Unipolar depression is a mental disorder that has experienced an unusual growing over the past 20 years. Based on data of the WHO in 2018, nearly 322 million people of all ages suffered from depression(1). According to age strata, these figures were significantly higher in older adults. In Spain, it is estimated that more than 1·1 million of community-dwelling people were affected by this disorder in 2010(Reference Lara, Garin and Ferrari2).
Depression is considered as one of the main leading cause of disability, increasing personal and community costs(Reference Lopez, Mathers and Ezzati3). In particular, late-life depression negatively affects health outcomes specifically on CVD in adults with Metabolic Syndrome (MetS)(Reference Ortega, Aragonès and Piñol4). Traditional treatments based on antidepressant drugs are not enough to counteract its burden, besides to enhancing a great cost to the health care system(Reference Sobocki, Jönsson and Angst5).
Several risk factors for depressive symptoms in later life have been identified(Reference Rajapakshe, Sivayogan and Kulatunga6). Among them, lifestyle factors, specifically diet, are highlighted. Dietary intake has long been suggested as a contributing factor to this condition. Currently, a growing body of evidence has reported the association between dietary intake patterns and depression status(Reference Quirk, Williams and O’Neil7). In this sense, healthy dietary patterns like Mediterranean Dietary pattern (MedDiet)(Reference Sánchez-Villegas, Martínez-González and Estruch8,Reference Skarupski, Tangney and Li9) or anti-inflammatory dietary patterns(Reference Akbaraly, Kerleau and Wyart10,Reference Bergmans and Malecki11) have been considered in a protective, while the adherence to non-healthy dietary patterns such as a westernised dietary pattern seems to exert detrimental effect(Reference Lai, Hiles and Bisquera12,Reference Li, Lv and Wei13) .
Although dietary patterns reflect the complexity of dietary intake in relation to diseases(Reference Schulze, Hoffmann and Kroke14), their use could be complex in nutritional epidemiology and does not provide an accurate assessment of dietary intake. On the one hand, the adherence to these patterns is based on the intake level of the population, and they also need complex software to compute the nutrient composition of the diet. On the other hand, they are not simple to administer and are difficult to apply across different countries(Reference Fung, Isanaka and Hu15).
The Prime Diet Quality Score (PDQS) is a simple food-based diet quality score developed by Fung et al. (Reference Fung, Isanaka and Hu15). This score was devised as a simple tool to evaluate its association with the risk of CVD. Mainly, the PDQS distinguishes itself by the ability to differentiate healthy foods from unhealthy ones. Despite the use of this score in association with chronic diseases such as diabetes, hypertension and dyslipidaemia(Reference Alvarez-Alvarez, Toledo and Lecea16,Reference Gicevic, Gaskins and Fung17) , to our knowledge, no study has assessed the association between PDQS and depression in older adults.
Thus, this study aims to explore (1) the cross-sectional association between the adherence to the PDQS and the prevalence of depressive symptoms at baseline (cross-sectional analysis) and (2) the prospective association of baseline PDQS with changes in depressive symptomatology after 2 years of follow-up in a Spanish cohort from PREDIMED-Plus trial.
Methods
Design of the study
The PREDIMED-Plus study is an ongoing 6-year multicentre, randomised, parallel-group and primary prevention trial conducted in Spain. The aim of the trial is to assess the effect of an intensive weight loss intervention programme based on an energy-restricted traditional Mediterranean diet, physical activity promotion and behavioural support, on hard cardiovascular events, in comparison with usual care and dietary counselling intervention only with energy unrestricted Mediterranean Diet (control group). More detailed information concerning the study protocol can be found elsewhere(Reference Martínez-González, Buil-Cosiales and Corella18).
Ethics approval
The protocol of the study was written in accordance with the principles of the Declaration of Helsinki. The respective Institutional Review Board (IRB) of all study centres approved the study protocol. The trial was registered at the International Standard Randomized Controlled Trial (ISRCT) in 2014 with number 89898870. All participants provided written informed consent.
Participants and data collection procedures
Eligible participants were community-dwelling adults (men: aged 55–75 years, and women: aged 60–75 years) with overweight or obesity (BMI ≥ 27 and <40 kg/m2) who were free of CVD at study recruitment, who met at least three components of the MetS according to the updated harmonized criteria of the International Diabetes Federation and the American Heart Association and National Heart, Lung, and Blood Institute(Reference Alberti, Eckel and Grundy19).
From the 6874 participants enrolled in the PREDIMED-Plus study, we selected for the present analysis those participants who completed a semiquantitative FFQ and a depressive symptoms questionnaire (Beck Depression Inventory-II) at baseline. Those who failed to complete the Beck Depression Inventory-II questionnaire at baseline (n 21) were excluded from this sub-study. Among the available participants, we also excluded those individuals without information about dietary intake or with values for total energy intake in FFQ beyond predefined limits at baseline (<800 kcal/d or > 4000 kcal/d for men); (<500 kcal/d or > 3500 kcal)/d for women)(Reference Willet20) (n 241). The final sample for the cross-sectional analysis was 6612 participants. For the longitudinal analysis, we excluded those who failed to complete the Beck Depression Inventory-II questionnaire after 2 years of follow-up (n 1089). Finally, 5523 participants were included for the longitudinal analysis (Fig. 1). Furthermore, out of the eligible individuals, we performed a sensitivity analysis excluding those participants who reported a clinical diagnosis of depression at baseline and/or those who had a Beck Depression Inventory-II score ≥ 18 points at baseline (n 1444).
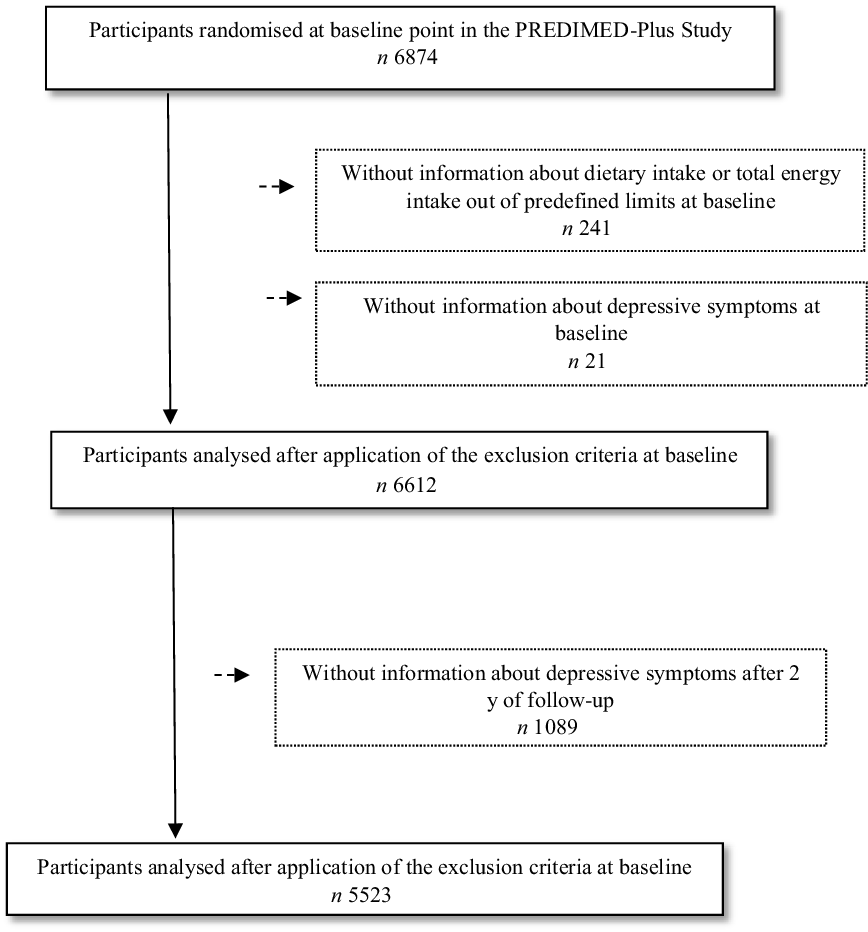
Fig. 1. Flow chart of the study participants.
Dietary assessment
Data on dietary intake were collected at baseline by trained dietitians. This information was appraised by a validated 143-item semi-quantitative FFQ(Reference Martin-Moreno, Boyle and Gorgojo21). The questionnaire provides a list of foods commonly used by the Spanish population and includes nine frequency options for a specified serving size, ranging from never or almost never to ≥ 6 times/d. In face-to-face interview, participants were asked about the frequency of consumption of each food item during the past year, specifying usual portion sizes.
Prime diet quality score construction
Using the baseline 143-item validated FFQ mentioned above, we calculated an energy-adjusted PDQS using the residual method. This PDQS is based on the Prime Screen Questionnaire, a short diet assessment tool developed for clinical use to quickly assess diet quality(Reference Rifas-Shiman, Willett and Lobb22) and widely used by other authors(Reference Fung, Isanaka and Hu15). This score classifies foods as healthy and unhealthy based on two major considerations: (1) data from the literature on the direction of association with the risk of non-communicable diseases and (2) nutrient contribution in the worldwide setting. According to this fact, the food groups included that were considered healthy were vegetables (dark leafy green vegetables, cruciferous vegetables, carrots and other vegetables), fruits (whole citrus fruits and other whole fruits), legumes, nuts, poultry, fish, eggs, whole grains and liquid vegetable oils. Meanwhile the non-healthy food groups included in the score were red meat, potatoes, processed meat, whole milk dairy, refined grains and baked goods, sugar sweetened beverages, fried foods obtained away from home and desserts and ice creams. The points of adherence in each food component were assigned according to the following criteria as follows: 0–1 serving/week (0 point) compared with 2–3 servings/week (1 point) compared with ≥ 4 servings/week (2 points) for the healthy food groups. Scoring was reversed and points deducted for the unhealthy food groups. Points for each food group were then summed to give an overall score. The PDQS has twenty-one food groups and ranges from 0 to 42 total points. Finally, PDQS was categorised in quintiles (Q) and the cutoff points were Q1: <19·7, Q2: ≥ 19·7–<21·9, Q3: ≥ 21·9–<23·8, Q4: ≥ 23·8–<26·0, Q5: ≥ 26·0.
Outcome assessment
Depressive symptoms were collected at baseline and yearly by trained PREDIMED-Plus staff through the Beck Depression Inventory-II (Beck-II) previously validated in Spanish population. The Beck Depression Inventory-II includes twenty-one questions with four possible answers sorted according to symptoms severity and score ranges from 0 to 63 points(Reference Ibáñez, Pino and Olmedo23). Prevalent depression was defined as the presence of depressive symptoms at baseline (Beck ≥ 18 points) or the reporting of a lifetime prevalence of depression. Lifetime prevalence of depression was collected at baseline and defined as a self-reported lifetime medical diagnosis of depression. In the baseline analysis, in order to analyse depressive symptoms across categories of PDQS, we considered the Beck-II score as a continuous outcome. Finally, to assess changes in depressive symptomatology after 2 years of follow-up, we have calculated the difference in Beck punctuation (2-year follow-up Beck-II questionnaire score minus baseline Beck-II questionnaire score).
Covariate assessment
At baseline and once yearly, trained staff collected information about socio-economic and lifestyle factors. The variables included were sex, age, education level (primary level, secondary level and tertiary level which includes University studies), civil status (married or not, which includes widowed, divorced/singled or others) and whether they lived alone or not. Other lifestyle variables such as smoking habits (former smoker, never smoker and current smoker), sleep duration (hours per day) and physical activity status (active, moderately active and less active) were recorded. Regarding sleep, participants reported their average daily sleeping time for both weekdays and weekends, using the non-validated open question ‘How many hours do you sleep on average per day on weekdays and weekends?’ Leisure-time physical activity was assessed using the short form of the Minnesota Leisure Time Physical Activity Questionnaire validated in Spain(Reference Elosua, Marrugat and Molina24,Reference Elosua, Garcia and Aguilar25) (including questions to collect information about types of physical activity, their frequency (number of days) and duration (min/d)). Leisure-time activities were computed by assigning a metabolic equivalent score to each activity, multiplied by the time spent for each activity and summing up all activities. The intensity was assigned based on the compendium of physical activity(Reference Ainsworth, Haskell and Herrmann26). Furthermore, at each visit, anthropometric variables were also measured by trained personnel: weight (using high-quality electronic calibrated scales, in kg) and height (using a wall-mounted stadiometer, in m2). BMI was calculated dividing weight by height squared. BMI measure was expressed (in kg/m2).
Finally, personal history of chronic diseases (hypertension, dyslipidaemia and type 2 diabetes) was collected from the patients ‘medical records’.
Statistical analysis
The association between PDQS (in quintiles) and depressive symptoms at baseline was assessed using multivariable linear regression models. Logistic regression models were fitted to assess the relationship between PDQS and the prevalence of depression at baseline. OR and their 95 % CI were calculated considering the lowest quintile (PDQS = Q1) as the reference category. Linear mixed-effects models were used to explore the associations between concurrent changes in self-reported depressive symptomatology (Beck-II score) and quintiles of PDQS at 2 years of follow-up. Changes in repeated measured variables were calculated as the difference between the results from each follow-up assessment (changes from 0 to 2 years follow-up). To control for potential confounding factors, the results were adjusted for the socio-demographic and lifestyle variables mentioned previously. We analysed the possible interaction between sex-age and depression. As well as, the possible modifier effect of allocation group in the outcome measured. In order to evaluate the effect that the recruitment centre exerts on the dietary intervention and assuming in any case that the results could be heterogeneous, we adjusted the models also for this variable. Finally, allocation group (intensive intervention group or usual care (control) group) was also taken into account in the longitudinal adjusted models.
We have used a significance level of 0·05 for all analyses. Data were analysed using Stata (15.0, StataCorp LP).
Results
Characteristics of the study subjects at baseline according to Prime Diet Quality Score quintiles
The current study included a total of 6612 participants (3414 men). The baseline characteristics of participants according to PDQS quintiles are presented in Table 1. Among those who showed better dietary quality intake (higher PDQS quintile, Q5), there were more women, older and tended to have lower BMI. Participants in the lowest quintile of PDQS (Q = 1) were mainly smokers, and participants with a higher educational level, less physically active and they did not live alone. No difference in disease prevalence and depressive status was found among PDQS quintiles.
Table 1. Baseline characteristics of PREDIMED-plus participants according to quintiles of prime diet quality score (PDQS)
(Number and percentages; mean values and standard deviations)

Q, quintile. Values are presented as means ± sd for continuous variables and n (%) for categorical variables. Pearson’s χ 2 test was performed for categorical variables and ANOVA test for continuous variables.
Cross-sectional association between Prime Diet Quality Score and depressive symptomatology
We fitted a multivariable linear regression model to analyse depressive symptoms across categories of PDQS (Table 2) taking into account the Beck-II score as a continuous outcome. Depressive symptomatology was inversely associated with PDQS in both models (models 1 and 2). That is, the punctuation of depressive symptoms decreases across PDQS quintiles, in model 2 (multivariable β-coefficients (95 % CI) = –0·73 (−1·29, −0·18) and −0·62 (–1·17, −0·06)) and for Q3 and Q4, respectively. Nevertheless, for the higher score of PDQS (Q5), we only found significant differences in model 1 (adjusted only for sex and age).
Table 2. Multivariable linear regression models for the association between Prime Diet Quality Score (PDQS) and symptomatology of depression in the PREDIMED-plus study participants
(regression coefficients and 95 % confidence intervals)

PDQDS, Prime diet quality score; Q, quintile.
Values are presented as adjusted means ± se, together with β-coefficients and 95 % CI for symptomatology of depression (Beck Depression Inventory II) as continuous variable according to PDQS. Model 1: Adjusted for sex and age. Model 2: Additionally adjusted for smoking habits, physical activity, educational level, BMI, living alone, civil status, sleep duration and presence of chronic diseases. The interaction between sex age and depression symptomatology was not significant (P = 0·414).
* Values showed a statistically significant association (P < 0·05).
Cross-sectional associations between Prime Diet Quality Score and prevalence of depression
Our results showed that PDQS was inversely associated with depressive status (≥ 18p at Beck II inventory at baseline and/or lifetime prevalence of depression) in logistic analysis (Table 3). Participants in the highest quintile of PDQS (Q5) showed a significant decrease in the odds of depression´s prevalence as compared with those participants in the lowest quintile (Q1) (OR (95 % CI) = 0·82 (0·68, 0·98) for model 2 (OR (95 % CI) = 0·77 (0·64, 0·92) for model 1). This significant association was consistent across PDQS quintiles (P for trend = 0·015).
Table 3. Multivariable logistic regression models for the association between Prime Diet Quality Score (PDQS) and prevalence of depression in the PREDIMED-plus study participants
(Odds ratios and 95 % confidence intervals)

PDQS, prime dietary quality score; Q, quintile.
Values are presented as adjusted means ± se, together with OR and 95 % CI for prevalence of depression (≥ 18p at Beck Depression Inventory II and/or lifetime prevalence of depression) as categorical variable according to PDQS. Model 1: Adjusted for sex and age. Model 2: Additionally adjusted for smoking habits, physical activity, educational level, BMI, living alone, civil status, sleep duration and presence of chronic diseases. The interaction between sex-age and depression symptomatology was not significant (P = 0·430).
* Values showed a statistically significant association (P < 0·05).
Longitudinal associations between Prime Diet Quality Score and changes in depressive symptomatology after 2 years of follow-up
Table 4 shows main association between concurrent changes in self-reported depressive symptoms and PDQS after controlling for potential confounders. In multivariable-adjusted model 1, comparison of the highest v. the lowest quintile of PDQS revealed decreases in depressive symptomatology during the follow-up, Q5 v. Q1 in model 1 (β − 0·67 z-score, 95 % CI 1·17, −0·18). Model 2, additionally adjusted as shown in Table 4, did not found any significant difference.
Table 4. Association of concurrent changes in self-reported depressive symptoms and Prime Diet Quality Score (PDQS) after 2 year of follow-up in the PREDIMED-plus trial
(Coefficients values and 95 % confidence intervals, n 5523)

PDQS, prime dietary quality score; Q, quintile.
The values show the β coefficients (95 % CI) for changes in depressive symptomatology after 2 years of follow-up as continuous variable according to PDQS. Mixed-effects linear models were performed. Model 1: Adjusted for sex and age. Model 2: Additionally adjusted for depressive symptomatology at baseline, smoking habits, physical activity, educational level, BMI, living alone, civil status, sleep duration, presence of chronic diseases, allocation group and recruitment centre. The interaction between sex-age and depression symptomatology was not significant (P = 1·00).
* Values showed a statistically significant association (P < 0·05).
In ancillary analysis (sensitivity analysis), we excluded those subjects with a Beck-II score higher than eighteen points at baseline or with lifetime prevalence of depression. In this sub-sample, the results were no longer significant although the magnitude of effect was quite similar to that observed in the overall sample. A higher baseline PDQS (Q5 v. Q1) was associated with a decrease in depressive symptomatology (β-coefficient (95 %) CI = −0·58 (–1·06, −0·11)) in model 1. Nevertheless, when we adjusted for potentially confounding factors, we did not find any significant association between PDQS and changes in depressive symptomatology after 2 y-of follow-up (online Supplementary Table 1).
Understanding the possible impact of sex and age in the changes of depressive symptomatology (P for interaction < 0·05), we performed a supplementary analysis stratified by sex and age (55–65 men/60–75 women, 65–75 both sex groups). A supplementary analysis stratifying by these covariates is shown in Supplementary Tables 2, 3 and 4. In the cross-sectional analysis, we found a significant association between PDQS and depressive symptomatology (being the effect higher in older women (> 65 years old) for depressive symptomatology (online Supplementary Table 2) but higher in older men for the prevalence of depression (online Supplementary Table 3)). In the prospective analysis, the findings show a protective effect in younger population (women and men) but without a dose–response pattern (online Supplementary Table 4).
In order to assess a possible interaction between allocation group and PDQS in depression symptomatology, interaction terms were included in the longitudinal analyses. We did not find any significant interaction between PDQS and primary trial intervention in association with Beck-II score at the baseline during the follow-up (data not shown, P for interaction > 0·05).
Discussion
In this substudy of the PREDIMED-Plus trial analysed as an observational longitudinal cohort, we aimed to examine the association between PDQS and depression symptomatology in a community-dwelling adult population with overweight/obesity and MetS. Our findings suggest that participants with intermediate-high diet quality (Q3–Q4 v. Q1 of PDQS) had lower depressive symptoms and a lower odds of depression’s prevalence than those with poorer diet quality at baseline. These associations were robust to adjustments for a range of health parameters and behavioural factors in cross-sectional analysis. However, we have to remark that when the association between PDQS and changes in depressive symptomatology after 2 years of follow-up was assessed, the relationship was no longer significant.
The development of depressive illness is influenced by several factors, including genetic, hormonal and immunological factors. Diet can modulate each of these factors, and as a result, has impact on the development and course of this condition(Reference Gómez-Pinilla27). Traditionally, research on the association between diet and depression has focused primarily on nutrients such as fatty acids(Reference Freeman, Hibbeln and Wisner28) and the group B vitamins involved in the synthesis of some neurotransmitters(Reference Bourre29). Nevertheless, the protective effect of diet on chronic diseases as depression comes from the cumulative and synergic effect of nutrients from different sources of foods, rather than from specific nutrients(Reference Hu30). Currently, nutritional epidemiology has focused on associations between the effects of dietary patterns on health instead of isolated nutrients. Our results suggest a protective effect of a baseline adherence to an overall ‘healthy dietary pattern’ rich in fruits, vegetables and legumes and low in processed meat and sweetened foods. In line with our findings, prospective large studies based on dietary patterns characterised by similar foods to those included in the PDQS as the Mediterranean diet, or other healthy diets have reported an inverse relationship with depression outcomes in adults(Reference Nicolaou, Colpo and Vermeulen31–Reference Martínez-González and Sánchez-Villegas33). There are several plausible mechanisms underlying the association observed. One of them is that brain inflammation increases the risk of depression, thus foods as fruits and vegetables have been shown to have strong antioxidant/anti-inflammatory capacity, decreasing depression risk(Reference Miller, Maletic and Raison34).
However, we cannot elucidate if the relationship between diet and depression could be bidirectional, causing a possible reverse causality bias. That is, personal dietary choices are related with depressive symptomatology, as diminished appetite(Reference Burrows, Stewart and Antonacci35) and/or an increased desire for unhealthy foods(Reference Konttinen, Männistö and Sarlio-Lähteenkorva36,Reference Gibson-Smith, Bot and Brouwer37) , at the same time that unhealthy dietary habits increased the risk of developing depressive symptoms(Reference Lang, Beglinger and Schweinfurth38,Reference Dipnall, Pasco and Meyer39) . In an attempt to avoid this possible bias in the relationship between PDQS and depressive symptomatology, we evaluated the association between PDQS and depressive symptomatology after 2 years of follow-up. In this case, the results suggest a direct association between PDQS and changes in depressive symptoms although the findings were not significant. Contrary to our findings, a meta-analysis of twenty-four prospective studies reported that a high-quality diet, regardless of dietary pattern, was associated with a lower risk of depressive symptoms during the follow-up(Reference Molendijk, Molero and Ortuño Sánchez-Pedreño32). A possible explanation to the lack of statistical significance of our results could be exerted by the short follow-up period. This meta-analysis included studies with more than 2 years of follow-up. A longer follow-up with more adequate induction period could be useful to definitively assess the role of dietary quality in depression. The meta-analysis performed by Molendijk et al. reported that those studies that control for this variable observed significant association(Reference Molendijk, Molero and Ortuño Sánchez-Pedreño32). Nevertheless, we found no association between dietary score and changes in depressive symptoms either with or without adjustment for baseline Beck II score in sensitivity analysis. In Japan, Nanri et al. examined the association between the dietary score based on the Japanese Food Guide Spinning Top and risk of depressive symptoms. Similarly to our results, these authors found a statistically significant association only in the cross-sectional design but not in the prospective analysis(Reference Nanri, Nagai and Kochi40). As in the previous study, the null finding in our prospective analysis, including sensitivity, raises the possibility of reverse causality mentioned above. Further prospective investigation is required to analyse the association between PDQS and depressive symptoms.
Strengths and limitations
The current study has some limitations that need to be addressed. First, the community-dwelling population with overweight/obesity and MetS included in the study is not representative of the general population; however, our population represent an important proportion of current Western societies. Second, although the FFQ has been validated in nutritional studies(Reference Martin-Moreno, Boyle and Gorgojo21), self-reporting questionnaires, in combination with memory loss of older participants, might lead a no differential misclassification bias. However, this bias would tend the estimations towards the null; so, the association could be higher than that observed. Moreover, we excluded participants with energy intakes outside of predefined limits(Reference Willet20), and we used residual method in order to adjust for energy intake. Third, loss of participants after 2 years of follow-up could be a selection bias (only the more healthy participants were available for the longitudinal study) attenuating our associations. Another limitation to highlight in this substudy of the PREDIMED-Plus trial was the not adjustment by anti-inflammatory/immunomodulatory medications and drugs with potential psychotropic effects and personal/family history of depression that can modulate the results obtained. Despite this, we have controlled our models by the main socio-demographic and lifestyles variables related to depressive status. Finally, the follow-up time (2 years) is probably quite short to evaluate changes in the outcome.
Notwithstanding the above limitations, our study includes several strengths that reinforce the results obtained. We used a repeated measurements of outcome (depressive symptomatology assessed by Beck II depression questionnaire inventory) over 2 years. Another strength is not only the use of a DDS that provides a more intuitive view of the whole dietary pattern, but also the study of each of the food groups we have identified some of them as important components linked with depression status. Finally, the large sample size and the considerable amount of baseline information collected in a large ongoing primary prevention trial, using a standardised protocol that reduces information bias regarding reported food intakes, socio-demographic characteristics and lifestyles are other strengths that should be considered.
What our results suggest is that recommending diets with high diversity of vegetables, grains and protein food groups (fish/seafood, white meat, nuts and legumes) may represent an effective approach to improve depression outcomes in community-dwelling population. That is, in people with depressive symptoms, fostering healthy dietary patterns would presumably result in a far greater impact over prevalence and symptomatology on depression. Nevertheless, these associations were only found in cross-sectional analysis. It is necessary the entire cohort was followed-up for a longer period in order to establish significant associations between PDQS and depression status.
Conclusions
In summary, we observed that higher PDQS was related to a lower prevalence of depression and a lower depression symptomatology in Spanish community dwelling with overweight/obesity and MetS at baseline. Nevertheless, the null finding in our prospective analysis raises the possibility of reverse causality. Further prospective investigation is required to analyse the association between PDQS and depressive symptoms.
Acknowledgements
The authors especially thank the PREDIMED-Plus participants for their collaboration and the PREDIMED-Plus staff for their support and effort. CIBEROBN is an initiative of ISCIII, Spain.
The PREDIMED-Plus trial was supported by the European Research Council (Advanced Research Grant 2013-2018; 340918) grant to Miguel Ángel Martínez-González and by the official funding agency for biomedical research of the Spanish Government, ISCIII through the Fondo de Investigación para la Salud (FIS), which is co-funded by the European Regional Development Fund (four coordinated FIS projects led by Jordi Salas-Salvadó and Josep Vidal), including the following projects: PI13/00673, PI13/00492, PI13/00272, PI13/01123, PI13/00462, PI13/00233, PI13/02184, PI13/00728, PI13/01090, PI13/01056, PI14/01722, PI14/00636, PI14/00618, PI14/00696, PI14/01206, PI14/01919, PI14/00853, PI14/01374, PI16/00473, PI16/00662, PI16/01873, PI16/01094, PI16/00501, PI16/00533, PI16/00381, PI16/00366, PI16/01522, PI16/01120, PI17/00764, PI17/01183, PI17/00855, PI17/01347, PI17/00525, PI17/01827, PI17/00532, PI17/00215, PI17/01441, PI17/00508, PI17/01732 and PI17/00926. The Especial Action Project entitled: ‘Implementación y Evaluación de una intervención intensiva sobre la actividad física Cohorte PREDIMED-Plus’ grant to Jordi Salas-Salvadó, the Recercaixa grant to Jordi Salas-Salvadó (2013ACUP00194), grants from the Consejería de Salud de la Junta de Andalucía (PI0458/2013; PS0358/2016; PI0137/2018), the PROMETEO/2017/017 grant from the Generalitat Valenciana, the SEMERGEN grant and CIBEROBN and FEDER funds (CB06/03), ISCIII. International Nut&Dried Fruit Council- FESNAD Nº201302: Miguel Ángel Martínez-González (PI). None of the funding sources took part in the design, collection, analysis or interpretation of the data or in the decision to submit the manuscript for publication. Fernando Fernández-Aranda and the study was partially funded by the following grants: SLT006/17/00246, by the Department of Health, Generalitt de Cataluña by the calls “Acció instrumental de programes de recerca orientatsenlàmbit de la recercaila innovació en salut” and “Pla estrategic de recerca i innovació en salut (PERIS)”. We thank CERCA Programme/Generalitt de Catalunya for institutional support. This research was also partially funded by EU-H2020 Grants (Eat2beNICE/h2020-sfs-2016-2; ref.728,018; and PRIME/h2020-SC1-BHC-2018-2020; ref: 847,879) The corresponding author had full access to all the data in the study and had final responsibility to submit for publication.
N. C. -I., L. S-M., M. A. M.-G., J. S-S., D. C., J. A. M., J. W., J. V., D. R., J. L-M., R. E., A. B-C, J. A. T., V. M., X. P., M. D-R., P. M., J. V., L. D., E. R. and A. S-V. collected all the data from the PREDIMED-Plus trial. N. C-I., A. S-V., S. M-P., and A. B-C. designed the study; performed the analysis and wrote the first draft of the manuscript. All authors contributed to the editing of the manuscript. All authors have read and approved the final version of the manuscript.
There are no conflicts of interest.
Supplementary material
For supplementary material/s referred to in this article, please visit https://doi.org/10.1017/S0007114521004323