Introduction
Warning and response systems
The COVID-19 pandemic has led to an unprecedented global focus among international and civil society organisations and member states on warning and response systems for infectious diseases as a key tool to mitigate the risk of future pandemics (Butchard and Balogun, Reference Butchard and Balogun2023; Faviero et al., Reference Faviero, Stocking, Hoffman, Liu, Bausch and Galea2022; Gostin et al., Reference Gostin, Halabi and Klock2021; Krofah et al., Reference Krofah, Choe, Sud and Degarmo2022; Rogers et al., Reference Rogers, Anderson-Berry, Bogdanova, Fleming, Gitay, Kahandawa, Kootval, Staudinger, Suwa, Tsirkunov and Wang2020; Sirleaf and Clark, Reference Sirleaf and Clark2021; The White House US Government, 2021; UK Government, 2021).
The United Nations Disaster Risk Reduction Office (UNDRR) defines an early warning system (EWS) as “an integrated system for hazard monitoring, forecasting and prediction, disaster risk assessment, communication and preparedness activities, systems and processes that enables individuals, communities, governments, businesses and others to take timely action to reduce disaster risks in advance of hazardous events” (UNDRR, n.d.). This key concept applicable to both natural and biological hazards underlines the importance of coordinated prevention, preparedness and mitigation efforts.
However, it is important to note that while the shared effort to enhance warning and response systems for infectious diseases is unique, the disease itself is not. Understanding and addressing the broader trends, drivers and systems that enable their emergence and spread are essential for long-term effectiveness in handling COVID-19 and other emerging infectious diseases (EIDs).
Context, systems and trends linked to EIDs
Several studies suggest that for the past half-century, with trends beginning in the 1940s, the risk of EIDs, particularly zoonotic diseases, has been rising over time as a result of ecosystem changes (as measured by the frequency and richness of zoonotic disease outbreaks and the number of the first occurrence of zoonotic EIDs “events”) (Baudron and Liégeois, Reference Baudron and Liégeois2020; Cunningham et al., Reference Cunningham, Daszak and Rodríguez2003; B. A. Jones et al., Reference Jones, Grace, Kock, Alonso, Rushton, Said, McKeever, Mutua, Young, McDermott and Pfeiffer2013; K. E. Jones et al., Reference Jones, Patel, Levy, Storeygard, Balk, Gittleman and Daszak2008; Keesing et al., Reference Keesing, Belden, Daszak, Dobson, Harvell, Holt, Hudson, Jolles, Jones, Mitchell, Myers, Bogich and Ostfeld2010; Ostfeld, Reference Ostfeld2009; Rohr et al., Reference Rohr, Barrett, Civitello, Craft, Delius, DeLeo, Hudson, Jouanard, Nguyen, Ostfeld, Remais, Riveau, Sokolow and Tilman2019; Smith et al., Reference Smith, Goldberg, Rosenthal, Carlson, Chen, Chen and Ramachandran2014; Taylor et al., Reference Taylor, Latham and Woolhouse2001; Weiss and McMichael, Reference Weiss and McMichael2004). The rise of EIDs has been established after controlling for biased reporting of outbreaks over time. However, after controlling for internet usage, outbreak cases per capita appear to be declining over time. In combination, these findings suggest that while we are becoming more effective at reducing the number of infected people through early detection, control and treatment, the impact of human activities on wildlife is simultaneously increasing the risk of spill-overs and new infectious-disease outbreaks (K. E. Jones et al., Reference Jones, Patel, Levy, Storeygard, Balk, Gittleman and Daszak2008; Smith et al., Reference Smith, Goldberg, Rosenthal, Carlson, Chen, Chen and Ramachandran2014). Furthermore, in the long term, efforts to quickly mitigate infectious diseases may not be sufficient to compensate for the impact of ecological changes in promoting their emergence.
It is estimated that most EID outbreaks in the human population, as many as 70% are zoonoses – diseases caused by a spill-over of pathogens from animal hosts to humans (K. E. Jones et al., Reference Jones, Patel, Levy, Storeygard, Balk, Gittleman and Daszak2008; Taylor et al., Reference Taylor, Latham and Woolhouse2001). The influence of socio-economic, environmental and ecological changes, including forest loss and fragmentation, agricultural encroachment, development and irrigation, human and livestock demographics, antibiotic use, wildlife hunting and trade and climate change, on infectious-disease emergence and spread is difficult to disentangle due to the complex webs of interactions and feedback loops. While not all anthropogenic changes impact infectious-disease emergence and transmission, a significant amount has been showcased to influence it. A systematic review of 305 scientific articles investigating how specific types of anthropogenic land use change influence infectious-disease dynamics found that more than half of the studies (56.9%) documented an increase in pathogen transmission, whereas 10.4% of the studies observed a decrease in pathogen transmission (Gottdenker et al., Reference Gottdenker, Streicker, Faust and Carroll2014). Agricultural intensification and intensive livestock production, resulting in land-use change, deforestation, habitat fragmentation and antimicrobial resistance, is estimated to be the primary driver of infectious-disease risk (Allen et al., Reference Allen, Murray, Zambrana-Torrelio, Morse, Rondinini, Di Marco, Breit, Olival and Daszak2017; Baudron and Liégeois, Reference Baudron and Liégeois2020; Cunningham et al., Reference Cunningham, Daszak and Rodríguez2003; Keesing et al., Reference Keesing, Belden, Daszak, Dobson, Harvell, Holt, Hudson, Jolles, Jones, Mitchell, Myers, Bogich and Ostfeld2010; Olson et al., Reference Olson, Benedum, Mekaru, Preston, Mazet, Joly and Brownstein2015; Rohr et al., Reference Rohr, Barrett, Civitello, Craft, Delius, DeLeo, Hudson, Jouanard, Nguyen, Ostfeld, Remais, Riveau, Sokolow and Tilman2019).
However, there are barriers to which such trends can be accurately quantified, including limits of available zoonotic disease datasets, difficulties in disaggregating the probability of occurrence from the probability of detection and reporting and spatial-temporal heterogeneity in health surveillance. While measures to control for confounding factors have also been used (e.g., press freedom and internet usage, academic publication output, population size and density and GDP per capita), the validity of these has not been proven.
Nevertheless, even without including quantitative analyses, the correlations between anthropogenic changes and emerging infectious diseases have also been demonstrated through a wide range of case studies and individual analyses over the last two decades. For instance, the emergence of the Nipah virus in Malaysia was linked to the establishment of a sizable intensive pig farm combined with fruit trees within the foraging range of two roots of Nipah virus-infected fruit bats (B. A. Jones et al., Reference Jones, Betson and Pfeiffer2017); schistosomiasis transmission has been repeatedly linked to agricultural expansion, including dam construction and agrochemical pollution (Hoover et al., Reference Hoover, Rumschlag, Strgar, Arakala, Gambhir, de Leo, Sokolow, Rohr and Remais2020; Sokolow et al., Reference Sokolow, Jones, Jocque, La, Cords, Knight, Lund, Wood, Lafferty, Hoover, Collender, Remais, Lopez-Carr, Fisk, Kuris and de Leo2017); irrigation of desert land has been shown to lead to a dominance of Phlebotomus papatisi, a highly competent vector for visceral leishmaniasis (Garchitorena et al., Reference Garchitorena, Sokolow, Roche, Ngonghala, Jocque, Lund, Barry, Mordecai, Daily, Jones, Andrews, Bendavid, Luby, Labeaud, Seetah, Guégan, Bonds and De Leo2017). Climate change has also been correlated to a range of natural hazards, including floods, tropical cyclones, droughts and heatwaves, which in turn impact outbreaks of climate-sensitive infectious diseases (water-borne and vector-borne diseases) (Alcayna et al., Reference Alcayna, Fletcher, Gibb, Tremblay, Funk, Bhargavi and Lowe2022).
Hence, COVID-19 and infectious diseases more broadly should not be tackled as isolated health events, as they often result from economic and political systems that rely on unsustainable exploitation of resources and create the ecological and environmental conditions necessary to drive their emergence.
Purpose of this study
Considering the previous research on the context, systems and drivers linked to the emergence of infectious diseases, this study evaluates whether past and current warning and response systems account for these conditions and are thus suitable to prevent and respond to EIDs. It aims to provide an overview of the global trends and gaps in warning and response systems for infectious diseases, pinpoint areas of focus and identify opportunities to improve these systems worldwide.
The study enquires whether there is a failure in current warning systems to frame and address EIDs in ways that integrate human, animal and ecosystem health. It goes on to discuss the value, potential and limitations of monitoring and addressing the driving conditions that increase the risks of new and re-emergent infectious diseases. Finally, it discusses future directions to facilitate cross-sectoral governance and enhance the capabilities of warning and response systems to monitor and address EIDs drivers.
Methodology
This study is based on a mixed-method approach combining elements of a critical and mapping review (Grant and Booth, Reference Grant and Booth2009). As with a critical review, this study extends beyond merely describing identified articles; it incorporates analysis and conceptual innovation, developing unique categories for classifying warning systems. The methodology also borrows from the mapping review approach, aiming to contextualise the breadth of the literature, identify evidence gaps within warning systems and highlight key insights relevant to policy and practice (Grant and Booth, Reference Grant and Booth2009). The combination of these two methods allowed for a comprehensive exploration of the grey literature, including reports, conference documents, websites and articles, in addition to scientific articles. This is an indispensable element in this study given the nature of warning systems, many of which are informal and thus underrepresented in scientific journals.
The research was based on a combination of the following keywords: “early warning systems,” “warning systems,” “infectious diseases,” “health warning systems,” “pandemic,” “surveillance,” “alerts,” “notification,” “risk assessment,” “epidemic responses,” “preparedness,” “prevention,” “environmental health,” “ecological factors,”, “drivers” and “driving forces.” The review further targeted specific scales of analysis, identifying “local/community-based,” “regional,” “national” and “international” warning systems. The papers were extracted from Google Scholar, PubMed, Scopus, Google and Safari databases. The research often followed a “snowballing” approach, extracting further resources from the already found ones, per Sayers’s definition (Reference Sayers2007).
This research resulted in the identification, analysis and categorisation of 64 warning and response systems for infectious diseases. Warning systems were divided into four categories representative of their overarching objective and function: Reactive, Prevention-centred, Anticipatory and Preparedness-centred. Each system was carefully examined and sorted into a category based on several criteria, including data sources, surveillance methods, risk assessments, alert thresholds, communication platforms, forecasting methods and action mechanisms.
While not all systems included in the database are named or labelled as “early warning systems” or “warning system,” they align with the UNDRR’s latest definition of an EWS, as applied in the field of disaster risk reduction (2017). Specifically, each has a feedback loop where some surveillance mechanism leads to an alert, risk assessment and/or action protocol. This core functionality forms the key inclusion criteria to classify or not a system as a warning and response system and include it in the database. Surveillance alone, which is purely defined as monitoring and observation, does not in itself constitute a warning and response system. The database includes warning and response systems for animal and human infectious diseases, several of these systems integrating both. All warning systems identified from both grey and scientific literature, included in the database were confirmed to be operational or to have been operational in the past, rather than being theoretical models.
Categories
Reactive/passive warnings: disease surveillance
Reactive warning systems do not monitor the drivers or factors leading to new or re-emergent infectious diseases, but only the diseases themselves, often only once they have reached the human populations, but also, at times, the animal populations (Morin et al., Reference Morin, Semenza, Trtanj, Glass, Boyer and Ebi2018). They can be correlated with strategies that predict the further spread of viruses and passive/reactive strategies dedicated to stopping and reducing further transmission.
Prevention-centred warnings: surveillance of driving forces and proposed actions to prevent re-occurring spill-overs
Prevention-centred warning systems monitor the driving factors leading to spill-over events and increased infectious-disease emergence, such as environmental, social and climatic factors. They are connected to preventative strategies which aim to reduce the risk of spill-overs. They can also be used to predict future spill-overs and outbreaks to improve efforts for early detection and response once a disease has reached the human or animal populations.
Anticipatory warnings: surveillance of driving forces to anticipate new outbreaks and reduce disease spread
Similar to prevention-centred warnings, anticipatory warning systems are characterised by monitoring the drivers leading to spill-overs of infectious diseases. However, these warning systems are generally not linked to preventative strategies aiming to address the drivers leading to spill-overs of new or re-emergent infectious diseases. The information collected is mainly used to anticipate, that is, predict and detect future outbreaks more rapidly to initiate reactive strategies dedicated to stopping their spread.
Preparedness-centred warnings: monitoring and assessment of public health sector capabilities
Preparedness-centred warnings assess the health sector’s capabilities, or more broadly, the countries’ capabilities to monitor and respond to future outbreaks, identifying critical gaps and opportunities for improvement. They are linked to preparedness strategies aiming to improve responses to future outbreaks, such as training, improving information networks and platforms of communication, and capacity-building strategies, i.e., strengthening laboratory systems, reporting, medical countermeasures, personnel deployment, etc.
Even though other studies have also categorised warning systems as a method for analysis (Day and Fearnley, Reference Day and Fearnley2015; Nichols et al., Reference Nichols, Andersson, Lindgren, Devaux and Semenza2014), this same categorisation structure has yet to be used. While the database is not a complete assessment of all the warning systems involved or linked to infectious disease, it provides an overall perspective of the focus of warning systems, particularly at international and national levels. See “Limitations” section in this study for further information, and Figure 4a and b in Section “The value, potential of and limitations in creating prevention-centred warning and response systems” for visual representation.
Results
Analysis of EIDs warning and response systems
Overall, most warning systems (80%) within the database followed a reactive approach, also known as a passive approach (Morin et al., Reference Morin, Semenza, Trtanj, Glass, Boyer and Ebi2018). They monitor the threat only once it has reached a detectable threshold within the population and can only be linked with reactive strategies aiming to reduce disease transmission. Examples of reactive warning systems include Health Map (Bhatia et al., Reference Bhatia, Lassmann, Cohn, Desai, Carrion, Kraemer, Herringer, Brownstein, Madoff, Cori and Nouvellet2021) or Epitweetr (Espinosa et al., Reference Espinosa, Wijermans, Orchard, Höhle, Czernichow, Coletti, Hermans, Faes, Kissling and Mollet2021), which use informal internet data sources to detect potential health threats, such as monitoring tweets by time, place and topic, as well as larger organisations and more formal systems, such as WHO’s Global Outbreak Alert and Response Network (Christensen et al., Reference Christensen, Fisher, Salmon, Drury and Effler2021; Mackenzie et al., Reference Mackenzie, Drury, Arthur, Ryan, Grein, Slattery, Suri, Domingo and Bejtullahu2014; Sondorp et al., Reference Sondorp, Ansell, Stevens and Denton2011) which monitors acute public health events, deploying staff and resources to the affected countries. While reactive warning systems that monitor the disease once it has reached the human population to reduce its spread, e.g., the United States COVID-19 Forecast Hub (Cramer et al., Reference Cramer, Huang, Wang, Ray, Cornell, Bracher, Brennen, Rivadeneira, Gerding, House, Jayawardena, Kanji, Khandelwal, Le, Mody, Mody, Niemi, Stark, Shah and Reich2022), Global Influenza Surveillance and Response System (WHO, 2020) and during humanitarian emergencies, e.g., Red Cross, EWARS and EWARN; (Cambodian Red Cross, 2016; Mala et al., Reference Mala, Abubakar, Takeuchi, Buliva, Husain, Malik, Tayyab and Elnoserry2021), are needed, they only address one aspect of infectious-disease risk (Figure 1).
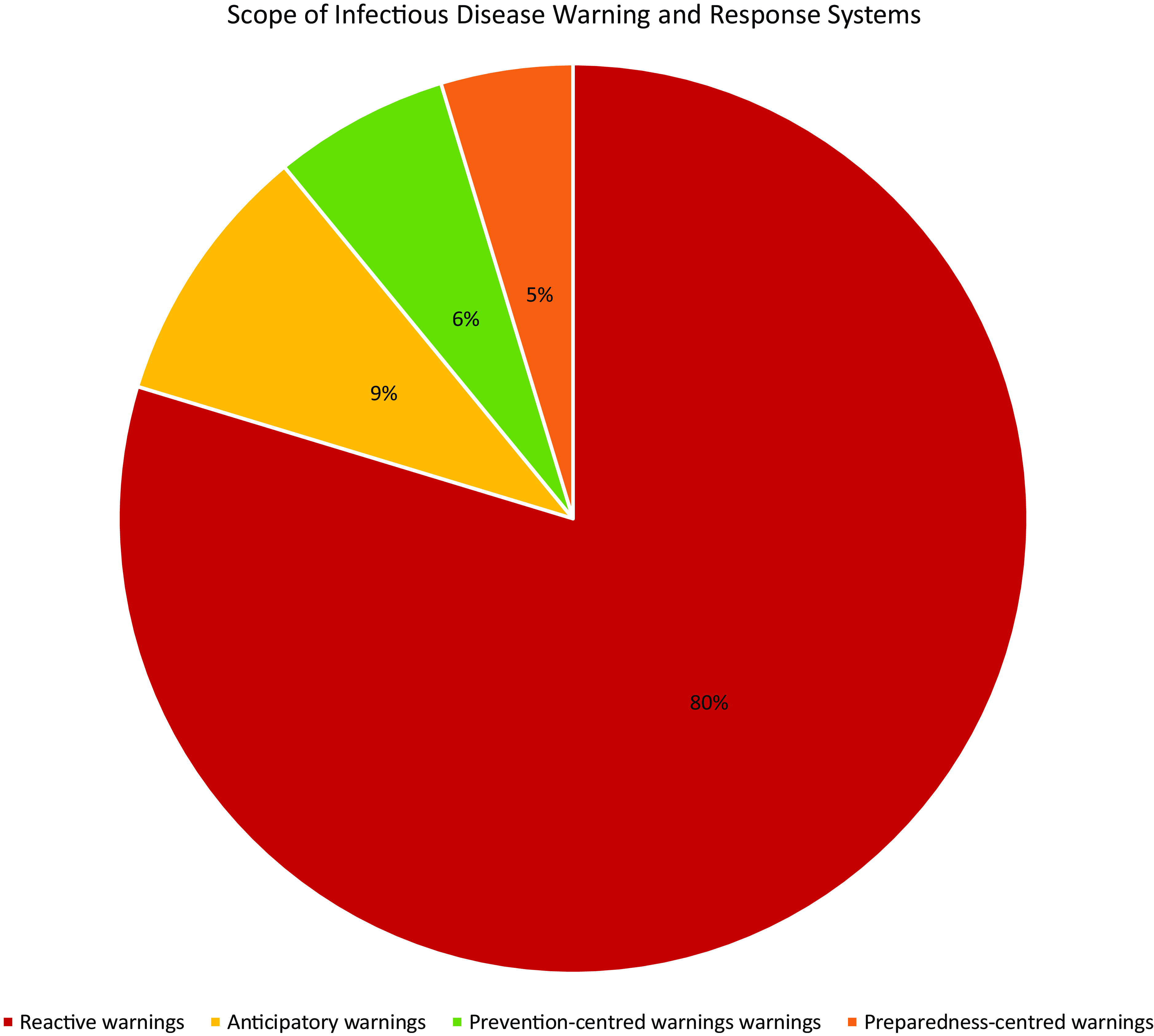
Figure 1. Scope of infectious-disease warning and response systems. Reactive/passive warnings: 80%, 52/65 warning and response systems.
Anticipatory warnings: 9%, 6/65 warning and response systems.
Prevention-centred warnings: 6%, 4/65 warning and response systems.
Preparedness-centred warnings: 5%, 3/65 warning and response systems (Fernandez de Cordoba Farini, 2023).
In comparison, anticipatory warning systems (9%) and prevention-centred warning systems (6%) that monitor the socio-economic, environmental and ecological conditions leading to increasing spill-overs are limited. In addition, only a few warning and response systems took a multi-hazard, multi-sectoral approach that jointly evaluated various hazards, their interdependencies and cascading impacts. Nevertheless, a few examples of prevention-centred warning systems from which we can learn exist, and these will be explored later in the paper.
In addition, few warning and response systems were linked to preparedness and capacity-building strategies (5%). Nevertheless, it is essential to be cautious when interpreting these findings. Any set-up warning system is in itself considered a preparedness measure, and efforts towards evaluating and improving public health sector capabilities are not often measured or constructed in the form of an interactive monitoring and warning system and therefore do not feature in this study. Furthermore, based on the literature review, the COVID-19 pandemic appears to have resulted in a significant push towards preparedness. This focus is apparent throughout various strategies and policy documents following the COVID-19 pandemic. Examples include WHO’s Independent Panel for pandemic preparedness and response (2020) (Sirleaf and Clark, Reference Sirleaf and Clark2021), Biden’s administration report on “American Pandemic Preparedness Transforming Our Capabilities” (The White House US Government, 2021), the UK government’s Partnership on Pandemic Preparedness (UK Government, 2021), the G20 panel on “Financing the Global Commons for Pandemic Preparedness and Response” (G20 High Level Independent Panel, 2021). All these strategies are centred around strengthening the capabilities of the human health sector (e.g., vaccine manufacturing, test and tracing, diagnostics etc.) to respond to and contain a public health crisis; no significant objectives or commitments to address the upstream driversFootnote 1 of infectious diseases are mentioned.
The emphasis and shift towards prevention are yet to be realised and integrated with public health measures, despite substantial research and evidence showcasing its critical importance (Figure 2).
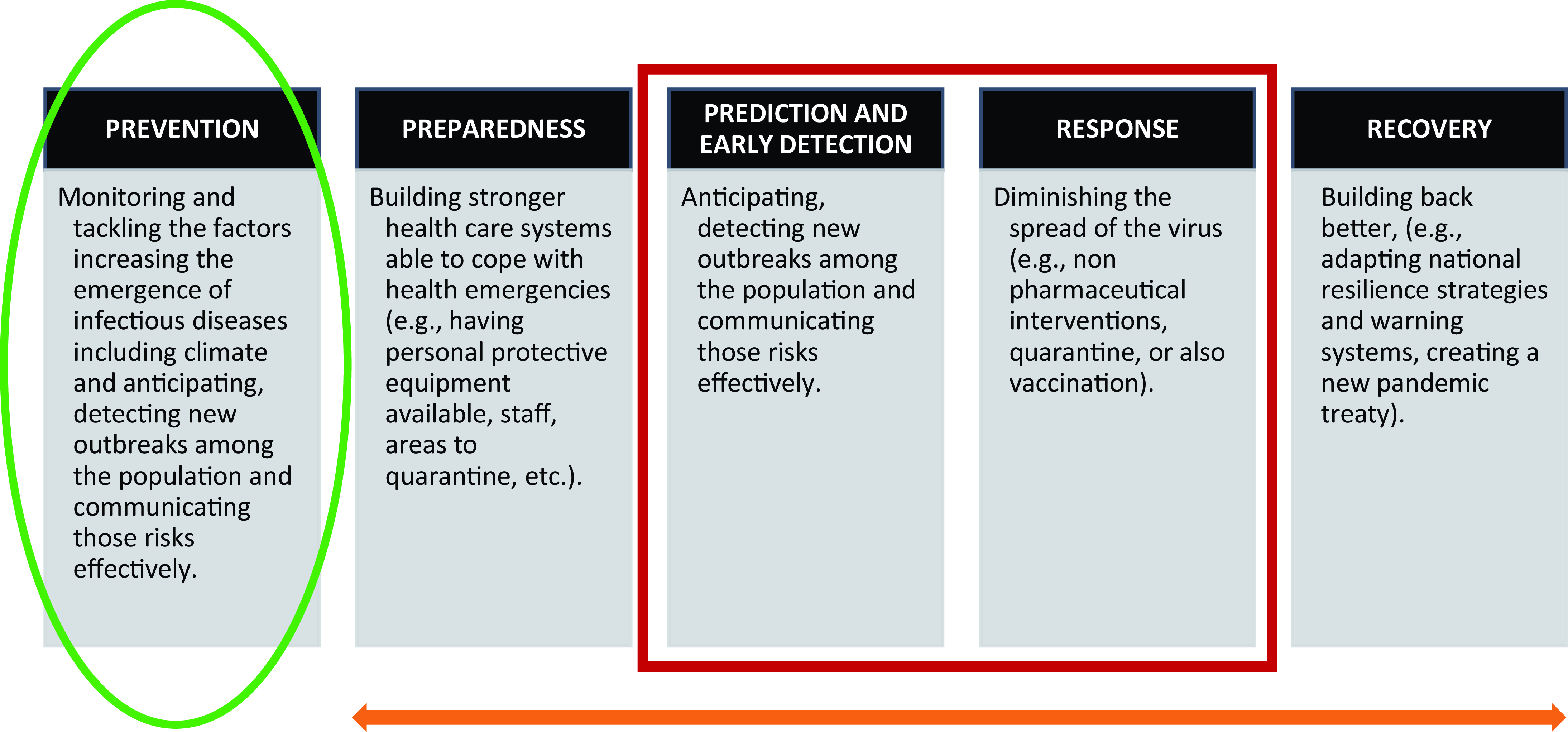
Figure 2. Components involved in responding to emerging infectious diseases, a simplified analysis of the current status quo. Primary focus of infectious-diseases strategies, warning and response systems.
Secondary focus as a result of the COVID-19 pandemic.
Missing links to strategies focused on prevention (Fernandez de Cordoba, 2023).
The links between equitable cross-disciplinary collaboration and EID prevention
A critical issue which explains the lack of prevention and anticipatory strategies is the need for multi-sectoral collaboration, particularly between public and environmental health sectors. Currently, most warning systems available for infectious diseases are designed by human health specialists, not veterinarians and particularly not ecologists. The focus on public health shapes how the problem is conceptualised and narrows the type of responses that can be taken. This is simply and clearly illustrated by Kenyon’s “schematic representation of the contrast between ecological and biomedical individualist conceptual frameworks for understanding the origins of SARS-CoV-2 and other zoonoses” (Kenyon, Reference Kenyon2020, p. 3). Warnings systems led by public health practitioners fail to account for the links between environmental and health issues, limiting the extent to which preventative and anticipatory strategies are deployed (Figure 3).

Figure 3. Schematic representation of the contrast between ecological (left) and biomedical individualist (right) conceptual frameworks for understanding the origins of SARS-CoV-2 and other zoonoses. Figure from Kenyon (Reference Kenyon2020).
One of the most prominent interdisciplinary frameworks looking to respond to zoonotic diseases is One Health. In principle, One Health pushes forward inclusivity, collaboration and multi-disciplinarity between frameworks. In practice, the responses issued as One Health have been limited by an anthropocentric hierarchy, e.g., WHO’s One Health agenda has traditionally been defined as “an approach to designing and implementing programmes […] in which multiple sectors communicate and work together to achieve better public health outcomes” (World Health Organisation, n.d.). The problem with anthropocentrism is that it can lead to a simplification of the interdependencies between humans, animals and the environment.
The risks of solely focusing on human interests in infectious-disease responses were seen during the H1N1 pandemic in Egypt. Political and religious narratives stigmatised pigs and supported a cull of 300,000 pigs under the pretext of safeguarding public health (Davis and Sharp, Reference Davis and Sharp2020; Leach and Tadros, Reference Leach and Tadros2014). However, pigs were used by a religious minority, the Zabbaleen, to sort through much of Cairo’s waste (Fahmi and Sutton, Reference Fahmi and Sutton2010). The culling led to a substantial urban waste problem, with people resorting to secretly disposing of garbage in irrigation channels and illegal dumping sites, leading to a range of public and environmental health issues. The focus on contamination and framing of animals as diseases failed to account for the agency and the positive impact animals had on human lives. Similarly, Kamenshchikova et al. (Reference Kamenshchikova, Wolffs, Hoebe and Horstman2021) criticised OH as conducive to hierarchical collaborations in international responses to AMR. They argued that anthropocentric framings fail “to create a discursive space for exploring and acting upon the different values at stake in antimicrobial resistance prevention” (Kamenshchikova et al., Reference Kamenshchikova, Wolffs, Hoebe and Horstman2021, p. 8). Furthermore, an analysis of 184 Global One Health networks (OHNs) (Mwatondo et al., Reference Mwatondo, Rahman-Shepherd, Hollmann, Chiossi, Maina, Kurup, Hassan, Coates, Khan, Spencer, Mutono, Thumbi, Muturi, Mutunga, Arruda, Akhbari, Ettehad, Ntoumi, Scott, Nel, Ellis-Iversen, Sönksen, Onyango, Ismail, Simachew, Wolking, Kazwala, Sijali, Bett, Heymann, Kock, Zumla and Dar2023) found that only 60% of them included the three sectors (human health, animal health and the environment or ecosystems), with the environmental sector being the most excluded. They note that over the last 15 years, the focus has been on “health security priorities” with “little regard” for the “upstream drivers of disease emergence” “or other crucial hazards and health emergencies” (Mwatondo et al., Reference Mwatondo, Rahman-Shepherd, Hollmann, Chiossi, Maina, Kurup, Hassan, Coates, Khan, Spencer, Mutono, Thumbi, Muturi, Mutunga, Arruda, Akhbari, Ettehad, Ntoumi, Scott, Nel, Ellis-Iversen, Sönksen, Onyango, Ismail, Simachew, Wolking, Kazwala, Sijali, Bett, Heymann, Kock, Zumla and Dar2023, p. 609).
The hierarchy assigned to One Health priorities has proven detrimental to all three sectors, including human health. Equitable collaboration is needed to create healthy interactions and feedback loops between species and maintain a sustainable environment. The inclusion of the United Nations Environment Programme (UNEP) in the Quadripartite – a coalition with the World Health Organisation (WHO), the World Animal Health Organisation (WOAH) and the Food and Agriculture Organisation (FAO) – marks a significant stride towards this objective. In 2021, the One Health High-Level Expert Panel, a body of experts from these organisations, put forth an updated One Health definition (Nzietchueng et al., Reference Nzietchueng, Kitua, Nyatanyi and Rwego2023). This new interpretation highlights principles of equity, stewardship and balance and acknowledges crucial elements such as clean water, energy, air, nutritious food and climate change action. This expanded scope represents a substantial shift in One Health, from its traditional focus on infectious-disease transmission and human and veterinary medicine to adopt a more equitable, humble perspective of the natural world wherein the welfare of non-human animals and the environment is accorded the same significance as human welfare. However, environmental determinants of health are yet to be thoroughly understood by all sectors. The Quadripartite’s One Health Joint Plan of Action identified environmental integration as one of six vital action tracks for the coming years (Jesudason, Reference Jesudason2022; “One Health Joint Plan of Action, 2022–2026,” 2022). The subsequent section will explore various examples and case studies demonstrating how to achieve this integration, especially in the context of warning and response systems.
The value, potential of and limitations in creating prevention-centred warning and response systems
For warning systems to work more equitably across sectors and subsequently result in preventative strategies, they need to consider and address the drivers leading to the emergence of infectious diseases. This paper reviews existing frameworks, approaches and warning systems focused on the drivers of EIDs and prevention. It analyses their benefits, potential and limitations.
In 2015, Olson et al., envisaged what a driver-centric surveillance system could look like compared to traditional disease surveillance and detection systems (Olson et al., Reference Olson, Benedum, Mekaru, Preston, Mazet, Joly and Brownstein2015). This paper reviews their theoretical framework, linking it to practical examples and existing warning systems to assess the status and progress towards reaching that framework. Olson et al., portrayed a traditional disease detection surveillance system (see Figure 4a) as a simple association between the number of cases and a digital disease signal which acts as a warning. These types of surveillance systems lead to what has been termed in this paper as a reactive warning or approach. They can be used to control and mitigate the transmission of diseases only once they have reached the human population. Examples of this type of approach could be Geo-sentinel (Hamer et al., Reference Hamer, Rizwan, Freedman, Kozarsky and Libman2021), a warning system which tracks disease among travellers and releases reports to control and avoid the transmission of health threats cross-borders, or IHRs, which issues warnings for public health events of potential international concerns such as an Ebola epidemic.

Figure 4. (a) Linking Theory and Practice – Adaptation from Olson et al., figure 1, graph A, from paper “Drivers for emerging infectious diseases as a framework for Digital Detection.” (b) Linking Theory and Practice – Adaptation from Olson et al., figure 1, graph C from paper “Drivers for emerging infectious diseases as a framework for Digital Detection.”
In contrast to a traditional disease detection system, according to Olson et al., a driver-centric surveillance system would monitor the drivers leading to the emergence of diseases such as climate, weather, economic development and poverty (Olson et al., Reference Olson, Benedum, Mekaru, Preston, Mazet, Joly and Brownstein2015). Hence, driver-centric surveillance could result in anticipatory warnings that shorten the time of disease detection resulting in early action and mitigation strategies. An example of an anticipatory warning system is the Columbia university framework for a Malaria Early Warning system which was used to forecast malaria epidemics based on seasonal climate forecasts, weather monitoring and case surveillance (Grover-Kopec et al., Reference Grover-Kopec, Kawano, Klaver, Blumenthal, Ceccato and Connor2005; Thomson et al., Reference Thomson, Indeje, Connor, Dilley and Ward2003). Another example is PREDICT I, which collected information on disease drivers to strengthen country-level capacities for routine infectious-disease detection and outbreak response (Joly et al., Reference Joly, Johnson, Goldstein, Anthony, Karesh, Daszak, Wolfe, Murray and Mazet2016). Driver-centric surveillance systems could also result in Prevention-centred warnings that identify and reduce risk factors leading to infectious-disease emergence. An example of an already existing driver-centric and prevention-centred warning system is the European Environment and Epidemiology Network (Semenza, Reference Semenza2015; Semenza and Paz, Reference Semenza and Paz2021), whose approach is discussed later in the paper. Another initiative which is being created as prevention-centred is IDAlert. IDAlert aims to develop new climate and health indicators of disease drivers to strengthen monitoring mechanisms, provide innovative early warning systems that integrate the environment and inform policy development in human, animal, and environmental sectors (IDAlert, 2022).
While there are scarce streamlined approaches which collect and jointly analyse data across sectors to evaluate EIDs’ risk, relevant data sets exist separately across these sectors. However, these have not necessarily been conceptualised to be used and analysed jointly. For instance, the FAO–WOAH–WHO Global Early Warning System (GLEWS+) – a tool designed to handle health threats and emerging risks at the human–animal–ecosystems interface – has customarily not effectively monitored and integrated information on environmental drivers of disease emergence and transmission in its early warning and intelligence efforts (Quadripartite, 2022). The lack of integration of environmental health insights limits the scope and effectiveness of subsequent responses for EIDs developed based on that intelligence. The integration of the UNEP provides an opportunity to address this gap and leverage the range of information and databases the UNEP reports on e.g., trends in biodiversity, ecosystem health and environmental health (UN Biodiversity Lab), international wildlife trade per CITES (the Convention on International Trade in Endangered Species of Wild Fauna and Flora), the Global Forest Watch and CLIMsystems a climate insights data portal.
Similarly, a range of data sources and information from different sectors including human (e.g. the WHO and the European Centre for Disease prevention and Control (ECDC) data repositories, the Global Health Security Index, the Institute for Health Metrics and Evaluation (IHME) Global Burden of Disease, the Global Health Observatory, Our World in Data, government resources, etc.), animal (FAO, WOAH, Action for Animal Health data repositories etc.) and environmental sectors (IPCC report, World Bank data repository, Databases from Sustainable Development Goals Lancet Countdown, OECD Stat, Environmental Performance Index, Global Ocean Health Index scores, UNEP, etc.) exist, particularly in developing countries. It is essential to further investigate the interconnections among these databases and information systems and explore how they can be leveraged to foster cross-sector initiatives and multi-sectoral warning and response systems.
Cross-sector collaboration is critical to optimise the use of the diverse data collected, offering a more integrated approach to addressing complex problems. For instance, the smartphone application Ethiopian differential diagnosis information environment is a diagnosis system, developed by The University of Strathclyde, Addis Ababa University and Brooke, to help veterinarians and veterinary paraprofessionals determine the most likely diagnosis affecting an animal and, therefore, helping them decide the most appropriate treatment (Beyene et al., Reference Beyene, Asfaw, Getachew, Tufa, Collins, Beyi and Revie2018). The system is beneficial when access to laboratory diagnosis is not available. While it provides a form of passive animal health surveillance data, the system is currently not being leveraged as a warning and response system. However, the data collected could, in the future, have much broader applications. It could be used to analyse what recurrent diseases and systemic issues the country is most likely facing and issue alerts. If sufficient environmental data was collected, it could be used to determine which environmental factors are driving these diseases and take preventative, earlier action. Similarly, the system could be used to assess “cold spots,” areas where there is a lack of monitoring of disease outbreaks, and subsequently, potential health risks are not being evaluated. This demonstrates opportunities for expanding the application of existing information systems to function as warning systems, including prevention-centred warnings. Thus, developing an effective driver-centric or prevention-centred warning system will not always require collecting data from scratch but rather mapping existing data sources that could be taken advantage of and gaps where there is a need to collect further information.
Furthermore, collaborative platforms exist from which we can learn, such as the European Environmental Epidemiology Network (E3 Network). For example, E3 data was used to stop and prevent the continuous emergence of malaria in Greece (Semenza, Reference Semenza2015). From 2009–2012, Greece experienced re-emergent malaria cases, despite control and endemic elimination programmes (Sudre et al., Reference Sudre, Rossi, Van Bortel, Danis, Baka, Vakalis and Semenza2013). To help guide malaria control efforts, the E3 network attempted to identify areas suitable for malaria emergence and transmission. Geo-referenced climatic and environmental information from Greece and other data sources were retrieved and processed for spatial modelling, such as seasonal variations of vegetation, land cover categories, temperature, etc. These data sources were used to characterise the climatic and environmental profiles of locations with active transmission cycles to predict other sites at risk of malaria re-emergence in Greece. For example, among several other factors, areas suitable for transmission had warmer temperatures and intensive irrigated agriculture. Based on that information, the E3 Network produced a disease risk map, which they shared with Greek public health practitioners responsible for preparedness and response activities. This combined approach enabled the disruption of malaria transmission in 2013 through targeted entomological and epidemiological surveillance, vector control activities and rising awareness among the public and health workers of the areas that are environmentally suitable for transmission. The E3 Network’s capacity to interrupt malaria transmission showcases how collaboration across disciplines can help reduce spillover events and prevent the continuous rise of EIDs. A crucial step, if viable, is to harness data from anticipatory warning systems to confront the root causes, effectively transforming these systems into ones centred on prevention. While the E3 network’s approach is mainly based in European countries, other joint strategies, such as the Shanghai Multi-hazard Warning System (Tang et al., Reference Tang, Feng, Zou and Mu2012) or PulseNet (Ribot et al., Reference Ribot, Freeman, Hise and Gerner-Smidt2019) exist internationally that can be used as reference models to advocate and guide collaboration efforts in the prevention of EIDs.
Nevertheless, issues hindering the integration of datasets will need to be addressed. “The effects of global environmental change do not adhere to typical effect-response relationships which traditional epidemiological methods have been refined (and perfected) to measure” (Semenza, Reference Semenza2015, p. 6336), but they are often based on complex pathways which can be direct but also often indirect, diffused (impacts are not constant), or delayed (Mcmichael, Reference Mcmichael2013; Olson et al., Reference Olson, Benedum, Mekaru, Preston, Mazet, Joly and Brownstein2015). Whether is it to develop scenario-based models that account for those complexities or to create real-time multi-sectoral warning and response systems, several issues in integrating datasets demand attention. Firstly, navigating issues related to data access and ownership is critical. Crafting data-sharing agreements, though demanding, can facilitate collaboration among stakeholders from different departments or organisations, enabling data sharing and access. Nevertheless, even after that first step issues with data compatibilityFootnote 2 , temporal resolutionFootnote 3 and spatial scaleFootnote 4 will need to be addressed for the data to be effectively integrated (Olson et al., Reference Olson, Benedum, Mekaru, Preston, Mazet, Joly and Brownstein2015; Semenza, Reference Semenza2015). Moreover, in some cases, such as with GLEWS+, direct data sharing is not feasible. In order to circumvent this issue, the quadripartite has developed agreements to share key insights derived from the data, rather than the raw data itself (Quadripartite, 2022). This approach proves particularly relevant in ensuring proper contextualisation and interpretation of the information, as experts from different disciplines adhere to varying standards for data collection, quality and time frames. Whether sharing data or the derived insights, effective communication of the assumptions surrounding the information, including limitations, expectations and appropriate usage, is critical. This transparent approach ensures the success of cross-sectoral warning and response systems and prevents potential unintended consequences that may arise from barriers in language and understanding across different disciplines.
Facilitating cross-sectoral governance
Establishing prevention-centred warning systems requires changes in governance that foster equitable engagement within and across sectors, hazards and organisations. Internationally, organisations like the World Bank, the UN, the WHO, the FAO and WOAH possess well-defined mandates that delineate their responsibilities and the scope of their work. Similarly, government departments also tend to address human, animal and environmental health as separate concerns. However, these divisions can result in fragmented decision-making and responses, limiting the scope and effectiveness of warning and response systems. Several recommendations are presented underneath to address these silos and strengthen interagency and multi-sectoral collaborations at the international and national levels.
First, addressing silos across international agreements stands as a pivotal approach to achieving this objective. For instance, despite the clear links between health and natural hazards, the UN’s 2022–2027 Early Warning for All InitiativeFootnote 5 – aiming for universal protection from hazardous meteorological events – has yet to reference or integrate health and infectious diseases within its objectives and strategy (World Meteorological Organization, 2022; Alcayna et al., Reference Alcayna, Fletcher, Gibb, Tremblay, Funk, Bhargavi and Lowe2022). This represents a gap that if addressed correctly could result in more effective cross-sectoral warnings at the international, national, regional and local levels, that account for the drivers and cascading impacts of climate and meteorological hazards. Similarly, the new pandemic accord currently under negotiation by WHO member states needs to strive to be aligned and integrated with other international agreements and strategies addressing EID risks, including the Convention to Combat Desertification, the Convention for Biological Diversity (CBD), the Convention on International Trade in Endangered Species of Wild Fauna and Flora (CITES) and the United Nations’ Early Warnings for All Initiative. Although not originally designed to reduce EIDs, these agreements significantly influence EID risk, and their interconnections should be acknowledged and utilised.
Furthermore, despite the unique mandates of international organisations, it is essential to incorporate shared multi-sectoral objectives into the international agreements they spearhead, to prevent international compartmentalisation from fostering national divisions. The 2022 Kunming-Montreal Global Biodiversity Framework (GBF) serves as an example of good practice, exemplifying the promotion of cross-sectoral collaboration and the establishment of unified objectives. Historically, health-focused civil society organisations have shown limited participation in the CBD and its negotiations (Willetts, Comeau, et al., Reference Willetts, Comeau, Vora, Horn, Studer, Martin, Lem, Pétrin-Desrosiers, Grant and Webb2023). However, this trend has been changing and in 2022 five such organisations became accredited and attended the agenda-setting and decision-making meetings at the 15th UN Biodiversity Conference (COP 15). As a result, several of the targets of the GBF integrate health and biodiversity considerations such as “reduction of disease […] through nature-based solutions” (target 11), or “Ensure that the use, harvesting and trade of wild species is sustainable, safe and legal […] reducing the risk of pathogen spill-over” (target 5). The commitment of 186 states to revise their national biodiversity strategies and action plans (NBSAPs) by 2024, guided by these targets, presents an opportunity to strengthen integrated decision-making, policy coordination and cross-sectoral collaboration, particularly concerning the linkages between biodiversity and health (Willetts, Siege, et al., Reference Willetts, Siege, Stewart-Ibarra, Horn, Chotthong, Tanawat, Omido, Sharma, Alqodmani, Bennett, Golden, Wangari Githaiga and Vora2023). Valuable insights can be drawn from the recent integrated decision-making efforts of the CBD to support the development of integrated strategies and targets in other agreements, such as the pandemic accord and the Early Warning for All Initiative.
Moreover, in addition to strengthening multi-sectoral cooperation in international agreements; mechanisms to facilitate collaboration at the national level need to be further developed to prevent fragmented responses. For instance, the international health regulations (IHR) set obligations for countries to detect and report a potential public health event of international concern. However, the responsibility often falls solely within the public health department resulting in siloed approaches, as showcased by the survey of IHRs National Focal Points (NFPs) experiences in carrying out their functions (2021) which found that one key challenge was “insufficient inter-sectoral collaboration within their countries, including limited access to, or a lack of cooperation from, key relevant ministries” (Packer et al., Reference Packer, Halabi, Hollmeyer, Mithani, Wilson, Ruckert, Labonté, Fidler, Gostin and Wilson2021, p. 1). To address this gap, an obligation that could be implemented is to designate One Health NFPs, that coordinate across all relevant ministries to address complex transdisciplinary issues such as pandemic prevention. These should communicate with existing NFPs as part of the IHRs. Support for this new objective is prevalent across various civil societies and organisations in the context of the new pandemic accord (Action for Animal Health Organisation, 2022; Wildlife Conservation Society, 2022). Additionally, to promote equitable multi-sectoral collaboration and assessment, it is essential to strengthen incentives, such as making it a prerequisite to secure funding for projects related to complex, intersectional problems. This strategy could position NFPs as a central element in the development of driver-centric, multi-hazard and multi-sectoral warning systems that are adapted to the individual threats faced by each country (Vlieg et al., Reference Vlieg, Fanoy, Van Asten, Liu, Yang, Pilot, Bijkerk, Van Der Hoek, Krafft, Van Der Sande and Liu2017).
Finally, community-based and bottom-up initiatives must be further developed in the context of pandemic prevention. Currently, most warning systems work in a top-down manner, led by international and national organisations, and only involve communities as system users (Mwatondo et al., Reference Mwatondo, Rahman-Shepherd, Hollmann, Chiossi, Maina, Kurup, Hassan, Coates, Khan, Spencer, Mutono, Thumbi, Muturi, Mutunga, Arruda, Akhbari, Ettehad, Ntoumi, Scott, Nel, Ellis-Iversen, Sönksen, Onyango, Ismail, Simachew, Wolking, Kazwala, Sijali, Bett, Heymann, Kock, Zumla and Dar2023). In contrast to international organisations, which tend to be divided into sectors and are prone to working in silos, local communities experience problems and their context first-hand and are not discipline-bound. Empowering them in the decision-making process and design of warning systems is crucial to ensure warning systems are context-based and adapted to the distinct vulnerabilities and drivers leading to the emergence of infectious diseases in different populations and world regions.
Limitations
While this study incorporated numerous local and community-based infectious-disease warning systems into the database, the proportion of such systems identified at the local level was significantly smaller compared to the national or international level. This discrepancy could be attributed to the informal character of these systems, which might not be as prominently featured in literature, potentially resulting in a less representative sample. A systematic review of community-based Early Warning Systems (EWS) found that the majority of the documented systems focused on natural hazards such as floods, droughts and landslides (Macherera and Chimbari, Reference Macherera and Chimbari2016). Conversely, community-based early warning systems targeting human diseases were found to be relatively scarce, even though similar systems operate at national, regional and global levels (p. 1). This observation could suggest that community-based EWS for infectious diseases are indeed less prevalent than those for other hazards, which could explain the observed gap in research.
Moreover, language barriers could also have impeded the recognition of certain warning systems from different countries. Only papers in English, Spanish and French were examined, which could impact how representative the sample is. Is important to stress that this research doesn’t present a systematic review of all infectious-disease warning systems but aims to highlight overall trends, gaps and opportunities. It should serve as a starting point for further evaluations rather than an endpoint. Furthermore, due to the broad scope of this study, the specific differences among disease drivers and the necessary adaptations for warnings according to these variations were not examined. This aspect warrants additional research for a more specific understanding. In addition, the categorisation of warning and response systems and the information presented is derived from publicly available sources. Consequently, there may be inaccuracies in the categorisation of certain warning systems if there have been changes to them that have not been publicly disclosed.
Finally, although this paper underscores the role of emerging infectious-disease drivers and the need for prevention-centred warnings, it is necessary to recognise that in many settings there is still not yet the capacity or resources to routinely collect information on human disease cases, let alone gathering data on animal diseases, especially in wildlife. Consequently, there still remains a necessity to further refine and develop “reactive” warning and response systems.
Conclusion
Despite the impact of socio-economic, ecological and environmental factors in driving EIDs risk, these factors are largely excluded from warning, surveillance and response systems. Of 64 warning systems examined in this study, 80% are “reactive.” They can only signal a warning and response once the disease reaches a detectable threshold in the population. Currently, warning systems are predominantly used as a method of adaptation, reducing the impact of existing risks rather than addressing them directly.
Nevertheless, there is potential for change and efforts to develop driver-centric surveillance systems that monitor and address EIDs drivers appear to be increasing. Indeed, 6% of warning and response systems within the database were “prevention-centred,” they both monitored and were linked to strategies to address disease drivers and prevent new or re-emerging infectious-disease spill-overs.
A key element that explains the disconnect between research and warning systems for EIDs is the lack of multi-sectoral collaboration in strategies to respond to these diseases. Current governance systems, both within and between international organisations, as well as in national governments, tend to divide responsibilities across departments and sectors. EIDs are perceived as a problem that falls within the responsibility of the public health sector. As a result, most warning systems are managed by human health specialists, not veterinarians and especially not ecologists. This shapes how the problem is conceptualised and how warning systems are designed, and subsequently, the responses issued.
Moreover, while more interdisciplinary efforts such as One Health are being developed, they largely continue to rely on an anthropocentric perspective which reproduces the same limitations of systems acting within disciplinary silos. Engagement between organisations and sectors needs to strive to be equitable rather than hierarchical to ensure that the interdependencies between human, animal and environmental sectors are not simplified, leading to unintended consequences (e.g., Avian Flu, Egypt).
The study highlights several overarching recommendations to facilitate cross-sectoral governance for EIDs, and transition from a reactive to a preventative approach. These include addressing silos across different international agreements such as the UN Early Warnings for All Initiative and the Pandemic Accord and developing shared multi-sectoral objectives within international agreements as exemplified by the 2022 Global Biodiversity Framework. This study also urges the development of One Health national focal points to enable multi-sectoral collaboration at the national level. Finally, it advocates for the development of bottom-up initiatives and community-based warning systems. Further research is needed to explore how to develop multi-sectoral, multi-hazard warning systems that account for the risks, cascading impacts and feedback loops between sectors and hazards.
We must take advantage of the momentum for change created by COVID-19 to switch from a responsive to a preventative approach that does not merely wait and prepares itself for the next pandemic but addresses the systemic, multi-sectoral issues that are making us more likely to experience another one in the first place.
Supplementary material
The supplementary material for this article can be found at https://doi.org/10.1017/one.2023.10
Comments
No accompanying comment.