Background
Mild cognitive impairment (MCI) develops heterogeneously: this clinical syndrome may be an intermediate stage of cognitive decline between healthy cognitive aging and neurodegenerative dementias, such as Alzheimer’s disease (AD) or dementia with Lewy bodies (DLB). However, not all MCI cases progress to dementia, and those that do may differ in their rates of clinical transition.
Identifying specific etiologies in MCI, such as MCI due to AD (MCI-AD) or MCI with Lewy bodies (MCI-LB), may help to prospectively identify cases at risk of dementia, with the latter possibly having a greater annual risk of clinical transition to dementia (Hamilton et al., Reference Hamilton2021). However, there is considerable variability in clinical prognosis within MCI etiologies, not just across them. Specific pathophysiological mechanisms may underlie these associations with progression to dementia, irrespective of etiology; previous research has suggested that abnormalities on electroencephalography (EEG) recordings may be associated with risk-associated clinical features of dementia—visual hallucinations and cognitive fluctuations (Hamilton et al., Reference Hamilton2021; Law et al., Reference Law2020)—and so may predict dementia onset across etiologies.
Such EEG abnormalities, including qualitative abnormalities and slowing on quantitative EEG (qEEG), have been identified in MCI-AD and MCI-LB as well as their respective dementias, suggesting that these may precede the onset of clinical dementia in some cases. Profiles of abnormalities may differ between etiologies, often being greater in MCI-LB and DLB (Law et al., Reference Law2020), but may also differ within these syndromes: theta/alpha ratio, previously shown to be greater in MCI-LB than MCI-AD and healthy controls (Massa et al., Reference Massa2020; Schumacher et al., Reference Schumacher2020), has been associated with shorter time to dementia in MCI patients who developed DLB (van der Zande et al., Reference van der Zande2020). Theta/alpha ratio may therefore be a useful qEEG measure to predict the onset of dementia in prospectively assessed MCI, particularly when Lewy body disease is suspected.
We, therefore, aimed to assess whether slowing of qEEG, measured by increased theta/alpha ratio, would predict the onset of clinical dementia in a prospective MCI cohort, hypothesizing that a greater shift towards slower frequencies would be associated with a greater risk of dementia.
Methods
Participants
Patients
One-hundred and three participants with MCI were included in a longitudinal study, as described previously (Schumacher et al., Reference Schumacher2020). These were recruited from older persons healthcare and memory services in North East England. All were aged ≥60 years, had a health service diagnosis of MCI, and were considered for eligibility if their medical records reported the possible presence of any core clinical features of DLB (complex visual hallucinations, rapid eye movement sleep behavior disorder [RBD], cognitive fluctuations, or parkinsonism), or any other clinical feature supportive of DLB, but also found in AD (e.g. mood change, sleep disturbance, or autonomic dysfunction). Participants provided written informed consent prior to detailed initial clinical assessment, and were subsequently excluded if this identified the presence of dementia at baseline, absence of objective cognitive impairment, presence of parkinsonism for longer than 12 months prior to the onset of cognitive symptoms, or presence of possible frontotemporal or vascular etiologies. This study received ethical approval from the Newcastle and North Tyneside 2 Research Ethics Committee (15/NE/0420).
Comparators
Thirty-four healthy comparators were recruited from local dementia research participation services, or from friends or families of MCI participants. Inclusion criteria were being age ≥ 60, and being cognitively healthy with no known brain disease. All participants in both groups were required to be medically stable at baseline assessment.
Table 1. Baseline characteristics of cohort with qEEG available

Mean (SD), median [range], or count (%).
Design
All participants underwent detailed assessment at baseline, and longitudinal follow-up (initially at 12-month intervals, but with adaptive scheduling necessitated by COVID-19 from 2020). For healthy comparators, follow-up assessments took place after approximately 12 and 30 months from baseline. For MCI patients, follow-up assessments took place approximately 12, 24, and 42 months from baseline.
Cognitive and clinical assessment
Participants underwent detailed neuropsychological assessment at baseline and follow-up, as described previously (Donaghy et al., Reference Donaghy2020). Participants were also assessed in a clinical interview by a study research nurse or medical doctor at baseline and follow-up. This included measures of cognitive function, daily independence, and clinical features of MCI-LB to be assessed by an expert panel of old age psychiatrists (see below).
Imaging
All participants from both MCI and cognitively healthy groups were offered 123I-Nfluoropropyl-2β-carbomethoxy-3β-(4-iodophenyl) single photon emission computed tomography (FP-CIT SPECT) and 123iodine-metaiodobenzylguanidine (MIBG) at baseline, with FP-CIT also repeated at first follow-up. FP-CIT imaging in this cohort has been described previously in detail (Roberts et al., Reference Roberts2020); briefly, images were visually rated as normal or abnormal by a panel of trained image analysts, blind to diagnostic information. Delayed MIBG images were separately quantified as described previously (Roberts et al., Reference Roberts2021), blind to diagnosis, using a heart:mediastinum ratio abnormality cutoff of <1.86. Results for each imaging modality were then incorporated into differential classifications of MCI.
Diagnosis
At baseline and after each follow-up, a three-person panel of experienced old age psychiatrists independently reviewed research notes from the clinical interview, and rated (A) the presence or absence of MCI according to National Institute on Aging at National Institutes of Health and the Alzheimer’s Association criteria (Albert et al., Reference Albert2011), or all-cause dementia and (B) the presence or absence of each of the four core clinical features of MCI-LB (complex visual hallucinations, RBD, cognitive fluctuations, and parkinsonism).
Including these ratings, FP-CIT and MIBG imaging, MCI diagnoses were characterized as either MCI-AD (Albert et al., Reference Albert2011), or MCI-LB according to current research diagnostic criteria (McKeith et al., Reference McKeith2020): probable MCI-LB given the presence of 2+ core clinical features of DLB in MCI, or one core feature and abnormal FP-CIT or MIBG imaging; possible MCI-LB given the presence of 1 core feature of DLB, or 0 core features with abnormal FP-CIT or MIBG; MCI-AD given normal FP-CIT, MIBG, and 0 core features of DLB. These diagnoses were updated after follow-up if there was new clinical information to change previous ratings, emergent clinical features of DLB, or change in the repeat FP-CIT.
A diagnosis of dementia was made when the participant was judged by the panel to no longer function independently, thus not meeting the criteria for MCI. After diagnosis of dementia, participants were withdrawn from further follow-up.
EEG recording and processing
As described previously (Schumacher et al., Reference Schumacher2020), all included participants were offered EEG recording at baseline. Resting state eyes-closed EEG was recorded from 128 electrodes using Waveguard caps (ANT Neuro). Preprocessing was performed using the EEGLAB toolbox in Matlab (R2017a) and included the following steps: bandpass filtering between 0.3 and 54 Hz, segmentation of the EEG into 2-s long nonoverlapping epochs, visual inspection and exclusion of noisy channels and epochs, and independent component analysis for identifying and removing artifacts representing muscular, cardiac, ocular, or electrical line noise. Excluded channels were then replaced using spherical spline interpolation, and the data were average-referenced. From each participant’s EEG, the first 45 artifact-free epochs were selected for further analysis, out of a maximum of 150 epochs. This ensured as many participants could be included as possible, while excluding artifacts related to drowsiness and movements which are more likely further into the recording.
Power spectral density was estimated for each 2-s epoch using Bartlett’s method in Matlab with a frequency resolution of 0.5 Hz and a Hamming window. Mean band power within the theta (4–5.5 Hz) and alpha (8–13 Hz) frequency bands was calculated as described previously (Schumacher et al., Reference Schumacher2020), and the average theta/alpha ratio across all electrodes was computed.
Longitudinal analysis
A competing risks model was estimated with the msm package for R to assess the associations between qEEG slowing, as measured by theta/alpha ratio, and risks of clinical transition from MCI to dementia. This model accounted for death as a competing outcome, with exactly observed transition times, and controlled for additional hazards with a theorized association with a faster decline to dementia including chronological age (centered at 74 years) and baseline global cognitive performance (Addenbrooke’s Cognitive Examination – Revised [ACE-R] Total Score). All MCI subgroups were included under a single MCI state, with etiology included as a covariate due to previously observed associations between the MCI-LB syndrome and risk of dementia onset (Hamilton et al., Reference Hamilton2021). Theta/alpha ratios and ACE-R scores were standardized into Z-scores from the healthy group data, and comparators were not included in the analysis of dementia conversion.
Gender and education, which were not found to significantly predict dementia transitions in a larger cohort (Hamilton et al., Reference Hamilton2021), were not included as covariates.
Data availability
Data supporting these analyses are available upon reasonable request through the Medical Research Council Dementias Platform UK (study: ‘SUPErB’).
Results
Demographics and baseline
One-hundred and twenty-three participants had qEEG available (Table 1); data were not available for 3 comparators and 11 MCI.
Longitudinal analysis
Fourteen cases of dementia were observed following baseline diagnosis of MCI (mean = 1.5 years after baseline, SD = 0.5), and five deaths (mean = 1.9 years after baseline, SD = 1.3). Of five cases of dementia due to AD, all five had previous diagnoses of MCI-AD; there were nine cases of probable DLB, all nine had earlier diagnoses of probable MCI-LB.
The estimated transition rates from MCI to dementia given a healthy mean theta/alpha ratio (Z-score of 0) were 14.5% over 1 year, 26.3% over 2 years, and 35.9% over 3 years (controlling for age, cognition, and MCI subtype). However, this hazard significantly increased with increasingly slower qEEG profiles [hazard ratio (HR) = 1.84, 95% CI: 1.01–3.35 per 1 SD increase in theta/alpha ratio, Z = 1.99, p = .046; see Figure 1]. For a 1 SD increase in theta/alpha ratio, this corresponded to estimated MCI to dementia transition rates of 24.1% over 1 year, 40.0% over 2 years, and 50.0% over 3 years.
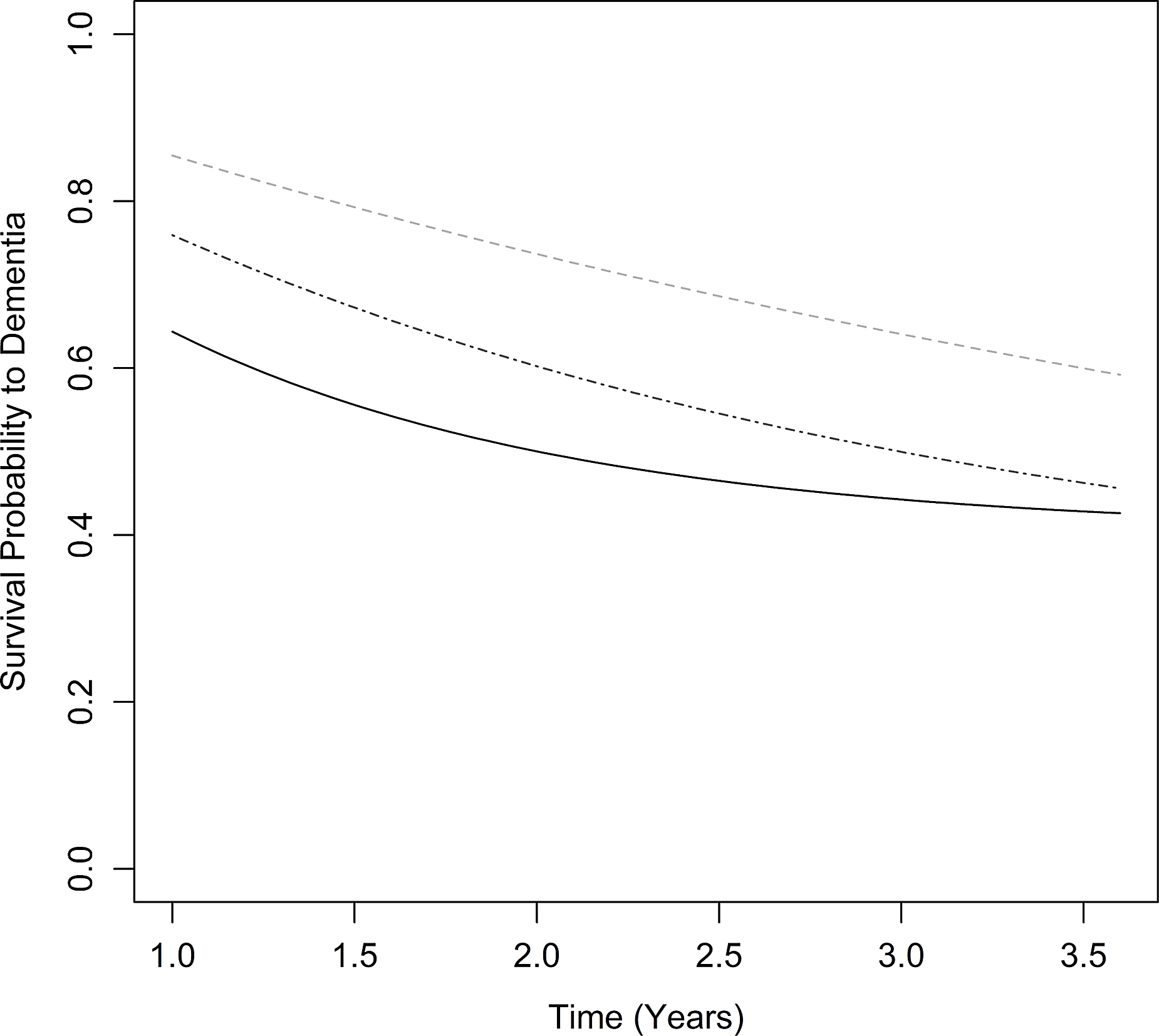
Figure 1. Estimated dementia-free survival in MCI with greater EEG slowing from healthy norm (lighter gray, dashed), +1 SD (darker gray, dash and dot), and +2 SD (black, solid) theta/alpha ratio.
In a sensitivity analysis controlling for the presence of complex visual hallucinations and cognitive fluctuations, previously found to be associated with worse prognosis in MCI in a larger cohort including this sample, this effect was no longer evident (HR = 1.17, 95% CI: 0.66–2.07). However, there was no clear association between theta/alpha ratio in MCI-LB and severity of either visual hallucinations as rated by the North-East Visual Hallucinations Inventory (rho = −0.1, p = .607) or cognitive fluctuations as rated by the Dementia Cognitive Fluctuation Scale (rho = 0.1, p = .517).
In a further analysis instead controlling for use of cholinesterase inhibitors in MCI, again this effect was no longer evident (HR = 1.80, 95%CI: 0.97–3.32). MCI-LB were more likely to be in receipt of cholinesterase inhibitors than MCI-AD, but within diagnostic groups, there were no significant differences found with a Mann–Whitney U test in theta/alpha ratio between those receiving and those not receiving cholinesterase inhibitors (MCI-AD: W = 61, p = .163; MCI-LB: W = 277, p = .223).
Discussion
We hypothesized that greater qEEG slowing would predict transition to dementia; our finding that an increased theta/alpha ratio was associated with an increased hazard of dementia per year supported this. These findings are consistent with previous observations of a shorter time to DLB from MCI with a greater theta/alpha ratio (van der Zande et al., Reference van der Zande2020), and suggest that regardless of etiological classification, qEEG slowing may anticipate the onset of dementia in prospectively identified MCI; a clinically complex group with uncertain prognosis.
There may be a mediating role of the pathophysiological mechanisms underlying EEG abnormalities in the relationship between neurodegenerative etiology, clinical features, and longitudinal prognosis. Previous research has suggested that such EEG abnormalities in MCI may reflect loss of cholinergic drive associated with nucleus basalis of Meynert degeneration; greater cholinergic dysfunction in MCI-AD or MCI-LB may therefore be associated with faster progression to dementia, along with qEEG slowing, cognitive decline, and clinical features of DLB such as fluctuating cognition and visual hallucinations (Law et al., Reference Law2020).
Limitations
While dementia onset was typically soon after EEG recording, and overall follow-up was brief, all participants had clinically judged MCI at the time of recording, and differences in baseline cognitive function were controlled for in the state transition analysis. While the estimated annual dementia incidence in this cohort is consistent with past research on prospectively identified MCI, the absolute number of dementia cases was low.
This provides early, marginal evidence that electrophysiological biomarkers may anticipate onset of dementia in MCI across LB and AD etiologies in prospective settings. This extends previous findings from functional magnetic resonance imaging studies (Schumacher et al., Reference Schumacher, Thomas, Peraza, Firbank, O’Brien and Taylor2021) to suggest that early-identified functional abnormalities may anticipate clinical progression in neurodegenerative disease.
Conflicts of interest
None.
Authors’ roles
C. Hamilton undertook data collection, analysis of longitudinal data, and drafted the manuscript.
J. Schumacher processed and analyzed qEEG data, and co-wrote the manuscript.
F. Matthews supervised data analysis and reviewed the manuscript’s intellectual content.
J.P. Taylor co-designed the study, contributed to the diagnostic panel, and reviewed the manuscript’s intellectual content.
L. Allan co-designed the study and reviewed the manuscript’s intellectual content.
N. Barnett contributed to data collection and reviewed the manuscript’s intellectual content.
R. Cromarty contributed to data collection and reviewed the manuscript’s intellectual content.
P. Donaghy co-designed the study, contributed to the diagnostic panel, and reviewed the manuscript’s intellectual content.
R. Durcan contributed to data collection and reviewed the manuscript’s intellectual content.
M. Firbank contributed to data collection and reviewed the manuscript’s intellectual content.
S. Lawley contributed to data collection and reviewed the manuscript’s intellectual content.
J. O’Brien co-designed the study and reviewed the manuscript’s intellectual content.
G. Roberts contributed to data collection and reviewed the manuscript’s intellectual content.
A. Thomas co-designed the study, supervised data collection, contributed to the diagnostic panel, and reviewed the manuscript’s intellectual content.
Acknowledgements
The authors would like to acknowledge the assistance of the National Institute for Health Research (NIHR) Clinical Research Network North East and Cumbria in recruitment for this study, and the research support secretary Ms Helen Kain. GE Healthcare provided the FP-CIT radioligand for use in this investigator-led study.