Introduction
Agricultural land use provides not only crops and livestock but also non-material benefits, such as aesthetic appreciation and opportunities for recreation associated with beautiful landscapes (Swinton et al. Reference Swinton, Lupi, Robertson and Hamilton2007, Zhang et al. Reference Zhang, Ricketts, Kremen, Carney and Swinton2007, Calvet-Mir et al. Reference Calvet-Mir, Gómez-Baggethun and Reyes-García2012). However, because farmers are mainly interested in efficient yield increases, agricultural land, particularly in less favoured areas, has been abandoned, leading to the degradation of agroecosystem services. To prevent these trends and conserve valuable landscapes by integrating non-material agroecosystem services into farmers’ income (i.e., economic incentives), relevant policy measures have been introduced worldwide (e.g., direct payment schemes for agroecosystem service conservation in less favoured areas; Cooper et al. Reference Cooper, Baldock, Rayment, Kuhmonen, Terluin and Swales2006). However, these economic supports often fail to maintain agricultural landscapes because they overlook or underestimate most sources of landscape value (Bernués et al. Reference Bernués, Rodríguez-Ortega, Ripoll-Bosch and Alfnes2014, Mameno & Kubo Reference Mameno and Kubo2022).
Although it is challenging to assess the value of cultural ecosystem services associated with agricultural lands (Daniel et al. Reference Daniel, Muhar, Arnberger, Aznar, Boyd and Chan2012), the last decade has witnessed an increase in research on the valuation of agricultural landscapes and their ecosystem services in order to resolve complex trade-offs and prioritize ecosystem management (Cheng et al. Reference Cheng, Van Damme, Li and Uyttenhove2019). These valuation studies predominantly relied on stated preference methods (e.g., Campbell Reference Campbell2007, Arnberger & Eder Reference Arnberger and Eder2011, Chen et al. Reference Chen, Qiu, Usio and Nakamura2018); few studies have applied revealed preference approaches to assess the value of agricultural landscapes, such as the hedonic price model (Vanslembrouck et al. Reference Vanslembrouck, Van Huylenbroeck and Van Meensel2005) or the travel cost model (TCM; Fleischer & Tsur Reference Fleischer and Tsur2000, Carpio et al. Reference Carpio, Wohlgenant and Boonsaeng2008, van Berkel & Verburg Reference van Berkel and Verburg2014).
Although revealed preference methods using observed data have several advantages over stated preference methods, the conventional revealed preference approaches can face data collection challenges (see Alberini Reference Alberini2019, Mendelsohn Reference Mendelsohn2019). For example, the TCM often relies on self-reports of respondents, which can cause sampling bias (Loomis Reference Loomis2007, Marini Govigli et al. Reference Marini Govigli, Górriz-Mifsud and Varela2019). Furthermore, despite the existence of seasonality in ecosystem services (e.g., Kubo et al. Reference Kubo, Uryu, Yamano, Tsuge, Yamakita and Shirayama2020), data limitations prevent most revealed preference studies from exploring seasonality (e.g., Fleischer & Tsur Reference Fleischer and Tsur2000). Because agricultural practices and vegetation change throughout the year, researchers must develop methods that address seasonality and provide scientific evidence to support the decision-making by practitioners and policymakers.
The objective of this study was to assess the recreational ecosystem services of a traditional Japanese agricultural landscape by applying mobile phone GPS data to a zonal TCM. We also aimed to evaluate the seasonal heterogeneity of the recreational ecosystem services of the agroecosystem. The application of mobile phone data has attracted attention for its potential to overcome some of the challenges of the revealed preference approach. Following developments in information and communications technology, some valuation studies have attempted to apply several types of big data to TCMs (Ghermandi & Sinclair Reference Ghermandi and Sinclair2019), such as data from Flickr (Ghermandi Reference Ghermandi2018, Sinclair et al. Reference Sinclair, Ghermandi and Sheela2018, Reference Sinclair, Mayer, Woltering and Ghermandi2020, Reference Sinclair, Ghermandi, Signorello, Giuffrida and De Salvo2022), eBird (Kolstoe & Cameron Reference Kolstoe and Cameron2017) and mobile phone networks (Jaung & Carrasco Reference Jaung and Carrasco2020, Kubo et al. Reference Kubo, Uryu, Yamano, Tsuge, Yamakita and Shirayama2020). As data from mobile phone networks are not self-reported, their application is likely to overcome some data collection challenges, such as sampling bias (Kubo et al. Reference Kubo, Uryu, Yamano, Tsuge, Yamakita and Shirayama2020). Moreover, mobile phone data allow for the acquisition of high-resolution spatiotemporal data, which could help address the seasonality of agroecosystem services. However, to the best of our knowledge, little research exists on the application of mobile phone GPS data to valuation methods, which might provide more accurate locational information for mobile phone users than previous approaches (Wang et al. Reference Wang, He and Leung2018).
We focused on a traditional agricultural landscape in Japan. Shiroyone terraced paddy land is a less favoured area for farming; however, farming plays an important role in maintaining not only a historic cultural landscape but also traditional knowledge about sustainable cultivation based on Japanese history and culture, biodiversity and rural cultural heritage, amongst others. As a result, this ancient terraced paddy has been designated as a Globally Important Agricultural Heritage System (GIAHS) by the Food and Agriculture Organization of the United Nations (FAO 2002), as the best terraced rice paddy land in Japan and as a nationally designated cultural landscape property (Chen et al. Reference Chen, Qiu and Nakamura2016, Reference Chen, Qiu, Usio and Nakamura2018, FAO 2020). The Japanese government introduced subsidies to maintain agroecosystems and their services in Shiroyone terraced paddy land, such as direct payment schemes for agroecosystem conservation. For example, farmers or local communities can receive up to USD 210 per 1000 m2 in direct payment for less favoured areas each year, and local communities can receive direct payments of up to USD 54 per 1000 m2 annually for farmland multifunction conservation (i.e., agroecosystem services; Ministry of Agriculture, Forestry and Fisheries 2021). Although direct payment schemes have positive impacts on paddy land maintenance (e.g., Takayama et al. Reference Takayama, Hashizume and Nakatani2020, Reference Takayama, Nakatani, Senda and Fujie2021), it remains unclear whether payments are commensurate with the value of the terraced landscapes in Shiroyone terraced paddy lands.
The Shiroyone terraced paddy land is an ideal site for investigating seasonality. In this area, cultivation begins in April, rice is planted in early May and harvested in September and, in winter, the paddy land lies fallow, has no water and is artificially illuminated at night. Therefore, the present analysis can provide practical information by considering seasonality based on consistent measures.
The present study fills a gap in the literature by addressing the seasonality of recreational value and the impact of policy measures associated with these services using daily GPS data from mobile phones gathered during 2018–2020. The research period includes the beginning of the COVID-19 crisis. This allowed us to assess the impact of the crisis on services associated with terraced paddy fields as well as seasonality. Many studies have explored the impact of the COVID-19 pandemic on recreational use in several areas, such as urban green spaces, national parks and mountain trails (e.g., Berdejo-Espinola et al. Reference Berdejo-Espinola, Suárez-Castro, Amano, Fielding, Oh and Fuller2021, Derks et al. Reference Derks, Giessen and Winkel2020, Souza et al. Reference Souza, Rodrigues, Correia, Normande, Costa and Guedes-Santos2021). However, little is known about the impacts of the COVID-19 crisis and related policies on recreation in farmland; the results of this study offer contributions to the fields of farmland and tourism management as well as food production.
Methods
Study site and data
Shiroyone terraced paddy land (Fig. 1) provides c. 6 tonnes of rice annually (Hirata Reference Hirata2017), which is sold at c. USD 10/kg (an exchange rate of 100 Japanese yen (JPY) = USD 1 is assumed). The terraced paddy land, which is located along the coast, has an area of 4 ha and consists of more than 1000 c. 18-m2 paddy fields. According to counters supplied by the local government at the entrance to the rest area via a roadside station close to the paddy land, the area attracted c. 600 000 visitors per year before the COVID-19 pandemic.
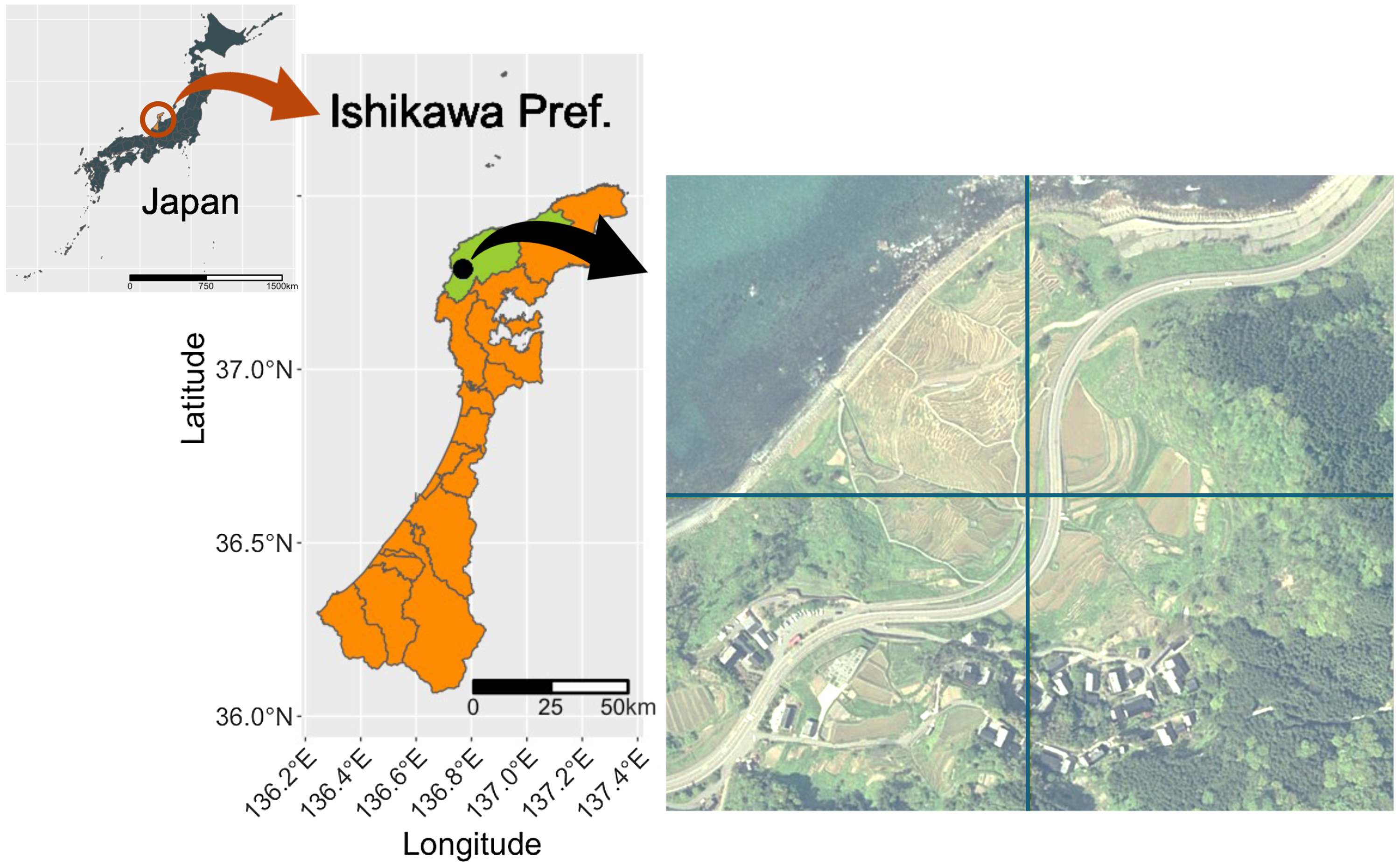
Figure 1. Location of the study area. The satellite map was processed and created from the GSI Maps vector published by Geospatial Information Authority of Japan (https://maps.gsi.go.jp/vector/).
KDDI, Inc. – the second largest mobile phone company in Japan (Kubo et al. Reference Kubo, Uryu, Yamano, Tsuge, Yamakita and Shirayama2020) – provided the GPS location data from the mobile phones used in this study. KDDI data use the extrapolated population based on the location data of smartphones; the extrapolated population was estimated using both market share and national census data. To avoid selection bias due to device ownership by age and unfamiliarity with application operations, census data were used to correct the estimation. The number of visitors to the study area was based on the number of mobile phone users who were over 20 years old, with each grid having a minimum area of 10 m2. The data analysed did not include foreign travellers. Data were collected every 2 min and included information about the users’ residential areas. The residential area was assumed to be the area where mobile phone users stayed for the longest time at night during the month before the paddy land visit. It should be noted that researchers were only given access to aggregated data in some grids (minimum 125 m2) to protect personal privacy. In other words, only the number of users in each grid and aggregated residential information were utilized; we could not access any individual’s information. To protect user privacy, the company also deleted information from grids with fewer than 10 people per grid per day; for detailed information on the GPS-based mobile phone location data provided by KDDI, Inc., see Kim et al. (Reference Kim, Shoji, Mameno, Kubo and Aikoh2023a) and Kim et al. (Reference Kim, Kubo and Nishihiro2023b).
The location of the data source – the Shiroyone terraced paddy land – was composed of four 250-m grids (based on the national grid square code, Nos. 5636-1719-22, 5636-1709-44, 5637-1010-11 and 5637-1000-33; see Appendix S1 for details). As the visitors in each grid were identified by the mobile phone company, duplicate data (visitors) were removed. Even if a mobile phone user visited several of the four grids, the number of visitors was counted as 1. Data on the number of visitors to the grid and their areas of residence were collected daily from 1 June 2018 to 30 September 2020 (823 days) to cover seasonal variations and the impact of the COVID-19 pandemic. To remove non-visitors (i.e., passers-by), only data from people who stayed in the Shiroyone terraced paddy land for over 15 min were included. In total, 643 764 visitor data points were extrapolated from the mobile phone GPS data over the study period. The highest number of monthly visitors occurred in October 2018 (total visitors = 41 845; daily mean = 1349.8), followed by May 2019 (total visitors = 40 262; daily mean = 1298.8). The total number of monthly visitors estimated by KDDI, Inc., was lower than that counted by the local government using the automatic counter, although the number of visitors showed a high correlation (R2 = 0.7991, p < 0.001) with the automatic counter data (Appendix S2).
Econometric model (zonal travel cost model)
This study applied mobile phone GPS data to a zonal TCM to quantify the recreational ecosystem services of the agroecosystem associated with agricultural landscapes in monetary terms. Zonal TCM is a traditional non-market valuation technique for recreational ecosystem services based on revealed behaviour (e.g., Becker et al. Reference Becker, Inbar, Bahat, Choresh, Ben-Noon and Yaffe2005, Mayer & Woltering Reference Mayer and Woltering2018). Recent studies have assessed recreational ecosystem services by applying big data to zonal TCM as the model uses aggregated big data, thereby protecting personal privacy (e.g., Jaung & Carrasco Reference Jaung and Carrasco2020, Kubo et al. Reference Kubo, Uryu, Yamano, Tsuge, Yamakita and Shirayama2020).
Zonal TCM can evaluate the monetary benefits (i.e., consumer surplus; CS) of a recreational ecosystem service of an agroecosystem by deriving a demand curve for the agricultural landscape. The curve is estimated by analysing the relationship between the travel costs incurred to access a recreational site and visitation rates. CS is defined as the difference between the total amount that visitors are willing to pay for specific goods or services and the total amount that they actually pay, which is one of the most common welfare measures in economics (Freeman et al. Reference Freeman, Herriges and Kling2014). The visitation rate (
$V{R_i}$
) was estimated by applying the residential area–destination data of the mobile phone users as per Equation 1:

where
${V_i}$
is the number of visitors to Shiroyone terraced paddy land from residential zone
$i$
and
${N_i}$
is the population of zone
$i$
.
${V_i}$
was calculated by considering the number of actual visitors and the market share of the mobile service company in zone
$i$
, and
${N_i}$
was obtained from the Statistics Bureau of Japan (SBJ; http://www.stat.go.jp/data/jinsui/).
The travel costs (
$T{C_i}$
) incurred by a visitor for a round trip are calculated as per Equation 2:

where
$gcosts$
is the cost of gas, which is assumed to be USD 1.28 per litre (Ministry of Economy, Trade, and Industry; https://www.enecho.meti.go.jp/statistics/petroleum_and_lpgas/pl007/results.html#headline1), and
$feffic$
is the fuel efficiency, which is assumed to be 21.6 km/L (Ministry of Land, Infrastructure, Transport and Tourism; https://www.mlit.go.jp/jidosha/content/001338019.pdf). The distance (
$dist$
) between the terraced paddy land and visitors’ residential area was estimated using gmapsdistance in R (Melo et al. Reference Melo, Rodriguez and Zarruk2018). We first assumed that all visitors travelled by car, except for visitors from two remote islands (Miyako and Ishigaki islands); the distance was subsequently calculated using the Google Map distance matrix application programming interface. The second term of Equation 2 represents opportunity costs (Cesario Reference Cesario1976, Englin & Shonkwiler Reference Englin and Shonkwiler1995, Matthews et al. Reference Matthews, Scarpa and Marsh2018). To estimate the
$wage$
, we used the average of Japanese wage data (Ministry of Internal Affairs and Communications; http://www.stat.go.jp/data/kakei/longtime/index.htm). We also assumed that visitors travelled by car and that the average driving speed in Japan was 60 km/h to calculate the
$time$
to reach the Shiroyone terraced paddy land from each residential area. As there were no shipping routes from the two islands, visitors were assumed to travel by aeroplane from the respective airports on the islands (i.e., Miyako–Naha–Komatsu and Ishigaki–Naha–Komatsu).
In our model, a traditional functional form of travel cost was employed; the dependent variable was the log of the visitation rate and the independent variable was the travel cost
$TC$
(Equation 3):

Referring to previous studies that applied big data to zonal TCM (Jaung & Carrasco Reference Jaung and Carrasco2020, Kubo et al. Reference Kubo, Uryu, Yamano, Tsuge, Yamakita and Shirayama2020), we employed a log-linear model, which is more suitable than other functional forms. Based on the coefficient of travel costs,
$\beta $
, the CS of a visitation per person was estimated as per Equation 4 (Englin & Shonkwiler Reference Englin and Shonkwiler1995, Zhang et al. Reference Zhang, Wang, Nunes and Ma2015):

In our daily CS analysis, we excluded days when there were few visitors and/or small variations in visitors’ residential areas because we could not estimate the coefficient of travel cost using TCMs. We also omitted days when the coefficient of
$TC$
had a positive parameter value from our analysis, which is in accordance with previous studies that applied big data to TCM (e.g., Kubo et al. Reference Kubo, Uryu, Yamano, Tsuge, Yamakita and Shirayama2020, Sinclair et al. Reference Sinclair, Ghermandi, Signorello, Giuffrida and De Salvo2022). This is because such values contradict economic theory, which assumes negative preferences for cost increases.
Results
Our model estimated a statistically significant CS (i.e., monetary value per visit per person) over 192 days at the 5% level. The daily mean CS and daily mean recreational value were USD 45.28 (SD = 24.60) and USD 40 544.84 (SD = 41 221.43), respectively. The recreational value of terraced paddy land varied seasonally (Fig. 2).
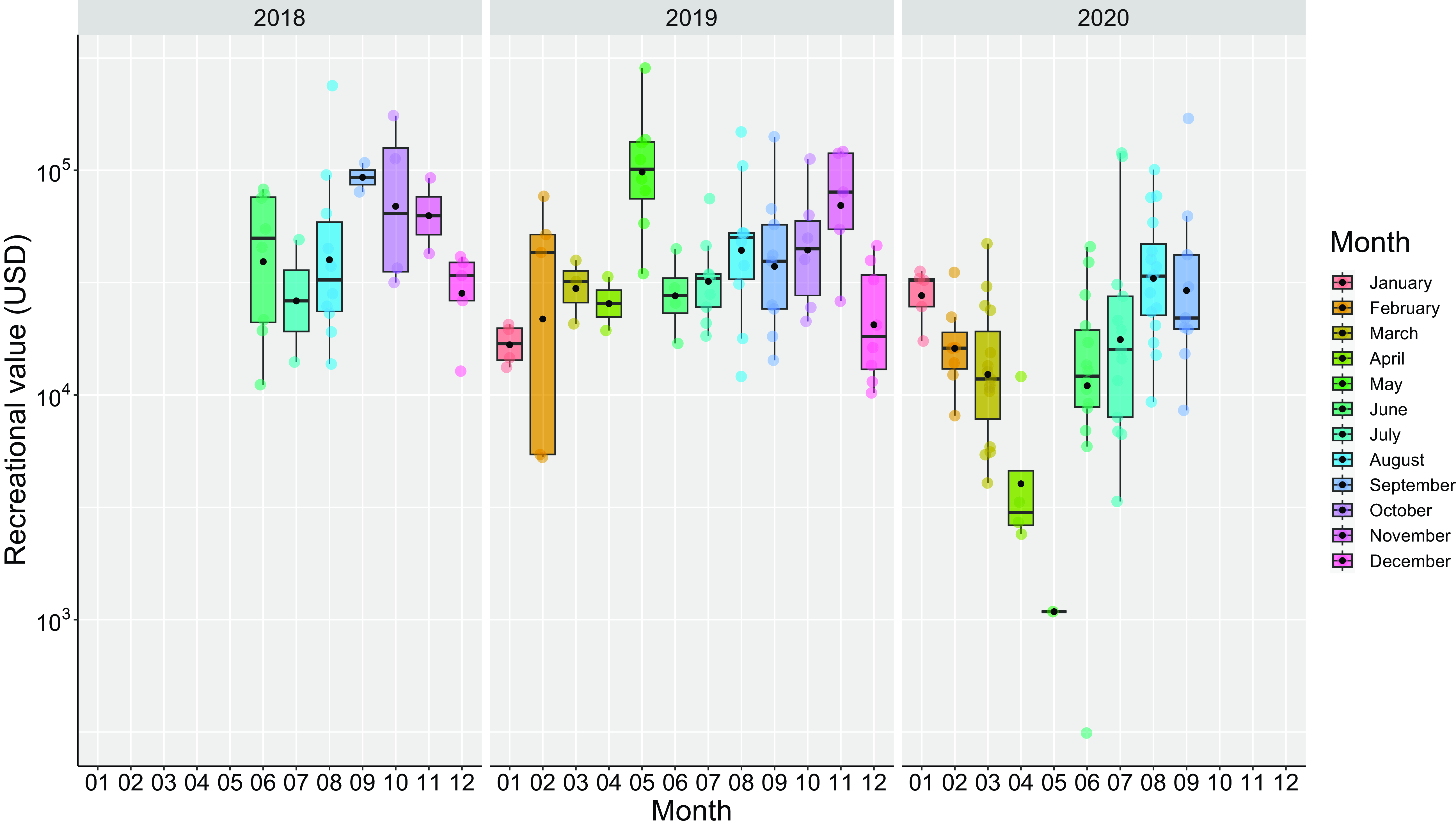
Figure 2. Monthly means of daily recreational value (USD) by year (2018, 2019, 2020). Monthly means of daily recreational value are presented as black dots; boxplots represent the median, first and third quartile and maximum and minimum values except for the outlier recreational values. Each colour dot represents the daily recreational value by month.
The daily recreational value was highest on 3 May 2019 and lowest on 1 June 2020 (USD 285 425 and 312.78, respectively; Table 1). Before the COVID-19 crisis, the daily mean recreational value was USD 51 037.76 (Table 1). The estimated annual total recreational value in 2019 was USD 3 649 319 (daily mean = 49 990.67; SD = 45 124.43; all estimated daily CSs and valuations; see Appendix S3).
Table 1. The consumer surplus (CS) per visit per visitor and recreational value per day of Shiroyone terraced paddy land at the 5% significance level.

a Values were calculated as the CS on each day multiplied by the total population of visitors on each day.
b Daily means (SD) were calculated by excluding data from during the COVID-19 crisis (i.e., from February to September 2020).
During the COVID-19 pandemic, beginning in February 2020, the daily mean recreational value was significantly lower than that before the pandemic (t = 4.6759, p < 0.001; Fig. 3). Daily mean recreational values before and during the COVID-19 pandemic were USD 51 037.76 (SD = 45 392.93) and USD 25 801.62 (SD = 28 889.74), respectively. However, after the Japanese government introduced a travel promotion policy from July 2020, the difference in the daily mean recreational value before and during the COVID-19 pandemic was no longer statistically significant (t = 1.4771, p = 0.1437).
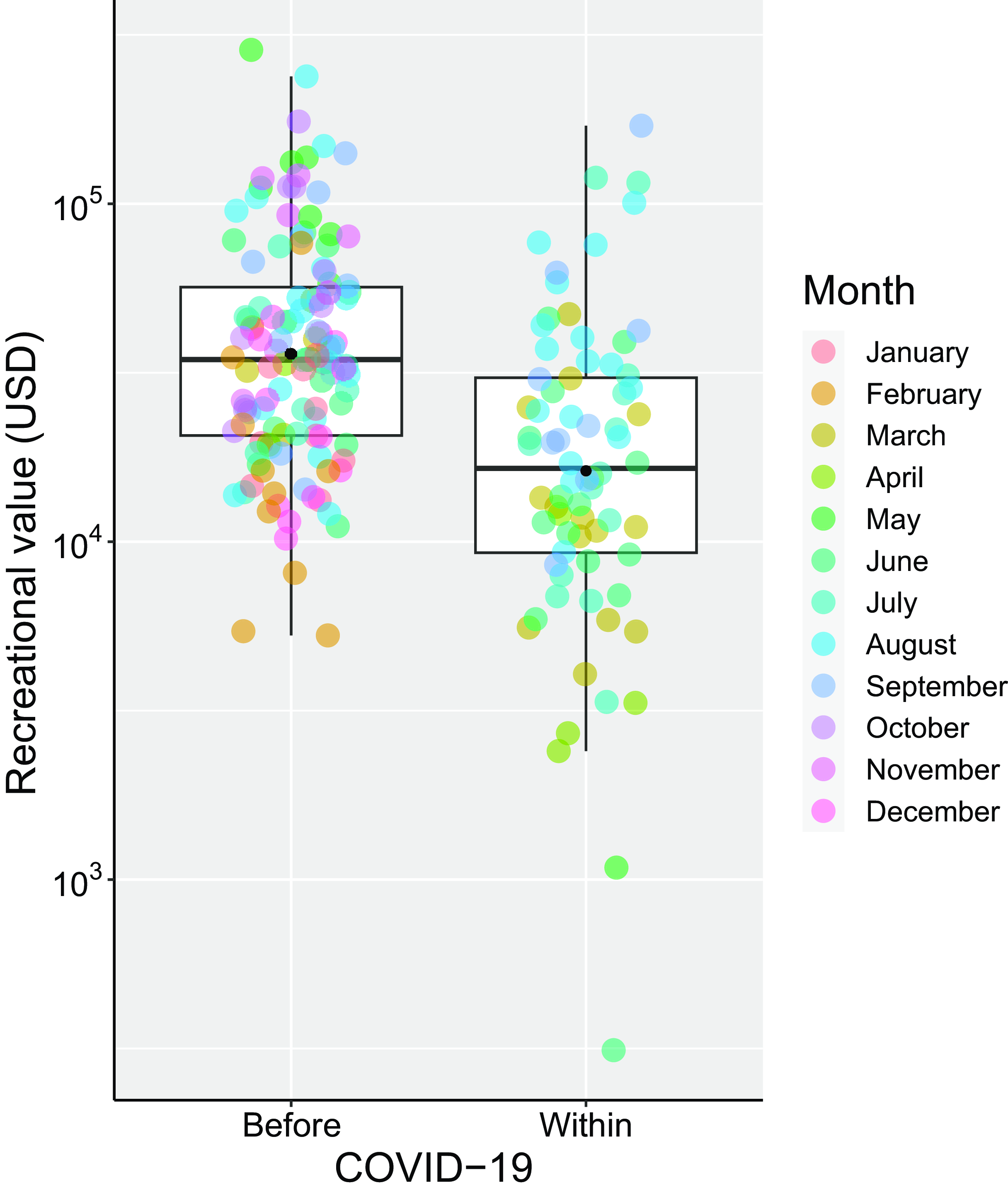
Figure 3. Mean recreational value (USD) before and within the COVID-19 crisis. Black dots represent mean recreational values before and during the COVID-19 crisis; boxplots represent the median, first and third quartile and maximum and minimum values except for the outlier recreational values. Each dot represents the daily recreational value coloured by month.
Discussion
The daily mean recreational value of Shiroyone terraced paddy land was over USD 51 037 (Table 1); thus, its annual recreational value prior to the COVID-19 crisis could be over USD 18 million, and even a conservative estimate would provide a valuation of c. USD 3.6 million (i.e., annual recreational value in 2019). Although it is difficult to compare the recreational value of Japanese agricultural landscapes with those of other countries, the values obtained in this study are lower than those in previous revealed preference studies (Fleischer & Tsur Reference Fleischer and Tsur2000, Carpio et al. Reference Carpio, Wohlgenant and Boonsaeng2008). For example, the CS and value associated with agricultural landscapes were estimated to be USD 167 per visit per visitor and USD 82 million per year, respectively, in agricultural landscapes in Hula, Israel (Fleischer & Tsur Reference Fleischer and Tsur2000). However, our annual mean CS of USD 48 per person is approximately twice as large as that of a Dutch agricultural landscape, for which the TCM estimated the recreational value was c. EUR 23 per person (van Berkel & Verburg Reference van Berkel and Verburg2014). One possible explanation for the differences in these results may be sampling procedures and periods. For example, Fleischer and Tsur (Reference Fleischer and Tsur2000) conducted a face-to-face survey only in the spring. Although some challenges associated with conventional valuation surveys can be overcome with mobile phone data, our analysis included other challenges that could lead to over- or underestimating the recreational ecosystem services of paddy land. Mobile phone data privacy protections for mobile phone users prevented us from conducting more detailed analyses of visitor behaviour (e.g., Kubo et al. Reference Kubo, Uryu, Yamano, Tsuge, Yamakita and Shirayama2020). For instance, when someone visits several destinations during a trip or stays for several days in the area, our model overestimates agroecosystem services. This limitation could be solved by combining the present approach with data sourced from other areas, such as social media or mobile phone data from other companies (e.g., Tieskens et al. Reference Tieskens, Van Zanten, Schulp and Verburg2018). For example, another Japanese mobile phone company provides ‘people flow data’, enabling tracking of detailed individual behaviour within a small grid. However, these data must be used responsibly to address the trade-offs between research robustness and privacy protection (Ghermandi et al. Reference Ghermandi, Langemeyer, Van Berkel, Calcagni, Depietri and Vigl2023). Alternatively, given that the number of visitors estimated by mobile phone data was lower than that obtained by the automatic counter (Appendix S2), our results from the mobile phone data may be underestimates. We did not have detailed information regarding counter calibration efforts and counter management. Future collaboration between local practitioners and researchers is required to investigate ground data and mobile phone data simultaneously whilst devoting significant effort to monitoring particular areas.
Our approach detected seasonal heterogeneity in the recreational ecosystem services of the agroecosystem. Despite the difficulty of comparing the stated and revealed preference methods, the annual mean CS is much higher than the mean visitor willingness to pay of USD 8.99 for the conservation of Shiroyone terraced paddy land (Chen et al. Reference Chen, Qiu, Usio and Nakamura2018). The highest CS in this study was greater than USD 8.99; conversely, the lowest CS was less than USD 8.99 (Table 1). Therefore, the values of agricultural landscapes may be over- or underestimated if seasonality is neglected (Mkwara et al. Reference Mkwara, Marsh and Scarpa2015). Our study demonstrates that the application of big data can overcome the challenge of limited data availability when measuring the seasonality of the recreational ecosystem services of an agroecosystem.
Our findings also revealed that the recreational aspects of terraced paddy land improved during the period when the travel promotion policy was introduced, even during the COVID-19 pandemic. Thus, the COVID-19 pandemic altered the recreational use of agricultural land. Such impacts have been reported for urban green spaces, national parks and mountain trails (Berdejo-Espinola et al. Reference Berdejo-Espinola, Suárez-Castro, Amano, Fielding, Oh and Fuller2021, Derks et al. Reference Derks, Giessen and Winkel2020, Souza et al. Reference Souza, Rodrigues, Correia, Normande, Costa and Guedes-Santos2021). Our results further indicate the importance of the sampling period when evaluating recreational ecosystem services owing to the susceptibility of recreational ecosystem services to the COVID-19 crisis. Moreover, the findings raise the issue of the conservation of aesthetically terraced paddy landscapes during the COVID-19 crisis, in that the decline in the number of visitors may have had a negative impact on the local society and economy, leading to a decrease in the motivation for conservation (Gössling Reference Gössling1999). The COVID-19 crisis might have affected not only food production (FAO 2020, Dixon et al. Reference Dixon, Weerahewa, Hellin, Rola-Rubzen, Huang and Kumar2021, Mishra et al. Reference Mishra, Bruno and Zilberman2021) but also the conservation of terraced paddies and people’s mental and physical health by preventing them from enjoying the recreational ecosystem services of the agroecosystem.
These findings support previous evidence that ancient agricultural landscapes play an important role as a public good (Tilman et al. Reference Tilman, Cassman, Matson, Naylor and Polasky2002, Rasmussen et al. Reference Rasmussen, Coolsaet, Martin, Mertz, Pascual and Corbera2018). Even before the COVID-19 pandemic, the value of recreational ecosystem services was 10 times greater than that of food supply services, which was c. USD 6 million (i.e., 6 tonnes × USD 10 per kg) in Shiroyone terraced paddy land (Hirata Reference Hirata2017). This indicates the importance of non-material benefits derived from sustainable agricultural practices in terraced paddy land, even if the land is considered less favourable for rice production. Our findings also support the validity of payments for the maintenance of multiple agroecosystem services, such as the direct payment scheme to conserve multifunctional farmland. Moreover, given that the landscape supports traditional knowledge of its sustainable cultivation as well as being a historic cultural landscape (Antrop Reference Antrop2005), we support the validity of the FAO’s nomination of Shiroyone terraced paddy land as a GIAHS. Although the effects of GIAHS status on agroecosystem conservation in Shiroyone terraced paddy land remain unclear, a high cultural value has been maintained in this land; GIAHS designation might contribute to the conservation of Shiroyone terraced paddy land and its ecosystem services.
We also demonstrated that the farmers might not receive sufficient benefits associated with the recreational ecosystem services of the agroecosystem through the direct payment scheme for the conservation of multifunctional farmland. Given that a total of USD 54 per 1000 m2 is paid directly for agroecosystem services and that Shiroyone terraced paddy land comprises c. 20 000 m2, farmers receive only c. USD 1080 per year on average for the conservation of their multifunctional land. This is much lower than the annual value of the recreational ecosystem services of the agroecosystem in terraced paddy lands before the COVID-19 pandemic. Our findings support the idea that the total economic value of mountain agroecosystems is higher than the current level of support for agri-environmental policies (Bernués et al. Reference Bernués, Rodríguez-Ortega, Ripoll-Bosch and Alfnes2014). Given that our approach only captures recreational value and that traditional terraced paddy lands provide multiple ecosystem services, such as flood protection, wildlife habitats and conservation of traditional knowledge (Antrop Reference Antrop2005, Porter et al. Reference Porter, Costanza, Sandhu, Sigsgaard and Wratten2009, Mameno et al. Reference Mameno, Kubo and Shoji2021, Mameno & Kubo Reference Mameno and Kubo2023), there is room for the government to reconsider the direct payment scheme to properly reflect public demand.
By using mobile phone data, researchers can quantify the impacts of environmental changes and human activities, such as artificial lighting in winter and seasonality of land use, which influence the seasonality of recreational ecosystem services. The present approach revealed the seasonality of recreational benefits through long-term collection of daily visitor data. Thus, our study demonstrates that the application of GPS data from mobile phones has the potential to overcome some challenges in evaluating cultural ecosystem services (Daniel et al. Reference Daniel, Muhar, Arnberger, Aznar, Boyd and Chan2012). Our detailed analysis and findings also suggest the potential regarding using large amounts of mobile phone data in measuring policy impacts, such as the effect of GIAHS registration on visitor numbers, because these data are useful for the long-term monitoring of recreational ecosystem services. Another advantage is the opportunity for a more objective valuation using recent big data approaches (e.g., Kolstoe & Cameron Reference Kolstoe and Cameron2017, Ghermandi Reference Ghermandi2018, Sinclair et al. Reference Sinclair, Ghermandi and Sheela2018). Owing to the non-self-reported and non-stated preference nature of our data, our estimates are not subject to sampling (i.e., preferences of survey samples for participation in research and the survey efforts of researchers) or hypothetical biases. Solving these biases could contribute to evidence-based policymaking. Traditionally, biased evidence often makes policymakers reluctant to apply such results in practice. Overall, the application of GPS data from mobile phones enables the objective identification of spatial and seasonal disparities in agroecosystem services, providing valuable insights for effective evidence-based policymaking. Moreover, because of the high correlation with counter data, the use of GPS data from mobile phones could be beneficial for recreational management and monitoring, as doing so could represent an opportunity to evaluate the validity of existing monitoring tools, such as entrance counters.
Conclusions
By integrating mobile phone GPS big data into environmental valuation methods (e.g., Fleischer & Tsur Reference Fleischer and Tsur2000), we reveal seasonality in recreational agroecosystem services associated with terraced paddy land and show how the high value of the recreational services affirm the validity of agri-environmental policies such as payment for ecosystem services. We also show that the incentives provided by the public/government may be insufficient relative to the recreational value. Our findings could help inform the development of agri-environmental policies by assessing the non-market value of agroecosystem services as monetary and indicating the appropriate amount of economic support required to achieve sustainable agricultural land use.
Supplementary material
To view supplementary material for this article, please visit https://doi.org/10.1017/S0376892924000122.
Acknowledgments
The authors thank Dr K Ujiie and Dr H Oguma for their helpful comments on our analysis and research. We would also like to express our gratitude for the helpful comments of the journal editor and anonymous reviewers of our drafts. The authors thank Wajima City for providing data on the number of automatically counted visitors shown in Appendix S2.
Author contributions
Kota Mameno: conceptualization (equal); data curation (lead); formal analysis (lead); methodology (equal); visualization (equal); resources (equal); funding acquisition (supporting); writing – original draft (lead). Arne Arnberger: resources (equal); writing – review & editing (equal). Yasushi Shoji: conceptualization (equal); formal analysis (supporting); methodology (equal); supervision (lead); writing – review & editing (equal). Takahiro Kubo: conceptualization (equal); formal analysis (supporting); methodology (equal); visualization (equal); resources (equal); project administration (lead); supervision (supporting); funding acquisition (lead); writing – review & editing (equal).
Financial support
This work was supported by the Japan Society for the Promotion of Science (Nos. 19H03095 and 22H02377 and Overseas Research Fellowships), the Sompo Environment Foundation (Grant Program for Doctoral Course Students) and the Environment Research and Technology Development Fund (JP- MEERF20224003) of the Environmental Restoration and Conservation Agency, provided by the Ministry of Environment of Japan.
Competing interests
The authors declare that they have no known competing financial interests or personal relationships that could influence the work reported in this paper.
Ethical standards
None.