast were inversely associated with sugar-sweetened beverage consumption, whereas watching television while eating and campus food purchasing were positively associated with sugar-sweetened beverage consumption.
Table 4 Correlates of sugar-sweetened beverage consumption (in the past month, number of sugar-sweetened beverages per day) among young adult, two- and four-year college students (n 1013), Minneapolis/St. Paul metropolitan area, MN, USA, spring 2010

Coeff., coefficient; Ref., referent category.
Each category was mutually adjusted for Models 1–5 (i.e. by entering three variables assessing food preparation simultaneously into Model 1, these variables are mutually adjusted for each other). Model 6 was a model that included only significant variables from previous models. Model 7 was additionally adjusted for gender, two- or four-year school, race/ethnicity, relationship status and age.
* P<0·05.
Table 5 presents the results of the linear regression models of protective and risky factor indices on fruit and vegetable, fast-food and sugar-sweetened beverage consumption. These new indices were created as the sum of the meal routines and practices variables that were significantly associated with at least one of the three dependent variables of interest. The Protective Factors Index included preparing meals at home, preparing meals with vegetables, eating an evening meal and eating breakfast. The Risky Factors Index included eating on the run, watching television while eating, playing video games while eating, buying food on campus and buying food from campus vending. In both crude and adjusted models, the Protective Factors Index was strongly positively associated with fruit and vegetable consumption, and negatively associated with fast-food and sugar-sweetened beverage consumption. In contrast, the Risky Factors Index yielded significant, positive associations with fast-food consumption and sugar-sweetened beverage consumption. P values for the associations between the Risky Factors Index and fruit and vegetable intake were P=0·10 in the crude and P=0·05 in the adjusted analyses.
Table 5 The association of index scores of correlates and daily fruit and vegetable, weekly fast-food and monthly sugar-sweetened beverage consumption among young adult, two- and four-year college students (n 1013), Minneapolis/St. Paul metropolitan area, MN, USA, spring 2010

Coeff., coefficient.
All adjusted models included gender, two- or four-year school, race/ethnicity, relationship status and age.
† Index represents a summary score of the correlates from Tables 2–4 that were robustly associated with our outcomes of interest. Specifically, the Protective Factors Index included preparing meals at home, preparing meals with vegetables, eating an evening meal and eating breakfast. The Risky Factors Index included eating on the run, watching television while eating, playing video games while eating, buying food on campus and buying food from campus vending.
A sensitivity analysis was conducted removing data collected from students who reported residing in university dormitories (n 99) and re-running all regression models. There were no substantive differences in the results between the models that included or excluded these students. Therefore, all students with complete data on the variables of interest remained in the analyses.
Discussion
The findings from the present study suggest that meal routines and practices are associated with dietary patterns among young adults. The factors most strongly associated with healthier dietary patterns were those related to home food preparation (i.e. routinely preparing meals at home and preparing meals with vegetables) and meal regularity (i.e. routine consumption of both an evening meal and breakfast). In contrast, those factors most strongly associated with poorer dietary patterns included eating on the run, using media while eating (i.e. watching television and/or playing video games) and purchasing food or beverages on campus.
Using the significant associations that emerged from the first series of linear regression models, two new meal routines and practice index scores were created. The Protective Factors Index, as sum of those factors associated with healthier dietary intake, yielded positive associations with fruit and vegetable intake and inverse associations with fast-food and sugar-sweetened beverage intake. Although the behaviours included in the Risky Factors Index were significantly associated with higher consumption of fast foods and sugar-sweetened beverages as expected, the sum of these behaviours did not yield significant associations with fruit and vegetable consumption at the P<0·05 level. Fruits and vegetables may or may not be displaced by other foods consumed as part of the behaviours associated with the Risky Factors Index (for example, energy-dense convenience products typically consumed while eating on the run and away from home), and these patterns of eating behaviour are likely very complex.
The creation of these index measures is valuable because by identifying a set of factors that collectively represent risk and protection, future analyses using these indices will have the opportunity to reduce degrees of freedom by having one index represent a set of several behaviours. Thoughtful approaches to data reduction are particularly advantageous when many independent variables are present in an analysis, as is often the case when modelling complex constructs such as the factors that influence dietary intake. To our knowledge, previous research has only examined these constructs independently, and yet there is a value in aggregation and understanding collective patterns. In order to better understand the young adult age group and to inform intervention strategies, future research is needed to examine the characteristics of young adults who exhibit this series of protective v. risky behaviours, as well as environmental factors that may help facilitate more healthful meal routines and practices. In addition, it will be important to understand how we can help protective factors begin to germinate with individuals or groups.
Overall, the ways in which individuals structure mealtimes and the contextual characteristics of eating may have important influences on the foods they choose to eat. Many aspects of healthy, structured meal routines and practices may be of particular concern to the young adult age group. Previous results from a recent study of young adults (18–23 years of age) showed that a quarter of all eating occasions occurred while watching television, one-third involved other multi-tasking and half occurred alone( Reference Laska, Graham and Moe 17 ), and most participants in this previous study reported not thinking about food choices in advance of eating occasions. Importantly, although many young adults’ eating occasions consisted of a wide range of highly processed, energy-dense, convenience products( Reference Laska, Graham and Moe 17 ), the consumption of healthier foods was more likely to occur in the absence of television viewing or multi-tasking, as well as at home and/or with others. These findings are similar to results of other studies in this area( Reference Barr-Anderson, Larson and Nelson 18 , Reference Sisson, Shay and Broyles 33 – Reference Todd, Mancino and Lin 37 ). The findings from our current study complement and build upon these results, providing additional evidence that it is important to not only focus on what we eat, but also the wide array of factors surrounding how we eat.
Although our study is among the first of its kind and includes data from a large, diverse sample, it has several weaknesses. Our sample was drawn from one geographic region, which may limit generalizability. Self-reported measures are also subject to error and reporting bias. Some of our exposure measures were closely linked with our outcomes of interest (e.g. preparing a meal with vegetables and fruit/vegetable consumption), so the associations observed between these two factors were not entirely surprising. However, the observation of robust associations across a broad array of measures, and the subsequent index score creation that resulted from those findings, marks an important contribution to the scientific literature. Finally, although post-secondary institutional settings may provide an important framework for the delivery of health promotion interventions, it is important to note that a significant proportion of high-risk youth at this age may not enrol in colleges and universities. Future research is needed to understand health behaviours among young adults not attending college.
National guidelines for health in the USA, such as the Dietary Guidelines for Americans( 38 ), focus almost exclusively on what we eat and specific food choices; however, it may also be important to be more directive in developing nutrition messaging on the contextual influences of food choice, specifically the context of how and where we eat. Other countries around the world recognize the importance of such contextual factors in their national guidelines for health. For example, dietary guidelines from other countries include recommendations such as ‘enjoy communication at the table with your family … and participate in the preparation of meals’ and ‘establish a healthy rhythm by keeping regular hours for meals’ (Japan( 39 )), ‘eat calmly, never eat when driving or at work’ (Hungary( 40 )), ‘eat some meal for breakfast’ (Indonesia( 41 )), eat ‘three meals a day’ (Netherlands( 42 )) and ‘enjoy your food!’ (Ireland( 43 )). In the future, the US Dietary Guidelines Advisory Committee should consider directly addressing these aspects of healthy, structured mealtimes in the Dietary Guidelines for Americans, including concepts such as avoiding meal skipping, eating on the run and encouraging home food preparation and family mealtimes. Such factors may play an important role in our dietary patterns and in our long-term health, and nutrition messaging around these issues may be understandable and easy to operationalize by the general public.
Acknowledgements
Financial support: This work was funded by the National Cancer Institute (Principal Investigator M.N.L., grant number K07CA126837), (Principal Investigator R. Jeffery, grant number U54CA116849); and the American Heart Association (Midwest Affiliate Office) (Principal Investigator M.N.L., grant number 2261399). The content of this manuscript is solely the responsibility of the authors and does not necessarily represent the official views of the National Cancer Institute or the American Heart Association. Conflict of interest: None. Authorship: M.N.L. conceptualized the study, directed the data collection and led the writing of the manuscript. M.O.H. conducted the data analyses and contributed to the writing of the manuscript and interpretation of findings. K.L., L.A.L. and M.S. contributed to the development of data collection tools, critical revision of the manuscript and interpretation of findings. Ethics of human subject participation: This study was approved by the University of Minnesota Institutional Review Board.