Introduction
Depression is associated with morbidity as well as disability among the elderly (Cole and Dendukuri, Reference Cole and Dendukuri2003). They constitute a major public health problem worldwide and their prevalence rates range between 10 and 55% (Sherina et al., Reference Sherina, Rampal and Mustaqim2004; Chi et al., Reference Chi2005; Tsai et al., Reference Tsai, Yeh and Tsai2005; Khattri and Nepal, Reference Khattri and Nepal2006; Kaneko et al., Reference Kaneko, Motohashi, Sasaki and Yamaji2007). The long-term prognosis of geriatric depression is bleak with incomplete recovery (Jhingan et al., Reference Jhingan, Sagar and Pandey2001) and higher relapse rates (Mitchell and Subramaniam, Reference Mitchell and Subramaniam2005). Along with the physiological and psychological changes associated with aging, changes in the associated risk factors also modify the prevalence and prognosis of geriatric depression (van't Veer-Tazelaar et al., Reference van't Veer-Tazelaar2008). Medical co-morbidity (Jongenelis et al., Reference Jongenelis, Pot, Eisses, Beekman, Kluiter and Ribbe2004) and cognitive impairment (Steffens and Potter, Reference Steffens and Potter2008) have a complex bidirectional relationship with geriatric depression.
Available epidemiological studies on geriatric depression have mostly diagnosed depression by short screening instruments. The low positive predictive value (Arthur et al., Reference Arthur, Jagger, Lindesay, Graham and Clarke1999) as well as doubtful external validity of screening instruments (Kørner et al., Reference Kørner2007) may yield many false positive cases and may inflate the prevalence rates of geriatric depression in community settings. Concerns regarding the different cut-off values used to diagnose depression across various settings also exist (McGivney et al., Reference McGivney, Mulvihill and Taylor1994; Arthur et al., Reference Arthur, Jagger, Lindesay, Graham and Clarke1999). Many studies have not employed structured assessment of depression, cognitive function and disability (Osborn et al., Reference Osborn2003; Sherina et al., Reference Sherina, Rampal and Mustaqim2004; Chi et al., Reference Chi2005; Tsai et al., Reference Tsai, Yeh and Tsai2005; Khattri and Nepal, Reference Khattri and Nepal2006; Kaneko et al., Reference Kaneko, Motohashi, Sasaki and Yamaji2007). Community studies from India on depression among the elderly are sparse. The only available Indian study employing the Geriatric Depression Scale (GDS), a screening tool, in a small sample of elderly has reported prevalence of up to 45.9% (Jain and Aras, Reference Jain and Aras2007). Another larger Indian study evaluating the Hindi version of the GDS studied only the depressive symptoms and not the depressive disorders (Ganguli et al., Reference Ganguli, Dube, Johnston, Pandav, Chandra and Dodge1999). We aimed to study the nature, prevalence and factors associated with geriatric depression in a rural south Indian community with an adequate sample size and with detailed as well as structured methods of assessment.
Methods
Study design
We employed a cross-sectional study to examine the nature and prevalence of geriatric depression in a rural community. We used a case control design framework to identify factors associated with geriatric depression. This study was part of the 10/66 Dementia Research Group population based studies. The methodology employed in this study has been reported elsewhere (Jacob et al., Reference Jacob, Senthil Kumar, Gayathri, Abraham and Prince2007a) and is only briefly mentioned here.
Setting
Kaniyambadi block of Vellore district is in the southern Indian state of Tamil Nadu. It is spread over 127.4 square kilometers with 85 villages housing a total population of 110,000. The Department of Community Health, Christian Medical College, Vellore has been running a community health program in this block for the past five decades. It has developed a four-tier monitoring and computerized surveillance system of the residents of Kaniyambadi block, which is updated monthly. The community health workers, who live within the various village communities, provide the detailed statistics (Joseph et al., Reference Joseph, Joseph and Kamaraj1991). They are supervised by public health nurses and physicians.
Recruitment of participants
Participants were identified using a computerized list and a door-to-door survey. We invited all elderly people over 65 years of age to participate in this study.
Assessment
The following instruments were employed to assess the participants: (i) Geriatric Mental State (GMS; Copeland et al., Reference Copeland, Dewey and Griffith-Jones1986), (ii) Community Screening Instrument for Dementia (CSID; Hall et al., Reference Hall, Hendrie and Brittain1993; Reference Hall, Gao, Emsley, Ogunniyi, Morgan and Hendrie2000), (iii) World Health Organization Disability Assessment Scale II (WHODAS II; World Health Organization, 2001), (iv) Modified CERAD 10-word list learning task (Ganguli et al., Reference Ganguli1996), (v) History and Aetiology Schedule Dementia Diagnosis and subtype (HAS-DDS; Copeland et al., Reference Copeland, Prince, Wilson, Dewey, Payne and Gurland2002), (vi) Neuropsychiatric Inventory, and (vii) a structured proforma to assess socio-demographic characteristics, medical history and anthropometrics.
Translation of instruments, training of personnel and quality control procedure
The Tamil versions of the GMS (Rajkumar et al., Reference Rajkumar, Kumar and Thara1997) and WHODAS II (World Health Organization, 2001) have been employed in earlier studies. The translation of the other instruments followed standard procedure. The training of research staff, data collection and quality control procedures were in accordance with 10/66 norms for population-based studies (Prince et al., Reference Prince, Acosta, Chiu, Scazufca and Varghese2003). The Institutional Review Board of Christian Medical College, Vellore, approved the study.
Data analysis
We initially analyzed the socio-demographic data, medical history, psychopathology, cognitive profile and the disability status using descriptive statistics. We derived the psychiatric diagnoses based on the computerized algorithms developed by the 10/66 Dementia Research Group (Prince et al., Reference Prince, Acosta, Chiu, Scazufca and Varghese2003). We converted the continuous exposure variables to categorical measures by splitting them into two categories using their median values (Ragin, Reference Ragin1994). We calculated the odds ratios with 95% confidence intervals for various risk factors. We performed multivariate analysis using determinants, which were significant on univariate analysis, employing conditional logistic regression with the statistical software package SPSS 16.0.
Results
Participant characteristics
The details of recruitment of participants are shown in Figure 1. We recruited 1000 participants and the overall response rate was 97.75%. The socio-demographic, psychiatric and disability profiles of the participants are shown in Table 1. The majority of the participants were women, lacking formal education, widowed and from the low-income category. The average age of the participants was 72.5 (SD 5.8) years.
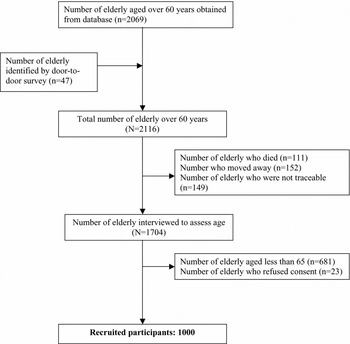
Figure 1. Flowchart of recruitment of participants.
Table 1. Socio-demographic and psychosocial profiles of the participants (n = 1000)

Rs. = rupees; DSM-IV = Diagnostic and Statistical Manual of Mental Disorders, 4th edition; WHODAS = World Health Organization Disability Assessment Scale.
Nature and prevalence of geriatric depression
The prevalence of any ICD-10 (World Health Organization, 1992) depressive episode in our sample was 12.7% (95% CI 10.64–14.76 %). Among those 127 participants who had geriatric depression, 32 had a mild depressive episode (3.2%; 95% CI 2.11–4.29%), 76 had a moderate depressive episode (7.6%; 95% CI 5.96–9.24%), and 19 had a severe depressive episode (1.9%; 95% CI 1.05–2.75%). The prevalence of major depression according to DSM-IV (American Psychiatric Association, 1994) criteria was 5.2% (95% CI 3.82–6.58). The prevalence of suicidal ideation within the previous month was 2.1% (95% CI 1.21–2.99). The prevalence of dementia defined by DSM-IV was 0.8% and by the education-adjusted 1066 criteria was 10.6%.
Correlates of geriatric depression
The correlates of geriatric depression are shown in Table 2. Low income, experiencing hunger within the previous one month, having diabetes, cardiac illnesses, transient ischemic attacks and head injury were correlated with geriatric depression after adjustment for the effects of age, gender, education and the other determinants using conditional logistic regression analysis. Having more confidants had a significant protective effect for depressive disorders. Female gender, age, hypertension, cognitive impairment and more disability were not significantly correlated with geriatric depression.
Table 2. Factors associated with depression in the elderly

a Adjusted odds ratios calculated using conditional logistic regression. The following variables were included in the conditional logistic regression analysis: age, gender, education, low income, experiencing hunger, living alone, living within five miles from siblings, having more confidants, no regular physical exercise, diabetes, tuberculosis, heart problems, transient Ischemic attack, head injury, hearing impairment and smaller mid-arm circumference.
b Walking for at least 500 meters less than six times per week.
c Diagnosis based on DSM-IV criteria.
LOC = loss of consciousness.
When we ascertained major depression according to the DSM-IV criteria, the following variables were significantly associated with geriatric depression after adjustment for the effects of age, gender, education and the other determinants using conditional logistic regression analysis: experiencing hunger within the previous month (OR 2.55; 95% CI 1.24–5.24); diabetes (OR 4.15; 95% CI 1.47–11.73); transient ischemic attacks (OR 3.23; 95% CI 1.31–7.96); past head injury (OR 3.69; 95% CI 1.42–9.55); mid-arm circumference less than 24 cm (OR 4.52; 95% CI 1.72–11.89); and more disability (OR 4.36; 95% CI 1.94–9.81). Having more confidants (OR 0.12; 95% CI 0.04–0.41) was protective.
The significant correlates for recent suicidal ideation, adjusting for age, education and gender using multivariate logistic regression were: being depressed (OR 4.35; 95% CI 1.75–10.78); experiencing hunger within the previous one month (OR 2.71; 95% CI 1.06–6.92); average monthly income of the family less than Rs. 1100 (OR 2.84; 95% CI 1.01–8.00). The significant correlates for more functional disability charcterized by WHODAS II total score more than 25, adjusting for age, education and gender using multivariate logistic regression were: being demented (OR 2.84; 95% CI 1.78–4.53); transient ischemic attacks (OR 4.73; 95% CI 2.18–10.26); hard of hearing (OR 2.23; 95% CI 1.67–2.97); age above 71 years (OR 1.97; 95% CI 1.52–2.56); decreased vision (OR 1.75; 95% CI 1.27–2.40); female gender (OR 1.62; 95% CI 1.19–2.20); lack of formal education (OR 1.52; 95% CI 1.09–2.12); experiencing hunger (OR 1.51; 95% CI 1.04–2.20). A severe depressive episode was not significantly associated with more functional disability (OR 1.10; 95% CI 0.43–2.81).
Discussion
This study examined the factors associated with depression among the elderly in a rural south Indian community. Its strengths include a low refusal rate, adequate sample size and ascertaining depression with a standardized diagnostic interview. The possibility of selection bias was avoided by recruiting all consecutive elderly people and by the low refusal rate. We attempted to minimize the recall bias by collaborating with local community health workers and by using a detailed and structured interview. Its limitation was its cross-sectional design and consequent temporal ambiguity.
Physical illnesses and poverty were significantly correlated with geriatric depression, as in other studies (Cole and Dendukuri, Reference Cole and Dendukuri2003; Osborn et al., Reference Osborn2003; Sherina et al., Reference Sherina, Rampal and Mustaqim2004; Tsai et al., Reference Tsai, Yeh and Tsai2005; Chi et al., Reference Chi2005; Khattri and Nepal, Reference Khattri and Nepal2006; Jain and Aras, Reference Jain and Aras2007; Kaneko et al., Reference Kaneko, Motohashi, Sasaki and Yamaji2007). However, female gender, advancing age and cognitive impairment were not associated with geriatric depression. More surprisingly, depressive disorders did not indicate more functional disability in this population. The depressed individuals in low-income communities rarely subscribe to biomedical causal models and hold more to psychosocial as well as interpersonal explanatory models for depression (Pereira et al., Reference Pereira, Andrew, Pednekar, Pai, Pelto and Patel2007). Such explanatory models may mitigate perceived stigma (Raguram et al., Reference Raguram, Weiss, Channabasavanna and Devins1996). The meager expectations by families of their elderly relatives may also contribute towards high tolerance of depressive symptoms and functional impairment (Jacob et al., Reference Jacob, Senthil Kumar, Gayathri, Abraham and Prince2007b).
Elderly people report depressive symptoms when they are distressed, when they are ill or are worried about the implication of their symptoms. Stressful life events and inability to cope with psychosocial problems may also lead the elderly to mention such symptoms. Consequently, the difficulty in separating distress from depression becomes a major issue (Heath, Reference Heath1999). While psychiatrists suggest that brief screening instruments can easily identify people with depression (Kessler et al., Reference Kessler, Lloyd, Lewis and Gray1999), most general practitioners (GPs) would argue that many of those identified are distressed (Heath, Reference Heath1999). The depression seen in the community and which many GPs encounter is often viewed as a result of personal and social stress, life style choices or as a product of habitual maladaptive patterns of behavior. Consequently, the general population and primary care physicians often hold psychological and social models for depression (Jacob, Reference Jacob2006). Psychiatrists, with their biomedical frameworks, would on the other hand argue for disease models (Kessler et al., Reference Kessler, Lloyd, Lewis and Gray1999). The relationship between poverty, social isolation, physical health and mental health is complex (Kuruvilla and Jacob, Reference Kuruvilla and Jacob2007). However, the cross-sectional and observational nature of this study mandates the need for cohort designs to confirm associations and randomized trials to assess intervention.
Conflict of interest
None.
Description of authors' roles
K. S. Jacob and M. Prince designed the study and supervised data collection and analysis. P. Senthilkumar and K. Gayathri collected the data and assisted with writing the paper. A.P. Rajkumar, P. Thangadurai and K. S. Jacob analyzed the data and wrote the paper.
Acknowledgments
This study is part of the population-based investigations of the 10/66 Dementia Research Group of Alzheimer's Disease International. It was supported by grants from the World Health Organization and the Institute of Psychiatry, London. We thank all the study participants as well as their families and the community health workers for their cooperation, and the research fellows Ms. A. Kanagathara and Ms. C. Symon for data collection.