Introduction
Climate change predictions that are affecting most agricultural regions and livestock transportation routes are related to increasing ambient temperatures, rainfall variability, water availability, and increased climatic anomalies, such as heatwaves, frosts, bushfires, and floods, affecting livestock health, welfare, and productivity. These events have triggered and prioritized a critical digital transformation within livestock research and industries to be more predictive than reactive, implementing new and emerging technologies on animal monitoring for decision-making purposes. Several advances in smart livestock monitoring aim for the objective measurement of animal stress using digital technology to assess the effect of livestock welfare and productivity using biometrics and artificial intelligence (AI).
The most accurate methods to measure livestock health and welfare are invasive tests, such as analysis of tissue and blood samples, and contact sensors positioned on the skin of animals or internally either by minor surgery, intravaginal, or rectally implanted (Jorquera-Chavez et al., Reference Jorquera-Chavez, Fuentes, Dunshea, Jongman and Warner2019a; Zhang et al., Reference Zhang, Zhang and Liu2019b; Chung et al., Reference Chung, Li, Kim, Van Os, Brounts and Choi2020). However, these are apparently impractical approaches to monitor many animals for continuous assessments on farms. These approaches require a high level of know-how by personnel for sampling, sensor placement, data acquisition processing, analysis and interpretation. Furthermore, they impose medium to high levels of stress on animals, introducing biases in the analysis and interpretation of data; for this reason, researchers are focusing on developing novel contactless methods to improve animal welfare (Neethirajan and Kemp, Reference Neethirajan and Kemp2021). There are also visual assessments that can be made by experts and trained personnel to assess levels of animal stress and welfare. However, these can be subjective and require human supervision and assessment with similar disadvantages of the aforementioned physiological assessments and sensor technologies (Burn et al., Reference Burn, Pritchard and Whay2009).
Recent digital advances in sensor technology, sensor networks with The Internet of Things (IoT) connectivity, remote sensing, computer vision and AI for agricultural and human-based applications have allowed the potential automation and integration of different animal science and animal welfare assessment approaches (Morota et al., Reference Morota, Ventura, Silva, Koyama and Fernando2018; Singh et al., Reference Singh, Kumar, Tandon, Sood and Sharma2020). There has been increasing research on implementing these new and emerging digital technologies and adaption to livestock monitoring, such as minimal contact sensor technology, digital collars and remote sensing (Karthick et al., Reference Karthick, Sridhar and Pankajavalli2020). Furthermore, novel analysis and modeling systems have included machine and deep learning modeling techniques to obtain practical and responsible AI applications. The main applications for these technologies have been focused on assessing physiological changes from animals to be related to different types of stress or the early prediction of diseases or parasite infestation (Neethirajan et al., Reference Neethirajan, Tuteja, Huang and Kelton2017; Neethirajan and Kemp, Reference Neethirajan and Kemp2021). One of the most promising approaches is implementing AI incorporating remote sensing and machine learning (ML) modeling strategies to achieve a fully automated system for non-invasive data acquisition, analysis, and interpretation. Specifically, this approach is based on inputs from visible, thermal, multispectral, hyperspectral cameras and light detection and ranging (LiDAR) to predict targets, such as animal health, stress, and welfare parameters. This approach is presented in detail in the following sections of this review.
However, much of the research has been based on academic work using the limited amount of data accumulated in recent years to test mainly different AI modeling techniques rather than deployment and practical application to the industry. Some research groups have focused their efforts on pilots for AI system deployments to assess the effects of heat stress on animals and their respective production, welfare on farming and animal transport, animal identification for traceability, and monitoring greenhouse emissions to quantify and reduce the impact of livestock farming on climate change.
This review is based on the current research on these new and emerging digital technologies applied to livestock farming to assess health, welfare, and productivity (Table 1). Some AI-based research applied for potential livestock applications have tried to solve too many complex problems rather than concentrating on simple and practical applications, and with few deployment examples. However, the latter is a generalized problem of AI applications within all industries, in which only 20% of AI pilots, have been applied to real-world scenarios and have made it to commercial production. The latter figures have increased slightly due to COVID-19 for 2021, with increases up to 20% for ML and 25% for AI deployment solutions, according to the Hanover Enterprise Financial Decision Making 2020 report (Wilcox, Reference Wilcox2020). By establishing a top-down approach (identifying goldilocks problems), specific and critical solutions could be easily studied to develop effectively new and emerging technologies, including AI. In Australia and worldwide, several issues have been identified for livestock transport in terms of the effect of climate change, such as effects of increased temperatures, droughts, and heatwaves on livestock welfare; especially during long sea trips through very hot transport environments, such as those in the Persian Gulf, with temperatures reaching over 50°C) and the identification and traceability of animals. Many livestock producing countries have identified AI and a digital transformation as an effective and practical solution for many monitoring and decision-making problems from the industry.
Table 1. Summary of biometric methods to assess health and welfare for cattle, sheep, and pigs
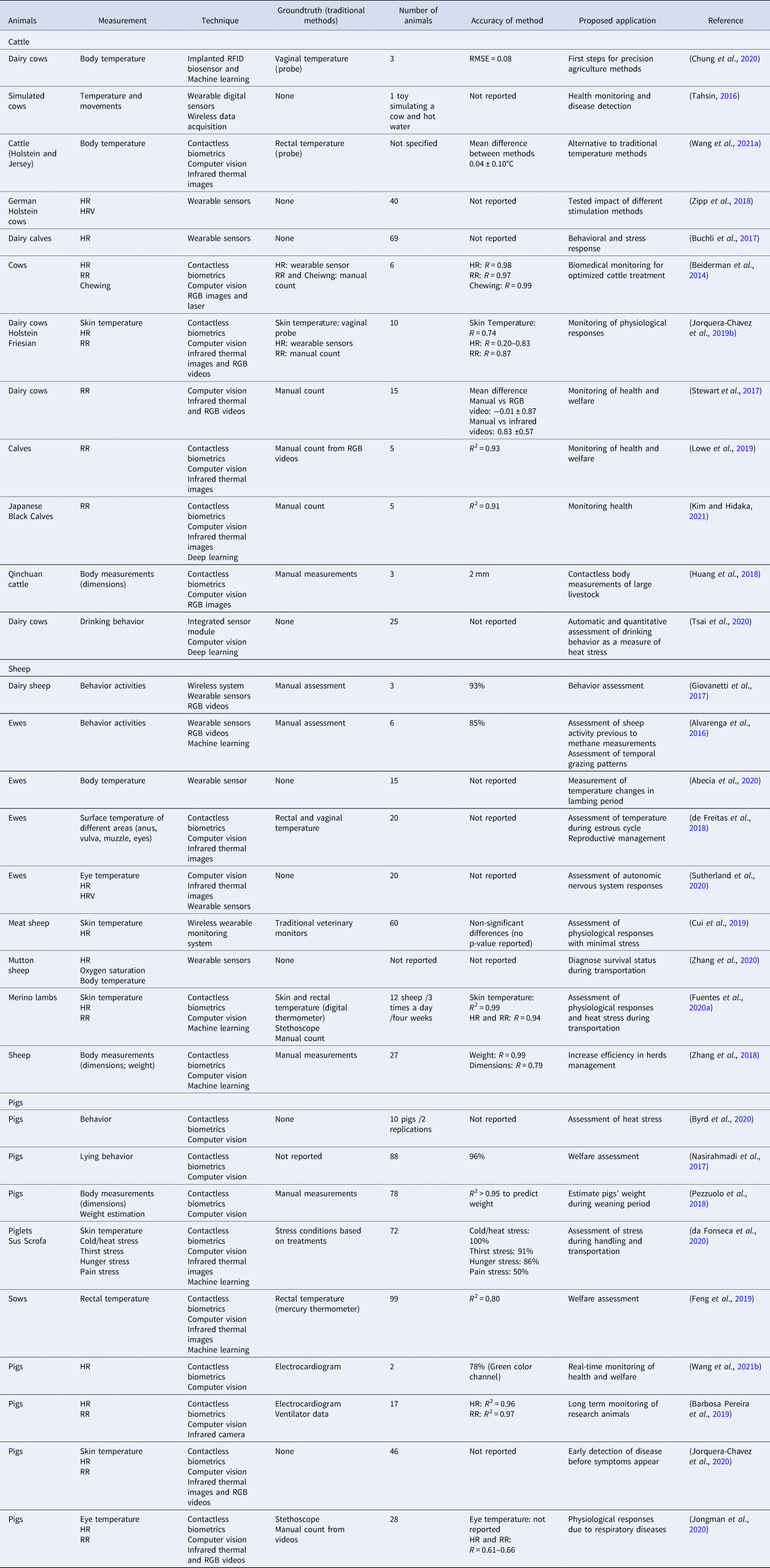
* Abbreviations: RFID, radio frequency identification; RMSE, root mean squared error; HR, heart rate; HRV, heart rate variability; RR, respiration rate; RGB, red, green, blue.
Biometric techniques for health and welfare assessment
The most common methods for animal welfare and health assessment are either visual and subjective, specifically for animal behavior, or invasive. They may involve collecting blood or urine samples to be analyzed using expensive and time-consuming laboratory techniques such as enzyme-linked immunosorbent assay (ELISA) and polymerase chain reaction (PCR) (Neethirajan et al., Reference Neethirajan, Tuteja, Huang and Kelton2017; Du and Zhou, Reference Du and Zhou2018; Neethirajan, Reference Neethirajan2020). Other measurements that are usually related to the health and welfare of animals are based on their physiological responses such as body temperature, heart rate (HR), and respiration rate (RR) (Fuchs et al., Reference Fuchs, Sørheim, Chincarini, Brunberg, Stubsjøen, Bratbergsengen, Hvasshovd, Zimmermann, Lande and Grøva2019; Halachmi et al., Reference Halachmi, Guarino, Bewley and Pastell2019). To measure body temperature, the most reliable methods are intravaginal or measured in the ear, with the most common devices based on mercury or digital thermometers (Jorquera-Chavez et al., Reference Jorquera-Chavez, Fuentes, Dunshea, Jongman and Warner2019a; Zhang et al., Reference Zhang, Zhang and Liu2019b). Body temperature is vital for early detection and progression of heat stress, feed efficiency, metabolism, and disease symptoms detection such as inflammation, pain, infections, and reproduction stage, among others (McManus et al., Reference Mcmanus, Tanure, Peripolli, Seixas, Fischer, Gabbi, Menegassi, Stumpf, Kolling and Dias2016; Zhang et al., Reference Zhang, Zhang and Liu2019b).
Traditional techniques to assess HR may involve manual measurements using stethoscopes (DiGiacomo et al., Reference Digiacomo, Simpson, Leury and Dunshea2016; Jorquera-Chavez et al., Reference Jorquera-Chavez, Fuentes, Dunshea, Warner, Poblete and Jongman2019b; Fuentes et al., Reference Fuentes, Gonzalez Viejo, Chauhan, Joy, Tongson and Dunshea2020a), or automatic techniques based on electrocardiogram (ECG) devices, such as commercial monitor belts with chest electrodes, such as the Polar Sport Tester (Polar Electro Oy, Kempele, Finland) (Orihuela et al., Reference Orihuela, Omaña and Ungerfeld2016; Stojkov et al., Reference Stojkov, Weary and Von Keyserlingk2016), and photoplethysmography (PPG) sensors attached to the ear (Nie et al., Reference Nie, Berckmans, Wang and Li2020). The HR parameter and variability are usually used as an indicator of environmental stress, gestation period, metabolic rate, and diagnosis of cardiovascular diseases (Fuchs et al., Reference Fuchs, Sørheim, Chincarini, Brunberg, Stubsjøen, Bratbergsengen, Hvasshovd, Zimmermann, Lande and Grøva2019; Halachmi et al., Reference Halachmi, Guarino, Bewley and Pastell2019). On the other hand, RR is typically measured by manually counting the flank movements of animals resulting from breathing in 60 s using a chronometer (DiGiacomo et al., Reference Digiacomo, Simpson, Leury and Dunshea2016; Fuentes et al., Reference Fuentes, Gonzalez Viejo, Chauhan, Joy, Tongson and Dunshea2020a) or counting the breaths in 60 s using a stethoscope, or by attaching sensors in the nose, or thorax, which can detect breathing patterns (Jorquera-Chavez et al., Reference Jorquera-Chavez, Fuentes, Dunshea, Jongman and Warner2019a). Respiration rate can be used to indicate heat stress and respiratory diseases (Mandal et al., Reference Mandal, Gupta, Joshi, Kumar and Mondal2017; Slimen et al., Reference Slimen, Chniter, Najar and Ghram2019; Fuentes et al., Reference Fuentes, Gonzalez Viejo, Chauhan, Joy, Tongson and Dunshea2020a).
The main disadvantage of traditional methods based on contact or invasive sensors to assess physiological responses is the potential stress they can cause to the animal by the methodology used, which can introduce bias. The stress may be caused by the anxiety provoked by the restraint and manipulation/contact with their bodies for the actual measurement or to attach different sensors. Furthermore, these methods tend to be costly and time-consuming, making it very impractical assessing a large group of animals. In manual measurements, they may also have human error and, therefore, are subjective and not that reliable. Some specific applications for different livestock will be discussed, separating cattle, sheep and pigs (Table 1).
Cattle
To assess the body temperature of cattle continuously, Chung et al. (Reference Chung, Li, Kim, Van Os, Brounts and Choi2020) proposed an invasive method for dairy cows by implanting a radio frequency identification (RFID) biosensor (RFID Life Chip; Destron Fearing™, Fort Worth, TX, USA) on the lower part of ears of three cows that were monitored for 1 week; however, this method showed medium-strength correlations when compared directly to the intravaginal temperature probe for two of the cows (R 2 = 0.73) and low correlation in the third cow (R 2 = 0.34). The authors then developed a ML model based on the long short-term memory method to increase prediction accuracy. However, the study only reported the root mean squared error (RMSE = 0.081) of the model but left out the accuracy based on the correlation coefficient as it should be done for regression ML models. On the other hand, Tahsin (Reference Tahsin2016) developed a remote sensor system named Cattle Health Monitor and Disease Detector, connected using a wireless network. This system integrated a DS1620 digital thermometer/thermostat (Maxim Integrated™, San Jose, CA, USA) and a Memsic 2125 thermal accelerometer (Parallax, Inc., Rocklin, CA, USA) to assess the activity of animals by measuring the lateral and horizontal movements of the cow. The integrated sensors node was placed on the neck using a collar, with the option to be powered using a solar panel. Furthermore, Wang et al. (Reference Wang, Shih, Juan, Su and Wang2021a) developed a non-invasive/contactless sensor system to assess the body temperature of cattle using an infrared thermal camera (AD-HF048; ADE Technology Inc., Taipei, Taiwan), an anemometer (410i; Testo SE & Co., Kilsyth, VIC, Australia), and a humiture sensor (RC-4HA; Elitech Technology, Inc., Milpitas, CA. USA). These sensors were placed in the feedlot at 1 m from the cows and 0.9 m above the ground to record the head of each cow, while these were restrained using a headlock. The authors used a rectal thermometer as groundtruth to validate the method and reported a difference of 0.04 ± 0.10°C between the grountruth and the method proposed. The anemometer and humiture sensor were used to remove the frames affected by external weather factors to extract outliers.
In the case of HR, Zipp et al. (Reference Zipp, Barth, Rommelfanger and Knierim2018) used Polar S810i and RS800CX sensors attached to the withers and close to the heart to measure HR and HR variability (HRV) while locked after milking to assess the impact of different stimulation methods (acoustic, manual and olfactory). However, the authors reported technical problems to acquire HR and HRV, which led to missing values and altered the analysis. This is another drawback of using contact sensors as they can become unreliable due to different reasons, such as natural animal movements causing sensors to lose contact with the animal skin and connectivity problems. Buchli et al. (Reference Buchli, Raselli, Bruckmaier and Hillmann2017) used a Polar S810i belt attached to the torso of calves to measure HR while the animals were in their pen. However, similar to the previous study, these authors had errors in the data acquired and excluded data from eight calves. To avoid these problems, remote sensing methods have been explored, such as those developed by Beiderman et al. (Reference Beiderman, Kunin, Kolberg, Halachmi, Abramov, Amsalem and Zalevsky2014), based on an automatic system to assess HR, RR and chewing activity using a tripod holding a PixeLink B741 camera (PixeLink, Rochester, NY, USA) and a Photop D2100 laser connected to a computer. The laser pointed at the neck and stomach of the cow. The acquired signal was analyzed using the ‘findpeaks’ Matlab® (Mathworks, Inc., Natick, MA, USA) function to assess HR from the neck area and RR and chewing from the stomach section. The authors reported a correlation coefficient R = 0.98 for HR, R = 0.97 for RR and R = 0.99 for chewing data compared with manual measurements for RR and chewing and Polar sensor for HR. These latter methods may solve the contact problems and unreliability of data quality; however, they seem to still be manual methods requiring operators. The authors did not propose an automation system for measurements.
Jorquera et al. (Reference Jorquera-Chavez, Fuentes, Dunshea, Warner, Poblete and Jongman2019b) also presented contactless methods to assess skin temperature, HR and RR of dairy cows using remote sensing cameras and computer vision analysis. These authors used a FLIR AX8 camera (FLIR Systems, Wilsonville, OR, USA) integrated into a Raspberry Pi V2.1 camera module to record infrared thermal images (IRTI) and RGB videos of the face of the cows while restrained in the squeeze chute. The IRTIs were analyzed automatically using the FLIR Atlas software development kit (SDK) for Matlab® and cropped the videos in the eye and ear sections. The RGB videos were used to assess HR using the PPG method based on the luminosity changes in the green channel of the eye, forehead and full face of the cows; these signals were then further analyzed using a customized Matlab® code previously developed for people (Gonzalez Viejo et al., Reference Gonzalez Viejo, Fuentes, Torrico and Dunshea2018) and adapted for animals. On the other hand, the authors used a FLIR ONE camera to record non-radiometric videos of the cows. These were analyzed using Matlab® based on the change in pixel intensity in the nose section to measure the inhalations and exhalations from which RR was calculated.
Regarding the RR techniques, besides the manual counts usually conducted based on visual assessment of the flank movement of animals, researchers have also developed computer vision techniques, which aid in the reduction of human error and bias. Stewart et al. (Reference Stewart, Wilson, Schaefer, Huddart and Sutherland2017) assessed 15 dairy cows using three comparative methods to determine RR with (i) manual counts of the flank movements by recording the time it took the cow to reach 10 breaths, (ii) manual counts of flank movements similar to method (i) but from an RGB video recorded using a high-dynamic-range (HDR) CX220E camera (Sony Corporation, Tokyo, Japan), and (iii) manual count of the air movement (temperature variations) from the nostrils. The latter was performed from infrared thermal videos recorded using a ThermaCam S60 camera (FLIR Systems, Wilsonville, OR, USA). The three methods showed similar responses with the highest average difference of 0.83 ± 0.57 between methods (i) and (iii). Furthermore, Lowe et al. (Reference Lowe, Sutherland, Waas, Schaefer, Cox and Stewart2019) presented a similar approach but tested only in five calves. In the latter study, the two methods were (i) manual count of flank movements from an RGB video recorded using a Panasonic HCV270 camera (Panasonic, Osaka, Japan), which was made by recording the time taken for the calf to reach five breath cycles, and (ii) manual count of the thermal fluctuations (color changes) in the nostrils from infrared thermal images recorded using a FLIR T650SC camera. The Adobe Premiere Pro CC (Adobe, San Jose, CA, USA) was used for the manual counts for both methods. A high determination coefficient (R 2 = 0.93) was reported comparing both methods. More recently, Kim and Hidaka (Reference Kim and Hidaka2021) used a FLIR ONE PRO infrared thermal camera to record IRTIs and RGB videos from the face of calves. The authors first measured the color changes from the nostril region manually as the time it took for the calf to complete five breaths. A mask region-based convolutional neural network (Mask R-CNN) and transfer learning were used to automatically develop a model using the RGB video frames to automatically detect and mask the calves' noses. Once the nose was detected and masked in the RGB videos, co-registered IRTIs were used to automatically extract the mean temperature of the region of interest. The authors reported an R 2 = 0.91 when comparing the manual and automatic methods.
Besides those used to assess physiological responses, other biometrics have been explored to be applied in beef and dairy cattle. These methods consist of the use of biosensors and/or image/video analysis (remote sensing). For example, Huang et al. (Reference Huang, Li, Zhu, Fan, Zhang and Wang2018) developed a computer vision method to assess body measurements (dimensions) of cattle using an O3D303 3D LiDAR camera to record the individual animal side view and post-processing using filter fusion, clustering segmentation and matching techniques. Tsai et al. (Reference Tsai, Hsu, Ding, Rustia and Lin2020) developed an integrated sensor module composed of a Raspberry Pi 3B processing unit (Raspberry Pi Foundation, Cambridge, England), a Raspberry Pi V2 camera module and a BME280 temperature and relative humidity sensor for environmental measurement. This integrated module was placed on the top of the drinking troughs in a dairy farm to record the drinking behavior of the cows. The authors then applied convolutional neural networks (CNN) based on Tiny YOLOv3 real-time object detection deep learning network for the head detection of cows to predict the drinking length and frequency which were found to be correlated with the temperature-humidity index (THI; R 2 = 0.84 and R 2 = 0.96, respectively).
Sheep
Researchers have been working on different techniques to assess sheep behavioral and physiological responses using contact and contactless sensors. Giovanetti et al. (Reference Giovanetti, Decandia, Molle, Acciaro, Mameli, Cabiddu, Cossu, Serra, Manca and Rassu2017) designed a wireless system consisting of a halter with a three-axis accelerometer ADXL335 (Analog Devices, Wilmington, MA, USA) attached; this was positioned in the lower jaw of dairy sheep to measure the acceleration of their movements on x-, y- and z-axes. Furthermore, the authors used a Sanyo VPC-TH1 camera (Sanyo, Osaka, Japan) to record videos of the sheep during feeding and manually assessed whether the animals were grazing, ruminating or resting as well as the bites per minute. Similarly, Alvarenga et al. (Reference Alvarenga, Borges, Palkovič, Rodina, Oddy and Dobos2016) designed a halter attached below the jaw of sheep; this halter had an integrated data logger Aerobtec Motion Logger (AML prototype V1.0, AerobTec, Bratislava, Slovakia), which is able to measure acceleration in x-, y- and z-axes transformed into North, East and Down reference system. Additionally, they recorded videos of the sheep using a JVC Everio GZR10 camera (JVC Kenwood, Selangor, Malaysia) to manually assess grazing, lying, running, standing and walking activities. These data were used to develop ML models to automatically predict activities, obtaining an accuracy of 85%.
Abecia et al. (Reference Abecia, María, Estévez-Moreno and Miranda-De La Lama2020) presented a method to measure the body temperature of ewes using a button-size data logger DS1921 K (Thermochron™ iButton®, Maxim Integrated, San Jose, CA, USA) taped under the tail of the animals. This sensor was able to record temperature data every 5 min. Using remote sensing, de Freitas et al. (Reference De Freitas, Vega, Quirino, Junior, David, Geraldo, Rua, Rojas, De Almeida Filho and Dias2018) used a FLIR i50 infrared thermal camera to record images from different areas of the sheep: anus, vulva, muzzle, and eyes. The authors used the FLIR Quickreport software to manually select the different sections in each sheep and obtain each area's mean temperature. They concluded that the vulva and muzzle were the best areas to assess temperature during the estrous cycle in ewes. Sutherland et al. (Reference Sutherland, Worth, Dowling, Lowe, Cave and Stewart2020) also used an infrared thermal camera (FLIR Thermacan S60) to record videos of the left eye of ewes. These videos were analyzed to assess eye temperature using the Thermacam Researcher software ver. 2.7 (FLIR Systems, Wilsonville, OR, USA). Additionally, the authors used a Polar RS800CX sensor and placed it around the ewes thorax to assess HR and HRV.
In terms of potential applications of sensor technology, Cui et al. (Reference Cui, Zhang, Li, Luo, Zhang and Fu2019) developed a wearable stress monitoring system (WSMS) consisting of master and slave units. The master unit was comprised of environmental sensors such as temperature, relative humidity and global positioning system (GPS) attached to an elastic band and placed around the rib cage of sheep, while the slave unit was composed of physiological sensors such as an open-source HR sensor (Pulse Sensor, World Famous Electronics LLC, New York, NY, USA), and a skin temperature infrared sensor (MLX90615; Melexis, Ypres, Belgium). This system was tested on meat sheep during transportation and proposed as a potential method to assess physiological responses with minimal stress. Zhang et al. (Reference Zhang, Feng, Luo, Li and Zhang2020) designed a wearable collar that included two sensors to measure (i) HR and oxygen saturation in the blood (MAX30102; Max Integrated, San Jose, CA, USA), and (ii) body temperature (MLX90614; Melexis, Ypres, Belgium). These sensors were connected to the Arduino Mobile App (Arduino LLC, Boston, MA, USA) through Bluetooth® for real-time monitoring and used an SD card for data storage. The authors also proposed this system to assess physiological responses during the transportation of sheep. However, these studies can only monitor sentinel animals, making it laborious, difficult and impractical for the assessment of all animals transported.
To solve the later problem, Fuentes et al. (Reference Fuentes, Gonzalez Viejo, Chauhan, Joy, Tongson and Dunshea2020a) presented a contactless/non-invasive method to assess temperature, HR and RR of sheep using computer vision analysis and ML. The authors used a FLIR DUO PRO camera to simultaneously record RGB and infrared thermal videos of sheep. The infrared thermal videos were analyzed using customized Matlab® R2020a algorithms to automatically recognize the sheep's head and obtain the maximum temperature. Results showed a very high correlation (R 2 = 0.99) between the temperatures obtained with the thermal camera and the rectal and skin temperatures measured using a digital thermometer. On the other hand, RGB videos were analyzed using customized Matlab® R2020a codes to assess HR and RR based on the PPG principle using the G color channel from RGB scale for HR and ‘a’ from Lab scale for RR. An artificial neural network model was developed using the Matlab® code outputs to predict the real HR and RR (measured manually), obtaining high accuracy of R = 0.94. This study also proposed a potential deployment system to be used for animals in transport.
For other biometric assessments, Zhang et al. (Reference Zhang, Wu, Wuyun, Jiang, Xuan and Ma2018) developed a computer vision method to measure the dimensions of sheep using three MV-EM120C Gigabit Ethernet charge-coupled device (CCD) cameras (Lano Photonics, JiLin Province, China) located at different positions (top, left and right side) of the weighing scale for sheep. The recorded images were analyzed in Matlab® R2013 using the superpixel segmentation algorithm. The authors also obtained the dimension parameters manually and found correlations of R = 0.99 for weight and R = 0.79 for dimensions (width, length, height and circumference) using support vector machine.
Pigs
Pigs are also commonly studied to develop biometric techniques to assess behavioral and physiological responses. For example, Byrd et al. (Reference Byrd, Johnson, Radcliffe, Craig, Eicher and Lay2020) used a KPC-N502NUB camera (KT&C, Fairfield, NJ, USA) mounted on top of the pigs' pens to assess pig behavior. The authors used the GeoVision VMS software (GeoVision Inc, Taipei, Taiwan) and assessed whether the pigs were active (standing or sitting) or inactive (lying sternal or lateral). Nasirahmadi et al. (Reference Nasirahmadi, Hensel, Edwards and Sturm2017) assessed the lying behavior of pigs using closed-circuit television (CCTV) with a Sony RF2938 camera above the pen. Matlab® software was used to analyze the videos using computer vision algorithms to detect the position of each pig and analyze the distance between each animal considering their axes, orientation and centroid. On the other hand, Pezzuolo et al. (Reference Pezzuolo, Guarino, Sartori, González and Marinello2018) obtained body measurements and weight of pigs using a Kinect V1 depth camera (Microsoft Corporation, Redmond, WA, USA) positioned on the top and side of the pen. Videos were analyzed using the Scanning Probe Image Processor (SPIP™) software (Image Metrology, Lyngby, Denmark) to obtain length, front and back height, and heart girth. Furthermore, authors developed linear and non-linear models to predict weight, obtaining an accuracy R 2 > 0.95 in all modeling methods tested. The drawback that the authors mentioned from this technique is that the system can only record data from a single camera at a time because there is interference when using simultaneous data acquisition of the two cameras.
Regarding techniques to measure body/skin temperature from pigs, da Fonseca et al. (Reference Da Fonseca, Abe, De Alencar Nääs, Da Silva Cordeiro, Do Amaral and Ungaro2020) used a Testo 876-1 handheld infrared thermal camera (Testo Instruments, Lenzkirch, Germany) to record images of piglets' full bodies. The IRSoft v3.1 software (Testo Instruments, Lenzkirch, Germany) was used to obtain the maximum and minimum skin temperature values. Rocha et al. (Reference Rocha, Devillers, Maldague, Kabemba, Fleuret, Guay and Faucitano2019) presented a method to measure the body temperature of pigs using two IR-TCM284 infrared thermal cameras (Jenoptik, Jena, Germany). One camera was placed in the pen perpendicular to the pig's body, while the second one was positioned 2.6 m above the pigs in the loading alley for transportation. The areas of interest evaluated were neck, rump, orbital region, and the area behind the ears; these were manually selected using the IRT Cronista Professional Software v3.6 (Grayess, Bradenton, FL, USA) and extracting the minimum, maximum and mean temperatures. Authors found that the temperatures from the orbital region and behind the ears were the most useful to assess different types of stress (cold, heat, thirst, hunger, and pain) during handling and transportation. On the other hand, Feng et al. (Reference Feng, Zhao, Jia, Ojukwu and Tan2019) developed a computer vision and ML method to predict the rectal temperature of sows using a T530 FLIR infrared thermal camera to capture images. The FLIR Tools software (FLIR Systems, Wilsonville, OR, USA) was used to obtain the maximum and mean skin temperature in different areas such as ears, forehead, shoulder, back central and back end, and vulva. With these data, the authors developed a partial least squared regression (PLS) model to predict rectal temperature, obtaining an accuracy of R 2 = 0.80.
Wang et al. (Reference Wang, Youssef, Larsen, Rault, Berckmans, Marchant-Forde, Hartung, Bleich, Lu and Norton2021b) developed a contactless method to assess HR of pigs using two different setups (i) a webcam C920 HD PRO (Logitech, Tainan, Taiwan) located on top of the operation table with an anesthetized pig and (ii) a Sony HDRSR5 Handycam located on a tripod above resting individual housing with a resting pig. Matlab® was used to analyze the videos by selecting and cropping the (i) neck for the first setup and (ii) abdomen, neck and front leg for the dual setup. The authors used the PPG principle with the three color channels of the RGB scale and found the G channel provided the most accurate results compared to measurements using an ECG. Barbosa Pereira et al. (Reference Barbosa Pereira, Dohmeier, Kunczik, Hochhausen, Tolba and Czaplik2019) also developed a method using anesthetized pigs; they used a long wave infrared VarioCam HD head 820 S/30 (InfraTecGmbH, Dresden, Germany) to assess HR and RR. The videos were analyzed using Matlab® R2018a, and it included the segmentation using a multilevel Otsu's algorithm, region of interest (chest) selection, features identification and tracking using the Kanade–Lucas–Tomasi (KLT) algorithm, temporal filtering to measure trajectory and principal components analysis (PCA) decomposition and selection. This allowed them to obtain an estimated HR and RR at the selected frequency rates. The authors reported determination coefficients of R 2 = 0.96 for HR compared to the ECG method and R 2 = 0.97 for RR compared to ventilator data. Jorquera-Chavez et al. (Reference Jorquera-Chavez, Fuentes, Dunshea, Warner, Poblete, Morrison and Jongman2020) developed a contactless method to assess temperature, HR and RR of pigs using an integrated camera composed of a FLIR AX8 infrared thermal camera and a Raspberry Pi Camera V2.1 to record IRTIs and RGB videos, and a FLIR ONE infrared thermal camera to record non-radiometric videos. The authors used the same method as that reported for cows (Jorquera-Chavez et al., Reference Jorquera-Chavez, Fuentes, Dunshea, Warner, Poblete and Jongman2019b) using Matlab® R2018b selecting the eyes and ears as regions of interest for temperature, eye section for HR and nose for RR. The same method was used in the study developed by Jongman et al. (Reference Jongman, Jorquera-Chavez, Dunshea, Fuentes, Poblete, Rajasekhara and Morisson2020), but they used a FLIR DUO PRO R dual camera (infrared thermal and RGB) and reported a correlation coefficient within the R = 0.61–0.66 range for HR and RR compared to manual measurements.
Biometric techniques for recognition and identification
Correct and accurate identification of livestock is essential for farmers and producers. It also allows relating each animal to different productivity aspects such as health-related factors, behavior, production yield and quality and breeding. Furthermore, animal identification is essential for traceability, especially during transport and after selling, to avoid fraud and animal ledger or identification forging. However, traditional methods involve ear tags, tattoos, microchips and radio frequency identification (RFID) collars, which involve high costs, and some may be unreliable and easily hacked or interchanged. Furthermore, they require human labor for their maintenance, making them time-consuming, prone to human error and may lead to swapping tags (Awad, Reference Awad2016; Kumar et al., Reference Kumar, Tiwari and Singh2016, Reference Kumar, Singh and Singh2017a; Zin et al., Reference Zin, Phyo, Tin, Hama and Kobayashi2018). Therefore, some studies in recent years have focused on the development of contactless biometric techniques to automate the recognition and identification of different animals such as bears, using deep learning (Clapham et al., Reference Clapham, Miller, Nguyen and Darimont2020), and cows based on different features such as the face (Cai and Li, Reference Cai and Li2013; Kumar et al., Reference Kumar, Tiwari and Singh2016), muzzle (Kumar et al., Reference Kumar, Singh and Singh2017a), body patterns (Zin et al., Reference Zin, Phyo, Tin, Hama and Kobayashi2018), iris recognition (Lu et al., Reference Lu, He, Wen and Wang2014) or retinal patterns (Awad, Reference Awad2016).
Cattle
Most of these biometric techniques for recognition and identification have been developed for cattle. Authors have presented methods based on one of the three main techniques (i) muzzle pattern identification, (ii) face recognition and (iii) body recognition and identification. The first technique has been applied for cattle recognition using images of the muzzle and analyzed for features as it has a particular pattern that is different for each animal, similar to the human fingerprints. Once these features and patterns are recognized, a deep learning model is developed to identify each cow (Noviyanto and Arymurthy, Reference Noviyanto and Arymurthy2012; Gaber et al., Reference Gaber, Tharwat, Hassanien and Snasel2016; Kumar et al., Reference Kumar, Singh and Singh2017a, Reference Kumar, Singh, Singh, Singh and Tiwari2017b, Reference Kumar, Pandey, Satwik, Kumar, Singh, Singh and Mohan2018; Bello et al., Reference Bello, Talib and Mohamed2020). Face recognition methods using different techniques such as local binary pattern algorithms (Cai and Li, Reference Cai and Li2013) and CNN have been proposed for specific cattle breeds with different colors and patterns, such as Simmental (Wang et al., Reference Wang, Qin, Hou and Gong2020), Holstein, Guernseys and Ayrshires, among others (Kumar et al., Reference Kumar, Tiwari and Singh2016; Bergamini et al., Reference Bergamini, Porrello, Dondona, Del Negro, Mattioli, D'alterio and Calderara2018); however, none has been presented in single-coloured cattle breeds such as Angus. On the other hand, body recognition methods have been developed to identify cows within a herd using computer vision and deep learning techniques. Within the proposed methods are cattle recognition from the side (Bhole et al., Reference Bhole, Falzon, Biehl and Azzopardi2019), from behind (Qiao et al., Reference Qiao, Su, Kong, Sukkarieh, Lomax and Clark2019), different angles (de Lima Weber et al., Reference De Lima Weber, De Moraes Weber, Menezes, Junior, Alves, De Oliveira, Matsubara, Pistori and De Abreu2020) or from the top (Andrew et al., Reference Andrew, Greatwood and Burghardt2019). The latter was proposed to identify and recognize Holstein and Friesian cattle using an unmanned aerial vehicle (UAV) (Andrew et al., Reference Andrew, Greatwood and Burghardt2017, Reference Andrew, Greatwood and Burghardt019, Reference Andrew, Gao, Mullan, Campbell, Dowsey and Burghardt2020a, Reference Andrew, Greatwood and Burghardt2020b). Bhole et al. (Reference Bhole, Falzon, Biehl and Azzopardi2019) proposed an extra step for cow recognition from the side by recording IRTIs to ease the image segmentation and remove the background.
Sheep
While biometrics applied for the identification and recognition of sheep have not been deeply explored, the development of some proposed methods has been published. The techniques that have been reported for sheep consist of retinal recognition using a commercial retinal scanner, OptiReader (Optibrand®, Fort Collins, CO, USA) (Barron et al., Reference Barron, Corkery, Barry, Butler, Mcdonnell and Ward2008), and face recognition using classification methods such as machine or deep learning. Salama et al. (Reference Salama, Hassanien and Fahmy2019) developed a deep learning model based on CNN and Bayesian optimization and obtained an identification accuracy of 98%. Corkery et al. (Reference Corkery, Gonzales-Barron, Butler, Mc Donnell and Ward2007) proposed a method based on independent components analysis and the InfoMax algorithm to identify the specific components from the normalized images of sheep faces and then find them in each tested image; the authors reported an accuracy within 95–95%.
Pigs
The biometric techniques that have been published to identify pigs are based mainly on face recognition and body recognition from the tops of pens. Hansen et al. (Reference Hansen, Smith, Smith, Salter, Baxter, Farish and Grieve2018) developed a face recognition method using CNN with high accuracy (97%). Marsot et al. (Reference Marsot, Mei, Shan, Ye, Feng, Yan, Li and Zhao2020) developed a face recognition system based on a mix of computer vision to identify the face and eyes and deep learning CNN for classification purposes, obtaining an accuracy of 83%. On the other hand, Wang et al. (Reference Wang, Liu and Xiao2018) proposed a method to identify pigs from images recorded from the whole body using integrated deep learning networks such as dual-path network (DPN131), InceptionV3 and Xception, with an accuracy of 96%. Huang et al. (Reference Huang, Zhu, Ma and Guo2020) tested a Weber texture local descriptor (WTLD) identification method with different masks to detect and recognize individual features such as hair, skin texture, and spots using images of groups of pigs; the tested WTLD methods resulted in accuracies >96%. Kashiha et al. (Reference Kashiha, Bahr, Ott, Moons, Niewold, Ödberg and Berckmans2013) based their automatic identification method on computer vision to recognize marked pigs within a pen using the Fourier algorithm for patterns description and Euclidean distance, this technique resulted in 89% accuracy.
Machine and deep learning application in livestock to address complex problems
This section concentrates specifically on the research on AI application using ML and deep learning modeling techniques on livestock, specifically for cattle, sheep and pigs. One of the latest research studies has been focused on the use of AI to identify farm animal emotional responses, including pigs and cattle (Neethirajan, Reference Neethirajan2021). However, it may be difficult to assess and interpret the emotional state of farm animals only from facial expression and ear positioning, as proposed in the latter study, and more objective assessment could be performed using targets based on hormonal measurements from endorphins, dopamine, serotonin and oxytocin among others, which will require blood sampling. Therefore, all the in vitro and tissue applications were excluded from this section because they require either destructive or invasive methods to obtain data.
Cattle
A simple AI approach was proposed using historical data (4 years) with almost ubiquitous sensor technology in livestock farms, such as meteorological weather stations with daily temperature and relative humidity (Fuentes et al., Reference Fuentes, Gonzalez Viejo, Cullen, Tongson, Chauhan and Dunshea2020b). In this study, meteorological data was used to calculate temperature and humidity indices (THI) using different algorithmic approaches as inputs to assess the effect of heat stress on milk productivity as targets in a robotic dairy farm. This approach attempted to answer complex questions with potentially readily available data from robotic and conventional dairy farms and proposed a deployment system for an AI approach with a general accuracy of AI models of 87%. More accurate heat stress assessments could be achieved by either sensor technology, with minimal invasiveness to animals, such as ear clips, collars or similar, or remote sensing cameras, computer vision and deep learning modeling. However, the latter digital approach requires assessing individual animals using extra hardware and sensors, camera systems located in strategic positions allowing monitoring of every single animal (e.g. corral systems and straight alleys). Furthermore, these new digital approaches require the recording of new data. A big question in applications of AI in cattle, in this case, would be whether it is worth the significant extra investment in hardware and ML modeling using new data to increase the accuracy of models by an additional 10.
Sensor technology and sensor networks have been implemented in cattle to assess lameness, such as accelerometers, IoT connectivity and time series ML modeling approaches (Taneja et al., Reference Taneja, Byabazaire, Jalodia, Davy, Olariu and Malone2020; Wu et al., Reference Wu, Wu, Yin, Jiang, Wang, He and Song2020). These applications were the first approaches to be implemented in animals after applications in humans for fitbits. Sensor readings and connectivity using IoT will facilitate the implementation of this technology in a near or real-time fashion. However, there is a big downside of the requirement of sensors for every single animal to be monitored. This is valid to other applications for sensor integration (Neethirajan, Reference Neethirajan2020), such as collars, halter and ear tag sensors (Rahman et al., Reference Rahman, Smith, Little, Ingham, Greenwood and Bishop-Hurley2018), to detect physiological changes, behavior and other anomalies (Wagner et al., Reference Wagner, Antoine, Koko, Mialon, Lardy and Veissier2020).
As mentioned before, animal recognition using deep learning approaches should be considered the first step to apply further remote sensing and AI tools. A second step should be the identification of key features from animals using deep learning (Jiang et al., Reference Jiang, Wu, Yin, Wu, Song and He2019), which makes possible the extraction of physiological information from those specific regions using ML modeling, such as HR from the eye section or exposed skin (e.g. ears or muzzle) and RR from the muzzle section. These animal features should be recognized in a video to extract enough information to obtain physiological parameters that currently require 4–8 s (e.g. HR and RR) for the signal to stabilize and get meaningful data. Hence, the AI implementation steps should consider animal recognition, specific feature recognition and tracking and extraction of physiological parameters using ML.
Integration of UAV, computer vision algorithms and CNN have been attempted for the recognition of cattle from the air (Barbedo et al., Reference Barbedo, Koenigkan, Santos and Santos2019). However, these authors concentrated efforts on the feasibility and testing of different algorithms rather than the potential deployment of a pilot program. Furthermore, these approaches could also be used for animal recognition and the potential extraction of physiological parameters, such as body temperature (using infrared thermal cameras as payload). Dairy cows could offer more identification features than Angus cattle, which may require the implementation of multispectral cameras to include potential non-visible features from animals.
Sheep
Sensor technology and sensor networks have also been applied in parallel with ML approaches for sheep using electronic collars and ear sensors as input data and supervised selecting several behavior parameters as targets with a reported accuracy of <90% for both methods (Mansbridge et al., Reference Mansbridge, Mitsch, Bollard, Ellis, Miguel-Pacheco, Dottorini and Kaler2018). Some predictive approaches from existing data have been attempted to assess carcass traits from early life animal records (Shahinfar et al., Reference Shahinfar, Kelman and Kahn2019) using supervised and unsupervised regression ML methods with various low to high accuracies reported.
Similar detection systems mentioned before for other animals have been applied for sheep counting using computer vision and deep learning CNN methods (Sarwar et al., Reference Sarwar, Griffin, Periasamy, Portas and Law2018), which can also be used in parallel with other AI procedures to extract more information from animals for health or welfare assessments, such as sheep weight (Shah et al., Reference Shah, Thik, Bhatt and Hassanien2021). Following this approach, additional physiological parameters, such as HR, body temperature and RR, can be extracted from individual sheep non-invasively (Fuentes et al., Reference Fuentes, Gonzalez Viejo, Chauhan, Joy, Tongson and Dunshea2020a). The latter study also proposed using this AI approach for real livestock farming applications, such as animal welfare assessment for animals during transportation.
Other welfare assessments have been developed for sheep based on the facial classification expression for pain level applied using deep learning CNN and computer vision with 95% accuracy. However, no deployment was reported, which can be used to assess further animal welfare (Jwade et al., Reference Jwade, Guzzomi and Mian2019).
Pigs
Some simple ML applications have been implemented to predict water usage in pig farms using regression ML algorithms (Lee et al., Reference Lee, Ryu, Ban, Kim and Choi2017). However, this study reported a maximum determination coefficient of R 2 = 0.42 for regression tree algorithms, which could be related to poor parameter engineering, since only temperature and relative humidity were used.
Automatic pig counting (Tian et al., Reference Tian, Guo, Chen, Wang, Long and Ma2019), pig posture detection (Nasirahmadi et al., Reference Nasirahmadi, Sturm, Edwards, Jeppsson, Olsson, Müller and Hensel2019; Riekert et al., Reference Riekert, Klein, Adrion, Hoffmann and Gallmann2020), mounting (Li et al., Reference Li, Chen, Zhang and Li2019) and sow behavior (Zhang et al., Reference Zhang, Cai, Xiao, Li and Xiong2019a), localization and tracking (Cowton et al., Reference Cowton, Kyriazakis and Bacardit2019) aggressive behavior (Chen et al., Reference Chen, Zhu, Steibel, Siegford, Wurtz, Han and Norton2020) have been attempted using computer vision and deep learning. These are relatively complex approaches for meaningful questions considering further pipeline of analyses. These approaches could be used to extract more information from the individual pigs once they have been recognized, such as biometrics, including HR and RR extracted for other animals such as sheep, mentioned before (Fuentes et al., Reference Fuentes, Gonzalez Viejo, Chauhan, Joy, Tongson and Dunshea2020a), and cattle identification (Andrew et al., Reference Andrew, Greatwood and Burghardt2017) with accuracies in identification between 86 and 96% with a maximum of 89 individuals.
Other approaches have been implemented for the early detection (between 1 and 7 days of infection) of respiratory diseases in pigs using deep learning approaches (Cowton et al., Reference Cowton, Kyriazakis, Plötz and Bacardit2018). Other computer vision approaches using visible and infrared thermal imagery analysis without ML approaches also delivered an acceptable assessment of respiratory diseases in pigs (Jorquera-Chavez et al., Reference Jorquera-Chavez, Fuentes, Dunshea, Warner, Poblete, Morrison and Jongman2020).
Conclusions
Implementing remote sensing, biometrics and AI for livestock health and welfare assessment could have many positive ethical implications and higher acceptability by consumers of different products derived from livestock farming. Specifically, integrating digital technologies could directly impact increasing the willingness to purchase products from sources that introduced AI to increase animal welfare on the farm and transport for ethical and responsible animal handling and slaughtering. However, a systematic deployment of different digital technologies reviewed in this paper will require further investment, which some governments, such as Australia, have identified as a priority.
It is difficult to assess the applicability or deployment options from different research studies done so far on livestock, which have applied biometrics and AI, because there is no consistency in the reporting of the accuracy of models, performance, testing for over or underfitting of models, number of animals used or proposed pilot or deployment options (Table 1). Furthermore, in most of these studies, there are no follow-ups on the models either by establishing potential pilot deployments to test them in real-life scenarios. Many researchers only rely on the validation and testing protocols within the model development stage. The latter does not give any information on the practicality or applicability of these digital systems, because circumstances in real-life scenarios change over time and models need to be re-evaluated and continuously fed with new data to learn and adapt to different circumstances and scales of use.
It is also clear that most of the AI developments and modeling for livestock farming applications are academic, and very little research has focused on efficient and practical deployment to real-world scenarios. To change this, researchers should work on real-life problems in the livestock industry, starting with simple ones and pressing questions. The next step is to solve them using efficient and affordable technology, starting with big data analysis from historical data accumulated by different industries. The idea here is to initially apply AI where the data exists, to achieve maximum reach with high performance and scalable applications (e.g. heat stress assessment on milk production using historical weather information and productivity data). It is also required to check whether the correct data is available, avoid basing AI on reduced datasets, and restricted only to test different ML approaches. Academic exercises based on AI modeling for its sake only rarely reach pilot programs and applications in the field. Furthermore, data quality and data security are becoming fundamental issues that should be dealt using digital ledger systems for data and model deployments, such as blockchain implementation. This approach allows treating data and AI models as a currency to avoid hacking and adulteration, especially with AI models and data dealing with welfare assessments for animals in farms to claim ethical production or animals in transport.
To solve these problems, AI modeling, development and deployment strategies should have a multidisciplinary team with constant communication during the model development and deployment stages; what could be a better approach, but very rare nowadays is to have an expert on animal science, data analysis and AI dealing with companies. This could change soon through specialized Agriculture, Animal Science and Veterinary degrees in which data analysis, ML and AI are introduced in their respective academic curriculums.
Integrating new and emerging digital technology with AI development and deployment strategies for practical applications would create effective and efficient AI pilot applications that can be easily scaled up to production to create successful innovations in livestock farming.