Introduction
In today's multilingual and emotionally charged society (Dewaele, Reference Dewaele2010; Grosjean, Reference Grosjean2010; Pavlenko, Reference Pavlenko2014), it has become important to study how bilinguals communicate emotional content in the first (L1) and second (L2) language. Accumulating evidence points to bilinguals’ reduced sensitivity to emotional content presented in their L2 (Caldwell-Harris, Reference Caldwell-Harris2014; Pavlenko, Reference Pavlenko2012), possibly due to delayed acquisition of emotional concepts in L2 and relatedly reduced embodiment (Jończyk, Reference Jończyk2016; Pavlenko, Reference Pavlenko2012; Sheikh & Titone, Reference Sheikh and Titone2015; Wu & Thierry, Reference Wu and Thierry2012). Bilingualism research conducted thus far in this field has focused on investigating comprehension of emotional words and little attention has been devoted to production, which is arguably as important. Likewise, little is known about how socio-communicative cues (e.g., facial expressions or emojis) modulate word production in bilinguals’ two languages. To address these questions, we studied the brain dynamics of negative and neutral single word production in Polish (L1) – English (L2) bilinguals in two electrophysiological (EEG) experiments, in which words were embedded either in a neutral context (i.e., preceded by black or white circles cueing the task; Experiment 1), or in an emotional context (i.e., preceded by sad or neutral emojis also cueing the task and priming the upcoming word; Experiment 2).
Previous bilingualism research has pointed to decreased sensitivity to negative content when processing L2. This effect has been linked, among others, to differences in L1 and L2 age of acquisition, learning context, and/or proficiency level. Namely, bilinguals who acquired their L2 later in life, or in a formal rather than a natural setting, or bilinguals who achieved lower L2 proficiency, tend to experience emotional detachment in L2 (Caldwell-Harris, Reference Caldwell-Harris2014; Imbault et al., Reference Imbault, Titone, Warriner and Kuperman2020; Pavlenko, Reference Pavlenko2012). First documented in self-report and behavioural studies (Dewaele & Pavlenko, Reference Dewaele and Pavlenko2002; Pavlenko, Reference Pavlenko2006; Puntoni et al., Reference Puntoni, de Langhe and van Osselaer2009; Sutton et al., Reference Sutton, Altarriba, Gianico and Basnight-Brown2007), the emotional disembodiment hypothesis has received substantial neurophysiological support. For instance, decreased physiological arousal in response to L2 relative to L1 has been found in electrodermal and/or pupillometric research on childhood reprimands and taboo words, as well as emotional words, phrases, sentences, and narratives (Baumeister et al., Reference Baumeister, Foroni, Conrad, Rumiati and Winkielman2017; Eilola & Havelka, Reference Eilola and Havelka2010; Eilola et al., Reference Eilola, Havelka and Sharma2007; Harris, Reference Harris2004; Iacozza et al., Reference Iacozza, Costa and Duñabeitia2017; Jankowiak & Korpal, Reference Jankowiak and Korpal2018; Toivo & Scheepers, Reference Toivo and Scheepers2019), marking an attenuated activation of the autonomic nervous system when participants are exposed to L2 as compared to L1 content.
EEG research on emotional language processing, on the other hand, remains scant and less consistent. Initial studies found that reading emotional words in L1 and L2 leads to comparable modulations of the Early Posterior Negativity (EPN) or Late Positive Potential (LPP) – two event-related potential (ERP) components reflecting early and late processing of emotional information, respectively (Conrad et al., Reference Conrad, Recio and Jacobs2011; Opitz & Degner, Reference Opitz and Degner2012). These findings suggest that emotional words capture the brain's attention in both L1 and L2, albeit with a slight delay in L2. In another study, Chen et al. (Reference Chen, Lin, Chen, Lu and Guo2015) found increased EPN and decreased LPP amplitudes for positive as compared to neutral words in L1 only, suggesting more automatic processing of positive and neutral information in L1 at an early processing stage.
In contrast, studies where emotional words are embedded in a minimal semantic context have shown attenuated N400 amplitudes for negative words in L2 compared to L1 (Jończyk et al., Reference Jończyk, Boutonnet, Musiał, Hoemann and Thierry2016; Wu & Thierry, Reference Wu and Thierry2012; Zhang et al., Reference Zhang, Jończyk, Wu, Lan, Gao, Hu, Thierry and Gao2023). Because the N400 indexes cognitive effort associated with semantic processing (Kutas & Federmeier, Reference Kutas and Federmeier2011), the observed N400 attenuation was interpreted as pointing to shallower processing of negative meaning in L2 than L1. For instance, Wu and Thierry (Reference Wu and Thierry2012) showed that emotional valence filters access to native language representations in bilinguals such that negative words temporarily and unconsciously shut down access to native translation equivalents, whereas neutral and positive words do not. They showed this by manipulating the valence of the first word in a pair (prime) in an experiment concealing character repetition in Chinese–English bilinguals (as in Thierry & Wu, Reference Thierry and Wu2007). When prime words had a neutral or positive valence, ERPs were modulated by character repetition in (unseen) Chinese translations indicating access to Chinese. When prime words had a negative valence, however, such modulation was not observed (i.e., character repetition priming was not detected), suggesting that access to Chinese representations had been shut down. Zhang et al. (Reference Zhang, Jończyk, Wu, Lan, Gao, Hu, Thierry and Gao2023) recently replicated Wu and Thierry's result and further manipulated the position of the word carrying emotional valence. When valence was carried by the second word of a pair (target), they observed the expected modulation of brain activity by Chinese character repetition in all cases. The logical conclusion is that Chinese translation equivalents are accessed automatically irrespective of valence but an L2 negative word will induce inhibition of L1 access with a delay, providing a mechanism for the reduction of sensitivity to negative content in L2.
A recent study extended this effect to a pre-stimulus stage by showing decreased brain activity when participants anticipated negative information in L2 only (Jończyk et al., Reference Jończyk, Korolczuk, Balatsou and Thierry2019). These results are consistent with electrodermal and pupillometry studies (e.g., Baumeister et al., Reference Baumeister, Foroni, Conrad, Rumiati and Winkielman2017; Iacozza et al., Reference Iacozza, Costa and Duñabeitia2017; Jankowiak & Korpal, Reference Jankowiak and Korpal2018; Thoma et al., Reference Thoma, Hüsam and Wielscher2022; Toivo & Scheepers, Reference Toivo and Scheepers2019). Altogether, accumulating evidence from EEG and pupillometry suggests that communicating in L2 dampens emotional responses, in particular when bilinguals read or hear negative content embedded in a minimal semantic context that mimics natural language.
Relatively little is known about behavioural and neurophysiological correlates of emotional language production in bilinguals. Until recently, a direct neurophysiological investigation of language production was avoided due to speech-related artifacts such as muscle and mouth movements during overt speech (see Hinojosa et al., Reference Hinojosa, Méndez-Bértolo, Carretié and Pozo2010). Recent advancements in EEG signal processing have allowed to isolate and correct for articulation-related artifacts, making it possible to test overt language production (Ouyang et al., Reference Ouyang, Sommer, Zhou, Aristei, Pinkpank and Rahman2016; Porcaro et al., Reference Porcaro, Medaglia and Krott2015; Vos et al., Reference Vos, Riès, Vanderperren, Vanrumste, Alario, Huffel and Burle2010). For example, Rohr and Rahman (Reference Rohr and Rahman2018) asked German (L1) – English (L2) bilinguals to translate (aloud) visually presented positive, negative, or neutral words from L2 to L1 or to read them aloud. LPP amplitudes increased for negative relative to neutral words in both the production and reading tasks. The LPP effect was interpreted as indexing participants’ anticipation of the effect that producing a negative word may have in a social communicative interaction. Here, building on Rohr and Rahman's (Reference Rohr and Rahman2018) study, we investigate how emotional word content modulates language production in a bilingual's two languages.
Electrophysiological responses to emotional language content are further modulated by socio-communicative cues (e.g., faces, emojis) that accompany linguistic cues. In a study staging face-to-face interactions, Rohr and Rahman (Reference Rohr and Rahman2015) found early (50–100 ms) and long-lasting amplified EEG responses to emotionally valenced words presented in a communicative (i.e., video presentation of a person's face with open eyes and mouth) as compared to a non-communicative (i.e., closed eyes and mouth) context. Also, Schindler and Kissler (Reference Schindler and Kissler2016; see also Schindler et al., Reference Schindler, Vormbrock and Kissler2019) found early (P2, EPN, and P3) and late (LPP) ERP modulations when participants viewed emotional trait adjectives recognized as coming from a human sender vs. a computer. Here, we test whether such modulation can be observed when emotionally valenced words appear in a minimal emotional context introduced by emojis (sad/neutral).
The present study thus investigated the brain dynamics of emotional word production in Polish (L1) – English (L2) bilinguals. Speech production was elicited in an overt translation task and a control shadowing task (i.e., reading words aloud; Christofffels & Groot, Reference Christofffels and Groot2004; Christoffels et al., Reference Christoffels, Ganushchak and Koester2013; Jost et al., Reference Jost, Radman, Buetler and Annoni2018; Rohr & Rahman, Reference Rohr and Rahman2018). Unlike Rohr and Rahman (Reference Rohr and Rahman2018), we implemented a bi-directional translation task (i.e., L1–L2 and L2–L1) to evaluate how emotional word content affects both L1 and L2 word production (Jost et al., Reference Jost, Radman, Buetler and Annoni2018). Extending previous EEG, pupillometry, and electrodermal studies of emotional word comprehension (Baumeister et al., Reference Baumeister, Foroni, Conrad, Rumiati and Winkielman2017; Jankowiak & Korpal, Reference Jankowiak and Korpal2018; Jończyk et al., Reference Jończyk, Korolczuk, Balatsou and Thierry2019; Rohr & Rahman, Reference Rohr and Rahman2018; Toivo & Scheepers, Reference Toivo and Scheepers2019; Wu & Thierry, Reference Wu and Thierry2012), we also analysed potential contextual effects of emotional cues (Rohr & Rahman, Reference Rohr and Rahman2015; Schindler & Kissler, Reference Schindler and Kissler2016; Schindler et al., Reference Schindler, Vormbrock and Kissler2019). In Experiment 1 (neutral context), each word was preceded by a white or a black circle to indicate whether participants would be translating or reading the word aloud. In Experiment 2 (emotional context), words were preceded by white or black emojis, neutral or sad, indicating the nature of the task (translation vs. shadowing) and, simultaneously, announcing the emotional valence of the upcoming word. We predicted increased EPN amplitudes for negative compared to neutral words in both experiments (Kissler & Bromberek-Dyzman, Reference Kissler and Bromberek-Dyzman2021; Kunkel et al., Reference Kunkel, Filik, Mackenzie and Leuthold2018; Rohr & Rahman, Reference Rohr and Rahman2018), reflecting early attention allocation to emotional stimuli. Further, we predicted increased N400 amplitudes when participants were readying to translate words from L1 to L2, indexing greater cognitive effort to suppress L1 representations (Green, Reference Green1998; Jost et al., Reference Jost, Radman, Buetler and Annoni2018). N400 amplitudes were also expected to decrease in amplitude for negative relative to neutral words in L2 only, in line with prior EEG evidence showing attenuated response to negative words in L2 (Jończyk et al., Reference Jończyk, Boutonnet, Musiał, Hoemann and Thierry2016, Reference Jończyk, Korolczuk, Balatsou and Thierry2019; Wu & Thierry, Reference Wu and Thierry2012; Zhang et al., Reference Zhang, Jończyk, Wu, Lan, Gao, Hu, Thierry and Gao2023). Critically, if bilinguals show reduced sensitivity to emotional content in L2 during speech planning, then LPP amplitudes should increase when bilinguals translate negative words from L2 to L1 (i.e., when they produce a word in Polish) or when they read aloud L1 as compared to L2 words (cf., Rohr & Rahman, Reference Rohr and Rahman2018). We further predicted that this effect would be stronger in an emotional (Experiment 2) than a neutral (Experiment 1) context.
Experiment 1: Neutral context
Participants
Thirty-five participants took part in the experiment, following random assignment; nine participants were excluded from the analyses due to low quality of the recorded EEG signal. The final sample thus consisted of 26 Polish–English (L1–L2) bilinguals (22 females, 4 males) aged 22–32 (MAge = 24.42; 95% CI [23.40, 25.44])Footnote 1. Consistent with de Groot (Reference de Groot2011), participants were classified as highly proficient unbalanced Polish–English bilinguals who had not lived in the L2 (English) environment and had acquired their L2 in an instructional yet immersive learning context. They were engaged in an intensive English-only curriculum (the C2 level of the Common European Framework of Reference, CEFR) at the Faculty of English, Adam Mickiewicz University in Poznań, Poland (see Table 1). Participants reported no language, neurological, or psychiatric disorders, had normal or corrected-to-normal vision, and were not taking any psychoactive drugs around the time of data collection (see Table 2 for details on participants’ bio-demographic data). For their participation, they received a gift card of 200 PLN.
Table 1. Experiment 1: Participants sociolinguistic data (means with 95% confidence intervals)
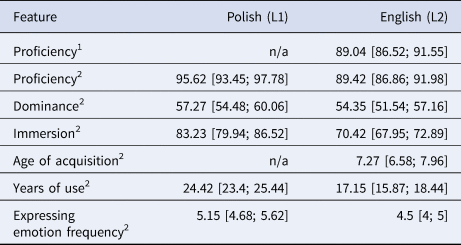
Measurements and ranges: 1LexTALE (Lemhöfer & Broersma, Reference Lemhöfer and Broersma2011): the B1 (0–59%), B2 (60–80%), C1 and C2 (80–100%) CEFR levels; 2Language History Questionnaire 3.0 (LHQ; Li et al., Reference Li, Zhang, Yu and Zhao2019, as translated into Polish by Naranowicz and Witczak): proficiency, dominance, and immersion scores [%], age of acquisition and years of use [years], and expressing emotion frequency (1 – never, 7 – always).
Table 2. Experiment 1: Participants bio-demographic data (means with 95% confidence intervals)
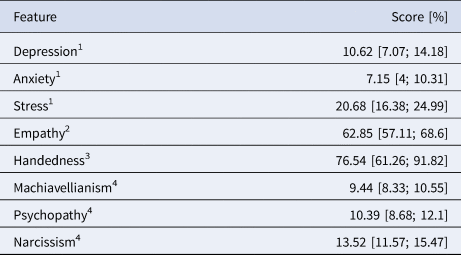
Measurements and ranges: 1DASS-21 (Lovibond and Lovibond, Reference Lovibond and Lovibond1995, as translated into Polish by Makara-Studzińska et al.): normal (0–21%), mild (22–31%), moderate (32–48%), severe (49–64%), and extremely severe (65–100%) levels of depression, anxiety, and stress; 2Empathy Quotient (Baron-Cohen & Wheelwright, Reference Baron-Cohen and Wheelwright2004, as translated into Polish by Wainaina-Woźna): low (0–39%), average (40–64%), above average (65–78%), and high (79–100%) levels of empathy; 3Edinburgh Handedness Inventory (Oldfield, Reference Oldfield1971): left-handedness (-100 – -28), ambidexterity (-29–48), and right-handedness (48–100); 4Polish adaptation of Dirty Dozen Scale (Czarna et al., Reference Czarna, Jonason, Dufner and Kossowska2016): Machiavellianism, psychopathy, and narcissism levels (percentages).
Materials
The stimuli consisted of 144 Polish (L1) and 144 English (L2) words, with 72 negative and 72 neutral words in each language. Equal proportions of concrete and abstract words as well as nouns and adjectives were included. The stimuli were controlled for a number of other lexico-semantic variables, as described in detail in Table 3. Polish and English words were not translation equivalents. Taboo, swear, and polysemous words, Polish–English cognates and interlanguage homonyms and homographs were excluded from the stimuli.
Table 3. Word characteristics.
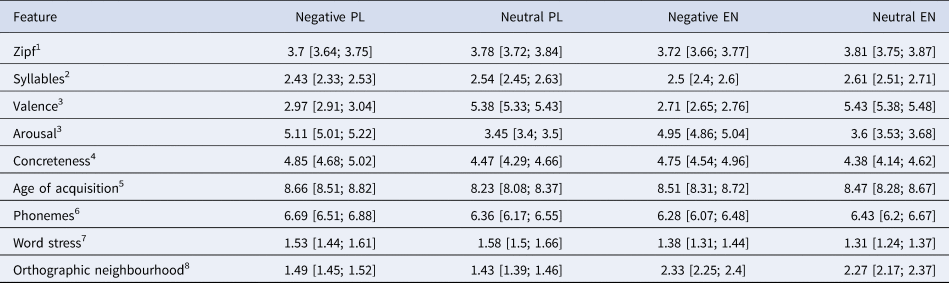
Measurements and ranges: 1Zipf: the 1–7 scale; the 2.7–5.1 range (i.e., medium frequency); databases: SUBTLEX-PL (Mandera et al., Reference Mandera, Keuleers, Wodniecka and Brysbaert2014); SUBTLEX-EN (van Heuven et al., Reference van Heuven, Mandera, Keuleers and Brysbaert2014). 2Syllables: the 1–5 range. 3Valence and arousal: the 1–9 scales; the 1.4–4.2 (valence) and 2.6–7.1 (arousal) ranges for negative words and the 4.5–6.4 (valence) and 2.3–5.4 (arousal) ranges for neutral words; databases: Imbir (Reference Imbir2016) for Polish, Warriner et al. (Reference Warriner, Kuperman and Brysbaert2013) for English. 4Concreteness: the 1–9 scale; the 4.7–7.4 range for the abstract words and the 1.0-4.8 range for the concrete words; databases: Imbir (Reference Imbir2016) for Polish, Brysbaert et al. (Reference Brysbaert, Warriner and Kuperman2013) for English. 5Age of acquisition: the 4.5–12.9 range; databases: Imbir (Reference Imbir2016) for Polish, Brysbaert et al. (Reference Brysbaert, Warriner and Kuperman2013) for English. 6Phonemes: the 3–11 range. 7Word stress: the 1–4 range. 8Orthographic neighborhood: calculated based on SUBTLEX-PL (Mandera et al., Reference Mandera, Keuleers, Wodniecka and Brysbaert2014) and SUBTLEX-EN (van Heuven et al., Reference van Heuven, Mandera, Keuleers and Brysbaert2014) using the vwr package for R (R Core Team, 2021).
Procedure
The procedures followed in the study were approved by the Ethics Committee for Research Involving Human Participants at Adam Mickiewicz University in Poznań, Poland (Resolution no. 7/2020/2021). Prior to data collection, participants were screened online by means of a Polish version of DASS-21 (Lovibond & Lovibond, Reference Lovibond and Lovibond1995), a medical history questionnaire, and LexTALE (Lemhöfer & Broersma, Reference Lemhöfer and Broersma2011).
The experiment was carried out in the Psychophysiology of Language and Affect (PoLA) laboratory (Faculty of English, Adam Mickiewicz University, Poznań, Poland). Participants were seated in a dimly lit and quiet booth, 75 cm away from an LED monitor with a screen resolution of 1280 x 1024 pixels. They completed Polish versions of the Empathy Quotient (Baron-Cohen & Wheelwright, Reference Baron-Cohen and Wheelwright2004), the Dirty Dozen (Czarna et al., Reference Czarna, Jonason, Dufner and Kossowska2016), the Edinburgh Handedness Inventory (Oldfield, Reference Oldfield1971) in privacy and the Language History Questionnaire 3.0 (Li et al., Reference Li, Zhang, Yu and Zhao2019) during the EEG cap preparation.
We used E-Prime 3.0 (Psychology Software Tools, Inc.) for stimulus presentation and ActiView (Biosemi B.V.) for EEG data acquisition. Participants completed one Polish (L1) and one English (L2) block in a counterbalanced order, each comprising 72 negative and 72 neutral randomly presented words (NTotal = 288). Participants were first presented with a visual cue – a black or white circle – followed by a word. The colour of the circle informed participants to either repeat (i.e., a shadowing task) or orally translate (i.e., a translation task) the subsequently presented word. Task colour coding was counterbalanced across participants. After every twenty trials, participants had a 7-second break to rest their eyes. Time sequence of stimuli presentation is presented in Supplementary Figure 1 (Supplementary Materials).
Behavioural data recording and analysis
The study conformed to a 2 (Language: Polish, English) × 2 (Valence: negative, neutral) × 2 (Task: translation, shadowing) within-subject design. Reaction time data were collected with a voice key using Chronos (Psychology Software Tools, Pittsburgh, USA). Data preprocessing was performed using the tidyverse library (Wickham et al., Reference Wickham, Averick, Bryan, Chang, McGowan, François, Grolemund, Hayes, Henry, Hester, Kuhn, Pedersen, Miller, Bache, Müller, Ooms, Robinson, Seidel, Spinu and Yutani2019) in R (R Core Team, 2021). Log-transformed reaction time data and task accuracy were analysed with linear mixed models (LMMs) and generalized linear mixed models (GLMMs), respectively, using the lmerTest package (Kuznetsova et al., Reference Kuznetsova, Brockhoff and Christensen2017). Tables with model summaries and interaction plots were computed with sjPlot package (Lüdecke, Reference Lüdecke2022). ERP plots were computed with ggplot2 (Wickham, Reference Wickham2016); ERP topographies were computed with eegUtils (Craddock, Reference Craddock2022).
For each (G)LMM, we computed a maximal model with a full random-effect structure, including subject- and item-related variance components for intercepts and by-subject and by-item random-slopes for fixed-effects (Barr et al., Reference Barr, Levy, Scheepers and Tily2013). All fixed effects were coded using sum contrast coding (-0.5; 0.5). If a maximal model did not converge and/or turned out to be too complex, we selected a parsimonious LMM following the recommendations from Bates et al. (Reference Bates, Kliegl, Vasishth and Baayen2018; see also Matuschek et al., Reference Matuschek, Kliegl, Vasishth, Baayen and Bates2017). Small variance parameters were removed using the lme4::rePCA and lme4::VarCorr (Bates et al., Reference Bates, Mächler, Bolker and Walker2015) functions until (G)LMMs were supported by the data. None of the models showed collinearity (Variance Inflection Factor < 1.5). The final structure of each model and model table summaries are available at https://doi.org/10.17605/OSF.IO/U6XQN.
Electrophysiological data recording and analysis
EEG data were acquired at 2048 Hz from 64 Ag/AgCl electrodes using a BioSemi ActiveTwo amplifier (BioSemi B.V., Amsterdam) and four peri-ocular electrodes (two vertical and two horizontal EOGs), placed according to the International 10–20 system. Preprocessing steps and analyses were performed using EEGLAB (v14.1.1; Delorme & Makeig, Reference Delorme and Makeig2004) and supplemented by Residue Iteration Decomposition (RIDE) (Ouyang et al., Reference Ouyang, Herzmann, Zhou and Sommer2011, Reference Ouyang, Sommer, Zhou, Aristei, Pinkpank and Rahman2016) toolbox in Matlab R2022a (The MathWorks, Inc.). Offline, continuous EEG data were downsampled to 500 Hz, high-pass filtered at 0.1 Hz and low-pass filtered at 30 Hz using the Hamming windowed sinc FIR filter (pop_eegfiltnew in EEGLAB). Unsystematic artifacts in continuous EEG data were manually detected and removed. Bad channels were identified based on the EEGLAB functions clean_rawdata (Mullen et al., Reference Mullen, Kothe, Chi, Ojeda, Kerth, Makeig, Jung and Cauwenberghs2015; correlation criterion = 0.8) and TrimOutlier (Lee & Miyakoshi; https://sccn.ucsd.edu/wiki/TrimOutlier) and supplemented by visual inspection (M rejected = 1.61, min = 0, max = 6). Next, data were re-referenced to the activity of all channels (global average reference) and epoched from -1 to 4 surrounding each trial (time-locked to word onset). Epochs were further pruned for non-stereotypical artifacts using the EEGLAB function pop_jointprob (removing absolute values exceeding 6 SDs at a single electrode and 3 SDs at all electrodes) and visual inspection (M rejected = 6.32%, min = 0.69%, max = 13.19%). Epochs were then subjected to Adaptive Mixture Independent Component Analysis (AMICA) (Palmer et al., Reference Palmer, Makeig, Kreutz-Delgado and Rao2008) implemented in EEGLAB. The resulting AMICA decomposition was inspected using IClabel (Pion-Tonachini et al., Reference Pion-Tonachini, Kreutz-Delgado and Makeig2019). Independent components containing ocular artifacts, bad channel activity and/or line noise were removed from the data (M rejected = 3.98, min = 1, max = 9).
Cleaned epochs were subjected to RIDE (Ouyang et al., Reference Ouyang, Herzmann, Zhou and Sommer2011). RIDE decomposes ERPs into several component clusters that show variability across trials. Typically, ERPs would be decomposed into a stimulus-locked (S), response-locked (R), and latency-variable (C) component clusters. RIDE has been shown to be effective in separating and rejecting articulation artifacts (Ouyang et al., Reference Ouyang, Sommer, Zhou, Aristei, Pinkpank and Rahman2016; Rohr & Rahman, Reference Rohr and Rahman2018). Here, we decomposed ERPs into the S-component (0–800 ms) and an R-component (-300–500 relative to speech onset trigger latency).
We focused on three ERP components: EPN, N400, and LPP. EPN was analysed over 10 electrodes (O1, PO3, P3, P5, P7, O2, PO4, P4, P6, and P8) in the 200–300 ms time window after word onset (Kissler et al., Reference Kissler, Herbert, Peyk and Junghofer2007, Reference Kissler, Herbert, Winkler and Junghofer2009). N400 was analysed over 9 electrodes (FC1, FC2, FCz, C1, C2, Cz, CP1, CP2, and CPz) between 300–500 ms after word onset (Jończyk et al., Reference Jończyk, Boutonnet, Musiał, Hoemann and Thierry2016, Reference Jończyk, Korolczuk, Balatsou and Thierry2019). LPP was analysed over 6 electrodes (C1, C2, Cz, CP1, CP2, and CPz) between 500–700 ms after word onset (Kissler & Bromberek-Dyzman, Reference Kissler and Bromberek-Dyzman2021; Kunkel et al., Reference Kunkel, Filik, Mackenzie and Leuthold2018; Rohr & Rahman, Reference Rohr and Rahman2018). Statistical analyses were conducted by means of repeated-measures ANOVAs with mean ERP amplitudes as dependent variables and Language (Polish, English), Valence (neutral, negative), and Task (shadowing, translation) as independent variables. A Greenhouse-Geisser correction was applied where applicable; p-values obtained from post-hoc comparisons were adjusted using the Bonferroni correction.
Results
Reaction times
A fixed effect of Task showed faster reaction times (RTs) in the shadowing (M = 714.14 ms, 95% CI [706.58, 721.71]) than translation (M = 1,017.17 ms, 95% CI [999.99, 1,034.35]) task, $\hat{\beta } = {-}0.13$, 95% CI [ − 0.15, − 0.12], t(32.94) = −15.02, p < .001. Task was further modulated by Language, $\hat{\beta } = {-}0.04$
, 95% CI [ − 0.06, − 0.02], t(31.18) = −3.45, p = .002, showing faster RTs when translating words from Polish (M = 988.36 ms, 95% CI [964.78, 1,011.94]) than from English (M = 1,047.45 ms, 95% CI [1,022.44, 1,072.45]), b = -0.03, z = -2.42, p = .015. Finally, Task was modulated by Valence, $\hat{\beta } = {-}0.02$
, 95% CI [ − 0.03, 0.00], t(8, 188.20) = −2.35, p = .019, with slower RTs to negative words in the translation (M = 1,028.35 ms, 95% CI [1,003.18, 1,053.53]) than shadowing (M = 710.96 ms, 95% CI [700.18, 721.74]) task, b = 0.13, z = 13.35, p < .001. In the same vein, RTs were slower when translating (M = 1,006.67 ms, 95% CI [983.18, 1,030.15]) than shadowing (M = 717.31 ms, 95% CI [706.68, 727.94]) neutral words, b = 0.14, z = 14.88, p < .001 (see Supplementary Figure 2, Supplementary Materials). We did not find other effects (ps > .1).
Accuracy
A fixed effect of Task showed higher accuracy in the shadowing (M = 97.92%, 95% CI [97.51, 98.32]) than translation (M = 83.10%, 95% CI [82.04, 84.17]) task, $\hat{\beta } = 2.62$, 95% CI [2.38, 2.86], z = 21.17, p < .001. Also, a fixed effect of Valence revealed higher accuracy for neutral (M = 92.09%, 95% CI [91.32, 92.86]) than negative (M = 88.93%, 95% CI [88.04, 89.82]) words, $\hat{\beta } = {-}0.55$
, 95% CI [ − 0.91, − 0.19], z = −3.01, p = .003 (see Supplementary Figure 3, Supplementary Materials). We did not find other effects (ps > .1).
Electrophysiological results
Early Posterior Negativity
EPN amplitudes increased for negative (M = 0.79 μV, 95% CI [0.76, 0.81]) as compared to neutral (M = 1.00 μV, 95% CI [0.98, 1.03]) words, F(1, 25) = 5.84, p = .023, $\hat{\eta }_G^2 = .004$, 90% CI [.000, .117] (see Supplementary Figure 4, Supplementary Materials). Also, EPN amplitude was larger in response to words in English (M = 0.74 μV, 95% CI [0.72, 0.77]) than Polish (M = 1.05 μV, 95% CI [1.02, 1.07]), F(1, 25) = 4.80, p = .038, $\hat{\eta }_G^2 = .008$
, 90% CI [.000, .143]. No other effects were found (ps > .1).
N400
N400 amplitudes increased for words in Polish (M = -0.98 μV, 95% CI [-1.00, -0.97]) as compared to words in English (M = -0.63 μV, 95% CI [-0.64, -0.62]), F(1, 25) = 8.63, p = .007, $\hat{\eta }_G^2 = .020$, 90% CI [.000, .182] and in the shadowing (M = -0.97 μV, 95% CI [-0.98, -0.96]) as compared to translation task (M = -0.64 μV, 95% CI [-0.66, -0.63]), F(1, 25) = 4.59, p = .042, $\hat{\eta }_G^2 = .018$
, 90% CI [.000, .175]. A Language-by-Valence interactionFootnote 2, F(1, 25) = 6.72, p = .016, $\hat{\eta }_G^2 = .003$
, 90% CI [.000, .107], showed larger N400 amplitudes for negative words in Polish (M = -1.06 μV, 95% CI [-1.08, -1.04]) than in English (M = -0.57 μV, 95% CI [-0.59, -0.56], b = 0.49, t = 3.42, p = .002). Further, in Polish only N400 amplitude increased for negative as compared to neutral words, b = -0.16, t = -2.21, p = .037 (see Figure 1). No other effects were found (ps > .1).
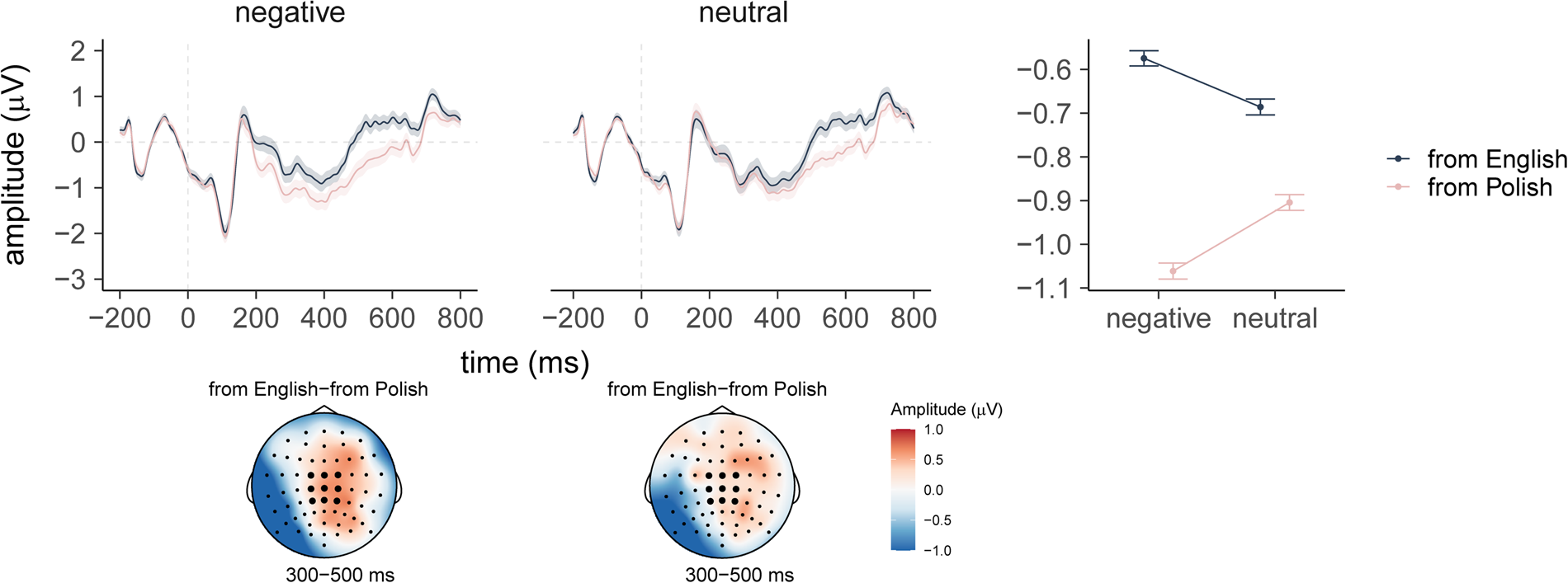
Figure 1. N400 elicited by negative and neutral words in Polish and English (Experiment 1). Waveforms illustrate brain potential variations computed via linear derivation from nine fronto-centro–parietal electrodes (FC1, FC2, FCz, C1, C2, Cz, CP1, CP2, and CPz). Time 0 coincides with word presentation onset. The topographic maps represent the difference in N400 amplitude between the conditions of interest in the 300–500 ms window. The line plot represents mean N400 amplitude averaged over the electrodes of interest in the 300–500 window. Shaded areas (ERP plot) and error bars (line plot) depict 95% confidence intervals (CI).
Late Posterior Positivity
LPP amplitudes were more positive for words presented in English (M = 0.83 μV, 95% CI [0.81, 0.84]) than in Polish (M = 0.27 μV, 95% CI [0.26, 0.29]), F(1, 25) = 18.46, p < .001, $\hat{\eta }_G^2 = .055$, 90% CI [.000, .247]. Language was further modulated by Task, F(1, 25) = 6.18, p = .020, $\hat{\eta }_G^2 = .007$
, 90% CI [.000, .136], showing greater LPP amplitudes when English words were to be translated (M = 1.07 μV, 95% CI [1.05, 1.09]) than read aloud (M = 0.58 μV, 95% CI = [0.57, 0.60]), b = -0.49, t = -2.66, p = .013; see Figure 2). No other effects were found (ps > .1).
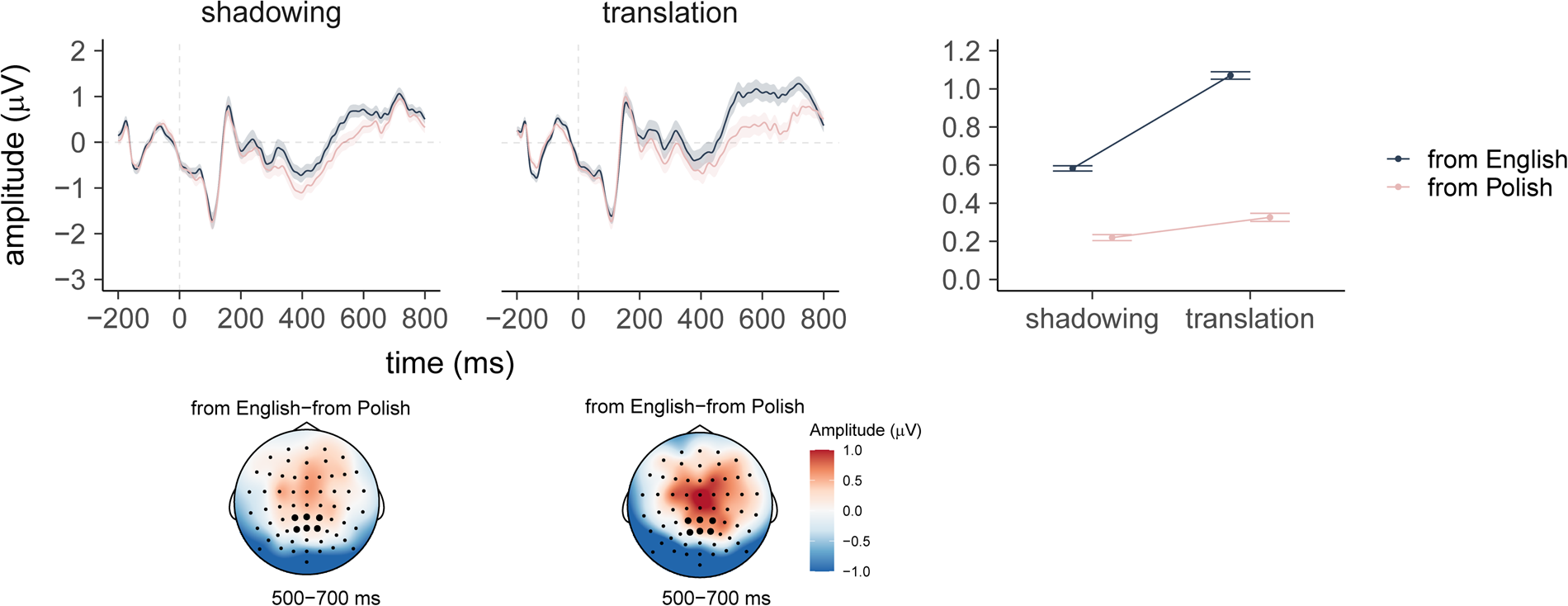
Figure 2. LPP elicited by words in Polish and English in the translation and shadowing task (Experiment 1). Waveforms illustrate brain potential variations computed via linear derivation from six centro–parietal electrodes (C1, C2, Cz, CP1, CP2, and CPz). Time 0 coincides with word presentation onset. Topographic maps represent the difference in LPP amplitudes between the conditions of interest in the 500–700 ms time window. The line plot represents mean LPP amplitudes averaged over the electrodes of interest in the 500–700 time window. Shaded areas (ERP plot) and error bars (line plot) depict 95% confidence intervals (CI).
Discussion
As expected, we found greater EPN amplitudes for negative than neutral words, reflecting early attention capture by emotional content (Kissler et al., Reference Kissler, Herbert, Peyk and Junghofer2007, 2009; Rohr & Rahman, Reference Rohr and Rahman2018). N400 amplitudes were larger for negative words presented in L1 than L2. This finding may initially seem surprising considering earlier reports of increased N400 amplitudes when fluent bilinguals comprehend or produce L2 (Martin et al., Reference Martin, Thierry, Kuipers, Boutonnet, Foucart and Costa2013; Thierry & Wu, Reference Thierry and Wu2007; Wu & Thierry, Reference Wu and Thierry2017). Increased N400 amplitudes should be expected given that semantic processing requires greater cognitive effort in L2. Here, we found reduced N400 amplitudes for negative words in L2 as compared to L1, irrespective of the task. This finding is consistent with prior evidence showing reduced N400 amplitudes when bilinguals process negative L2 words, which has been interpreted as reflecting lower sensitivity/filtering of negative L2 content (Jończyk et al., Reference Jończyk, Boutonnet, Musiał, Hoemann and Thierry2016; Wu & Thierry, Reference Wu and Thierry2012). However, the novel finding is that such reduction applies in conditions where bilinguals are preparing to produce a word and, quite remarkably, this effect applies irrespective of whether they simply read the word in L2 or translate it into L1.
We also observed an increase in the LPP amplitudes when participants translated L2 words into L1 compared to when they read them aloud, with no such difference between L1-to-L2 translation and reading aloud L1 words. If we assume that semantic information is more available in L1 than L2 for negative words, it makes sense that participants were more affected by negative valence when they had to translate L2 words into L1. However, it is unclear why this would apply to neutral words as well. We believe that this effect might be contextual in nature: negative L2 words likely triggered re-evaluation for the translation into L1 for all words within the block irrespective of valence.
In Experiment 2, we further investigated whether making participants aware of the valence of an upcoming word would affect emotional sensitivity / filtering in L2.
Experiment 2: Emotional context
Participants
Thirty-five participants took part in Experiment 2, following random assignment. Five participants were excluded from the analyses due to a low quality of the EEG signal. The final sample consisted of 30 Polish–English (L1–L2) bilinguals (28 females, 2 males) aged 22–33 (MAge = 25.23; 95% CI [24.30; 26.16]). As in Experiment 1, participants were classified as highly proficient unbalanced Polish–English bilinguals who had not lived in the L2 (English) environment and had acquired their L2 in an instructional yet immersive learning context. They were engaged in an intensive English-only curriculum (the C2 level of the Common European Framework of Reference, CEFR) at the Faculty of English, Adam Mickiewicz University in Poznań, Poland. Participants reported no language, neurological, or psychiatric disorders, had normal or corrected-to-normal vision, and were not taking any psychoactive drugs around the time of data collection (see also Table 4 and 5 for details on participants’ bio-demographic data). For their participation, they received a gift card of 200 PLN.
Table 4. Experiment 2: Participants sociolinguistic data (means with 95% confidence intervals)
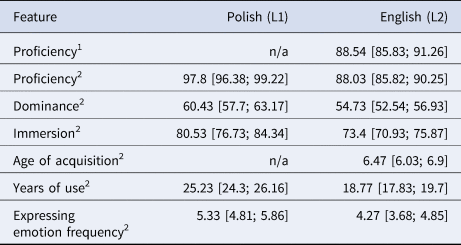
Measurements and ranges: 1LexTALE (Lemhöfer & Broersma, Reference Lemhöfer and Broersma2011): the B1 (0–59%), B2 (60–80%), C1 and C2 (80–100%) CEFR levels; 2Language History Questionnaire 3.0 (LHQ; Li et al. (Reference Li, Zhang, Yu and Zhao2019) as translated into Polish by Naranowicz and Witczak): proficiency, dominance, and immersion scores [%], age of acquisition and years of use [years], and expressing emotion frequency (1 – never, 7 – always).
Table 5. Experiment 2: Participants bio-demographic data (means with 95% confidence intervals)
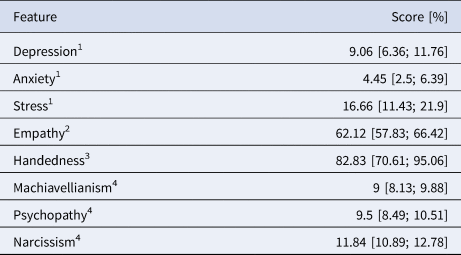
Measurements and ranges: 1DASS-21 (Lovibond & Lovibond, Reference Lovibond and Lovibond1995, as translated into Polish by Makara-Studzińska et al.): normal (0–21%), mild (22–31%), moderate (32–48%), severe (49–64%), and extremely severe (65–100%) levels of depression, anxiety, and stress; 2Empathy Quotient (Baron-Cohen & Wheelwright, Reference Baron-Cohen and Wheelwright2004, as translated into Polish by Wainaina-Woźna): low (0–39%), average (40–64%), above average (65–78%), and high (79–100%) levels of empathy; 3 Edinburgh Handedness Inventory (Oldfield, Reference Oldfield1971): left-handedness (-100– -28), ambidexterity (-29–48), and right-handedness (48–100); 4Polish adaptation of Dirty Dozen Scale (Czarna et al., Reference Czarna, Jonason, Dufner and Kossowska2016): Machiavellianism, psychopathy, and narcissism levels (percentages).
Materials
The stimuli were identical to Experiment 1, with the exception of the cues that consisted of white and black emojis that were either sad or neutral.
Procedure
The experimental procedure was identical to Experiment 1 (see Supplementary Figure 5, Supplementary Materials).
Results
Reaction times
A fixed effect of Task showed faster RTs in the shadowing (M = 715.63 ms, 95% CI [708.14, 723.12]) than translation (M = 1,036.24 ms, 95% CI [1,018.75, 1,053.72]) task, $\hat{\beta } = {-}0.14$, 95% CI [ − 0.16, − 0.13], t(31.99) = −14.65, p < .001. Task was further modulated by Language, $\hat{\beta } = {-}0.06$
, 95% CI [ − 0.08, − 0.04], t(32.53) = −5.40, p < .001, showing faster responses when participants translated Polish (M = 989.47 ms, 95% CI [965.78, 1,013.16]) than English (M = 1,083.76 ms, 95% CI [1,058.17, 1,109.36]) words, b = -0.04, z = -3.24, p = .001. A mirror effect was observed in the shadowing task, whereby participants read aloud English words (M = 699.59 ms, 95% CI [689.05, 710.13]) quicker than Polish words (M = 731.78 ms, 95% CI [721.18, 742.39]), b = 0.02, z = 2.34, p = .019. Finally, Task was modulated by Valence, $\hat{\beta } = {-}0.02$
, 95% CI [ − 0.03, 0.00], t(7, 660.57) = −2.39, p = .017, with marginally slower translations of negative (M = 1,056.86 ms, 95% CI [993.99, 1,039.93]) than neutral (M = 1,016.96 ms, 95% CI [1,030.30, 1,083.43]) words, b = -0.01, z = -1.69, p = .091 (see Supplementary Figure 6, Supplementary Materials). We did not find other effects (ps > .1).
Accuracy
A fixed effect of Task showed higher accuracy in the shadowing (M = 92.40%, 95% CI [91.65, 93.16]) than translation (M = 79.12%, 95% CI [77.97, 80.28]) task, $\hat{\beta } = 1.96$, 95% CI [1.63, 2.29], z = 11.65, p < .001. Also, a fixed effect of Valence showed higher accuracy for neutral (M = 87.25%, 95% CI [86.30, 88.20]) rather than negative words (M = 84.28%, 95% CI [83.25, 85.32]), $\hat{\beta } = {-}0.27$
, 95% CI [ − 0.51, − 0.02], z = −2.11, p = .035. Finally, Task was modulated by Valence, $\hat{\beta } = 0.42$
, 95% CI [0.09, 0.76], z = 2.50, p = .012 whereby participants were more accurate when translating neutral (M = 81.94%, 95% CI [80.40, 83.49]) than negative words (M = 76.30%, 95% CI [74.59, 78.02]), b = 0.48, z = 3.85, p = < .001 (see Supplementary Figure 7, Supplementary Materials). We did not find other effects (ps > .1).
Electrophysiological results
Early Posterior Negativity
The EPN amplitudes were more pronounced for words in English (M = 0.38 μV, 95% CI [0.35, 0.40]) than Polish (M = 0.82 μV, 95% CI [0.79, 0.84]), F(1, 29) = 16.76, p < .001, $\hat{\eta }_G^2 = .015$, 90% CI [.000, .153] (see Supplementary Figure 8, Supplementary Materials). We did not find other effects (ps > .1).
N400
The N400 amplitudes increased for words in Polish (M = -1.27 μV, 95% CI [-1.28, -1.25]) as compared to words in English (M = -1.02 μV, 95% CI [-1.04, -1.00]), F(1, 29) = 7.10, p = .012, $\hat{\eta }_G^2 = .005$, 90% CI [.000, .112]. N400 also increased for neutral (M = -1.21 μV, 95% CI [-1.22, -1.19]) relative to negative words (M = -1.08 μV, 95% CI [-1.09, -1.06]), F(1, 29) = 4.31, p = .047, $\hat{\eta }_G^2 = .001$
, 90% CI [.000, .073] (see Figure 3).
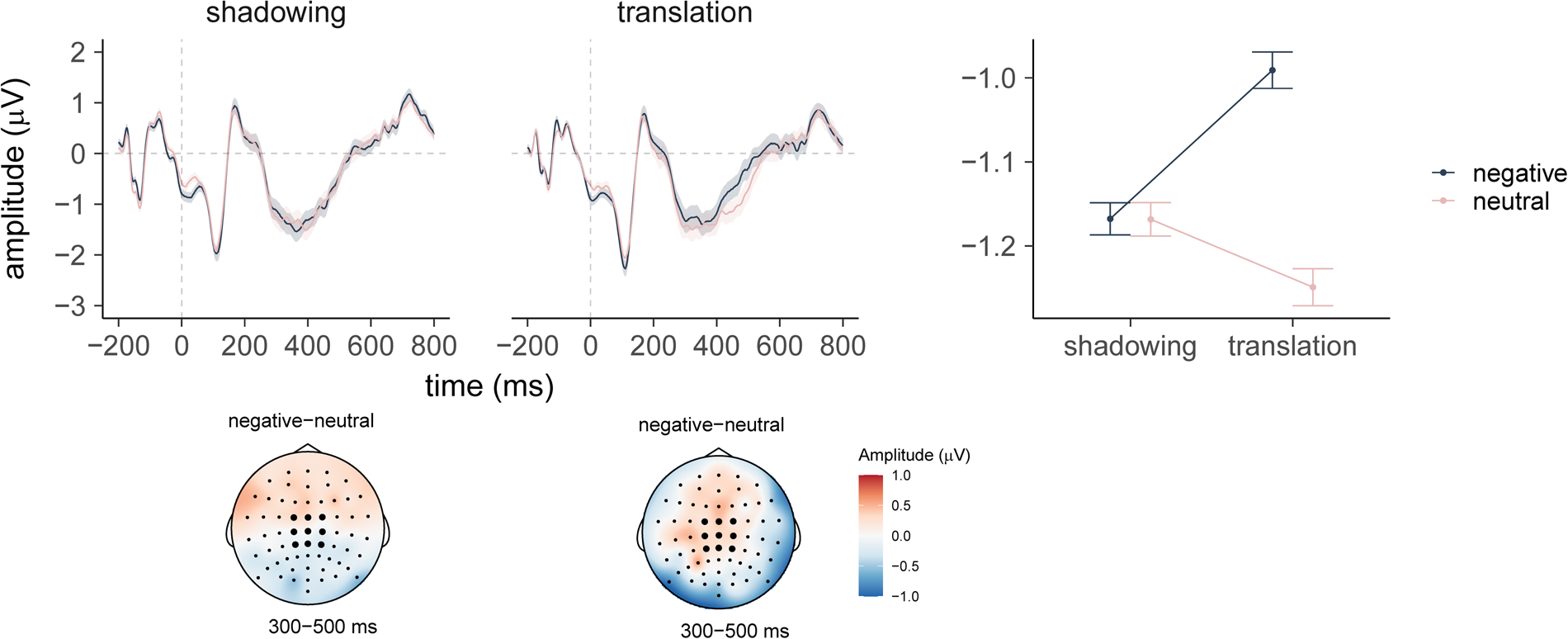
Figure 3. N400 elicited by negative and neutral words in the translation and shadowing task (Experiment 2). Waveforms illustrate brain potential variations computed via linear derivation from nine fronto-centro–parietal electrodes (FC1, FC2, FCz, C1, C2, Cz, CP1, CP2, and CPz). Time 0 coincides with word presentation onset. The topographic maps represent the difference in N400 amplitude between the conditions of interest in the 300–500 ms window. The line plot represents mean N400 amplitude averaged over the electrodes of interest in the 300–500 window. Shaded areas (ERP plot) and error bars (line plot) depict 95% confidence intervals (CI).
A Task-by-Valence interaction, F(1, 29) = 6.94, p = .013, $\hat{\eta }_G^2 = .001$, 90% CI [.000, .073] showed more pronounced N400 amplitudes for neutral (M = -1.25 μV, 95% CI [-1.27, -1.23]) than negative (M = -0.99 μV, 95% CI [-1.01, -0.97]) words in the translation task only, b = 0.26, t = 2.95, p = .006). We did not find other effects (ps > .1).
Late Posterior Positivity
The LPP amplitudes increased overall for words presented in English (M = 0.66 μV, 95% CI [0.64, 0.67]) as compared to Polish (M = 0.38 μV, 95% CI [0.37, 0.39]), F(1, 29) = 5.12, p = .031, $\hat{\eta }_G^2 = .009$, 90% CI [.000, .133].
A Language-by-Task interaction, F(1, 29) = 6.89, p = .014, $\hat{\eta }_G^2 = .005$, 90% CI [.000, .121] showed larger LPP amplitudes for words to be translated from English (M = 0.76 μV, 95% CI [0.74, 0.78]) than Polish (M = 0.27 μV, 95% CI [0.25, 0.29]), b = 0.49, t(29) = 2.81, p = .009.
A Language-by-Valence-by-Task interaction, F(1, 29) = 9.98, p = .004, $\hat{\eta }_G^2 = .005$, 90% CI [.000, .114], showed more positive LPP amplitudes when participants were translating negative words from L2 to L1 (M = 0.99 μV, 95% CI [0.95, 1.02]) than from L1 to L2 (M = 0.19 μV, 95% CI [0.16, 0.22]), b = 0.79, t(29) = 3.94, p = < .001. Further, we found greater LPP amplitudes when participants were translating negative than neutral words from L2 to L1 only (M = 0.54 μV, 95% CI [0.51, 0.57]), b = 0.45, t(29) = 2.83, p = .008. Finally, negative L2 words evoked increased LPP amplitudes in the translation task as compared to the shadowing task (M = 0.48 μV, 95% CI [0.46, 0.51]), b = -0.50, t(29) = -2.48, p = .019 (see Figure 4). We did not find other effects (p > .1).
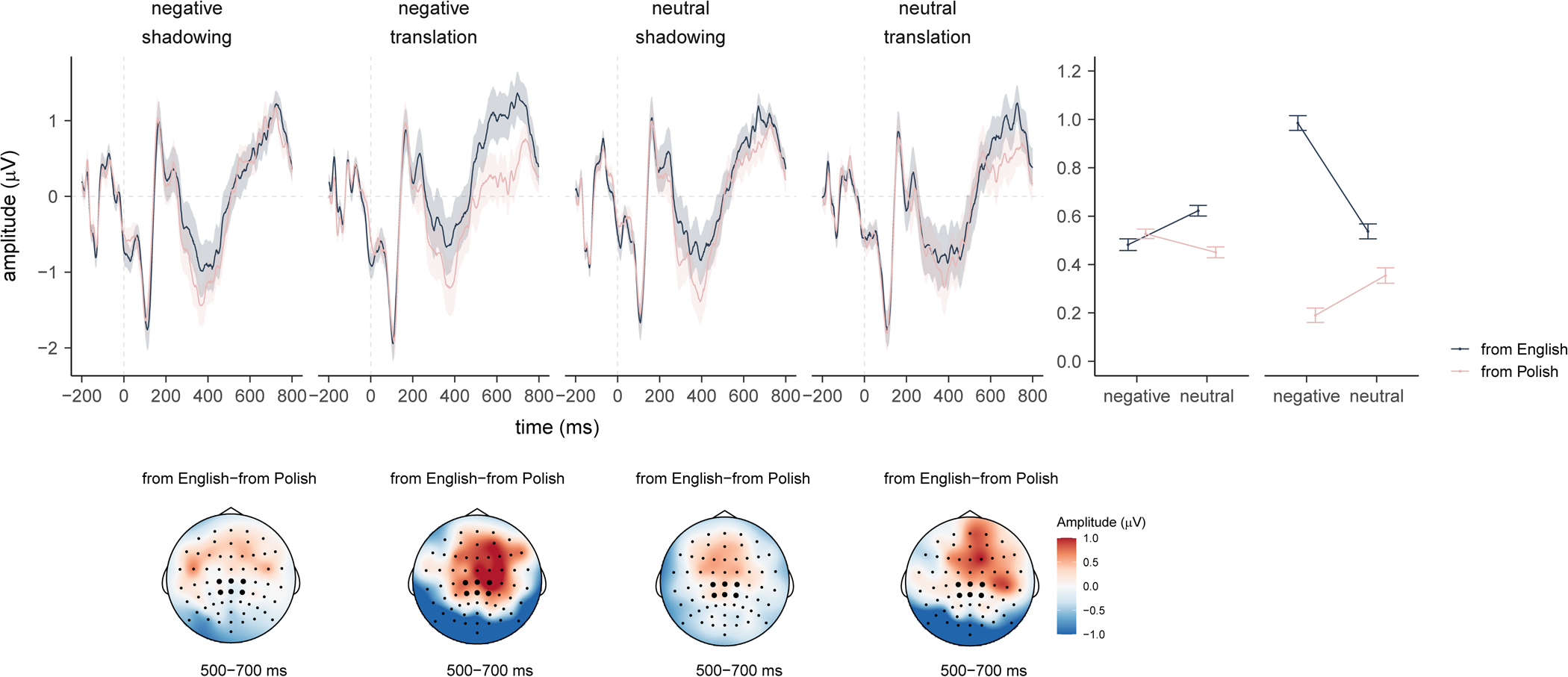
Figure 4. LPP elicited by negative and neutral words in Polish and English in the translation and shadowing task (Experiment 2). Waveforms illustrate brain potential variations computed via linear derivation from six centro–parietal electrodes (C1, C2, Cz, CP1, CP2, and CPz). Time 0 coincides with word presentation onset. Topographic maps represent the difference in LPP amplitudes between the conditions of interest in the 500–700 ms time window. The line plot represents mean LPP amplitudes averaged over the electrodes of interest in the 500–700 time window. Shaded areas (ERP plot) and error bars (line plot) depict 95% confidence intervals (CI).
Discussion
In Experiment 2, we did not find increased EPN amplitudes for negative relative to neutral words, nor did we find an interaction between Language and Valence within the N400 time frame. The lack of an EPN modulation contrasts with previous findings on emotional word processing (Kissler & Bromberek-Dyzman, Reference Kissler and Bromberek-Dyzman2021; Kissler et al., Reference Kissler, Herbert, Peyk and Junghofer2007, 2009). Note, however, that the EPN is usually associated with the processing of words presented outside an emotional context. This was not the case in this experiment, since words were always preceded by a congruent emotional cue. Thus, the cue may have primed and reduced the emotional impact of words at the source of the EPN modulation. The presence of the cue may also explain why we did not find an interaction between Language and Valence in the time frame of the N400. Indeed, negative cues may have generated a contextual effect extending to neutral cues, thus prompting lower emotional sensitivity to all stimuli within a block (see General Discussion for comparison with Experiment 1).
Note that Valence interacted with Task, such that the N400 elicited by negative words was reduced in amplitude as compared to neutral words only when words required translation. An intuitive explanation for this difference relates to differences in the scope of priming for negative as compared to neutral words. Indeed, negatively valenced words selected for our experiment (valence ratings between 1.4 and 4.2) form a considerably smaller corpus than neutral words (valence ratings between 4.5 and 6.4): negative words, n = 3372, and neutral words, n = 7831 (based on Warriner et al., Reference Warriner, Kuperman and Brysbaert2013) and therefore a negative cue is likely to induce greater priming than a neutral one. Furthermore, this difference would not apply to the same extent in the shadowing task as in the translation task, because full semantic access is required in the latter but not the former.
Critically, LPP amplitudes increased as participants prepared to translate negative words from L2 to L1 as compared to L1 to L2. We interpret this as a sign that negative L2 words were more demanding to translate into L1 than L1 words into L2. Beyond the fairly well-established finding that negative L2 content elicits reduced brain responses as compared to L1 in comprehension, this result shows that orally translating words into L1 in an emotional context boosts re-evaluation as compared to translating words into L2.
General discussion
Here, we investigated the brain dynamics associated with preparation for production of negative and neutral words in Polish (L1) – English (L2) bilinguals in the presence of neutral (Experiment 1) or emotional (Experiment 2) cues. In Experiment 1, we found reduced N400 amplitudes for negative as compared to neutral L2 words, irrespective of the task. We also found increased LPP responses when participants translated from L2 to L1, irrespective of valence. In Experiment 2, N400 amplitudes were also reduced for negative as compared to neutral words but only when participants had to translate and irrespective of translation direction. The LPP response increased in amplitude for negative over neutral words when participants translated words from L2 to L1.
Overall, we observed an attenuation of neural activity for the processing of L2 as compared to L1 negative words and a tendency for increased neural activity when participants had to translate negative words from L2 to L1. These results corroborate prior findings reported in EEG studies on comprehension in bilingualism (Jończyk et al., Reference Jończyk, Boutonnet, Musiał, Hoemann and Thierry2016; Wu & Thierry, Reference Wu and Thierry2012; Zhang et al., Reference Zhang, Jończyk, Wu, Lan, Gao, Hu, Thierry and Gao2023), adding support to the hypothesis that negative information may be suppressed in L2, but this time in a context where words have to be produced rather than simply understood.
In Experiment 2, unlike in Experiment 1, however, we did not find a modulation of the N400 by Language and Valence in either task. While the N400 amplitudes decreased for negative as opposed to neutral words in the translation task, the effect was of similar magnitude for both translation directions. This result suggests that emotional cueing was effective in Experiment 2: participants were not only instructed about the task but also about the emotional valence of the upcoming word, allowing for anticipation to occur. Anticipating a negative word likely diminished the impact of the language of operation on emotional word processing.
The LPP result in Experiment 2 showed the predicted effect of increased LPP amplitudes for negative words during translation from L2 to L1 (Rohr & Rahman, Reference Rohr and Rahman2018) – that is, when participants prepared for producing L1. This result also corroborates the existing electrodermal and pupillometry reports of increased late response to negative meaning in L1 likely related to the re-evaluation of emotional meaning (Baumeister et al., Reference Baumeister, Foroni, Conrad, Rumiati and Winkielman2017; Iacozza et al., Reference Iacozza, Costa and Duñabeitia2017; Jankowiak & Korpal, Reference Jankowiak and Korpal2018; Thoma et al., Reference Thoma, Hüsam and Wielscher2022; Toivo & Scheepers, Reference Toivo and Scheepers2019). Here, we extend these findings in a design testing production of emotional words in both L1 and L2. Bilinguals thus experience reduced sensitivity to negative content when processing words in L2 with a view of producing them in L1, even when they are cued to expect emotional words.
Our findings also show that the processing of emotional meaning is modulated by emotional cueing. Although we expected to find EPN modulation by valence in both experiments, EPN amplitudes were larger for negative than neutral words only when the cueing context was neutral (Experiment 1) – that is, when participants could not predict the emotional status of an upcoming word. By contrast, when information about emotional valence was available from the cue (Experiment 2), participants displayed lower sensitivity to negative words at the stage of stimulus encoding (as indexed by the EPN) and semantic processing (as indexed by the N400). These results suggest that emotional cueing elicits strong anticipation effects and that socio-emotional information coded in emojis carries sufficiently meaningful information to shape early and late stages of meaning processing (Hernández-Gutiérrez et al., Reference Hernández-Gutiérrez, Muñoz, Sánchez-García, Sommer, Rahman, Casado, Jiménez-Ortega, Espuny, Fondevila and Martín-Loeches2021, Reference Hernández-Gutiérrez, Muñoz, Sánchez-García, Sommer, Rahman, Casado, Jiménez-Ortega, Espuny, Fondevila and Martín-Loeches2022; Maquate et al., Reference Maquate, Kissler and Knoeferle2022; Rohr & Rahman, Reference Rohr and Rahman2015; Yang et al., Reference Yang, Yang, Xiu and Yu2021).
Behaviourally, as expected, participants were slower and less accurate when translating words as opposed to reading them aloud (Dottori et al., Reference Dottori, Hesse, Santilli, Vilas, Caro, Fraiman, Sedeño, Ibáñez and García2020). Reaction times were slower when participants translated words from L2 to L1, which is consistent with the LPP effect found in both experiments. Also, participants made more errors when translating negative words. This result supports the account that negative information attracts attention, resulting in modulation of neurophysiological responses, and, in turn, slower response times and/or more errors (i.e., the negativity-bias/attentional vigilance hypothesis; Estes & Adelman, Reference Estes and Adelman2008; Ito et al., Reference Ito, Larsen, Smith and Cacioppo1998; Kawasaki et al., Reference Kawasaki, Adolphs, Kaufman, Damasio, Damasio, Granner, Bakken, Hori and Howard2001; Pratto & John, Reference Pratto and John1991). While we did not observe the interaction between Language and Valence in behavioural measures in either experiment, previous studies suggest that behavioural measures may not be sensitive enough to detect such differences (Jończyk et al., Reference Jończyk, Boutonnet, Musiał, Hoemann and Thierry2016, Reference Jończyk, Korolczuk, Balatsou and Thierry2019; Wu & Thierry, Reference Wu and Thierry2012; Zhang et al., Reference Zhang, Jończyk, Wu, Lan, Gao, Hu, Thierry and Gao2023).
Limitations and future directions
Our findings are limited to a comparison between the production of negative and neutral words within and across the two languages of a bilingual. We decided not to include positive words from our design to increase statistical power (see also Jończyk et al., Reference Jończyk, Korolczuk, Balatsou and Thierry2019; experiment 2 in Rohr & Rahman, Reference Rohr and Rahman2018). Furthermore, single words are far from conveying the kind of emotional impact that can be anticipated from more complex constructs such as phrases, sentences, or even narratives. Also, the bilinguals tested here were highly proficient in English, which likely minimised potential differences between operating in L1 and L2. This is not really a limitation in the sense that one could expect effects reported here to be only larger in low proficient bilinguals.
It remains an open question whether the effects we found would be replicated in covert or imagined speech paradigms (e.g., Soroush et al., Reference Soroush, Herff, Ries, Shih, Schultz and Krusienski2023), which have the advantage of avoiding motor artifacts but the shortcoming of increasing the difficulty of response monitoring. Future studies may also want to consider alternative methods of speech artifact correction to increase generalizability (Abbasi et al., Reference Abbasi, Steingräber and Gross2021). Finally, researchers investigating production of emotional language in bilinguals may want to use more naturalistic stimuli for both emotional cues (e.g., faces, sounds) and targets (e.g., sentences, narratives) as well as look into different EEG metrics (see Janssen et al., Reference Janssen, van der Meij, López-Pérez and Barber2020 for temporal dynamics of independent components; Rossi et al., Reference Rossi, Soares, Prystauka, Nakamura and Rothman2022 for brain oscillations).
Conclusion
Across two EEG experiments, we provide novel evidence for attenuated semantic processing of negative L2 words and increased re-evaluation for negative L1 word production in Polish–English bilinguals. Our results align with prior EEG, EDA, pupillometry, and self-report evidence suggesting that when bilinguals operate in their second language, they tend to be less affected by negative information presented in L2. To our knowledge, this study provides the first account of reduced emotional response to L2 and increased re-evaluation of L1 in a production context, bringing us closer to understanding emotional communication in bilinguals.
Supplementary Material
For supplementary material accompanying this paper, visit https://doi.org/10.1017/S1366728924000075
Data availability statement
This manuscript was compiled using the papaja (Aust & Barth, Reference Aust and Barth2020) library in R Markdown. The R Markdown document along with raw data used to compile the manuscript is available here: https://doi.org/10.17605/OSF.IO/U6XQN
Acknowledgments
This project was funded by the National Science Center (to RJ, 2020/37/B/HS6/00610). GT was supported by a grant from the Polish National Agency for Academic Exchange under the NAWA Chair Programme (PPN/PRO/2020/1/00006).
The authors made the following contributions
Rafał Jończyk: Conceptualization, Methodology, Software, Investigation, Formal analysis, Visualization, Writing – Original Draft, Writing – Review & Editing, Funding acquisition, Supervision, Project administration;
Marcin Naranowicz: Methodology, Investigation, Formal analysis, Data curation, Writing - Review & Editing;
Tarik Bel S-Bahar: Formal analysis, Writing – Review & Editing;
Katarzyna Jankowiak: Methodology, Formal analysis, Investigation, Writing – Review & Editing;
Paweł Korpal: Methodology, Investigation, Writing – Review & Editing;
Katarzyna Bromberek-Dyzman: Methodology, Investigation, Writing – Review & Editing;
Guillaume Thierry: Formal analysis, Writing – Review & Editing.
Competing interests
The author(s) declare none.