1 Introduction
How well can adolescents predict significant life events? Reference Bruine de Bruin, Parker and FischhoffBruine de Bruin, Parker, and Fischhoff (2007) explored this question using data from the National Longitudinal Survey of Youth 1997 (Bureau of Labor Statistics, 1998), finding that teens’ judgments of their probability of experiencing various events (e.g., being arrested) correlated significantly with actual experiences of the events, reported one or more years later. These findings are consistent with research showing that, contrary to conventional wisdom, adolescents tend to perceive the dangers involved with risky behaviors but are also influenced by a range of other factors such as peers and the temptations of short-term benefits (see review in Reference Reyna and FarleyReyna & Farley, 2006).
The present study extended these analyses by comparing the predictive validity of teens’ self assessments of risk to a natural benchmark—known risk factors for each outcome. If teens’ self estimates of risk out-predict more traditional risk factors, then self estimates should be used to replace or augment known risk factors in identifying at risk youth. On the other hand, if teens cannot predict events as well as even a tiny subset of self-reported information about themselves, such a finding would cast doubt on their understanding of the situations and of behaviors underlying various risks.
2 Method
2.1 Participants and data
The National Longitudinal Survey of Youth 1997 (NLSY97) followed a large, representative cohort of youth in the U.S. (Bureau of Labor Statistics, 1998). Respondents were identified using a stratified multistage area probability sample and took part in hour-long computer-assisted personal interviews. Topics focused on schooling, employment, financial characteristics, family background, social behavior, and health status. In the first wave of data collection in 1997, 3436 adolescents (mean age 15.8 years, SD = .70) were asked a series of questions of the type, “What is the percent chance that you will […] in the next year?” The second wave in 1998 assessed the occurrence of the events. Here, data were examined for three outcomes: becoming pregnant (females only), being arrested, whether rightly or wrongly, at least once, and being a student in a regular school. These outcomes were chosen because of their social importance, and because the NLSY97 elicited relevant risk factor information.
2.2 Risk factors
Four traditional risk factors were identified for each outcome. (See Table 1 for simple descriptions and the Appendix for verbatim measures.) These were chosen based on previous research and guidelines for identifying at risk youth (Wisconsin Statutes § 118.153 (2012); Office of Juvenile Justice and Delinquency Prevention, 2000) and analyses in Reference Fischhoff, Parker, Bruine de Bruin, Downs, Palmgren, Dawes and ManskiFischhoff et al. (2000). The risk factors consisted largely of past behaviors and experiences. A measure of criminal history was created by adding the number of illicit behaviors the adolescent reported ever engaging in, including gang membership, destroying property, stealing money or property valued at less than $50, stealing money or property valued at greater than $50, committing other property crimes, attacking others, and selling drugs. These were combined into a simple tally (0-7; number of crimes self-reported), as this has been shown to be an effective forecasting technique in other domains (Reference Armstrong and CuzanArmstrong & Cuzan, 2006). Other risk factors included perceived peer norms—e.g., the estimated percent of peers who have had sex—and one community level variable—presence or absence of gangs in the respondent’s neighborhood or school.
Table 1: Correlations of variables assessed in 1997 with outcomes one year later.
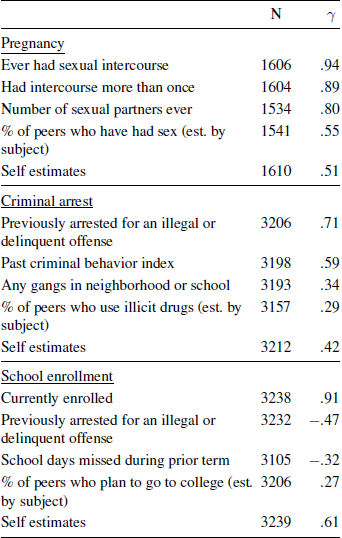
2.3 Analyses
Predictive validity was evaluated in three ways. First, self estimates and risk factors were correlated with each outcome. As in Bruine de Bruin et al. (2007), the nonparametric Goodman-Kruskal gamma correlation was used because of the skewed distribution of the outcome data and large numbers of ties (Reference Siegel and CastellanSiegel & Castellan, 1988).
Next, for each outcome, the risk-factor information was aggregated in order to make a statistical estimate of each respondent’s risk level. Since the optimal weighting of risk factors is typically unknown beforehand, the variables were standardized and unit weights were applied (Reference Dana and DawesDana & Dawes, 2005). Receiver operating characteristic (ROC) curves were then created based on (a) self estimates of risk alone, (b) risk factor information alone, and (c) a combination of self estimates and risk factors. ROC curves depict the hit rate and false positive rate based on all possible cutoff values of the predictors, thus providing a comprehensive measure of the predictive efficiency of each type of information. The area under each curve (AUC) was computed as a summary measure (Reference Hanley and McNeilHanley & McNeil, 1983). AUC represents the probability that a randomly selected adolescent who experienced the outcome (e.g., got pregnant) would score higher on the predictor variable or formula than a randomly selected adolescent who did not experience the outcome.
Last, binary logistic regressions were used to predict each outcome based on traditional risk factors alone, self estimates alone, and a combination of traditional risk factors and self estimates. This analysis revealed how well each outcome could be predicted in the best-case scenario in which optimal weights for predictors were estimated from the data.
3 Results
3.1 Correlations
Table 1 shows that, for each outcome, there was at least one traditional risk factor that exhibited a very strong correlation (>.70) with the outcome. These tended to be past behaviors with clear, intuitive links to the outcome, such as having had sex previously (pregnancy), having been arrested previously (arrest), and not being currently enrolled in school (school enrollment). In no case were self estimates the strongest predictor of behavior. Relative to the traditional risk factors, the predictive strength of self estimates was greatest for school enrollment and lowest for pregnancy.
3.2 ROC analysis
ROC curves are shown in Figure 1. The unit weighted risk factor information significantly outperformed self estimates of risk for predicting pregnancy (AUC = .87 vs. .65, z = 7.90, p < .0001), criminal arrest (AUC = .75 vs. .67, z = 3.50, p = .0005), and school enrollment (AUC = .71 vs. .63, z = 4.95, p < .0001). One reason for the comparatively poor performance of self estimates of risk was the large number of responses indicative of perceived invulnerability. For example, 63% of the teens who were not enrolled in school had, one year earlier, indicated a 0% chance of this occurring. Thus, any classification scheme based solely on self estimates of risk would fail to identify at least 63% of the adolescents who later left school. This issue also arose for predicting pregnancy and criminal arrest: 53% of those who became pregnant and 30% of those who were arrested had previously estimated a 0% chance of these events happening, thus capping the potential true positive rates at 47% and 70%, respectively.
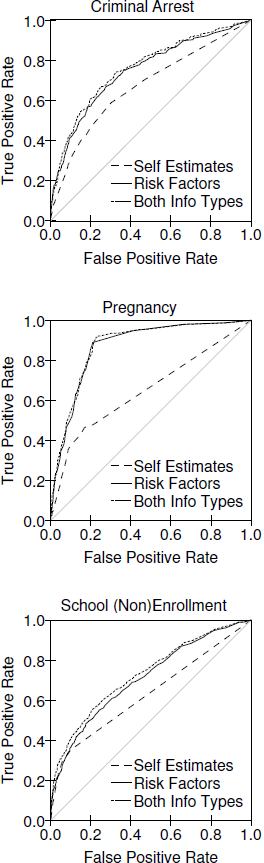
Figure 1: ROC curves comparing the predictive accuracy of self estimates of risk, traditional risk factors, and a combination of both information types.
Adding teens’ self estimates to the risk factor information tended to produce small or no gains in predictive accuracy. AUC increased from .75 to .76 for predicting criminal arrest (z = 2.45, p = .01) and from .71 to .73 for predicting school enrollment (z = 5.07, p < .0001). AUC remained at .87 for predicting pregnancy (z = 0.65, p = .52).
3.3 Binary logistic regressions
Results of the logistic regressions were similar to those of the ROC analyses. Traditional risk factors predicted future pregnancy as expected, with having had sex previously (OR = 18.90, p < .001), number of previous sex partners (OR = 1.05, p = .03), and estimated percent of peers who have had sex (OR = 1.40, p < .001) predicting pregnancy. Footnote 1 Self estimates of likelihood of becoming pregnant predicted future pregnancy in a separate regression (OR = 1.03, p < .001). Cox and Snell R-squared was .19 for the model with traditional risk factors alone and .04 for the model with self estimates alone. Cox and Snell R-squared remained at .19 after adding self estimates to the model with traditional risk factors.
Predictors of future criminal arrest included previously being arrested (OR = 2.77, p < .001), higher scores on the prior criminal behavior index (OR = 1.46, p < .001), and reporting gangs in one’s neighborhood or school (OR = 1.31, p = .07). Estimated percent of peers who use illicit drugs was directional but did not approach significance (OR = 1.08, p = .19). Self estimates predicted future criminal arrest in a separate regression (OR = 1.03, p < .001). The model with traditional risk factors alone produced a Cox and Snell R-squared of .08, while the model with self estimates alone gave a Cox and Snell R-squared of .03. Cox and Snell R-squared remained at .08 when self estimates were added to the model with traditional risk factors.
Results for predicting future school enrollment were similar. Current enrollment (OR = 17.39, p < .001), fewer days absent during the prior term (OR = .95, p < .001), higher estimated percent of peers planning to go to college (OR = 1.24, p < .001), and having not been arrested previously (OR = 0.55, p < .001) predicted future enrollment. In a separate regression, self estimates also predicted future enrollment (OR = 1.03, p < .001). Cox and Snell R-squared was .13 for the model with traditional risk factors alone and .07 for the model with self estimates alone. Adding self estimates to the model with traditional risk factors produced an increase in the Cox and Snell R-squared from .13 to .15.
4 Discussion
Recent studies have begun to question the conventional view of adolescents as naïve about risk and failing to appreciate possible consequences of their actions (Reference Reyna and FarleyReyna & Farley, 2006). Using the same data as in previous investigations (Reference DawesFischhoff et al., 2000; Reference Bruine de Bruin, Parker and FischhoffBruine de Bruin et al., 2007), however, the present study found that a small subset of risk factors significantly out-predicted teens’ self estimates of risk, highlighting the limitations of adolescents’ appraisals of the behaviors and situations underlying risk. “Invulnerable” teens—those who gave risk estimates of 0%—accounted for a considerable portion of those who ultimately experienced each outcome. These findings may reflect poor choices on the part of those who see themselves as invulnerable (Downs, Bruine de Bruin, Murray, & Fischhoff, 2004; Reference Dillard, Midboe and KleinDillard, Midboe, & Klein, 2009) as well as a failure to appreciate and prepare for the effects of variables such as sexual arousal and social influences on behavior (Reference Ariely and LoewensteinAriely & Loewenstein, 2006; Reference Wolosin, Sherman and CannWolosin, Sherman, & Cann, 1975). More generally, risks arising from incorrect beliefs or knowledge deficits are unlikely to be reflected in people’s self assessments of risk (Reference Dunning, Johnson, Ehrlinger and KrugerDunning, Johnson, Ehrlinger, & Kruger, 2003).
These results suggest that, although adding teens’ self estimates of risk to traditional risk factor information may boost accuracy for certain outcomes, gains are likely to be marginal. Notably, this study looked at only a small subset of traditional risk factors for each outcome. In identifying youth at risk of not graduating, for example, schools not only consider information about current enrollment, truancy, and delinquency, but they also have data on grades, credits, and assessments of basic skills (e.g., Wis. Stat. § 118.153 (2012)). In predicting juvenile delinquency, the effects of a wide range of risk factors and protective factors have been quantified, including aspects of the individual, family, peer group, school, and community (Office of Juvenile Justice and Delinquency Prevention, 2000). Adding meaningfully to such thorough risk composites may be unlikely given the marginal value of self estimates in the present study.
The results reported here further substantiate the well-known axiom that past behavior is often the strongest predictor of future behavior, an effect that occurs because measures of past behavior capture the influences of a variety of factors such as habits, psychological dispositions, and attitudes, and because past behavior can contribute to the formation of intentions in situations fostering conscious deliberation (e.g., Reference Ouellette and WoodOuellette & Wood, 1998; Reference TriandisTriandis, 1977). The results also extend the previous finding that risk factors tend to out-predict the intuitive judgments of psychologists, parole boards, loan officers, and others tasked with assessing risk (e.g., Reference Grove and MeehlGrove & Meehl, 1996). As Reference DawesDawes (1994, p. 105) put it:
In a majority of situations, an individual’s past behavior is the best predictor of future behavior. That doesn’t mean that people are incapable of changing. Certainly, many of us do, often profoundly. What it does mean is that no one has yet devised a method for determining who will change, or how or when. Professional psychologists cannot predict that.
The present results suggest that we, ourselves, also fall short in predicting such change, at least as adolescents. The findings highlight the potential for growth in teens’ understanding of themselves, their knowledge of the situations that lead to negative outcomes, and the skills and self-efficacy needed to achieve the types of lives they expect to live. While, as a group, adolescents engaged in risky activities may recognize their heightened risk of negative outcomes (e.g., HIV risk for those who engage in high-risk sex; Reference Murphy, Rotheram-Borus and ReidMurphy, Rotheram-Borus, & Reid, 1998), perceived invulnerability was present in a substantial proportion of at-risk youth in the present study, and individual self estimates were not sufficiently calibrated to out-predict traditional risk factors.
Appendix: Verbatim measures of predictors and outcomes.
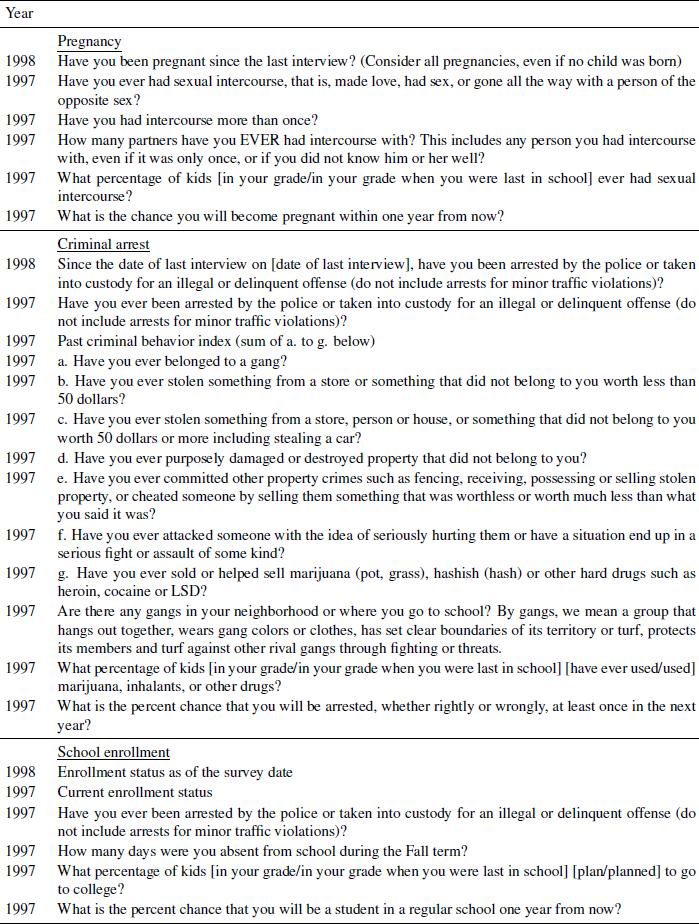