Introduction
A fundamental feature of native language production is that it is adaptive – attuned to recent linguistic experience. For example, speakers often reuse recently encountered words (Brennan & Clark, Reference Brennan and Clark1996) or syntactic structures (Bock, Reference Bock1986; Pickering & Garrod, Reference Pickering and Garrod2004), even when alternative choices would be equally felicitous. Regardless of the specific mechanisms underlying these effects of recent experience, they are considered to be an important aspect of language production (Chang, Dell & Bock, Reference Chang, Dell and Bock2006; H. H. Clark, Reference Clark1996; Dell & Ferreira, Reference Dell and Ferreira2016; Ferreira & Bock, Reference Ferreira and Bock2006; Jaeger & Snider, Reference Jaeger and Snider2013; Pickering & Garrod, Reference Pickering and Garrod2004, Reference Pickering and Garrod2013; Horton & Gerrig, Reference Horton and Gerrig2016). While the basic properties of such adaptation are now well-documented for native language (L1) production (for review, Pickering & Ferreira, Reference Pickering and Ferreira2008), it remains largely an open question to what extent speakers of a second language (L2) adapt their production to recent linguistic experience following principles similar to those of native speakers. Understanding how recent input affects L2 use is also relevant to L2 learning: the longitudinal acquisition of an L2 likely depends on how L2 users integrate recent experiences into their current L2 knowledge (see also Kaan, Reference Kaan2014; Kaan & Chun, Reference Kaan, Chun, Federmeier and Watson2018; Phillips & Ehrenhofer, Reference Phillips and Ehrenhofer2015).
The present study focuses on the moment-by-moment adaptation of language production to recently encountered input. Specifically, we ask what kind of linguistic structures L2 speakers expect to encounter in their L2 and how these expectations mediate adaptation in production. To address this question, we focus on a property of adaptation that has received increasing attention in recent years, the inverse preference (or inverse frequency) effect: Encountering structures that are less preferred (or less frequent) in a language leads to stronger adaptation than encountering structures that are more preferred (or more frequent) (in production: e.g., Bernolet & Hartsuiker, Reference Bernolet and Hartsuiker2010; Cho-Reyes, Mack & Thompson, Reference Cho-Reyes, Mack and Thompson2016; Hartsuiker & Kolk, Reference Hartsuiker and Kolk1998; Jaeger & Snider, Reference Jaeger and Snider2013; Peter, Chang, Pine, Blything & Rowland, Reference Peter, Chang, Pine, Blything and Rowland2015; Scheepers, Reference Scheepers2003; Segaert, Weber, Cladder-Micus & Hagoort, Reference Segaert, Weber, Cladder-Micus and Hagoort2014; in comprehension: e.g., Arai & Mazuka, Reference Arai and Mazuka2014; Fine & Jaeger, Reference Fine and Jaeger2013). For example in English, active sentences are more frequent than passive sentences, and English speakers are primed more by (i.e., they adapt more strongly to) passive sentences than active sentences (e.g., Bock, Reference Bock1986). Some of these studies further suggest that this effect is about speakers’ expectations: it is less expected structures – i.e., not only overall less frequent, but contextually less preferred structures – that lead to stronger adaptation (Arai & Mazuka, Reference Arai and Mazuka2014; Bernolet & Hartsuiker, Reference Bernolet and Hartsuiker2010; Jaeger & Snider, Reference Jaeger and Snider2007, Reference Jaeger and Snider2013). This inverse preference effect has played an important role in theoretical debates about priming: the effect follows directly from implicit learning accounts of priming (Chang et al., Reference Chang, Dell and Bock2006; Reitter, Keller & Moore, Reference Reitter, Keller and Moore2011). According to these learning accounts, speakers have implicit context-sensitive expectations about the upcoming input based on their long-term linguistic experience, and they update these expectations based on recently observed linguistic input (Dell & Chang, Reference Dell and Chang2014; Jaeger & Snider, Reference Jaeger and Snider2013). Though several specific learning mechanisms have been proposed, they share the prediction that the degree of change in expectations after a newly observed input (e.g., a passive sentence) is an inverse function of the prior expectedness of the input – or, equivalently, a positive function of the prediction error experienced while processing the input.Footnote 1 This makes the inverse preference effect a suitable behavioural signature to investigate changes in expectations.
If such implicit learning mechanisms are indeed continuously active during language processing (as is often assumed), we should also see their consequences during L2 use and learning (see, e.g., Ellis, Reference Ellis2006; Kaan, Reference Kaan2014; Kaan & Chun, Reference Kaan, Chun, Federmeier and Watson2018; MacWhinney, Reference MacWhinney, Robinson and Ellis2008; Phillips & Ehrenhofer, Reference Phillips and Ehrenhofer2015). This raises the following questions. If L2 learners – like native speakers – adapt to input as a function of their expectations, are L2 learners’ expectations determined by their L1, their L2, or a mixture of both (see also Flett, Branigan & Pickering, Reference Flett, Branigan and Pickering2013; Jackson & Ruf, Reference Jackson and Ruf2017; Kaan & Chun, Reference Kaan and Chun2017, discussed below)? And, how does this change as learners gain more experience and become more proficient in their L2 (see Leal, Slabakova & Farmer, Reference Leal, Slabakova and Farmer2016)? These questions – about whether L2 learners can acquire and draw on expectations that reflect the statistics of L2 language use, rather than being limited to expectations based on their prior L1 experiences – have been of continued interest, as they reflect on potential limitations of L2 learning (e.g., Brown & Gullberg, Reference Brown and Gullberg2013; Dussias & Cramer Scaltz, Reference Dussias and Cramer Scaltz2008; Dussias & Sagarra, Reference Dussias and Sagarra2007; Hohenstein, Eisenberg & Naigles, Reference Hohenstein, Eisenberg and Naigles2006; van Bergen & Flecken, Reference van Bergen and Flecken2017; for theoretical discussion, see Ellis, Reference Ellis2006; MacWhinney, Reference MacWhinney, Robinson and Ellis2008). Here we contribute to and extend this literature, using a cumulative priming paradigm in the domain of verb lexicalization in motion encoding (Talmy, Reference Talmy2000; see below). Native speakers of Spanish and L1-Swedish learners of L2 Spanish were primed in Spanish with lexicalization patterns that were either typical of Spanish or Swedish. We seek to test the prediction that less expected input will lead to greater adaptation. Crucially, in the case of L2 learners, expectations themselves might change with increasing proficiency in the L2, leading to changing adaptation patterns as a function of L2 proficiency.
Before elaborating on the aims of the present study, we review existing evidence as to whether L2 speakers adapt their production as a function of their expectations, and introduce the cross-linguistic difference in lexicalization that motivates the design of the present study.
Expectation-based adaptation in L2 learners
Extensive evidence of priming in non-native speakers suggests that L2 speakers develop abstract linguistic representations in the target language that are partly shared between languages (see Hartsuiker & Bernolet, Reference Hartsuiker and Bernolet2017 for a recent review). What is less clear, however, is the relation between L2 learners’ prior linguistic experience (both L1 and L2) and the strength of priming effects. The inverse preference effect should lead L2 learners to adapt more strongly to unexpected than expected structures in the L2. But what structures do L2 learners expect in their L2, and are expectations mainly shaped by the learner's L1 or by their L2 experience?
One line of research that speaks to the issue of L2 expectations is work on predictive sentence processing. Native speakers expect upcoming words and referents based on what they have heard so far (for overviews, see Huettig, Reference Huettig2015; Kuperberg & Jaeger, Reference Kuperberg and Jaeger2016). Regarding whether L2 speakers develop and deploy similar gradient expectations, however, the evidence is mixed.
Some studies suggest that L2 expectations differ qualitatively from native expectations (Ito, Martin & Nieuwland, Reference Ito, Martin and Nieuwland2017; Martin, Thierry, Kuipers, Boutonnet, Foucart & Costa, Reference Martin, Thierry, Kuipers, Boutonnet, Foucart and Costa2013) or that L2 speakers have a reduced ability to generate expectations in the L2 (Grüter, Lew-Williams & Fernald, Reference Grüter, Lew-Williams and Fernald2012). Other evidence suggests L2 speakers do generate expectations that they can use predictively in L2 processing (e.g., Dussias, Kroff, Tamargo & Gerfen, Reference Dussias, Kroff, Tamargo and Gerfen2013; Foucart, Martin, Moreno & Costa, Reference Foucart, Martin, Moreno and Costa2014; Hopp, Reference Hopp2013; Leal et al., Reference Leal, Slabakova and Farmer2016; van Bergen & Flecken, Reference van Bergen and Flecken2017). Importantly for the present study, previous work suggests that the expectations L2 speakers generate partly depend on the characteristics of their L1 (Dussias et al., Reference Dussias, Kroff, Tamargo and Gerfen2013; Molinaro, Giannelli, Caffarra & Martin, Reference Molinaro, Giannelli, Caffarra and Martin2017; van Bergen & Flecken, Reference van Bergen and Flecken2017). For example, van Bergen and Flecken investigated the online language understanding of L1 German, L1 English, and L1 French learners of L2 Dutch in a visual world eye-tracking study. While German and Dutch share the semantics of position verbs like zetten (‘put in a standing position’) and leggen (‘put in a lying position’), French and English do not share this property with Dutch. Van Bergen and Flecken found that L1 German learners of Dutch were able to predict (as indexed by anticipatory looks) the type of object that followed these verbs (e.g., bottle and book for zetten and leggen, respectively), whereas L1 English or French learners could not (van Bergen & Flecken, Reference van Bergen and Flecken2017). This would suggest that, at least in comprehension, learners do transfer their expectations from their L1 to the L2.
Studies like the above are informative about L2 learners’ expectations during L2 comprehension. Here we are interested in the role of expectations during production and, specifically, in how expectations mediate the effect of recently experienced input on subsequent production. Do learners adapt their production to recent L2 input as a function of their expectations? And are their expectations based on their L1 or L2 experience, or both? To our knowledge, three studies have addressed this issue.
Flett and colleagues tested whether advanced L2 English speakers’ structural preferences for prepositional object (PO) structures (The cowboy sells the apple to the nun) or double object (DO) structures (The cowboy sells the nun the apple) were influenced by L1-based preferences or only by experience in the L2 (Flett et al., Reference Flett, Branigan and Pickering2013). They compared two groups of English learners, whose L1s had different preferences for POs and DOs (Spanish and German), with respect to their susceptibility to being primed by each structure within their L2. The authors predicted that, if L2 learners were affected by their native language preferences, then Spanish learners would be more strongly primed by DOs than German learners, because Spanish only allows PO datives while German allows both. There was no such effect, from which the authors concluded that, in proficient bilinguals, L2 processing is not affected by L1 experience.
More recently, Kaan and Chun (Reference Kaan and Chun2017) investigated priming and cumulative adaptation of POs and DOs among native English speakers and Korean L2 learners of English of intermediate proficiency. Both English and Korean have DO and PO structures, but DOs are more frequent than POs in English, while the opposite is true in Korean. If Korean learners of L2 English transfer their structural preferences from their L1, they should prefer POs over DOs and – by the inverse preference effect – adapt more strongly to the latter than the former structure, while the opposite should be the case for native English speakers. This was indeed what the authors found, which suggests that L1-based expectations mediate how L2 speakers adapt to recent input at intermediate proficiency levels (Kaan & Chun, Reference Kaan and Chun2017).
Finally, Jackson and Ruf (Reference Jackson and Ruf2017) investigated production priming of word order variation in English learners of L2 German at lower proficiency levels. Their design compared priming for two types of adverbial fronting in German: temporal fronting like In winter the grandpa drinks hot chocolate (given here in its English transliteration) and locative fronting like On the mountain the grandpa drinks hot chocolate. Temporal fronting is more common in English (the learners’ L1) than locative fronting. By the inverse preference effect, this should lead to a stronger priming effect for locative than temporal phrases if learners are indeed transferring their L1 preferences. The results did not support this effect: short-term priming was of similar magnitudes for both types of fronting, and only temporal phrases (preferred in the L1) led to cumulative and long-term priming. However, the interpretation of these results is complicated by the fact that the same preference seems to hold in both L1 English and L2 German (Jackson & Ruf, Reference Jackson and Ruf2017, pp. 337–338), making it difficult to tease apart whether learners were failing to transfer L1-based expectations or to adapt to the L2 target patterns. An additional limitation of this study is that it did not include native German controls, so it is unclear if the expected inverse preference effect holds among natives in the first place.
In sum, the available evidence suggests that L2 speakers at intermediate proficiency levels adapt production to recent input partly as a function of their L1 preferences (Kaan & Chun, Reference Kaan and Chun2017), whereas highly proficient L2 speakers show the same priming patterns as native speakers (Flett et al., Reference Flett, Branigan and Pickering2013). These results are compatible with the hypothesis that L2 learners transition from primarily L1- to L2-based expectations during the course of L2 acquisition, as postulated by experienced-based accounts of L2 acquisition (e.g., Ellis, Reference Ellis2002; MacWhinney, Reference MacWhinney, Robinson and Ellis2008; Pajak, Fine, Kleinschmidt & Jaeger, Reference Pajak, Fine, Kleinschmidt and Jaeger2016). However, a direct test of this hypothesis within a single study has so far been lacking.
L2 lexicalization preferences as a test domain
In the present study, we focus on the well-known cross-linguistic differences in motion event descriptions (Talmy, Reference Talmy and Shopen1985, Reference Talmy2000; for an overview, see Filipović & Ibarretxe-Antuñano, Reference Filipović, Ibarretxe-Antuñano, Dabrowska and Divjak2015). Satellite-framed languages like Swedish and English tend to use the main verb of a sentence to express the manner of an action (push, roll). In this type of languages, an event like the one shown in Figure 1 would be typically described as He pushes the package up the dune. In contrast, verb-framed languages like Spanish preferentially use main verbs to encode the path of motion (Spanish: subir ‘ascend’, entrar ‘enter’). A typical Spanish description of the event in Figure 1 would be Sube la duna con/empujando el paquete (‘He ascends the dune with/pushing a package’).
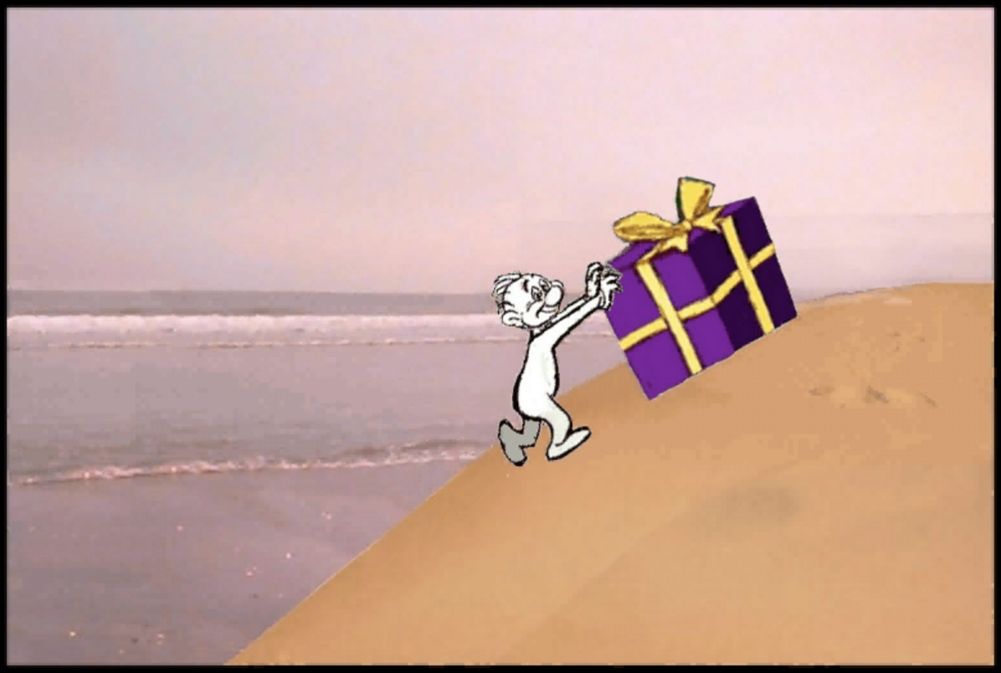
Fig. 1. (Colour online only) Example of a dynamic caused motion event used in the study (here shown as a still only).
These differences, however, are gradient rather than absolute, so that many situations can be described using either path or manner verbs in one and the same language (Beavers, Levin & Tham, Reference Beavers, Levin and Tham2010; Croft, Barðdal, Hollmann, Sotirova & Taoka, Reference Croft, Barðdal, Hollmann, Sotirova, Taoka and Boas2010). For example, while not being the dominant strategy in Spanish, native speakers of Spanish sometimes also describe the event in Figure 1 with a manner verb, as in Empuja un paquete por la duna ‘He pushes a package along the hill’ (Montero-Melis, Reference Montero-Melisunder review; Montero-Melis & Bylund, Reference Montero-Melis and Bylund2016). Acquiring lexicalization preferences, then, involves gaining knowledge about which types of verbs are more appropriate in a language when describing a given situation. For Swedish learners of L2 Spanish – the learner group investigated here – the challenge is to attune to the characteristic target lexicalization pattern in Spanish (path verbs) in the face of input that sometimes includes manner verbs, the lexicalization pattern that is strongly preferred in their L1 Swedish. To the extent learners’ expectations in their L2 are based on their L1 experience, Swedish learners of Spanish should have opposite expectations to Spanish native speakers about how motion events are lexicalized. We use this cross-linguistic difference to explore whether Swedish learners of L2 Spanish transfer their L1 expectations to their L2, as laid out next.
The present study
The overall aim of this study is to investigate the role of previous linguistic experience on adaptation in production. We focus on the inverse preference effect: speakers should adapt more strongly to input they expect less. Spanish native speakers and L1 Swedish learners of L2 Spanish described events like the one shown in Figure 1 in Spanish. Participants were assigned to one of three conditions: they either read sentences containing path verbs before each description (path priming), or sentences containing manner verbs (manner priming), or they described the events without priming (baseline condition). Manipulating the primed lexicalization pattern between subjects prevents the priming effects of one pattern cancelling out the effects of the other. We use generalized additive mixed models (GAMMs; Wood, Reference Wood2017) to analyze how native speakers and L2 learners cumulatively adapt to the Spanish prime sentences compared to the baseline condition. GAMMs provide a state-of-the-art analysis of time series data and allow for modelling of non-linear relations. Our approach in this study is largely based on visualization of model estimates and their confidence intervals rather than on explicit tests of significance.
Our first question (Q1) concerns whether native Spanish speakers will show an inverse preference effect, such that they adapt more to the less frequent lexicalization in Spanish (manner verbs) than to the more frequent lexicalization (path verbs). This allows us to test whether the inverse preference effect holds in the domain of lexicalization patterns, since most previous studies involved syntactic alternations.
Our second question (Q2) concerns whether Swedish learners of L2 Spanish adapt to the input as a function of both their L1 and L2 experience or only of their L2-specific experience. If L2 learners are not affected by their L1-specific experience, then L2 adaptation to path and manner verbs should be qualitatively indistinguishable from that of natives (as in Flett et al., Reference Flett, Branigan and Pickering2013). If, conversely, learners’ expectations are based on their L1, then they should differ qualitatively from native Spanish speakers in how they adapt to the same input, because a given pattern (e.g., path verbs) will be expected in one language (Spanish) but unexpected in the other (Swedish), or vice versa. In this scenario, we predict that Swedish learners should – compared to Spanish natives – adapt more strongly to path verbs (unexpected in Swedish) and less strongly to manner verbs (expected in Swedish).
Our third and last question (Q3) is whether L2 learner adaptation to input will become more native-like with increasing L2 proficiency, as indirectly suggested by comparison of the studies summarized above (Flett et al., Reference Flett, Branigan and Pickering2013; Kaan & Chun, Reference Kaan and Chun2017). If learners increasingly fine-tune their expectations to the statistics of the L2 and rely less on their L1 experience with increasing proficiency (cf. Leal et al., Reference Leal, Slabakova and Farmer2016), then more proficient learners should adapt to the input in a way that increasingly resembles native speaker adaptation. Crucially, we assess – for the first time for this type of study – the role of L2 proficiency within our study, rather than by comparing across studies.
The predictions outlined in the preceding paragraphs are derived from learning accounts that link changes in speakers’ productions to the continuous adaptation of implicit expectations based on recently observed linguistic input (e.g., Chang et al., Reference Chang, Dell and Bock2006; Dell & Chang, Reference Dell and Chang2014; Jaeger & Snider, Reference Jaeger and Snider2013). While traditional transfer accounts in L2 acquisition focus on overt transfer of structural patterns of the L1 into the L2 (Andersen, Reference Andersen, Gass and Selinker1983; Jarvis, Reference Jarvis2000; Selinker, Reference Selinker1969; Toribio, Reference Toribio2004), we examine instead the possible transfer of expectations from the L1 that will manifest itself in how speakers will adapt to L2 input (Flett et al., Reference Flett, Branigan and Pickering2013; Jackson & Ruf, Reference Jackson and Ruf2017; Kaan & Chun, Reference Kaan and Chun2017). Thus, the current study has the potential to inform the notion of transfer in L2 processing and acquisition.
Method
Participants
A total of 119 participants took part in the experiment in exchange for payment. Fifty-nine of them were native Spanish speakers living in Madrid with no advanced knowledge of other languages (henceforth natives; Mage = 22, SD = 1.9). The other 60 were Swedish adult learners of Spanish who lived in Sweden at the time of the experiment (henceforth learners; Mage = 36, SD = 13.7).Footnote 2 The learners’ mean age of onset for learning Spanish was 18.3 years (SD = 8.6; range: 7–63). Participants were randomly allocated to one of three conditions described below: baseline, path priming, manner priming. One learner was excluded due to recording failure.
The learners’ proficiency was first estimated in a short interview prior to the experiment to be at least that of an independent user (B1) according to the CEFR (Council of Europe, 2011). Additionally, an offline cloze test was administered after the experiment to obtain a continuous (cf. van Hell & Tanner, Reference van Hell and Tanner2012), experimentally elicited measure of global L2 proficiency (see Tremblay, Reference Tremblay2011). The test consisted of a 257-word text with every seventh word removed, leaving 37 gaps. Each gap was scored as 1 if the word was semantically and grammatically correct or as 0 otherwise (diacritics were disregarded). Learners’ mean score on this test was 24.1 (SD = 8.5). A one-way ANOVA showed there was no difference in learners’ scores across the three between-participant conditions: F(2, 56) = .135, p = .87 (nor was there any significant difference between groups with respect to any other variables we collected concerning their L2 status, see Appendix S2 of the Supplementary Materials). We refer to the score on the cloze test as ‘proficiency score’. Table 1 shows participant counts by condition and group. All background information collected about the participants is reported in Appendix S2 of the Supplementary Materials (Supplementary Materials).
Table 1. Number of participants and mean L2 proficiency score (and SD) by condition.

Materials
Event descriptions were elicited using 32 short animations depicting situations of caused motion, originally developed by Hendriks and colleagues (Hendriks, Hickmann & Demagny, Reference Hendriks, Hickmann and Demagny2008). All animations were about seven seconds long and showed events that involved an agent moving objects in varying manners along different paths (e.g., pushing a package up a sand dune or dragging a chair into a cave). For each of the 32 events, we created one pair of prime sentences. The two sentences always differed in whether the main verb was a path verb or a manner verb, but the other lexical items were the same in each pair, and the syntactic structure of the sentences was balanced across conditions. Table 2 shows the four different path and manner verbs used in the prime sentences.
Table 2. Path verbs and manner verbs used in the priming conditions.
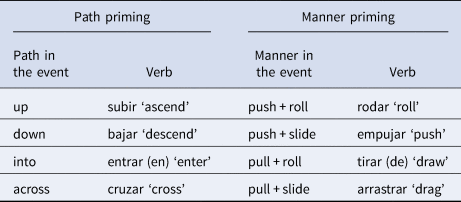
Each prime verb matched the specific path or the specific manner shown in the following event. Verbs were selected among the most frequently used verbs in native Spanish descriptions elicited in a previous norming study involving the same events (reported in Montero-Melis & Bylund, Reference Montero-Melis and Bylund2016). All target stimuli are described, and their matching prime sentences listed, in Supplementary Materials Appendix S1 (Supplementary Materials). An additional training item showed a similar caused motion event and was paired with a Spanish sentence that did not contain any motion information.
Procedure
Figure 2 shows a schematic outline of the experimental session. Participants were tested individually in Spanish by a native speaker of Spanish. Learners first carried out a computerized vocabulary task in which pictures of objects and landmarks had to be matched to the correct Spanish word among three alternatives. This task was deliberately easy and served to introduce learners to relevant vocabulary for the upcoming event description task; distractor words came from the prime sentences used in the main part of the experiment (see below), thus familiarizing learners with this vocabulary as well. No verbs, which were our critical manipulation, were included in the vocabulary task. Mean overall accuracy on the vocabulary task was 97.4% (SD = 5%) and mean accuracy was above 97% in all conditions.
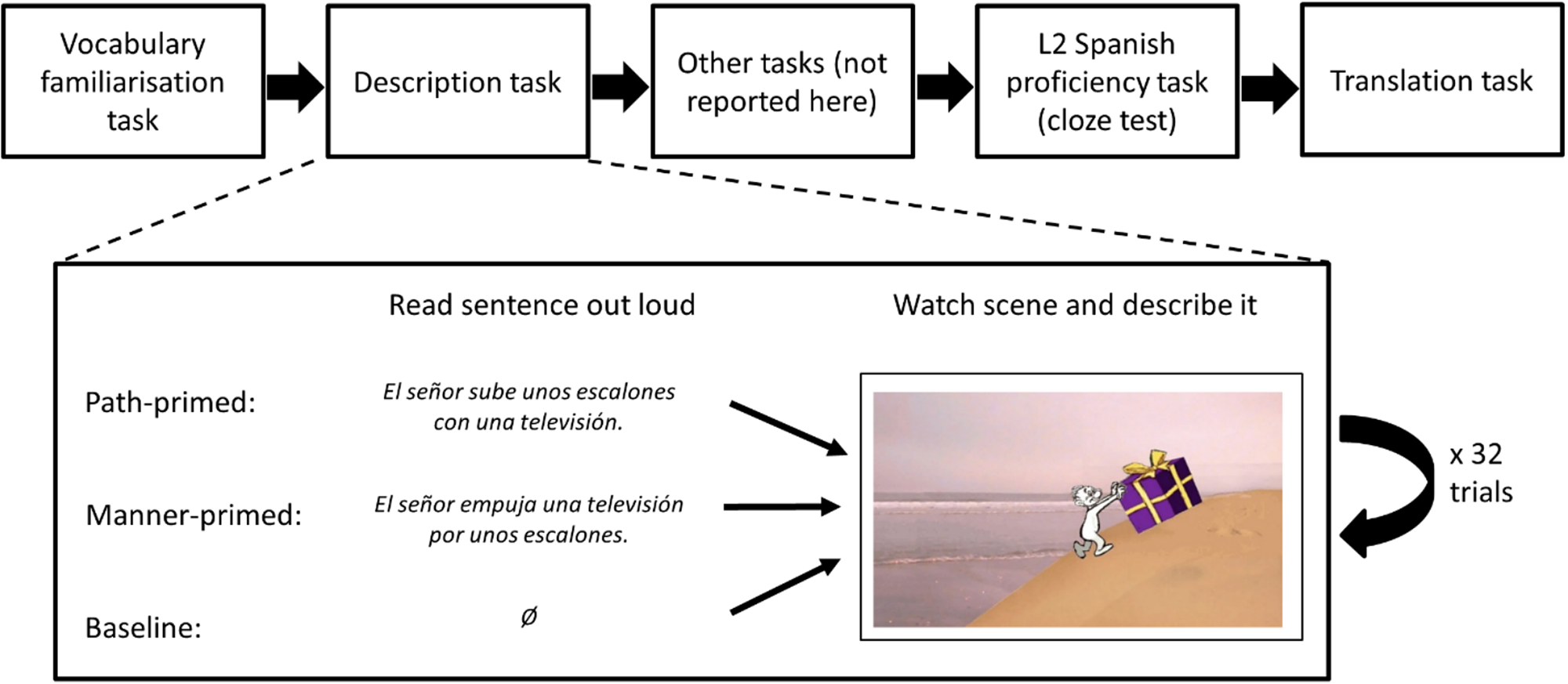
Fig. 2. (Colour online only) Schematic outline of the experimental session for L2 Spanish learners. Native speakers only carried out the description task (shown in greater detail).
The main task was the description task, which was identical for native speakers and L2 learners. This task either involved priming (for the path-priming and manner-priming conditions), or it did not (baseline condition). Participants had to watch each of the 32 events in its entirety. After each clip, participants described it, focusing on what had happened in the event. Events were shown in random order, with the constraint that two identical path or manner values were never shown in sequence. Participants in either priming condition additionally had to read a priming sentence aloud before describing each event. The verb in the priming sentence always matched the action in the upcoming event (see Appendix S1 of Supplementary Materials). Participants in the baseline condition read no sentences.
The task was self-paced and took approximately 15 minutes to complete. After this, the learners carried out a similarity arrangement task reported elsewhere (see Montero-Melis, Jaeger & Bylund, Reference Montero-Melis, Jaeger and Bylund2016), a cloze test to assess their Spanish proficiency (see Participants), and a translation task to assess they understood the meaning of the verbs used in the priming sentences.Footnote 3
Data coding and analysis approach
Each description was coded for whether the main verb encoded path information (e.g., subir ‘ascend’), manner information (e.g., empujar ‘push’), or other information (e.g., mover ‘move’). If both a path verb and a manner verb occurred as main verbs in a description (e.g., Sube la duna y empuja un paquete ‘He ascends the dune and pushes a package’), this was counted as an instance of both a path verb and a manner verb.Footnote 4
We briefly provide an overview of our analysis approach. All reported analyses model a binary outcome (1 vs. 0). The interpretation of this outcome depends on an indicator variable that is included as predictor in all our analyses, Verb Type (path vs. manner verb). When the value of the indicator variable is ‘path’, then an outcome of 1 means that the main verb in a participant's description on that trial expressed path information, and an outcome of 0 means the main verb did not express path. When the value of the indicator variable is ‘manner’, then an outcome of 1 means that the main verb expressed manner, and an outcome of 0 means it did not. To illustrate: the description Sube por la duna con un paquete (‘He ascends the dune with a package’), which contains a path verb only, would be coded as 1 when uttered by a path-primed participant or by a baseline participant being compared to a path-primed participant (in this case, the value of the indicator variable Verb Type is path). The same utterance, however, would be coded as 0 if uttered by a manner-primed participant or by a baseline participant being compared to a manner-primed participant (in which case Verb Type is manner). This approach lets us analyze an outcome that is underlyingly multinomial (see footnote 4) in a simpler multi-level model predicting the binary outcome of interest: the log-odds of producing a path/manner verb (as indicated by the Verb Type variable) or not using it. All analyses were conducted in R version 3.5.1 (R Development Core Team, 2013).
Further details are provided as part of the analyses below, as we employ both generalized linear mixed models (GLMMs) and generalized additive mixed models (GAMMs), depending on the question. In the main text, we rely on model visualizations to interpret the GAMMs (Winter & Wieling, Reference Winter and Wieling2016). Model summaries with detailed statistics are provided in Supplementary Materials (Supplementary Materials), and full analysis scripts and data files are publicly shared through a Dataverse repository at https://doi.org/10.7910/DVN/TOJ1UH.
Results
We first analyse the results from the baseline conditions (i.e., no priming) to assess overall production biases for path vs. manner verbs in native and L2 learners. We then address each of the three research questions in turn.
Baseline condition: Native and L2 speakers’ lexical preferences in the absence of priming
We expected native Spanish speakers to use path verbs mostly and to use manner verbs less frequently because Spanish is a verb-framed language (e.g., Sebastián & Slobin, Reference Sebastián, Slobin, Berman and Slobin1994; Talmy, Reference Talmy2000). Regarding the Swedish L2 learners, there are two competing factors that could potentially influence the results. On the one hand, L2 learners may transfer their L1-based Swedish pattern, which would lead them to mostly produce manner verbs (see Hendriks et al., Reference Hendriks, Hickmann and Demagny2008). On the other hand, the oral nature of the task might constrain L2 learners to produce whatever Spanish verb is easy to retrieve at the time of production. Since path verbs are more frequent than manner verbs in Spanish (see Larrañaga, Treffers-Daller, Tidball & Ortega, Reference Larrañaga, Treffers-Daller, Tidball and Ortega2012), learners could thus use path verbs simply because of frequency effects (Ellis, Reference Ellis2002), regardless of their underlying lexicalization preferences.
Table 3 shows verb lexicalization choices of L2 learners and native speakers in each of the three conditions. Focusing for now on the baseline conditions, native speakers used path verbs (56.5%) more often than manner verbs (34.3%), as expected. L2 learners used path verbs (60.4%) with similar frequency to the natives and manner verbs (14%) less frequently than natives.
Table 3. Path and manner main verbs used in the baseline and priming conditions by the two groups (native vs. L2 speakers).
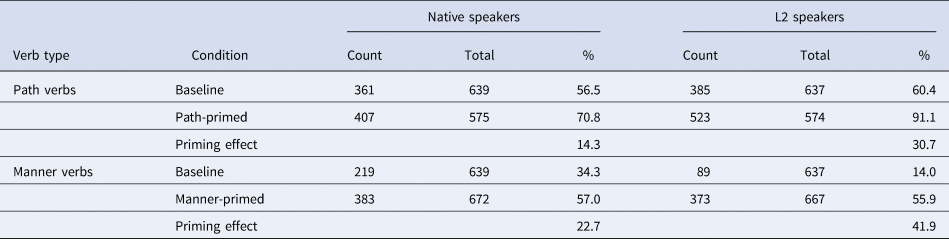
To assess the statistical significance of these patterns, we fitted a generalized (logit) linear mixed-effects model (GLMM) to the data from the baseline condition, using the glmer function from the lme4 package version 1.1-13 (Bates, Maechler, Bolker & Walker, Reference Bates, Maechler, Bolker and Walker2015). The sum-coded predictors were Group (1 = L2 learners, −1 = natives), the indicator variable Verb Type (1 = manner verb, −1 = path verb), and their interaction. The interpretation of the binary outcome (1 vs. 0) depends on the value of the indicator variable: presence/absence of path main verb if Verb Type = path, or presence/absence of manner main verb if Verb Type = manner (see Data coding and analyses). The model was fit with the maximal random-effects structure: by-participant random intercepts and slopes for Verb Type, as well as by-item random intercepts and random slopes for Group, Verb Type, and their interaction (items are each of the 32 scenes described).
The results are illustrated in Figure 3. Manner verbs were overall significantly less frequent than path verbs (Verb Type estimate = −0.66, z = −4.52, p < .001). There was also a main effect of Group, indicating that L2 learners were significantly less likely to produce path and manner verbs than natives (Group estimate = −.31, z = −2.20, p = .03). The interaction between Verb Type and Group was not significant (estimate = −0.42, z = −1.44, p = .15). For the full model output, see Appendix S3 in Supplementary Materials (Supplementary Materials).

Fig. 3. Results from mixed logistic regression model comparing native speakers and L2 learners in the baseline condition. The figures show the log-odds of producing path verbs (left panel) and manner verbs (right panel) as a function of group (L2 learners vs. native speakers). Error bars show model-estimated 95% confidence intervals (based on 1000 simulations of the model-estimated fixed effects using the R-function arm::sim); horizontally jittered dots show model-estimated speaker averages.
To summarize, in the baseline condition native speakers and L2 learners used more path verbs than manner verbs. Although native speakers numerically used manner verbs more often than learners, the difference did not reach significance. Learners’ prevalent use of path verbs in Spanish, rather than manner verbs which are typical in their L1 Swedish, could reflect the constraints of an oral production task in which the verbs produced are those that are most easily retrieved. Question 1 asks what type of verbs native L1 speakers expected to encounter as inferred from their patterns of adaptation to the input. Questions 2 and 3 ask what type of verbs L2 learners expected.
Trial-by-trial adaptation of native speakers (Question 1)
Predictions
Will native speakers show an inverse preference effect in how they adapt to the lexicalization patterns in the input? Theories that attribute adaptation to error-based learning mechanisms predict this to be the case. If so, natives should adapt more strongly to manner verbs (uncommon in Spanish) than to path verbs (preferred in Spanish). This finding would also provide a conceptual replication of previous work on inverse preference effects in L1 syntactic priming (see references in the introduction), but for lexical encoding and on another L1, Spanish.
Results
For the three main analyses (Questions 1–3), it is crucial to capture adaptation over time as the primed participants are exposed to more priming sentences. To model potentially nonlinear adaptation over time, we use generalized additive mixed models (GAMMs) with a logit link function (Wood, Reference Wood2017; for a tutorial, see Baayen, Vasishth, Kliegl & Bates, Reference Baayen, Vasishth, Kliegl and Bates2017). This technique relaxes the assumption that the relation between predictors and outcome variable has to be linear (for example, the use of a verb type could plateau after a certain amount of exposure). Critically, GAMMs penalize the additional complexity inherent in modelling nonlinear relations, so that simple (linear) functional forms are preferred if the data do not strongly support nonlinearity (see Wood, Reference Wood2017 for details). All GAMMs were fitted using the bam function from the mgcv package version 1.8.17, and visualized with the itsadug package version 2.2 (van Rij, Wieling, Baayen & van Rijn, Reference van Rij, Wieling, Baayen and van Rijn2016).
For Question 1, we used a GAMM to model the time course of native speaker adaptation to path and manner verb exposure. This model allows us to assess how much more likely native speakers became over time to use path verbs when they were primed with path verbs (path-priming condition) compared to when they were not (baseline condition) and, similarly, how much more likely they became to use manner verbs when primed with manner verbs (manner-priming condition) compared to when not (baseline condition). To model the two factors, Condition (primed vs. baseline) and Verb Type (manner vs. path verb), as well as their interaction, we created a single four-level Verb Type-by-Condition factor by crossing the levels of Condition and Verb Type (see De Cat, Klepousniotou & Baayen, Reference De Cat, Klepousniotou and Baayen2015 for an identical approach). In addition, the predictor Trial (1 through 32, indicating the order in which scenes were described) was included in the model as a potentially non-linear smooth that could interact with Condition and Verb Type. Default parameterization for the number and placement of knots as well as the type of the smooth was used. The model included a random by-participant factor smooth for Trial (which is the non-linear equivalent in GAMMs of random slopes in GLMMs) and random by-item intercepts.Footnote 5 The full model output and its interpretation is reported in Appendix S4 in Supplementary Materials (Supplementary Materials). For this and all subsequent GAMM analyses we rely on model visualizations when discussing the results, as the estimated coefficients in GAMMs are not directly informative of the shape or direction of an effect and visualization is essential (Winter & Wieling, Reference Winter and Wieling2016; see also Supplementary Materials).
Figure 4 shows trial-by-trial adaptation to path verbs (left panels) and manner verbs (right panels) in natives. We note that the use of path verbs increases throughout the experiment, whereas the use of manner verbs decreases. This general trend holds for both the baseline and the priming groups, and we observe it below for L2 speakers. One explanation for this trend would be self-priming (cf. Jacobs, Cho & Watson, Reference Jacobs, Cho and Watson2018; Jaeger & Snider, Reference Jaeger and Snider2007: Studies 3 and 4): since path verbs are more frequent in Spanish, participants might be slightly more likely to select path verbs at the onset of the experiment, and then tend to stick with this choice throughout the experiment.Footnote 6
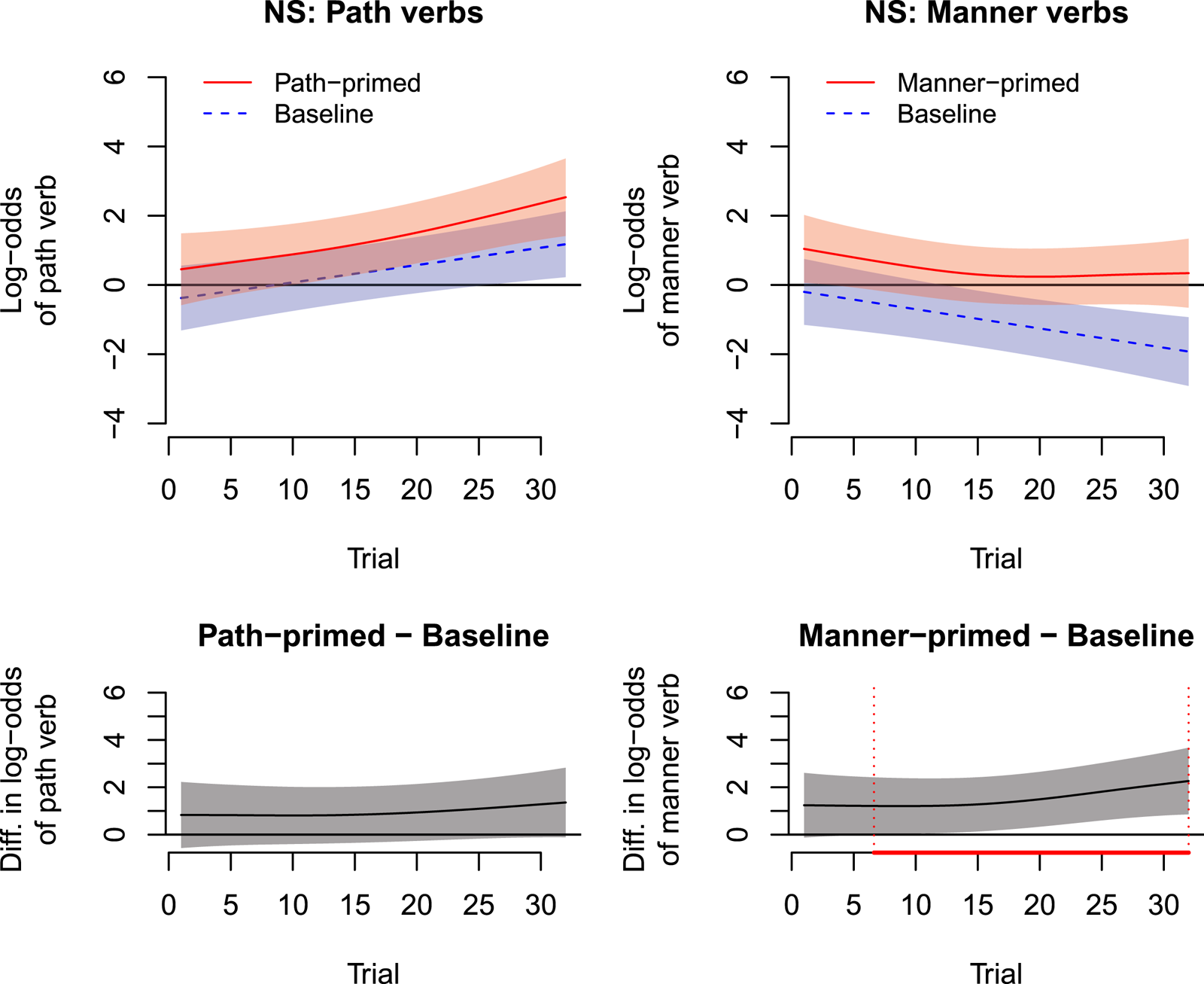
Fig. 4. (Colour online only) Visualization of the GAMM for trial-by-trial adaptation in native speakers (NS). The model predicted log-odds of path verbs (left panels) or manner verbs (right panels) as a function of the continuous predictor Trial (shown on the x-axis, ranging from 1 through 32) and Priming Condition (primed vs. baseline, continuous red and dashed blue lines, respectively). The upper panels show estimated log-odds that the corresponding verb type is used at each trial, including 95% confidence bands. The lower panels show the difference between primed and baseline conditions, that is, the adaptation effect (together with confidence bands). Marked areas in the bottom panels show where there is a significant adaptation effect.
Here we are interested in the adaptation effect shown in the lower panel of Figure 4 – i.e., the difference between the two curves in the upper panel (prime condition minus baseline). The left panels of Figure 4 show that path-primed and baseline participants did not significantly differ in their likelihood of using path verbs; that is, native speakers showed no significant adaptation to path-verb priming. The right panels show that, for manner verbs, a significant adaptation effect did emerge from around the sixth trial: natives who were exposed to sentences with manner verbs increased their use of those verbs relative to the use of manner verbs among participants in the baseline condition (see bottom right panel).
We cannot conclude from the above results that the adaptation effect was significantly different for the path-primed and the manner-primed conditions. We considered several ways to address this question within the GAMM framework, but currently available implementations in mgcv do not allow for an explicit test of the interaction of two factors and a linear predictor (confirmed with Simon Wood, p.c.). We thus pursued an alternative approach and fitted a GLMM (rather than GAMM) to the subset of our data in which the trial effect seems to be linear, though we note that this reduces the statistical power of our analysis (likely substantially). This analysis did not find a significant interaction (see Appendix S4 in Supplementary Materials). For now, we note that the results are qualitatively in line with an inverse preference effect (predicted under error-based learning mechanisms) and we return to issues of power in the discussion.
Trial-by-trial adaptation of L2 learners (Question 2)
Predictions
Our second question is whether L2 learners adapt to recent input as a function of their L1 or L2 experience, or both. If learners’ expectations about the L2 input are mediated by their L1 experience, this should bias them towards expecting manner verbs more, and path verbs less, than native Spanish speakers. In this case, learners should – in comparison to natives – adapt more to path verbs and less to manner verbs. If, on the other hand, learners’ expectations are only a function of their L2 experience, then learners should adapt qualitatively like native speakers: more strongly to manner verbs than to path verbs.
Results
The analysis employed the same GAMM as fitted to the native data in Question 1, only now using the data from L2 learners (see Appendix S5 in Supplementary Materials for model output). Figure 5 shows L2 learners’ trial-by-trial adaptation to the two lexicalization patterns. The upper left panel shows that path-primed L2 learners (solid red line) quickly switched to an almost exclusive use of path verbs. In comparison, learners in the baseline condition used path verbs less often (dashed blue line), which led to a large and early adaptation effect (lower left panel). The path-verb adaptation effect plateaued at about three logits (i.e., >95% probability of path verb) after approximately trial 10 (lower left panel of Figure 5). Regarding manner verbs, manner-primed L2 learners steadily increased their use of manner verbs with respect to baseline (upper right panel), resulting in a constantly increasing cumulative adaptation effect over the course of the experiment (lower right panel). Visual inspection suggests that the initial speed of adaptation (i.e., the slope of the curves in the two lower panels before the plateauing for path verbs) was of a similar magnitude for both verb types.
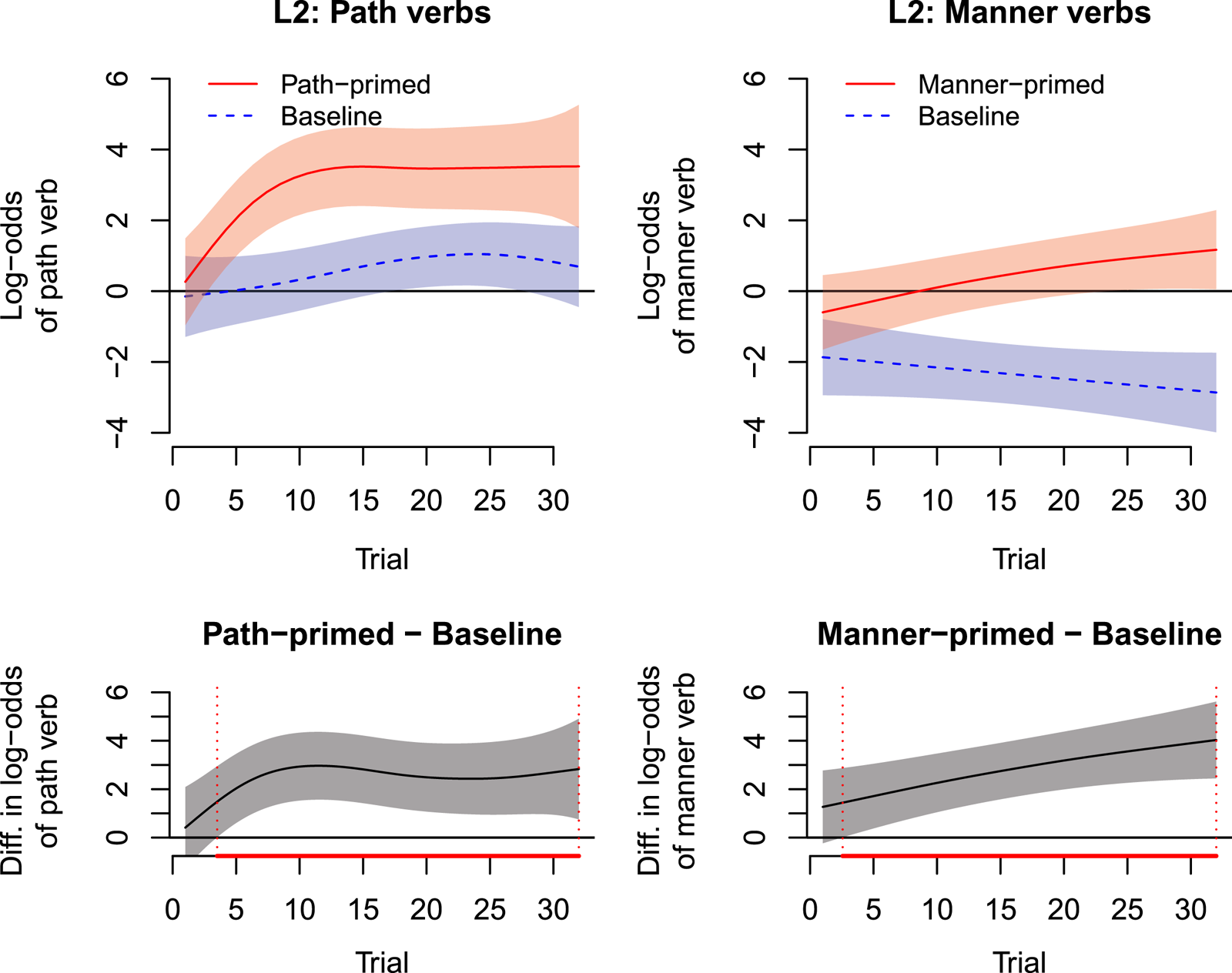
Fig. 5. (Colour online only) Visualization of the GAMM for trial-by-trial adaptation in L2 learners. For interpretation, see caption in Figure 4.
The above analysis shows that learners adapted to both types of priming. Indeed, follow-up GLMM analyses (see Appendix S5 in Supplementary Materials) found no significant difference in adaptation to the two different lexicalization patterns, which is consistent with learners basing their L2 expectations on a mixture of their L1 and L2 experience (though we note again that these analyses – conducted over a subset of our data – likely have substantially reduced power). As pointed out by an anonymous reviewer, the fact that L2 learners overall showed stronger adaptation effects than natives to both patterns (compare lower panels in Figures 4 and 5) has another potential interpretation: Because L2 learners have had less exposure to their L2 than native speakers, their representations might be less stable overall and thus more malleable to priming (as also found in Cho-Reyes et al., Reference Cho-Reyes, Mack and Thompson2016 for aphasic vs. normal speakers; and in Rowland, Chang, Ambridge, Pine & Lieven, Reference Rowland, Chang, Ambridge, Pine and Lieven2012 for children vs. adults), independent of whether L2 speakers are transferring expectations or not. Importantly, the two explanations are not in conflict: L2 speakers can be overall more sensitive to priming and still adapt as a function of their expectations. Here, we are interested in the latter issue. The results of Question 3 indeed suggest this to be the case.
A shortcoming of the analysis of L2 learners presented so far is that it treats L2 learners as a homogenous group, despite the fact that our L2 participants varied in L2 proficiency. Our next and last analysis provides a more informative way to assess how L2 expectations mediate adaptation. We ask how patterns of adaptation in learners change with increasing proficiency.
Effect of L2 proficiency on trial-by-trial adaptation (Question 3)
Predictions
Our third and last question is whether L2 learners come to increasingly resemble native speakers in how they adapt to recent input as they become more proficient in their L2. If learners’ expectations become more attuned to the L2 (and rely less on the L1) with growing proficiency, error-based learning leads to the prediction that learners should also progressively come to adapt more strongly to manner verbs (unexpected in Spanish) and less strongly to path verbs (expected in Spanish). This prediction does not readily follow from accounts of transfer in L2 processing/acquisition that emphasize direct transfer of L1 structures into the L2 (e.g., Andersen, Reference Andersen, Gass and Selinker1983; Jarvis, Reference Jarvis2000; Selinker, Reference Selinker1969; Toribio, Reference Toribio2004). Such a result would provide additional credence to the tendency for inverse preference effects observed for native speakers in the analysis for our first question, and thus for theories that attribute adaptation to error-based or related learning mechanisms.
Results
To assess the role of proficiency for L2 learners’ adaptation patterns, we fitted a new GAMM to the L2 data, this time adding L2 Proficiency Score as a continuous predictor that could interact with Verb Type, Priming Condition, and Trial (see Appendix S6 in Supplementary Materials for details). This model assesses how participants at different proficiency levels adapt to the different lexicalization patterns, by estimating smooth functions like the ones presented in Figure 5 for the range of proficiency levels of the participants in our sample.
Figure 6 shows model estimates for L2 learners’ adaptation effect to path verbs (upper panels) and manner verbs (lower panels) at different L2 proficiency levels. Specifically, each panel shows the difference between the log-odds of using a given verb type in the primed and in the baseline conditions (equivalent to the lower panels in Figures 4 and 5). The figure shows ‘snapshots’ of proficiency levels from 10 to 35 in regular steps for ease of visualization, but note that proficiency entered the model as a continuous predictor.

Fig. 6. (Colour online only) Changes in patterns of L2 adaptation with increasing proficiency. The upper panels show path adaptation (difference in log-odds of path verbs in the path-primed and baseline conditions), the lower panels equivalently manner adaptation. From left to right, the panels show snapshots of model estimates at increasing L2 proficiency levels. Adaptation to path and manner verbs shows opposite patterns as a function of L2 proficiency: it decreases for path verbs but increases for manner verbs.
Comparison of the upper and lower panels in Figure 6 shows that the effect of proficiency on adaptation differed markedly for the two lexicalization patterns. For path verbs, adaptation effects decreased with growing L2 proficiency (see upper panels from left to right). In contrast, adaptation to manner verbs increased with proficiency (see lower panels). The estimated patterns of adaptation at higher levels of L2 proficiency (e.g., proficiency scores of 35) are qualitatively similar to those of native speakers shown in Figure 4 – and this applies to both path and manner verbs.
With increasing proficiency, L2 speakers’ adaptation patterns become more native-like. This suggests that L2 learners at lower proficiency levels start out by transferring their expectations of lexicalization patterns from their L1. As learners get more proficient in the L2, their expectations become increasingly based on their L2-specific experience. These changing expectations mediate adaptation to the L2 input in the direction predicted by implicit learning mechanisms.
General discussion
Our aim has been to investigate how L2 speakers’ expectations change, and how these changing expectations affect adaptation during production. Based on the inverse preference effect, we predicted stronger adaptation to unexpected input. We tested this prediction by exploring the effects of cumulative priming to two competing lexicalization patterns: one that was typical of the learners’ L1 Swedish (use of manner verbs) and another that was typical of their L2 Spanish (use of path verbs). Implicit learning accounts of adaptation predict that L2 learners would change their adaptation patterns with increasing proficiency, starting out with stronger adaptation to path than manner verbs, but inverting that preference with growing proficiency. Generalized Additive Mixed Models (GAMMs) provided a flexible statistical tool to study cumulative adaptation as participants were exposed to each of these patterns.
For native Spanish speakers, we found the data to be qualitatively consistent with an inverse preference effect: native speakers significantly adapted to manner verbs, which constitute the less frequent lexicalization pattern in Spanish, but they did not significantly adapt to path verbs, which is the more frequent lexicalization pattern in Spanish. While the current analysis does not allow us to test the significance of this interaction, and thus does not provide a conclusive verdict, it suggests a similar picture to that found in the syntactic domain, where native speakers have shown inverse preference effects (e.g., Bernolet & Hartsuiker, Reference Bernolet and Hartsuiker2010; Cho-Reyes et al., Reference Cho-Reyes, Mack and Thompson2016; Jaeger & Snider, Reference Jaeger and Snider2013; Peter et al., Reference Peter, Chang, Pine, Blything and Rowland2015; Scheepers, Reference Scheepers2003; Segaert et al., Reference Segaert, Weber, Cladder-Micus and Hagoort2014). For L2 learners as a group, we asked whether they would adapt as a function of their combined L1 and L2 experience (as in Kaan & Chun, Reference Kaan and Chun2017) or their L2-specific experience only (as in Flett et al., Reference Flett, Branigan and Pickering2013). The results suggest that learner adaptation was determined by a mixture of L1 and L2 experience: learners adapted to both path-verb exposure and manner-verb exposure with approximately equal strength. Overall, this is in line with the results in Kaan and Chun (Reference Kaan and Chun2017), who found evidence of transfer of L1-based expectations in L2 production at intermediate proficiency levels.
Most importantly, our final analysis addressed the question of how learners’ adaptation to input – and thus the answer to our second question – changed with increasing L2 proficiency. L2 proficiency had an opposite effect on adaptation depending on the primed lexicalization pattern: higher proficiency led to decreasing adaptation to path verbs, but to increasing adaptation to manner verbs. In other words, learners at lower proficiency levels behaved as we would expect if they were transferring their L1 Swedish expectations, similar to the intermediate proficiency learners in Kaan and Chun (Reference Kaan and Chun2017). However, with growing proficiency, learners increasingly behaved like native Spanish speakers, similar to the advanced learners in Flett et al. (Reference Flett, Branigan and Pickering2013). This change in patterns of adaptation supports the conclusion that learners’ expectations increasingly become attuned to the typical lexicalization patterns of the L2 and rely less on L1 experience with growing proficiency, reconciling the different findings in Flett et al. (Reference Flett, Branigan and Pickering2013) and Kaan and Chun (Reference Kaan and Chun2017).
In the remainder of the discussion, we first highlight how implicit learning during language production provides a theoretical account of the current results. We then elaborate on how this learning mechanism bears on questions about transfer in L2 production. Finally, we discuss how a theoretical focus on error signals during L2 language processing and learning might open up new perspectives on the acquisition of L2 properties that are unexpected under the learner's L1.
Implicit learning as an explanation of the current results
What mechanism might explain the current results? The inverse preference effect during language production is generally attributed to implicit learning (Chang et al., Reference Chang, Dell and Bock2006; Chang, Dell, Bock & Griffin, Reference Chang, Dell, Bock and Griffin2000; Dell & Chang, Reference Dell and Chang2014; Jaeger, Bushong & Burchill, Reference Jaeger, Bushong and Burchillsubmitted; Jaeger & Snider, Reference Jaeger and Snider2013; Reitter et al., Reference Reitter, Keller and Moore2011). Three broad classes of learning accounts have been proposed. All three types of accounts more or less directly link priming effects – e.g., the sentence-to-sentence change in the probability with which a speaker will produce a certain structure – to changes in the relative expectedness of the structure. The inverse preference effect then falls out of the assumption of life-long implicit learning during language processing (Elman, Bates, Johnson, Karmiloff-Smith, Parisi & Plunkett, Reference Elman, Bates, Johnson, Karmiloff-Smith, Parisi and Plunkett1996; Plaut, McClelland, Seidenberg & Patterson, Reference Plaut, McClelland, Seidenberg and Patterson1996).
For example, Reitter et al. (Reference Reitter, Keller and Moore2011) present a model of syntactic priming within the ACT-R framework (Anderson, Bothell, Byrne, Douglass, Lebiere & Qin, Reference Anderson, Bothell, Byrne, Douglass, Lebiere and Qin2004). In this model, the inverse preference effect falls out of implicit base level learning: each time a structure is retrieved from memory – be it in production or comprehension – the representations of that structure receive a boost of activation (an assumption shared by most models of production). This boost in activation constitutes the priming effect: when speakers choose a structure to produce, they are assumed to essentially sample from the available structures proportional to their relative activation, so that a more highly activated structure is more likely to be selected again (see also Dubey, Keller & Sturt, Reference Dubey, Keller and Sturt2008). This assumption, too, is broadly shared among models of production (see, e.g., Dell, Reference Dell1986; Dell & Chang, Reference Dell and Chang2014; Ferreira, Reference Ferreira1996; Segaert, Wheeldon & Hagoort, Reference Segaert, Wheeldon and Hagoort2016; Stallings, MacDonald & O'Seaghdha, Reference Stallings, MacDonald and O'Seaghdha1998). Activation is assumed to exhibit power law decay over time (an assumption shared with many memory models). Since a power law never fully returns to zero, this means that the base level activation of a structure slowly increases over time as a function of its previous mentions. The inverse preference effect then follows because the boost in activation associated with each retrieval of a structure is assumed to be constant, so that it will be proportionally smaller for a structure that already has higher base level activation. This account leaves open why activation decays as a power law, so that the inverse preference effect emerges.
An alternative implicit learning account explicitly evokes the notion of prediction errors (Chang et al., Reference Chang, Dell and Bock2006, Reference Chang, Dell, Bock and Griffin2000; Chang, Janciauskas & Fitz, Reference Chang, Janciauskas and Fitz2012). Such error-based learning accounts build on constraint-based, connectionist and neural network approaches to language processing that were originally proposed to explain language acquisition from general learning mechanisms (for references and review, see Dell & Chang, Reference Dell and Chang2014; MacDonald, Reference MacDonald2013). As in the ACT-R framework, the selection of word sequences – and thus structures – during production is co-determined by the relative activation of the words in the current context. Implicit error-based learning takes place during comprehension: each time a word is observed, its relative (un)expectedness in the current context constitutes the prediction error, and this prediction error is used to adjust the weights of the network so as to increase the expectedness of the observed word – and lower the expectedness of all other words – for the next time the same context is observed (through back-propagation, Rumelhart, Hinton & Williams, Reference Rumelhart, Hinton and Williams1986). The inverse preference effect is thus a direct consequence of the learning mechanisms, which explicitly refers to the prediction error. This particular framing is often evoked in recent research on L2 processing and learning (see, e.g., Jackson & Ruf, Reference Jackson and Ruf2017; Kaan, Reference Kaan2014; Kaan & Chun, Reference Kaan and Chun2017, Reference Kaan, Chun, Federmeier and Watson2018).
The third type of learning account derives this sensitivity to the prediction error from general computational considerations about optimal information integration (Fine, Qian, Jaeger & Jacobs, Reference Fine, Qian, Jaeger and Jacobs2010; Jaeger & Snider, Reference Jaeger and Snider2013; Kleinschmidt, Fine & Jaeger, Reference Kleinschmidt, Fine and Jaeger2012; Myslín & Levy, Reference Myslín and Levy2016). Bayesian belief-updating describes how new information is optimally integrated into prior beliefs about the statistics of the input. Although such belief-updating does not reference prediction errors directly, it can be shown that the inverse preference effects – larger changes in expectations after the observation of less expected structures – follows optimal information integration during learning (specifically for syntactic priming and expectation adaptation, see Jaeger et al., Reference Jaeger, Bushong and Burchillsubmitted).
These three accounts are thus not necessarily in conflict, but rather provide subtly different perspectives on implicit learning during language processing. All three accounts share that the inverse preference effect reflects changes in implicit expectations. The present results suggest that, for L2 learners, these expectations are initially influenced by learners’ overall language experience (including, notably, their L1 experience), but the expectations become more attuned to the statistics of the L2 as learners accumulate enough experience in the L2 (e.g., Flett et al., Reference Flett, Branigan and Pickering2013; Leal et al., Reference Leal, Slabakova and Farmer2016; Treffers-Daller & Calude, Reference Treffers-Daller and Calude2015).
Continuous implicit learning qualitatively explains why, in the present study, Spanish native speakers and Swedish learners of Spanish adapted to recently encountered input the way they did: based on their overall linguistic experience, they were expecting different types of lexicalization patterns, which led to different prediction errors and, in turn, to different patterns of adaptation. If adaptation to input also leads to implicit long-term learning (as proposed in, e.g., Chang et al., Reference Chang, Dell and Bock2006; Dell & Chang, Reference Dell and Chang2014; Ellis, O'Donnell & Römer, Reference Ellis, O'Donnell and Römer2013; Pajak et al., Reference Pajak, Fine, Kleinschmidt and Jaeger2016; Reitter et al., Reference Reitter, Keller and Moore2011), the same mechanism also would explain why L2 learners in our study showed different patterns of adaptation with increasing proficiency: L2 proficiency arguably reflects learners’ experience with Spanish; therefore, more proficient learners will have encountered the typical Spanish lexicalization pattern more often, and they will have adapted their L2 expectations correspondingly.
To our knowledge, the present study is the first to provide evidence from changes in L2 adaptation in production that are predicted under implicit learning accounts. Previous studies have either dealt with L1 speakers only (e.g., Arai & Mazuka, Reference Arai and Mazuka2014; Chang et al., Reference Chang, Dell and Bock2006; Jaeger & Snider, Reference Jaeger and Snider2013; Reitter et al., Reference Reitter, Keller and Moore2011) or only with inverse preference effects in L2 speakers at the group level but without considering changes in expectations with increasing L2 proficiency (Flett et al., Reference Flett, Branigan and Pickering2013; Jackson & Ruf, Reference Jackson and Ruf2017; Kaan & Chun, Reference Kaan and Chun2017). We now elaborate on how the evidence presented here informs notions of transfer in L2 learning.
Transfer of L1 expectations in L2 production
The present study emphasizes the idea that learners may transfer L1 expectations. Accounts of L1 transfer in second language acquisition (SLA) have tended to focus on whether learners produce L1 structures in L2 speech (e.g., Jarvis, Reference Jarvis2000; Jarvis & Pavlenko, Reference Jarvis and Pavlenko2008; Selinker, Reference Selinker1969). A shortcoming of conceiving transfer as equivalent to overt transfer of L1 structures in L2 production is that learners might not show overt transfer simply because they lack sufficiently rapid access to the necessary lexical material in the L2, as might have happened in the current study with the learners in the baseline condition. If the presence of transfer is rejected solely on the basis that L1 structures were not produced, we will neglect cases in which L2 learners latently transfer their expectations from their L1, even though this transfer may never become overt in production because of limited lexical availability. Investigating, as in the present study, how recent experience shapes production in learners offers a window into learners’ expectations that may not become manifest in spontaneous production tasks.
The notion of transfer of expectations is, however, not new. In his competition model, for instance, MacWhinney (Reference MacWhinney, De Groot and Kroll1997, Reference MacWhinney, Robinson and Ellis2008) frames language learning partly as a process of acquiring a certain set of cues that can vary with regard to availability and reliability. For example, different languages may rely on different cues to identify agenthood, such as word order, verb agreement, case marking, etc. Although L2 learners may initially weigh cues by transferring their L1-based expectations, and even fail to perceive cues that are not available in their L1 (Ellis, Reference Ellis2006), increasing exposure seems to slowly adjust cue weights towards the target language distribution (Dussias & Sagarra, Reference Dussias and Sagarra2007). The current study contributes to those previous approaches by emphasizing more explicitly the link between, on the one hand, a speaker's current knowledge and, on the other, real-time adaptation in production to recently encountered linguistic input. A key construct is the notion of prediction error (Chang et al., Reference Chang, Dell and Bock2006; Dell & Chang, Reference Dell and Chang2014; Jaeger & Snider, Reference Jaeger and Snider2013), on which we elaborate next.
Prediction error and L2 acquisition
To illustrate how the notion of prediction error might inform the process of L2 acquisition, consider the predictions of some classical transfer accounts for the learner group in our baseline condition (who were not primed). Recall that for Swedish learners of L2 Spanish to acquire the Spanish lexicalization preferences, learners have to overcome a strong L1-based preference for manner verbs, despite L2 Spanish input that also includes instances of manner verbs (Montero-Melis, Reference Montero-Melisunder review; Montero-Melis & Bylund, Reference Montero-Melis and Bylund2016). Since Swedish learners’ L1-based strategy (use of manner verbs) is in fact an attested strategy in the target L2 (Spanish), some influential transfer accounts in SLA predict that Swedish learners will transfer their L1-based strategy to L2 Spanish, predominantly using manner verbs in Spanish. For example, Andersen's (Reference Andersen, Gass and Selinker1983) transfer-to-somewhere principle states that a grammatical form will be transferred “if and only if there already exists within the L2 input the potential for (mis-) generalization” (Andersen, Reference Andersen, Gass and Selinker1983, p. 183, emphasis in original). Such a “potential for misgeneralization” certainly exists for Swedish learners of Spanish, and so the prediction would be that they should transfer the use of manner verbs. Similarly, Toribio's (Reference Toribio2004) convergence-as-optimization-strategy account predicts that learners will strive towards economy in their representations; thus, if a single lexicalization strategy (here, the use of manner verbs) can do in both languages (L1 Swedish and L2 Spanish), this should be the adopted strategy. But these predictions are in conflict with our findings: Swedish learners of L2 Spanish did not produce many manner verbs in the baseline condition at all.
The implicit learning mechanism outlined above suggests a different perspective on how differences between the L1 and L2 may affect learning. At least in situations where L2 learners are able to process the relevant L2 input, input that is highly unexpected given L1-based expectations (e.g., path verbs for Swedish learners of L2 Spanish) may lead to a large prediction error that prompts a strong revision of those initial expectations. L2 learners could then potentially even temporarily over-generalise away from their L1 (contra, e.g., the tranfer-to-somewhere principle [Andersen, Reference Andersen, Gass and Selinker1983]). That is, strong expectations against a linguistic structure in the target language could lead to increased adaptation towards that structure if a large prediction error is experienced.
Evidence compatible with this view comes from a recent neuroimaging study that investigated the initial stages of (artificial) language learning (Weber, Christiansen, Petersson, Indefrey & Hagoort, Reference Weber, Christiansen, Petersson, Indefrey and Hagoort2016). Weber and colleagues found that processing repeated (primed) syntactic structures in the novel language had opposite effects depending on whether the syntactic structure was familiar from the participants’ L1: if the structure was known based on the L1, activation in brain areas related to syntactic processing was suppressed, but if the structure was unknown, activation in the same areas was enhanced. Repetition suppression for familiar structures was indeed expected under a predictive coding and similar accounts (e.g., Friston, Reference Friston2005) in which repeated processing of known structures results in smaller prediction errors and reduced neural activation. Repetition enhancement, on the other hand, was expected when building new representations (Weber et al., Reference Weber, Christiansen, Petersson, Indefrey and Hagoort2016).
In the context of L2 learning, this then raises the question as to why L2 learners sometimes fail to learn structures that differ from their L1 (e.g., Best, McRoberts & Goodell, Reference Best, McRoberts and Goodell2001; see Ellis, Reference Ellis2006, for a more general discussion). One possibility is that prediction errors and L2 learning interact: when differences between the L1 and L2 are successfully processed, they will lead to prediction errors, which in turn should lead to adaptation and learning; in the absence of error signals, however, no learning should take place. The lack of an error signal may be due, for example, to some L2 properties being either so difficult or different that they are not processed (see Hofmeister, Jaeger, Arnon, Sag & Snider, Reference Hofmeister, Jaeger, Arnon, Sag and Snider2013; Phillips & Ehrenhofer, Reference Phillips and Ehrenhofer2015 for recent discussions), or because earlier learned L1 cues block attention to relevant properties of the L2 input (Ellis & Sagarra, Reference Ellis and Sagarra2010). The above argument suggests that the notion of error signal has the potential to inform theoretical models of L2 acquisition by establishing a quantitative link between the mismatch of expectations with respect to encountered input and the degree of adaptation to that input, with adaptation eventually leading to learning (Chang et al., Reference Chang, Dell and Bock2006, Reference Chang, Janciauskas and Fitz2012; Kaan, Reference Kaan2014; Kaan & Chun, Reference Kaan, Chun, Federmeier and Watson2018; Pajak et al., Reference Pajak, Fine, Kleinschmidt and Jaeger2016).
Limitations and future directions
The present study employed a between-subject priming manipulation to study adaptation to different lexicalization patterns. Using GAMM analyses, we found non-linear adaptation effects that accumulated over the course of our experiment. We also found that the patterns of adaptation qualitatively support implicit learning accounts that link moment-to-moment changes in production preferences to prediction errors experienced when processing linguistic input, and the changes in expectations that this triggers. However, we were unable to assess the statistical significance of the interactions predicted by these accounts (e.g., for Question 1). The post-hoc analyses presented in Appendix S4–S5 in Supplementary Materials had to be limited to subsets of our data, substantially reducing their power.
It is possible that the results on the native Spanish speakers (Question 1) remain inconclusive for this reason. As pointed out by an anonymous reviewer, future studies might aim to increase power for this question through more participants or within-subject designs. The latter might be preferable, in particular, for questions regarding L2 speakers, which are often difficult to recruit in large numbers. Our most significant finding though – changing patterns of adaptation with increasing L2 proficiency – is necessarily dependent on a between-subjects design or longitudinal designs. Here, we hope for future replications that target recruitment of participants with maximally different proficiency levels. The necessary sample size to achieve enough power could be estimated based on the current data, which is publicly shared at https://doi.org/10.7910/DVN/TOJ1UH (Dataverse repository). As pointed out by an anonymous reviewer, a stronger test of the hypothesis investigated here would compare priming across two different types of structures, one of which differs between participants’ L1 and their L2 (as is the case in the present study) and one of which doesn't. We would only expect a change in adaptation patterns for the former.
Conclusion
We have argued that recent work on the role of expectations in native processing and learning can shed light on how L2 learners integrate recent experience into their subsequent encoding choices. Our data are well explained by accounts predicting that speakers will adapt to recently experienced input as a function of the perceived prediction error, which itself is a function of the divergence of their expectations and the actual input (e.g., Chang et al., Reference Chang, Dell and Bock2006; Dell & Chang, Reference Dell and Chang2014; Jaeger & Snider, Reference Jaeger and Snider2013; Malhotra, Reference Malhotra2009; Reitter et al., Reference Reitter, Keller and Moore2011). Moreover, learners’ expectations progressively come to resemble those of native speakers with increasing L2 proficiency, leading to more native-like patterns of adaptation. Spelling out the factors that modulate the perception of prediction errors in learners remains a major task for research that aims at casting L2 acquisition as error-based learning and will contribute to recent efforts to bridge theoretical accounts of language processing developed in the L1 literature with the field of L2 acquisition (e.g., Kaan, Reference Kaan2014; Phillips & Ehrenhofer, Reference Phillips and Ehrenhofer2015).
Supplementary Material
For supplementary material accompanying this paper, visit https://doi.org/10.1017/S1366728919000506
Acknowledgements
We thank Emanuel Bylund, colleagues at the Multilingualism Lab (Centre for Research on Bilingualism, Stockholm University), and the audience at EuroSLA 27 in Reading for insightful comments on previous versions of this manuscript. We thank Kayle Sneed for precious help with data transcription and coding. We also thank Paul-Christian Bürkner and Simon Wood for answering our questions about current implementations of Generalized Additive Mixed Models and non-linear smooths in R.