The value of collaboration and data-sharing is well recognised within the medical community and is one of the hallmarks of what has been called ‘the fourth age of research’, in which the pace of discovery has accelerated and international platforms for studying multifactorial problems have been built.Reference Adams1 The aggregation of data from individual research groups not only maximises the utility of individual data-sets and minimises demands on participants, but enables the joint analyses of complex data that can lead to incremental advances in elucidating disease aetiology.Reference Chawner, Mihaljevic, Morrison, Eser, Maillard and Nowakowska2 Within major psychiatric and neurodevelopmental conditions, few truly novel pharmacological treatments have been developed for several decades, with the noteworthy exceptions of ketamine for depressionReference Iadarola, Niciu, Richards, Vande Voort, Ballard and Lundin3 and atomoxetine for attention-deficit hyperactivity disorder (ADHD).Reference Childress4 Worryingly, many major pharmaceutical companies are decreasing their research efforts and investment in this area.Reference MacEwan, Seabury, Aigbogun, Kamat, van Eijndhoven and Francois5 This apparent stagnation in progress is the result of a lack of understanding of the pathogenesis of these conditions,Reference Owen Michael6 hindering the identification of novel targets for drug discovery and limiting the utility of current diagnostic categories in defining mechanistically discrete disorders. A route to address these limitations involves integrating biological data at scale and across, rather than within, diagnostic classifications.Reference Willsey, Morris, Wang, Willsey, Sun and Teerikorpi7 Research conducted in this manner can explore the aetiological and biological commonalities between diagnoses revealed by genetic studies,Reference Smoller, Andreassen, Edenberg, Glatt and Kendler8 accelerating discoveries on complex disorders and informing novel pharmacological and non-pharmacological therapeutic strategies, firmly grounded in biology.Reference Denny, Van Driest, Wei and Roden9
Recent large-scale studies have built on the hypothesis that psychiatric phenotypes do not always reflect distinct underlying pathogenic processes, and that some genetic risk factors are shared between neuropsychiatric disorders.Reference Baselmans, Yengo, van Rheenen and Wray10 This echoes the widely acknowledged clinical observation that many symptoms are features of multiple disorders and that patients often challenge current diagnostic classifications by presenting with characteristics of more than one disorder.Reference Plana-Ripoll, Pedersen, Holtz, Benros, Dalsgaard and de Jonge11 What is currently not known, however, is to what extent this distribution of cross-disorder symptoms is related to the shared genetic liability between neurodevelopmental conditions.Reference Baselmans, Yengo, van Rheenen and Wray10 Commonalities in genetic risk factors might help identify a shared underlying biology, but this line of inquiry cannot be pursued without well-characterised cross-disorder samples, scarce even within large international consortia. In fact, it has been explicitly suggested that the majority of samples used in published genetic discovery studies have not been collected with the required amount of phenotypic data necessary to advance diagnostics, stratification and treatment.Reference Merikangas and Merikangas12 Thus, many research groups have directed their efforts to access resources with large amounts of routinely collected data, such as population biobanks and electronic health record systems, from which rich phenotypic data can be derived.Reference Merikangas and Merikangas12,Reference Sanchez-Roige and Palmer13 However, some common limitations of these include selection biases and a low representation of clinically severe disorders.Reference Sanchez-Roige and Palmer13,Reference Underwood, DelPozo-Banos, Frizzati, John and Hall14 The latter can be exemplified by a recent study of schizophrenia genetic liability on 106 160 patients across four healthcare systems in the USA, where only 522 individuals with a formal diagnosis of schizophrenia were included;Reference Zheutlin, Dennis, Karlsson Linnér, Moscati, Restrepo and Straub15 a small figure, but in line with a lifetime morbid risk of 0.7% for this disorder.Reference McGrath, Saha, Chant and Welham16 Such is a classic quandary in psychiatric genomics,Reference Crowley and Sakamoto17 in which the setup of research studies leads to either a large case sample with minimal phenotyping or an extensively phenotyped one with fewer individuals.
Aims and objectives
The Digital Repository for Amalgamating Genomic and Neuropsychiatric Data (DRAGON-Data) was therefore established at Cardiff University as a means of developing a platform where cross-disorder analyses of large well-phenotyped samples are possible. This approach integrates multiple existing case data-sets with genetic, clinical, environmental and developmental data. The focus on mental health across disorder boundaries and at scale aims to improve understanding of the pathophysiology of adult- and child-onset neurodevelopmental and psychiatric disorders, providing opportunities to combine diagnosis-led and symptom-led research. DRAGON-Data shares a focus with ongoing efforts to collate phenotype data within the Psychiatric Genomics Consortium (PGC),Reference Sullivan, Agrawal, Bulik, Andreassen, Børglum and Breen18 as well as previous mental health-related initiatives such as the Genetics of Endophenotypes of Neurofunction to Understand Schizophrenia (GENUS) Consortium,Reference Blokland, del Re, Mesholam-Gately, Jovicich, Trampush and Keshavan19 the International Consortium for Schizotypy Research (ICSR),Reference Docherty, Fonseca-Pedrero, Debbané, Chan, Linscott and Jonas20 the International 22q11.2 Deletion Syndrome Brain Behaviour Consortium (22q11.2DS IBBC),Reference Gur, Bassett, McDonald-McGinn, Bearden, Chow and Emanuel21 the Psychosis Endophenotypes International ConsortiumReference Bramon, Pirinen, Strange and Lin22 and the Genes to Mental Health (G2MH) Network.Reference Jacquemont, Huguet, Klein, Chawner, Donald and van den Bree23 However, most of these projects have typically focused on a single psychiatric disorder or group of closely related conditions, whereas DRAGON-Data seeks to integrate genomic and phenotype data from a range of disorders across the developmental continua.
The current paper describes the formation of DRAGON-Data through the curation and harmonisation of phenotypic and genetic information across existing cohorts. These represent a broad diversity of psychiatric diagnoses, including ADHD, bipolar disorder, mood disorders, major depressive disorder, neurodevelopmental conditions, post-traumatic stress disorder and schizophrenia. This process has been informed by a series of legal and ethical considerations on the evolving landscape of individual-level data-sharing, which is required to ensure the sustainability of this repository as a resource for current and future researchers. Therefore, the governance framework of DRAGON-Data is also described, which enables the access and reuse of its data in ways that align with confidentiality regulations and the ethics of participating studies.
Method
Studies included
Fifteen studies from the MRC Centre for Neuropsychiatric Genetics and Genomics at Cardiff University (https://www.cardiff.ac.uk/mrc-centre-neuropsychiatric-genetics-genomics) were included in this project. A summary of the studies can be found in Table 1. Each study had its own approved research ethics, and ethical approval for the curation and development of DRAGON-Data was obtained from Cardiff University's School of Medicine Research Ethics Committee (approval reference 19/72). The studies included participants who were adults with psychiatric disorders, children (defined as up to age 18 years) with neurodevelopmental disorders, children of parents with psychiatric disorders and both children and adult carriers of rare neurodevelopmental risk copy number variants (ND-CNVs).
Table 1 Studies included in DRAGON-Data

BDRN, Bipolar Disorder Research Network; SCAN, Schedules for Clinical Assessment in Neuropsychiatry; COGS, Cardiff Cognition in Schizophrenia; DEFINE, Defining Endophenotypes From Integrated Neurosciences; ND-CNV, Neurodevelopmental Copy Number Variant; SCID, Structured Clinical Interview for DSM-IV; PAS-ADD, The Psychiatric Assessment Schedule for Adult with Developmental Disability; ECHO, Experiences of Children with copy number variants; IMAGINE, Intellectual Disability and Mental Health: Assessing Genomic Impact on Neurodevelopment; CAPA, Child and Adolescent Psychiatric Assessment; EPAD, Early Prediction of Adolescent Depression; DeCC/DeNt, Depression Case Control / Depression Network; NCMH, National Centre for Mental Health; CAPS-5, Clinician Administered PTSD Scale for DSM-5; PTSD, post-traumatic stress disorder; SAGE, Study of ADHD, Genes and Environment; ADHD, attention-deficit hyperactivity disorder.
a. Includes family data and/or (trios).
Ethics approval
The development of DRAGON-Data was reviewed by the Cardiff University School of Medicine Ethics Committee as part of the ‘Clinical, phenotypic and genomic research in psychiatry’ application (reference SMREC 19/72), approved on 05/09/19. Ethical clearances to conduct each of the DRAGON-Data studies are detailed in their parent publications.
Phenotypic data harmonisation strategy
The process of curating the phenotypic data is outlined in Figure 1, and a description of challenges we faced in our exercise is provided in Supplementary Appendix 1 available at https://doi.org/10.1192/bjo.2022.636. Initially, investigators from all studies completed a pro forma detailing the data and types of measures available, including the study clinical interviews, rating scales and self-report questionnaires. We compared all of the variables to identify overlaps and resolve situations where the same information might have been differently labelled across studies. We also defined a core set of variables (Table 2), focused on information relevant and applicable to cross-disorder research. A primary consideration for including a variable among this core set was whether it was collected as part of the National Centre for Mental Health (NCMH) research programme. The NCMH is a Welsh Government-funded research centre that investigates neurodevelopmental, psychiatric and neurodegenerative disorders across the lifespan. Its cohort is the largest sample with phenotype data available to us, and a cross-disorder resource in itself.Reference Underwood, Kendall, Berrett, Lewis, Anney and van den Bree36 As the NCMH is still being expanded by recruitment of participants, maximising its compatibility with DRAGON-Data was desired. Additionally, every core variable was required to be available in at least half of the current data-sets, taking into consideration that some data might be specific to child or adult cohorts. Variables that were not available in the NCMH and were present in fewer than half of the studies were only included if they could be derived from existing data to achieve the representation threshold. On receipt of each data-set, the variables were cleaned and matched with our defined core set of variables, and these were then signposted within our DRAGON-Data dictionary.
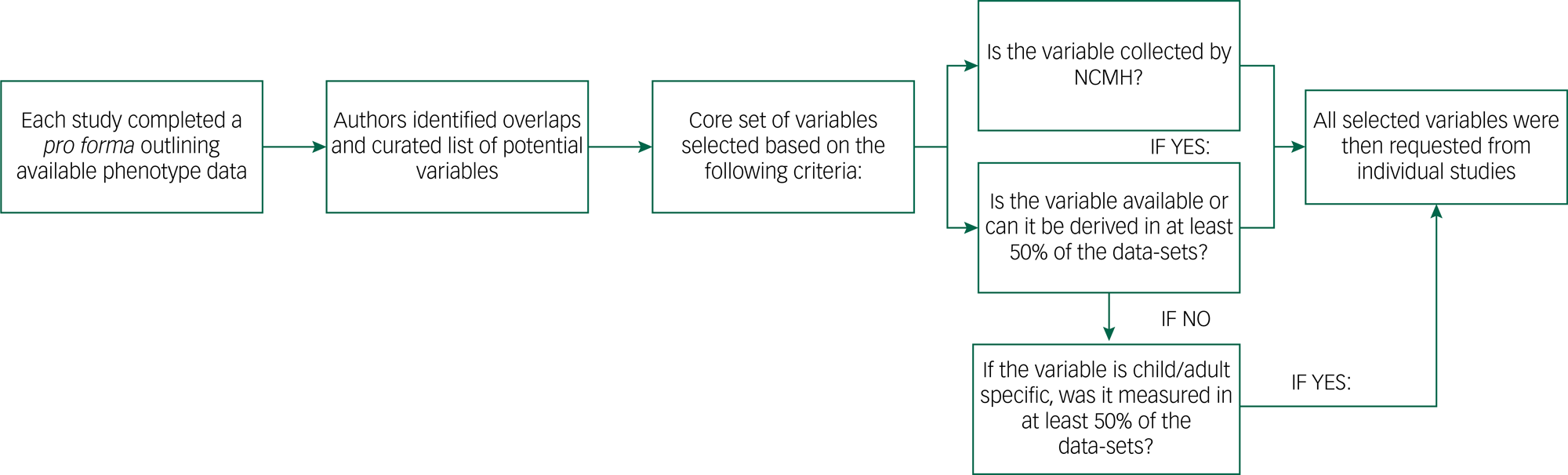
Fig. 1 DRAGON-Data pipeline for phenotypic data curation. NCMH, National Centre for Mental Health.
Table 2 List of phenotypic variables included in DRAGON-Data
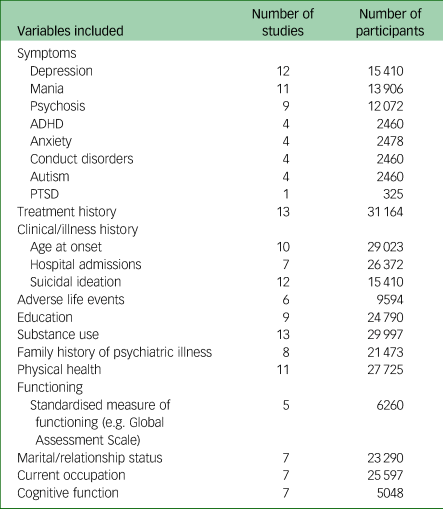
Number of participants refers to the number of data points available for each set of variables listed. PTSD, post-traumatic stress disorder; ADHD, attention-deficit hyperactivity disorder.
Genetic data harmonisation strategy
We developed an in-house genotype quality control pipeline to facilitate standardised procedures for all aspects of genetic analysis (Fig. 2), available at https://github.com/CardiffMRCPathfinder/GenotypeQCtoHRC. The pipeline begins with conversion of genotype data into binary PLINK format.Reference Chang, Chow, Tellier, Vattikuti, Purcell and Lee40,Reference Purcell, Neale, Todd-Brown, Thomas, Ferreira and Bender41 Genotyping platform, when not properly recorded in study logs, was inferred by comparing chromosome and base-pair positions of the genotypes on each data-set and 166 array manifests.Reference Rayner42 Across the data-sets in DRAGON-Data, Illumina chips are by far the most common (Table 1). Despite the standardisation inherent to genotype data-sets that is driven by platform commonalities and the PLINK format conversion, creating a harmonised multi-study data-set requires stringent study-wide and data-set-wide quality control. We minutely describe these quality control steps and the challenges they are meant to address in Supplementary Appendix 1.
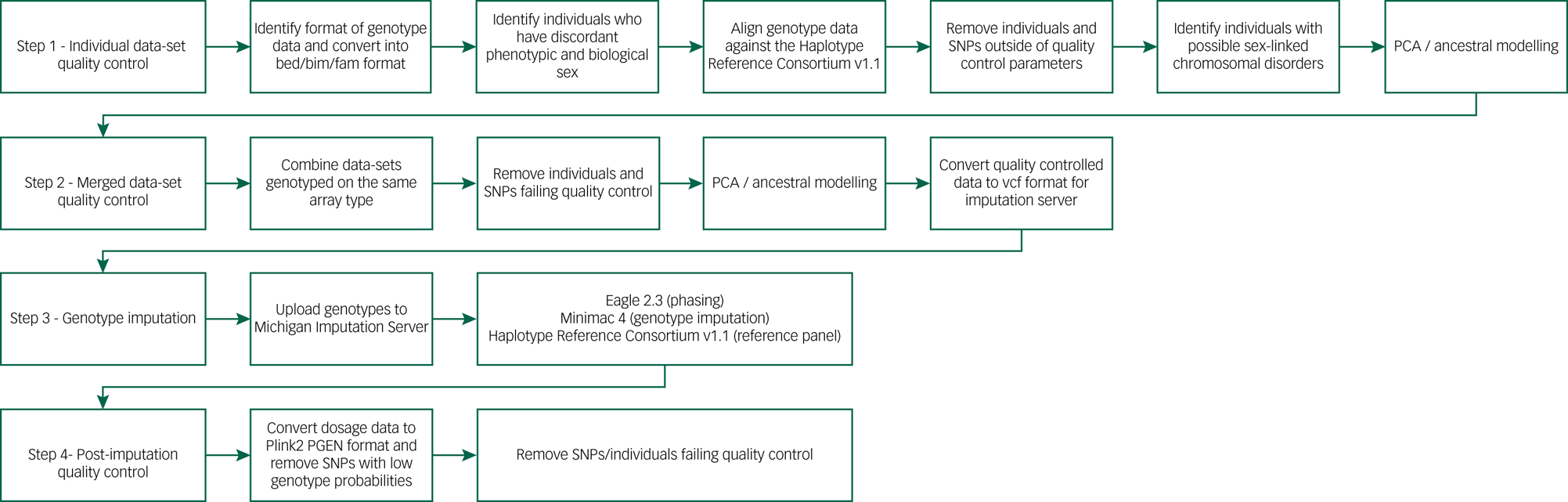
Fig. 2 DRAGON-Data pipeline for SNP genotype quality control and imputation. PCA, principal component analysis; SNP, single nucleotide polymorphism.
Results
The DRAGON-Data harmonised data-set
Table 2 displays an overview of the variables held by each study included in the final DRAGON-Data data freeze. A full list of the variables included in DRAGON-Data can be found in Supplementary Table 2, although the exact variables included varied between studies. All of the studies except for CLOZUK included a semi-structured clinical diagnostic interview, most commonly the Schedules for Clinical Assessment in Neuropsychiatry (SCANReference Wing, Babor, Brugha, Burke, Cooper and Giel43) for adults and the Child and Adolescent Psychiatric Assessment (CAPAReference Angold and Costello44) for children and adolescents. Twelve of the fifteen studies collected data on individual symptoms. The NCMH study includes a brief assessment that does not include questions about individual symptoms, although a small subgroup of this sample (n = 485/16 311) completed more detailed interviews that included symptoms. The most common types of symptoms covered across all studies were depressive, manic and psychotic symptoms. Aside from symptoms, other variables with good coverage across studies were lifetime history of treatment (13/15), substance use (13/15) and history of suicidal ideation and attempts (12/15). The demographic characteristics of the studies are shown in Supplementary Table 1. The harmonised phenotype data is stored in a pseudonymised format within a secure database. There is an accompanying data dictionary cataloguing all available variables with names, descriptions and ratings, and cross-referencing comparable measures across the studies.
Key recommendations for genotype-phenotype data harmonisation
Based on our experience developing DRAGON-Data, we suggest some recommendations for the harmonisation and analysis of clinical and genetic data:
(a) Consider the broad research questions that can be addressed with the creation of a clinical database. Consult with principal investigators and field researchers to identify the variables that will be needed to address these aims.
(b) Identify measures (e.g. questionnaires and interviews) that are in common across the data-sets included. These measures may be easier to harmonise for analysis, although factors outlined in Supplementary Appendix 1 (study protocol differences, use of diagnostic criteria) should be considered to ensure comparability.
(c) Record accurate information about each study variable, including measure used, version number, rating definitions, rating timeframe and source of information. This aids in the identification of comparable variables.
(d) Where new (secondary) variables have been derived by researchers and are designed to be comparable, information should be recorded about the (primary) variables used from each study to derive those secondary variables.
(e) A comprehensive data dictionary should accompany the database that incorporates the information outlined above. At a minimum, each variable should have recorded the name, description, values and corresponding labels (for categorical variables), as well as definition and coding of missing values. Within the data dictionary, variables should be highlighted if they are in common across the data-sets, as these may be suitable to analyse together. It is noteworthy that this curation and creation of dictionaries may often need to occur after the data collection, so researchers and funders should allow sufficient staff resources for the accurate completion of this task.
(f) Include basic demographic information to evaluate the representativeness of the sample, including age range, biological sex, gender identity, ethnicity and education.
(g) Data-sets do not need to be combined into a single data file. A database that houses the data-sets and allows an easy combination of selected studies and variables avoids the need for a single, large-scale data-set, and minimises the computational requirements for the querying and extraction of data.
(h) Data should only be shared and combined if there are suitable ethical and data-sharing agreements that participants have consented to. There may be separate ethical considerations for data-sharing within research settings and for linkage to other external data-sets, particularly public electronic health record databases.
(i) Imputation should only be performed on samples that have been genotyped on the same array backbone, or where there is substantial single nucleotide polymorphism (SNP) overlap after quality control. Furthermore, when performing quality control after imputation, removal of palindromic SNPs with high minor allele frequency (>0.4) is essential to minimising batch effects for samples genotyped on different arrays.
(j) When analysing copy number variants data across arrays, potential differences in probe density and coverage mean that it is vital that plots such as those for b-allele frequency drift, number of copy number variants called per individual and logR ratio standard deviation, are visually inspected to ensure the quality of the resulting calls.
Discussion
Using DRAGON-Data
All of the DRAGON-Data data have been securely stored in HAWK, a high-performance computing cluster supported by the Supercomputing Wales infrastructure,45 which comprises a network of 13 000 computer nodes distributed across four universities (Cardiff, Swansea, Bangor and Aberystwyth). This system allows the backed-up storage of genetic and phenotypic files, and their secure access by authorised users. Analysts in charge of curating genetic or phenotypic data are by default part of a ‘core project team’ with unrestricted access to the entire DRAGON-Data, whereas data-contributing researchers are granted access to their own raw and curated data for any purpose. Undertaking cross-disorder analyses is facilitated through a framework by which any curator or data-contributing researcher can send a structured analytic proposal to the board of investigators, who then decide whether to grant access to the relevant data on scientific grounds. This is modelled after successful international consortia such as the PGC,Reference Sullivan, Agrawal, Bulik, Andreassen, Børglum and Breen18 which in recent years has implemented responsible data-sharing practices among hundreds of investigators.
There are two main approaches to analysing the data within DRAGON-Data: combining individual-level information from across the studies (‘mega-analysis’) or through meta-analysis. Although the latter is relatively straightforward, jointly analysing all samples allows for a better assessment of heterogeneity in the data and can increase statistical power.Reference Boedhoe, Heymans, Schmaal, Abe, Alonso and Ameis46 However, combining samples is particularly problematic for the phenotypic data, as it requires recoding or modifying the variables to be comparable across studies, which could include deriving latent variables through factor analysis. Data combined in this way can be difficult to interpret because of the differences between studies outlined in the previous sections, and it is important to address this variability in both analytic techniques and interpretation of the results. Important considerations are whether the individual study variables are measuring the same construct and whether any variables derived from these are measuring the same construct as the original data. Note that none of these limitations applies to the genetic data, as (carefully) combining samples with large numbers of overlapping SNPs is a common procedure that is known to maximise both the number of successfully imputed variants and their quality.Reference Stanaway, Hall, Rosenthal, Palmer, Naranbhai and Knevel47 Thus, the suitability of a mega-analysis or meta-analysis approach for studies using DRAGON-Data will be decided based on the availability, characteristics and biases of the phenotypic data.
Outside of the data quality control pipelines, genetic analyses in DRAGON-Data can be undertaken using other consolidated tools, such as PLINKReference Chang, Chow, Tellier, Vattikuti, Purcell and Lee40 or GCTA.Reference Yang, Lee, Goddard and Visscher48 Responding to the rapid development of statistical methods to analyse complex phenotypes and ‘big data’, an effort has been made to integrate DRAGON-Data with the highly customisable R framework, via the use of data importers such as GWASTools Reference Gogarten, Bhangale, Conomos, Laurie, McHugh and Painter49 and bigsnpr. Reference Privé, Aschard, Ziyatdinov and Blum50 This allows for use of the approximately 1700 tools currently offered by the Bioconductor suiteReference Huber, Carey, Gentleman, Anders, Carlson and Benilton51 in a large-scale genome-wide setting, and facilitates applying complex analytic techniques such as mixed-model regressionReference Chen, Wang, Conomos, Stilp, Li and Sofer52 and survival analysis.Reference Rizvi, Karaesmen, Morgan, Preus, Wang and Sovic53 Large-scale genomic storage solutions have not currently been implemented in DRAGON-Data, as the weak compression implemented in PLINK files and related formats allows for efficient querying of genotype data even in its imputed form.Reference Chang, Chow, Tellier, Vattikuti, Purcell and Lee40,Reference Layer, Kindlon, Karczewski, Exome Aggregation Consortium and Quinlan54 However, these are active topics of research, and initiatives such as adopting the MPEG-G ISO standard will likely allow future data harmonisation projects to seamlessly incorporate whole-genome sequences.Reference Hernaez, Pavlichin, Weissman and Ochoa55
Governance
For studies to be incorporated into DRAGON-Data, the lead principal investigator needed to confirm approval from their institutional ethics committee. The protection and confidentiality of participant data were of the utmost importance throughout the design of DRAGON-Data, and a number of safeguards were put in place to ensure the security, integrity, accuracy and privacy of participant data. First, in line with the required safeguards for processing special category data stipulated in the EU General Data Protection Regulation (GDPR; Article 89),56 the principle of data minimisation was respected, with only limited individual-level data being requested from research groups. Furthermore, as a means of maintaining the confidentiality and privacy of participants, all data were pseudonymised, and no personal or phenotypic information that allowed individuals to be re-identified was retained. As genome-wide genetic information cannot effectively be anonymised without compromising its integrity,Reference Erlich, Williams, Glazer, Yocum, Farahany and Olson57 all researchers accessing it must explicitly state that they will not attempt participant re-identification.
This project was conducted in line with Cardiff University's Research Integrity and Governance Code of Practice, and ethical approval for the curation and development of the DRAGON-Data was obtained from Cardiff University's School of Medicine Research Ethics Committee (reference 19/72). As described above, procedural safeguards were put in place to ensure secure, managed access to the data-set through the HAWK system, with the most privileges restricted to the ‘core analyst team’. In addition, a process of oversight has been implemented for the approval of secondary research proposals, which are reviewed by the lead principal investigator of each contributing sample, and must be approved before access to relevant requested data can be granted. All genetic analyses carried out by secondary investigators also have to be carried out within the HAWK environment, which allows their monitoring and auditing to rapidly detect data misuses.
Challenges of data-sharing partnerships
The organisational challenges faced by DRAGON-Data highlight that potential data-sharing requirements should be considered, as much as reasonably possible, at the outset of any research study. Studies will benefit from having a data-sharing policy in place before the collection of any data, as a means of maximising the value of collected data, increasing transparency and ensuring responsible future sharing of data. This will depend on sharing with whom, and for what purpose. Consent processes have changed dramatically over the past 30 years and historical studies will not all have explicit consent on the data-sharing practices that are more commonly included today.Reference Rehm, Page, Smith, Adam, Alterovitz and Babb58 In certain situations, additional ethical permission may be required for data-sharing when the sample is historical and or individuals can no longer be contactable. Thus, data-sharing without that explicit permission can only occur within certain circumscribed situations.
When obtaining consent for future research, researchers should aim to be as inclusive as possible, and allow participants to provide their written informed consent for general areas of research activity. In the context of broad consent, we would also advise the implementation of an oversight mechanism for the approval of future research studies. Participants entrust researchers to make reasonable decisions regarding future research on their behalf, and the process of oversight adds further protection to participants, since not all future research uses can be predicted.
Limitations
Although there is rich demographic and clinical data available on patient cohorts in DRAGON-Data, the data on those without mental health disorders (‘controls’ in experimental study designs) is comparatively smaller and less detailed. The majority of the controls in DRAGON-Data came from the NCMH (n = 3508), and completed a brief interview that included demographic information and screening for the presence of psychiatric disorders. Four of the remaining studies in DRAGON-Data also collected data on participants without psychiatric diagnoses, but these were recruited because there were an unaffected sibling of a proband (Sib-Pairs cohort) or were ND-CNV carriers (ECHO, IMAGINE and DEFINE cohorts). Although these samples might not be representative of a standard control population, given their ascertainment, they might still be relevant for future DRAGON-Data studies. For example, merged data-sets with affected, relatives of affected, and unaffected individuals have been used for research into the additivity of risk factors for neurodevelopmental traits and in the validation of polygenic score methods.Reference Klei, McClain, Mahjani, Panayidou, De Rubeis and Anna-Carin59
All of the studies in DRAGON-Data predated the publication of the ICD-11, which may have implications for how findings obtained using the data translate to current clinical practice. However, DRAGON-Data includes variables covering individual symptoms, onset and duration of illness, episodes and illness course, and this data could be used to derive diagnoses according to the most recent diagnostic criteria (ICD-11 and DSM-5). There was variation across the studies in how biological sex at birth and gender identity was measured and recorded, and many studies did not include standardised questions to probe sex at birth or gender identity. This is a common problem in historical data-sets, and even recent census questions on sex and gender for social science research vary across countries.Reference Kennedy, Khanna, Simpson, Gelman, Jia and Teitler60 An advantage of DRAGON-Data is the inclusion of genetic data, meaning biological sex can be identified for most participants. In addition, the largest sample with phenotype data in DRAGON-Data, the NCMH, included questions for both sex at birth and gender identity.
Finally, there is limited ancestry diversity within DRAGON-Data, as all of the included samples were recruited in the UK and contained a majority of individuals with European ancestry. Therefore, findings from DRAGON-Data may not be generalisable to individuals from different populations, although some cohorts (e.g. CLOZUK) can contain as much as 20% of non-European individuals from different ancestries or admixed backgrounds.Reference Legge, Pardiñas, Helthuis, Jansen, Jollie and Knapper61
Open data prospects
At present, DRAGON-Data has been designed as a way of maximising the present and future utility of data collected at the MRC Centre for Neuropsychiatric Genetics and Genomics at Cardiff University during the past 30 years. Given the complexity of the data, particularly the phenotypic portion, the first cross-disorder analyses of DRAGON-Data have been carried out by members of the core analytic team and the participating investigator groups. Results of these analyses will be shared through Cardiff University online data repositories and communicated through standard scientific channels such as peer-reviewed publications. Ultimately, through adapting the PGC open-science model62 and taking advantage of the data-sharing frameworks supported by Health Data Research UK, such as the DATAMIND Hub,63 the DRAGON-Data resource will be available for external investigators where individual study consent and ethics permit such data-sharing. This will ensure compliance with the permissions and ethics of individual studies, and will be based on the secondary analysis principles detailed in the Governance section.
Supplementary material
Supplementary material is available online at https://dx.doi.org/10.1192/bjo.2022.636
Data availability
All data relevant to the study are included in the article. Data from individual studies are available from multiple repositories and open resources as described in their parent publications (Table 1). Code for the genomic data harmonisation pipelines is available in a GitHub repository (https://github.com/CardiffMRCPathfinder/).
Author contributions
M.J.O., J.H. and J.T.R.W. conceptualised and designed the study. A.J.L. designed and implemented the phenotypic data curation protocol into DRAGON-Data. L.H. and A.F.P. designed and implemented the genotypic data curation protocol into DRAGON-Data. A.J.L., L.H. and S.K. reviewed and implemented governance and ethical protocols into DRAGON-Data. S.S.A., J.I.B., M.B.M.v.d.B., S.J.R.A.C., N.C., M.C.O., I.R.J., G.K., K.L., J.M., F.R., N.P.R., A.T., R.A. and M.J.O. contributed genetic and/or phenotypic data from individual studies into DRAGON-Data. A.J.L., J.F.G.U., L.H., S.S.A., S.J.R.A.C., K.L., J.M., R.A. and A.F.P. led the genetic and/or phenotypic data curation of individual DRAGON-Data studies. A.J.L., S.K., J.F.G.U., L.H. and A.F.P. wrote the draft of the manuscript and incorporated the revisions by the co-authors. All authors reviewed the manuscript for intellectual content, contributed to revisions and approved the final version for publication.
Funding
This work was supported by a Medical Research Council (MRC) ‘Mental Health Data Pathfinder’ Award (number MC_PC_17212). Recruitment, genotyping data collection and curation of the included samples was supported by MRC Centre (grant number MR/L010305/1), Program (grant number MR/P005748/1) and Project (grant numbers MR/L010305/1, MR/L011166/1, MR/L011794/1 and MR/N022572/1) grants to Cardiff University; funding from the Wellcome Trust (grant numbers 078901, 079711 and 220488/Z/20/Z); Health and Care Research Wales (grant number 514032); Jules Thorn Charitable Trust (grant number JTA/06); Economic and Social Research Council (grant number ES/J011657/1) Action Medical Research and Baily Thomas Charitable Fund. The National Centre for Mental Health was funded by the Welsh Government through Health and Care Research Wales. We also acknowledge the support of the Supercomputing Wales project, which is part-funded by the European Regional Development Fund via the Welsh Government. J.F.G.U. was supported by a Wellcome Trust GW4-CAT Clinical Doctoral Fellowship (number 222849/Z/21/Z). S.J.R.A.C. was supported by a Medical Research Foundation Fellowship (number MRF-058-0015-F-CHAW). J.M. was supported by a NARSAD Young Investigator Grant from the Brain & Behavior Research Foundation (grant number 27879). A.F.P. was supported by an Academy of Medical Sciences ‘Springboard’ Award (number SBF005\1083). This research was funded in whole, or in part, by the Wellcome Trust. The funding organisations had no role in the design and conduct of the study; collection, management, analysis and interpretation of the data; preparation, review or approval of the manuscript; and decision to submit the manuscript for publication.
Declaration of interest
M.C.O., M.J.O. and J.T.R.W. are investigators on a grant from Takeda Pharmaceuticals Ltd. to Cardiff University, for a project unrelated to the work presented here.
eLetters
No eLetters have been published for this article.