Introduction
Diminished reward functions and reduced interest in pleasurable activities are core characteristics of major depressive disorder (MDD) (Cao et al., Reference Cao, Park, Subramaniapillai, Lee, Iacobucci, Mansur and McIntyre2019; Rømer Thomsen, Whybrow, & Kringelbach, Reference Rømer Thomsen, Whybrow and Kringelbach2015). Understanding the altered brain reward functions in MDD patients is crucial for elucidating the neural mechanisms of reward functional impairments in MDD. Importantly, MDD is characterized by deficits in multiple stages of reward functions, including reward anticipation, reward processing, and reward learning (Pizzagalli, Reference Pizzagalli2022). Decreased reward function is considered as the core manifestation of anhedonia, which is a hallmark symptom of MDD (Pizzagalli, Reference Pizzagalli2022). Anhedonia is also conceptually associated with negative affective symptoms such as apathy, as both implicate reduction of reward functions (Husain & Roiser, Reference Husain and Roiser2018). However, while apathy often refers more specifically to reduced reward-based motivation, anhedonia can encompass broader aspects of reward function deficits including anticipation, processing, and learning (Pizzagalli, Reference Pizzagalli2022).
Reward anticipation refers to the emotional and cognitive processes associated with the expectation of rewards (Rømer Thomsen et al., Reference Rømer Thomsen, Whybrow and Kringelbach2015), which are associated with brain activations in the striatum, thalamus, insula, prefrontal cortex (PFC), and occipital cortex (Oldham et al., Reference Oldham, Murawski, Fornito, Youssef, Yücel and Lorenzetti2018). However, past findings on the changes in reward anticipation activities in MDD patients were inconsistent. Two recent meta-analyses examined the differences in MDD patients compared to healthy controls (HCs) on reward anticipation activities (Keren et al., Reference Keren, O'Callaghan, Vidal-Ribas, Buzzell, Brotman, Leibenluft and Stringaris2018; Yang et al., Reference Yang, Su, Yang, Song, Yan, Luo and Zeng2022). While one study only found reduced right caudate activities in MDD patients (Keren et al., Reference Keren, O'Callaghan, Vidal-Ribas, Buzzell, Brotman, Leibenluft and Stringaris2018), the other study showed that MDD patients displayed increased activities in the lateral and medial PFC, but decreased striatal and limbic activities (Yang et al., Reference Yang, Su, Yang, Song, Yan, Luo and Zeng2022). Notably, the first meta-analysis included participants of all ages as well as both MDD patients and ‘at-risk’ individuals (Keren et al., Reference Keren, O'Callaghan, Vidal-Ribas, Buzzell, Brotman, Leibenluft and Stringaris2018), while the second meta-analysis was restricted to results using the monetary incentive delay (MID) task (Yang et al., Reference Yang, Su, Yang, Song, Yan, Luo and Zeng2022). Given the well-known change in reward function among the older population (Shao et al., Reference Shao, Gao, Lin, Huang, Liu, Toh and Lee2022), combining the results of adult and older patients may result in heterogenous and inaccurate findings. Also, MDD-related changes in reward functions as assessed with tasks other than the MID remained to be elucidated.
Reward processing refers to the experience of pleasure derived from consuming rewards (Rømer Thomsen et al., Reference Rømer Thomsen, Whybrow and Kringelbach2015). Significant brain activations in the ventral striatum, amygdala, and orbitofrontal cortex (OFC)/ventromedial prefrontal cortex (VMPFC) have been reported during reward processing in healthy adults (Oldham et al., Reference Oldham, Murawski, Fornito, Youssef, Yücel and Lorenzetti2018). One previous meta-analysis reported reduced brain activities in the caudate nucleus during reward processing in MDD patients compared to HCs (Keren et al., Reference Keren, O'Callaghan, Vidal-Ribas, Buzzell, Brotman, Leibenluft and Stringaris2018). However, a recent meta-analysis showed that MDD patients exhibited higher activities in the right temporal cortex, and lower activities in the striatum (including the caudate), thalamus, and lateral PFC compared to HCs during reward processing (Yang et al., Reference Yang, Su, Yang, Song, Yan, Luo and Zeng2022). While blunted caudate activities in MDD patients were consistently observed, reduced activities in other brain regions such as the PFC were not always found, which could be due to methodological limitations of those two meta-analyses as described above.
Reward learning refers to the utilization of prediction error (PE) signals (Rømer Thomsen et al., Reference Rømer Thomsen, Whybrow and Kringelbach2015), which reflect the discrepancy between expected and actual outcomes, to guide future decisions based on past rewards (Schultz, Dayan, & Montague, Reference Schultz, Dayan and Montague1997). Human PE signals have been mainly found in the midbrain, striatum, thalamus, amygdala, and PFC (Corlett, Mollick, & Kober, Reference Corlett, Mollick and Kober2022). A recent meta-analysis did not find significant differences between MDD patients and HCs in brain signals associated with reinforcement learning, which could be due to the study simultaneously analyzing both reward and punishment PE signals (Yaple, Tolomeo, & Yu, Reference Yaple, Tolomeo and Yu2021).
It is important to note that different aspects of reward functions, including reward anticipation, reward processing, and learning, are essentially integrative in nature and closely associated with each other (Schultz, Reference Schultz2016; Zald & Treadway, Reference Zald and Treadway2017). Consistent with this, previous studies have shown that the striatum-thalamus circuitry is implicated across multiple reward functions. The striatum, which evaluates the value of outcomes and update knowledge on the conditional occurrence of stimuli (Cox & Witten, Reference Cox and Witten2019), was previously found to show lower activities during both reward processing (Pizzagalli et al., Reference Pizzagalli, Holmes, Dillon, Goetz, Birk, Bogdan and Fava2009) and learning (Kumar et al., Reference Kumar, Goer, Murray, Dillon, Beltzer, Cohen and Pizzagalli2018) in MDD patients compared to HCs. Also, the thalamus is involved in updating knowledge on the conditional occurrence of stimuli, and using this information to guide future beliefs and actions (Hill-Bowen, Flannery, & Poudel, Reference Hill-Bowen, Flannery and Poudel2020). This region also showed reduced activities in both reward anticipation (Smoski et al., Reference Smoski, Felder, Bizzell, Green, Ernst, Lynch and Dichter2009) and learning (Rothkirch, Tonn, Köhler, & Sterzer, Reference Rothkirch, Tonn, Köhler and Sterzer2017) in MDD patients compared to HCs. However, no meta-analysis has synthesized past findings across reward anticipation, reward processing, and learning functions to determine any overlapping neural substrate that shows abnormalities in MDD. Looking for such ‘common’ reward system would also allow pinpointing neural targets for intervention that ameliorates MDD-related deficits in multiple reward functions (e.g. Wang et al., Reference Wang, He, Chen, Shi, Yang, Geng and Yu2021).
Therefore, we conducted an up-to-date meta-analysis on brain activity differences between MDD patients and HCs, across reward anticipation, reward processing, and learning functions, through utilizing a method that incorporates both positive and negative findings from past studies. To address limitations of past meta-analyses, we comprehensively synthesized previous findings generated using a variety of task paradigms (e.g. MID, card guessing task, and probabilistic learning task), while taking into account potential confounding effects due to patient clinical (e.g. comorbidity) and demographic (e.g. age and sex) characteristics, as well as the task paradigm employed. Importantly, we tested whether certain regions showed activity changes in MDD patients compared to healthy individuals across multiple reward functions.
Methods and materials
This review was conducted following the Preferred Reporting Items for Systematic Reviews and Meta-Analyses (PRISMA) guidelines. The review protocol was pre-registered at PROSPERO, International prospective register of systematic reviews (Reference number: CRD42023426657). The PRISMA checklist can be found in online Supplementary Table S1.
Search strategy
A systematic literature search was conducted in four online databases including Pubmed, Embase, PsycINFO, and Web of Science. The initial search was completed on 23 January 2023, with the following keywords: ‘depress*’ AND (‘reward’ OR ‘motivation’ OR ‘reinforcement’ OR ‘prediction error’ OR ‘decision making’ OR ‘anhedonia’ OR ‘pleasure’) AND (‘functional magnetic resonance imaging’ OR ‘fMRI’). Only studies including adult participants were included (aged 18–65 years). Other inclusion criteria were: (1) the study included currently diagnosed MDD patients; (2) the study provided anatomical coordinates for group difference in neural activity, in either MNI or Talairach space.
The exclusion criteria were: (1) reviews, book chapters or meta-analysis; (2) the study included remitted rather than current MDD patients; (3) the study included participants aged <18 or >65 years; (4) the study only conducted ROI-based rather than whole-brain analyses; (5) the study did not provide results on between-group comparisons; (6) the study used social reward stimuli such as facial emotions, due to potential differences in social and non-social reward signals in the brain (Britton et al., Reference Britton, Phan, Taylor, Welsh, Berridge and Liberzon2006; Sankar et al., Reference Sankar, Yttredahl, Fourcade, Mickey, Love, Langenecker and Hsu2019); (7) studies with unavailable full texts and (8) non-English literature. Detailed inclusion/exclusion criteria are shown in online Supplementary Table S2.
The initial search generated 1978 articles. After removing duplicates, a total of 838 unique studies remained. Study selection and screening procedure followed the PRISMA guidelines (Fig. 1). Two reviewers (X.Z. and R.S.) independently screened the titles and abstracts during first-stage screening. Studies that met any of the exclusion criteria were screened out. The remaining 149 studies were passed to the second stage for full-text assessment. Any discrepancies in the screening results were resolved by discussion, and unresolved discrepancies were referred to a third reviewer (K.L.). Finally, 18 papers published between 2008 and 2022 were channeled to data extraction.
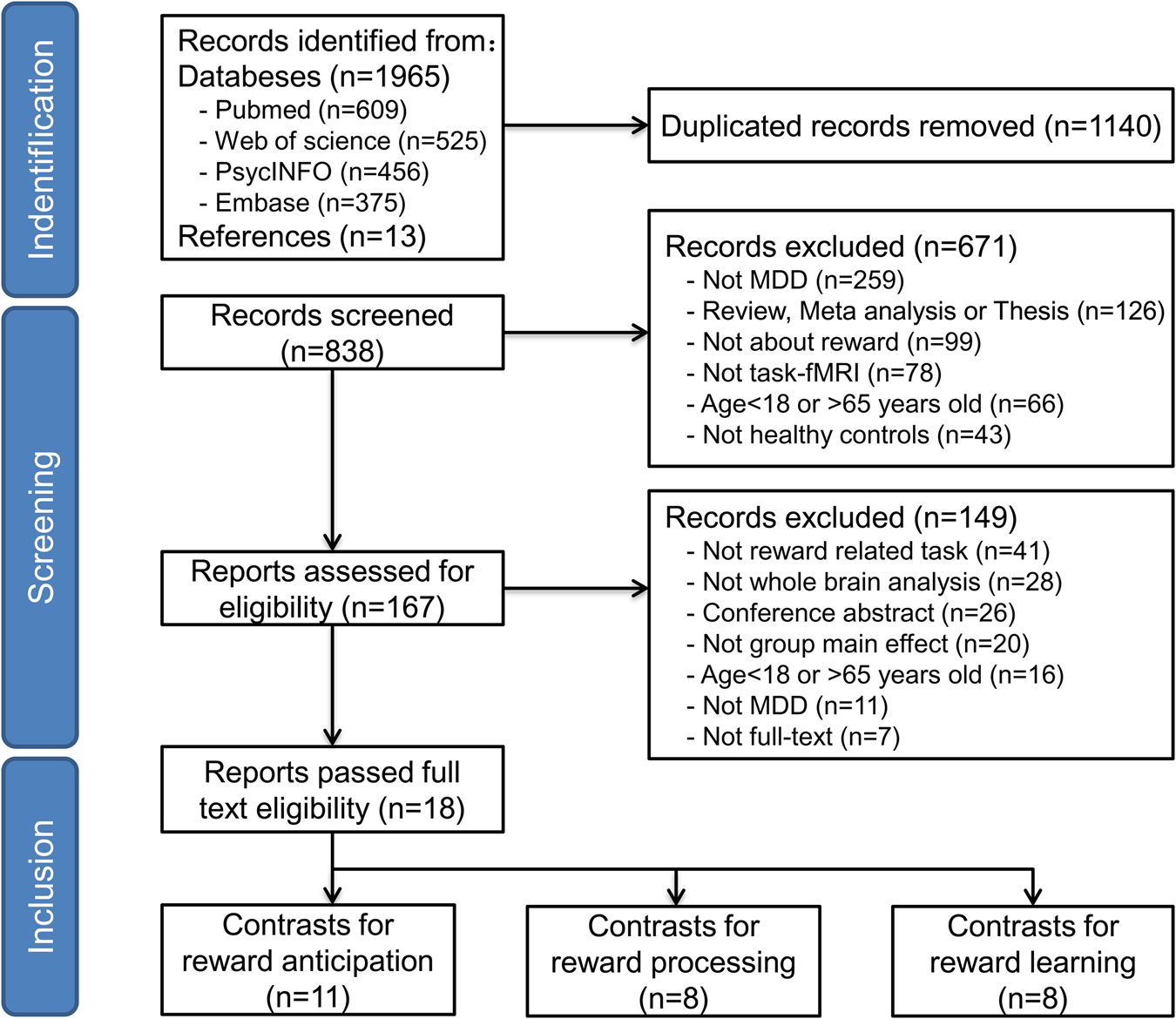
Figure 1. The PRISMA flowchart for literature searching and screening.
Data extraction and quality assessment
Data extraction was performed independently by two authors (X.Z. and R.S.). The following key information was extracted from each eligible article: author(s), year of publication, participant demographic characteristics (sample size, age, sex ratio), clinical characteristics (Hamilton Depression Scale (HAMD) and Beck Depression Inventory (BDI-II) scores, comorbidities, medication use), task paradigm, statistical threshold employed, and major findings including the peak coordinates of significant results.
To assess the quality of the included intervention studies, we evaluated the articles using the following criteria: completion of demographic information, methods of recruitment, task design, image acquisition and analysis procedures, and the overall consistency of the study's conclusions (Sanderson, Tatt, & Higgins, Reference Sanderson, Tatt and Higgins2007; Shepherd, Matheson, Laurens, Carr, & Green, Reference Shepherd, Matheson, Laurens, Carr and Green2012). The full assessment is included in online Supplementary Table S3.
Task fMRI meta-analysis method
We employed the signed differential mapping (SDM) method to perform task-based fMRI meta-analysis (https://www.sdmproject.com/). The SDM method has the advantages that it can synthesize both significant and nonsignificant fMRI results and quantifies each result using t statistics (Radua & Mataix-Cols, Reference Radua and Mataix-Cols2009).
Main voxel-based meta-analysis
In this study, we were primarily interested in analyzing the difference between MDD patients and HCs in three contrasts of interest, namely reward anticipation (anticipating rewarding stimuli – anticipating neutral stimuli), reward processing (processing rewarding outcome – processing neutral outcome) (one study (Segarra et al., Reference Segarra, Metastasio, Ziauddeen, Spencer, Reinders, Dudas and Murray2016) compared win outcome against ‘full-loss’ outcome in a simulated slot-machine paradigm.), and reward learning (brain signals correlating with the PE signals of rewarding stimuli). We used the SDM method to perform voxel-based fMRI meta-analysis using the following pipeline. First, for between-group difference in each contrast of interest, we extracted individual studies' significant peak coordinates, t/z values, p values, at the whole-brain level. Second, for each contrast of interest, we generated an effect-size brain map for each individual study, which integrated both positive and negative results. Using these single-study maps, we then computed the weighted mean, variance, and effect-size map through averaging across all studies using random-effects meta-analytic model, which were weighted by study sample sizes.
The study-average map was then statistically evaluated using default SDM kernel size and thresholds, including a FWHM of 20 mm, voxel-level p value of 0.005, peak-height |SDM-Z| value of 1, and a cluster extent of 10 voxels (Radua et al., Reference Radua, Mataix-Cols, Phillips, El-Hage, Kronhaus, Cardoner and Surguladze2012, Reference Radua, Rubia, Canales-Rodríguez, Pomarol-Clotet, Fusar-Poli and Mataix-Cols2014). The map significance was computed using permutation testing (50 times) as previously recommended (Radua et al., Reference Radua, Mataix-Cols, Phillips, El-Hage, Kronhaus, Cardoner and Surguladze2012). The null distribution was generated by extracting a random effect size from each included study for every voxel (Radua et al., Reference Radua, Mataix-Cols, Phillips, El-Hage, Kronhaus, Cardoner and Surguladze2012). The actual statistical map was then compared to the null distribution to identify voxels which exceeded the p < 0.005 threshold. According to the software developer, 50 times permutation would be sufficient to generate a stable null distribution, based on which statistical threshold of SDM-Z value was calculated. The above statistical threshold was determined to optimize the balance between sensitivity and specificity in the SDM method (Radua et al., Reference Radua, Mataix-Cols, Phillips, El-Hage, Kronhaus, Cardoner and Surguladze2012), which had been widely adopted (Alegria, Radua, & Rubia, Reference Alegria, Radua and Rubia2016; Fullana et al., Reference Fullana, Albajes-Eizagirre, Soriano-Mas, Vervliet, Cardoner, Benet and Harrison2018; Hart, Radua, Nakao, Mataix-Cols, & Rubia, Reference Hart, Radua, Nakao, Mataix-Cols and Rubia2013).
Reliability analysis
To evaluate the influence of individual studies on the estimated pooled effect size and to assess the overall reliability of the findings, a whole-brain jack-knife sensitivity analysis was conducted (Radua & Mataix-Cols, Reference Radua and Mataix-Cols2009). This analysis involved iteratively removing each study from the meta-analysis and examining the resulting effect size maps, to identify effects that consistently emerged.
Subgroup meta-analysis
To account for potential sample and methodological differences among the studies, subgroup meta-analyses were conducted for each contrast of interest (reward anticipation, reward processing, and learning). In each subgroup analysis, we assessed the extent to which the main analysis results overlapped with the subgroup results.
For the contrasts of reward anticipation and reward processing, the majority of studies (8/11 and 5/8 respectively) employed the MID task. Thus, subgroup analysis was conducted for all studies that employed the MID paradigm. For the contrast of reward learning, the task paradigm employed by previous studies was diverse, but the majority of studies (6/8) used monetary reward stimuli. Thus, subgroup analysis was conducted for all studies which used monetary rewards.
Moreover, for each contrast of interest, subgroup analyses were performed for studies that included subjects with comorbid generalized anxiety disorder (GAD).
Meta-regression analysis
Finally, meta-regression analyses were performed to assess the impact of between-study heterogeneity on group differences in the contrasts of interest. We assessed the moderating effect of mean patients' illness severity, as indexed by their average BDI scores (for studies reporting HAMD scores, those were converted to BDI scores according to (Furukawa et al., Reference Furukawa, Reijnders, Kishimoto, Sakata, DeRubeis, Dimidjian and Cuijpers2020)), on group differences in brain signals. We also assessed the moderating effects of patients' mean age and female ratio (results are included in the online Supplementary Results).
In order to assess the direct correlation between patients' anhedonia levels and group difference (MDD v. HC group) in functional activities, we additionally performed meta-regression analysis among studies which reported patients' average anhedonia scores. Due to the small number of studies in this case, we only performed this analysis for reward learning (study N = 5), but not reward anticipation or reward processing (both study N = 3).
Overlapping analysis
We additionally examined overlapping brain regions which showed significantly different brain activities between the MDD and HC groups across multiple contrasts of interest (reward anticipation, reward processing, and reward learning). Similar to the main analyses, we also assessed the moderating effect of patient symptom severity on between-group activity differences.
Software and toolboxes
We used the Seed-based d mapping (SDM) toolbox (v.5.15) to conduct the meta-analysis (Radua et al., Reference Radua, Mataix-Cols, Phillips, El-Hage, Kronhaus, Cardoner and Surguladze2012, Reference Radua, Rubia, Canales-Rodríguez, Pomarol-Clotet, Fusar-Poli and Mataix-Cols2014). Labeling of anatomical locations of the results was performed using the xjview toolbox (v.10.0, https://www.alivelearn.net/xjview), which was implemented in Matlab R2022a (Mathworks). Statistical analysis on the demographic variables and correlation analyses were conducted using SPSS (v.26, IBM Corp.). Figures were produced using MRIcron v1.0.20190902 (https://www.nitrc.org/projects/mricron) and Microsoft Office 2016 Powerpoint.
Results
Study and sample characteristics
A total of 18 studies were included in the meta-analysis, including 11 studies on reward anticipation, 8 studies on reward processing, and 8 studies on reward learning. All studies included MDD patients on medication. Detailed study characteristics for each contrast of interest (reward anticipation, reward processing, and reward learning) are included in online Supplementary Results. The studies' quality assessment scores ranged between 10.5 and 14 (out of 15), with an average score of 12.6, suggesting medium-to-high quality (Table 1).
Table 1. Demographic and clinical characteristics of individual studies included in the meta-analysis
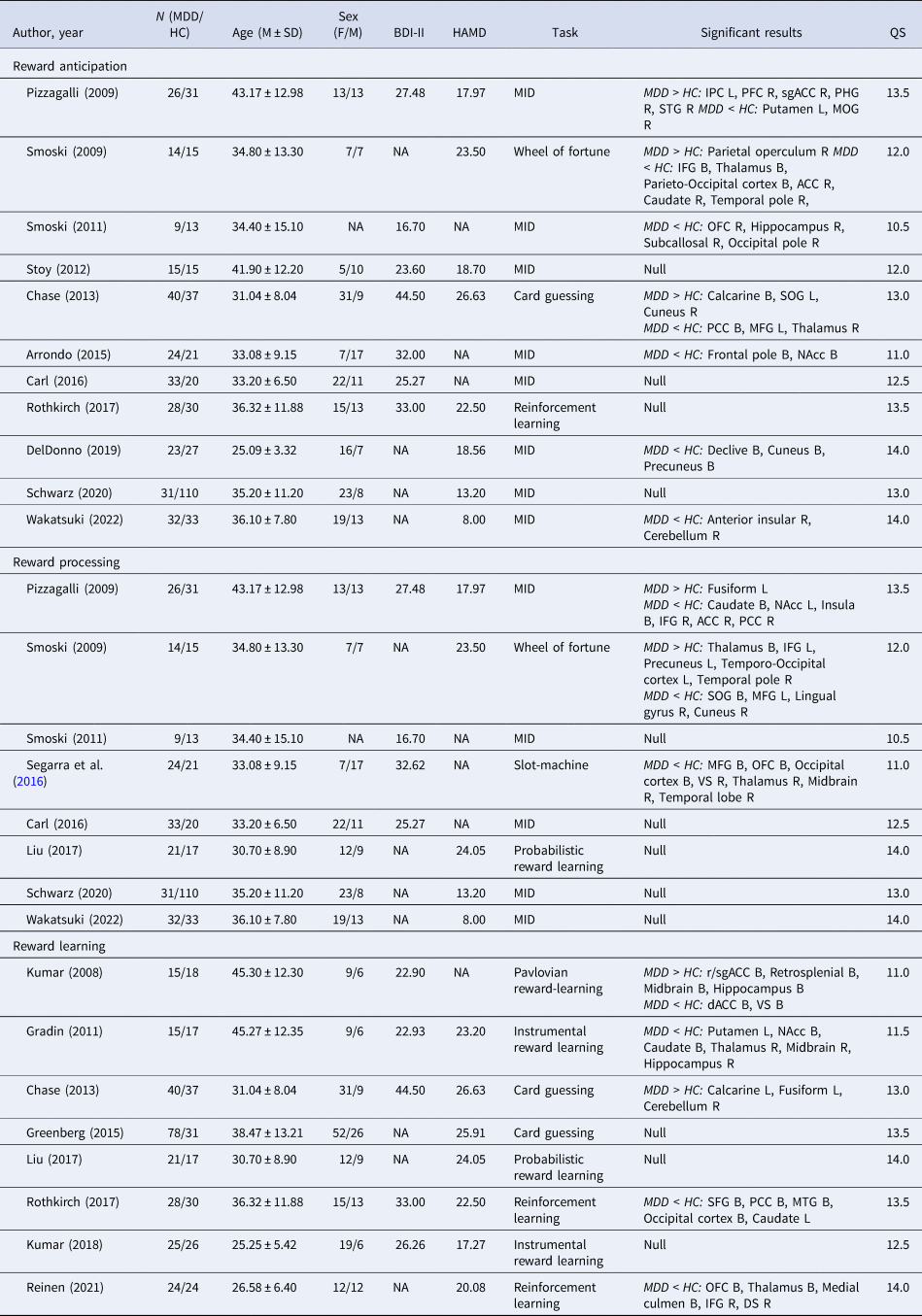
QS, Quality Assessment Score; MID, Monetary Incentive Delay; IPC, Inferior Parietal Cortex; PFC, Prefrontal Cortex; sgACC, subgenual Anterior Cingulate Cortex; PHG, Parahippocampal Gyrus; STG, Superior Temporal Gyrus; MOG, Middle Occipital Gyrus; IFG, Inferior Frontal Gyrus; ACC, Anterior Cingulate Cortex; OFC, Orbitofrontal Cortex; SOG, Superior Occipital Gyrus; PCC, Posterior Cingulate Cortex; MFG, Middle Frontal Gyrus; NAcc, Neuclus Accumbens; VS, Ventral Striatum; r/sgACC, rostal/subgenual Anterior Cingulate Cortex; dACC, dorsal Anterior Cingulate Cortex; SFG, Superior Frontal Gyrus; MTG, Middle Temporal Gyrus; DS Dorsal Striatum; B, Bilateral; L, Left; R, Right.
Meta-analysis of group difference in reward anticipation activities
Main meta-analysis
Compared to HCs, MDD patients exhibited higher activities in the right cuneus when anticipating rewards compared to neutral stimuli. Conversely, MDD patients showed significantly lower activities in the right occipital cortex, thalamus, orbitofrontal (OFC) and ventrolateral prefrontal cortex (VLPFC), and in the left middle frontal gyrus (MFG), and middle occipital gyrus (MOG), during reward anticipation. Further details are presented in Table 2 and Fig. 2a.
Table 2. Main meta-analysis of brain activities differences between MDD and HCs
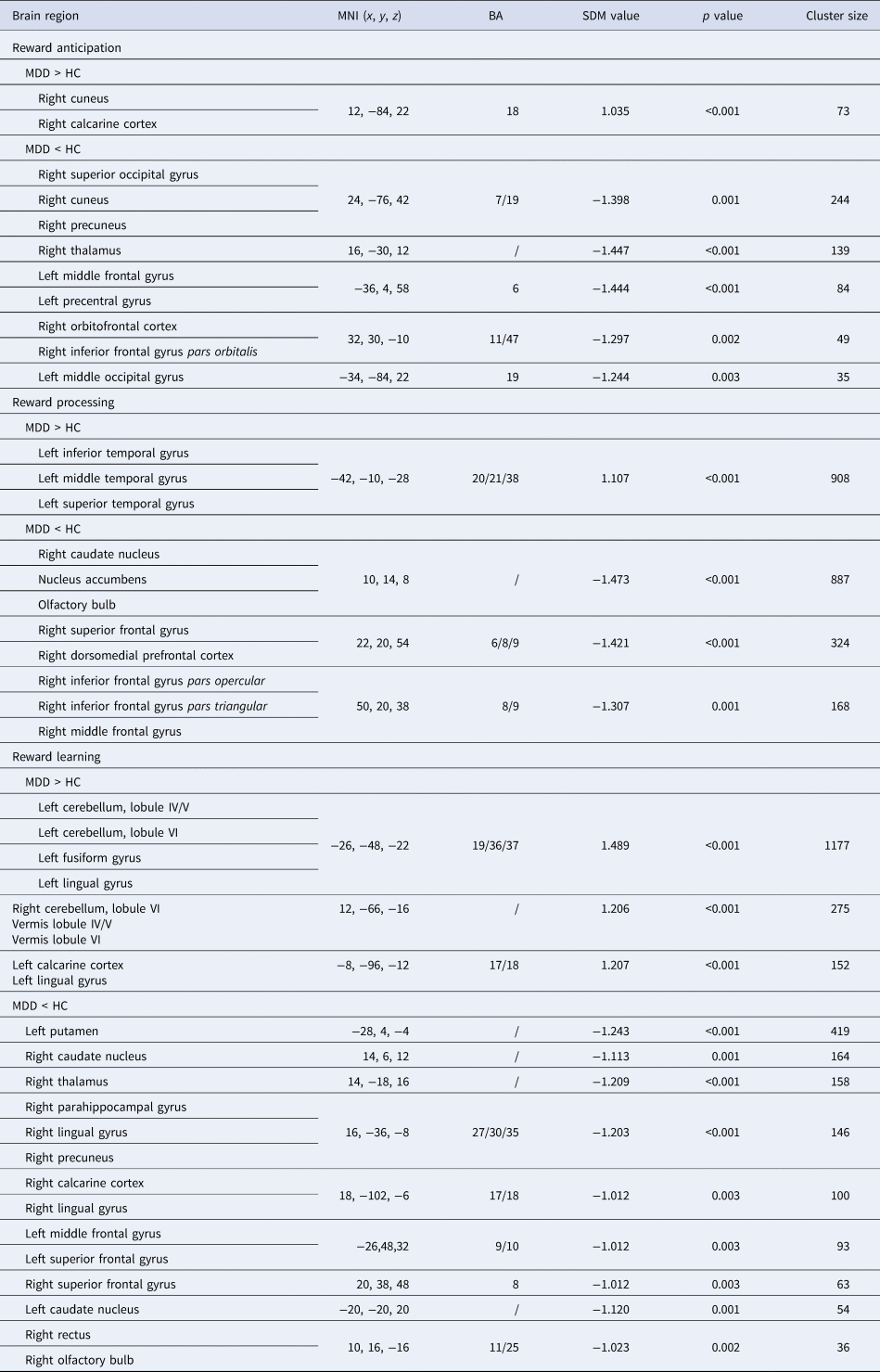
MDD, Major Depressive Disorder; HC, Healthy Controls; MNI, Montreal Neurological Institute; SDM, Seed-based d Mapping; BA, Brodmann's Area.
Note: Clusters were identified at voxel-wise p < 0.005, |SDM-Z| > 1, and cluster size > 10 voxels.
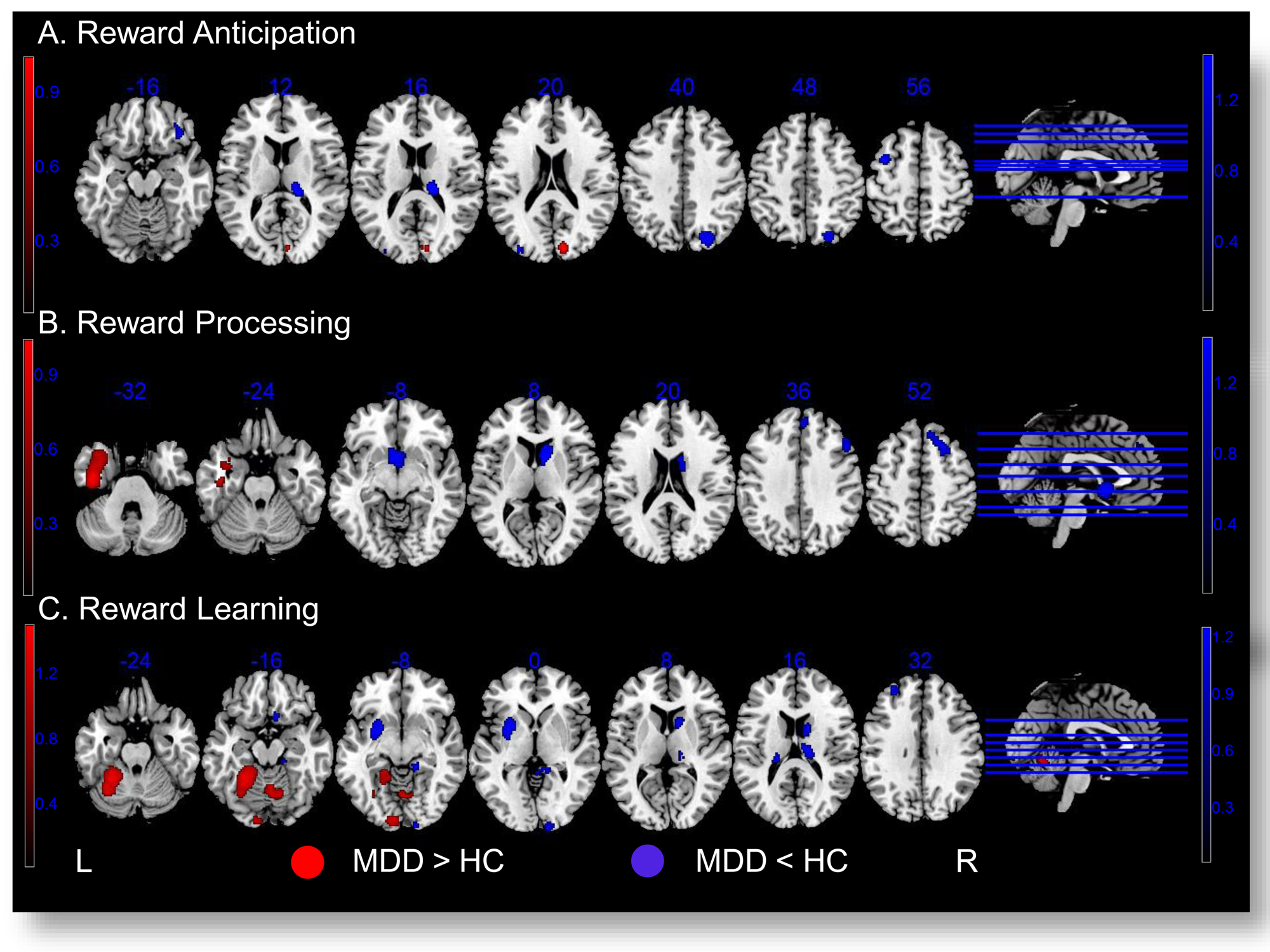
Figure 2. Activity differences between MDD and HCs in three contrasts of interest: reward anticipation (a), reward processing (b), reward learning (c). Brain regions showing higher activities in MDD patients compared to HCs are displayed in Red . Brain regions with lower activities in MDD patients compared to HCs are displayed in Blue
.
Jack-knife sensitivity analysis
The higher activities in the right cuneus and lower activities in the left MFG and right thalamus, which were observed in MDD patients compared to HCs, were consistently found in all but one combination of studies. Additionally, the lower activities observed in MDD v. HCs in the left MOG, right OFC, and right occipital cortex remained significant in all but two combinations (online Supplementary Table S4).
Subgroup meta-analysis
In the first subgroup analysis, which included 8 studies using the MID paradigm, the higher cuneus activity in the MDD v. HC group was not replicated. The lower activity in the right OFC/VLPFC among MDD patients v. HCs was replicated (100% of 49 voxels in the cluster). However, the reduced activities in the right occipital cortex, thalamus, and in the left MFG and MOG among MDD patients were not replicated (online Supplementary Table S5 and Supplementary Fig. S1a).
In the second subgroup analysis comprising 6 studies with patients comorbid with GAD, the higher activities in the right cuneus (95.89% of the 73 voxels in the main results) and the lower activities in the right OFC/VLPFC (10.20% of the 49 voxels in the main results) among MDD patients were partly replicated. However, the reduced activities in the right occipital cortex, thalamus, and in the left MFG and MOG among MDD patients were not replicated (online Supplementary Table S5 and Supplementary Fig. S1b).
Meta-regression analysis
Studies including MDD patients with higher illness severity reported larger increase in right cuneus activity (MNI coordinates: x = 12, y = −84, z = 22; SDM-Z = 1.826; p = 0.003; 18 voxels), and larger decrease in left MFG (MNI coordinates: x = −36, y = 6, z = 58; SDM-Z = −2.338; p < 0.001; 611 voxels) and right thalamus (MNI coordinates: x = 18, y = −28, z = 12; SDM-Z = −2.702; p < 0.001; 354 voxels) activities, in MDD patients compared to HCs.
Meta-analysis of group difference in reward processing activities
Main meta-analysis
For reward processing, MDD patients exhibited higher activities in the left temporal cortex, and lower activities in the right caudate nucleus including nucleus accumbens (NAcc), and the lateral prefrontal cortex (LPFC) along with the dorsomedial prefrontal cortex (DMPFC). Further details are presented in Table 2 and Fig. 2b.
Jack-knife sensitivity analysis
The higher activities in the left temporal cortex and lower activities in the right caudate nucleus/NAcc, which were observed in MDD v. HC group, were consistently observed in all but one combination of studies. Additionally, the reduced activities in MDD v. HCs in the right LPFC/DMPFC remained significant in all but two combinations (online Supplementary Table S6).
Subgroup meta-analysis
In the first subgroup analysis, which included five studies using the MID paradigm, the higher activities in the left temporal cortex (49.78% of 908 voxels in the main results), and the lower activities in the right caudate nucleus/NAcc (10.15% of 887 voxels in the main results), and in the right LPFC/DMPFC (18.50% of 492 voxels in the main results) among MDD patients compared to HCs were partly replicated (online Supplementary Table S7 and Supplementary Fig. S2a).
In the second subgroup analysis, which included five studies with subjects comorbid with GAD, the higher activities in the left temporal cortex (49.67% of 908 voxels in the main results), and the lower activities in the right caudate nucleus/NAcc (10.15% of 887 voxels in the main results), and in the right LPFC/DMPFC (18.50% of 492 voxels in the main results) among MDD patients compared to HCs were partly replicated (online Supplementary Table S7 and Supplementary Fig. S2b).
Meta-regression analysis
Studies including MDD patients with higher illness severity reported larger decrease of right IFG activity (MNI coordinates: x = 50, y = 28, z = 38; SDM-Z = −1.207; p < 0.001; 97 voxels), in MDD patients v. HCs.
Meta-analysis of group difference in reward learning activities
Main meta-analysis
For reward learning, MDD patients displayed higher activities in the bilateral cerebellum, the left fusiform gyrus, and calcarine cortex compared to HCs. MDD patients showed significantly lower activities than HCs in the left putamen, MFG, the bilateral caudate nucleus, superior frontal gyrus (SFG), and the right thalamus, parahippocampal/lingual gyrus, calcarine cortex, and rectus. Further details are presented in Table 2 and Fig. 2c.
Jack-knife sensitivity analysis
The higher activities in the bilateral cerebellum, the left fusiform gyrus and calcarine cortex, as well as the lower activities in the right thalamus, in the MDD v. HC group were consistently observed in all but one combination of studies. Other brain regions remained significant in all but two or three combinations (online Supplementary Table S8).
Subgroup meta-analysis
In the first subgroup analysis, which included six studies using monetary rewarding stimuli, the higher activities among MDD patients v. HCs in the left cerebellum along with fusiform gyrus (25.15% of 1177 voxels in the main results), the right cerebellum (68.36% of 275 voxels in the main results), and the left calcarine cortex (100% of 152 voxels in the main results) were replicated. The lower activities in the right thalamus (37.97% of 158 voxels in the main results) and the right parahippocampal/lingual gyrus (28.08% of 146 voxels in the main results) among MDD patients v. HCs were also replicated. However, the lower activities among MDD patients in the left putamen, MFG, the bilateral caudate nucleus, SFG, the right calcarine cortex, and rectus were not replicated (online Supplementary Table S9 and Supplementary Fig. S3a).
In the second subgroup analysis, which included five studies with subjects comorbid with GAD, the higher activities among MDD patients v. HCs in the left cerebellum along with fusiform gyrus (27.27% of 1177 voxels in the main results), the right cerebellum (64% of 275 voxels in the main results), and the left calcarine cortex (98.68% of 152 voxels in the main results) were replicated. The lower activities in the right thalamus (65.19% of 158 voxels in the main results) and the right parahippocampal/lingual gyrus (54.79% of 146 voxels in the main results) among MDD patients v. HCs were also replicated. However, the lower activities among MDD patients in the left putamen, MFG, the bilateral caudate nucleus, SFG, the right calcarine cortex, and rectus were not replicated (online Supplementary Table S9 and Supplementary Fig. S3b).
Meta-regression analysis
Studies including MDD patients with more severe illness reported larger increase in right cerebellum (MNI coordinates: x = 14, y = −60, z = −12; SDM-Z = 1.431; p = 0.001; 395 voxels) and left calcarine cortex (MNI coordinates: x = −10, y = −94, z = −12; SDM-Z = 1.411; p = 0.001; 67 voxels) activities, in MDD patients v. HCs.
Also, studies including MDD patients with higher anhedonia levels reported larger decrease in left putamen activity during reward learning (MNI coordinates: x = −24, y = 6, z = −8; SDM-Z = −1.109; p < 0.001; 337 voxels), in MDD patients v. HCs.
Overlapping analysis across reward anticipation, reward processing and learning
Across the three contrasts of interest, no overlapping region was observed for the MDD>HC difference (Fig. 3a). Conversely, for the MDD < HC difference, the right thalamus (78 voxels) was significant in both reward anticipation and reward learning, while the right caudate nucleus (162 voxels) was significant in both reward processing and reward learning (Fig. 3b).
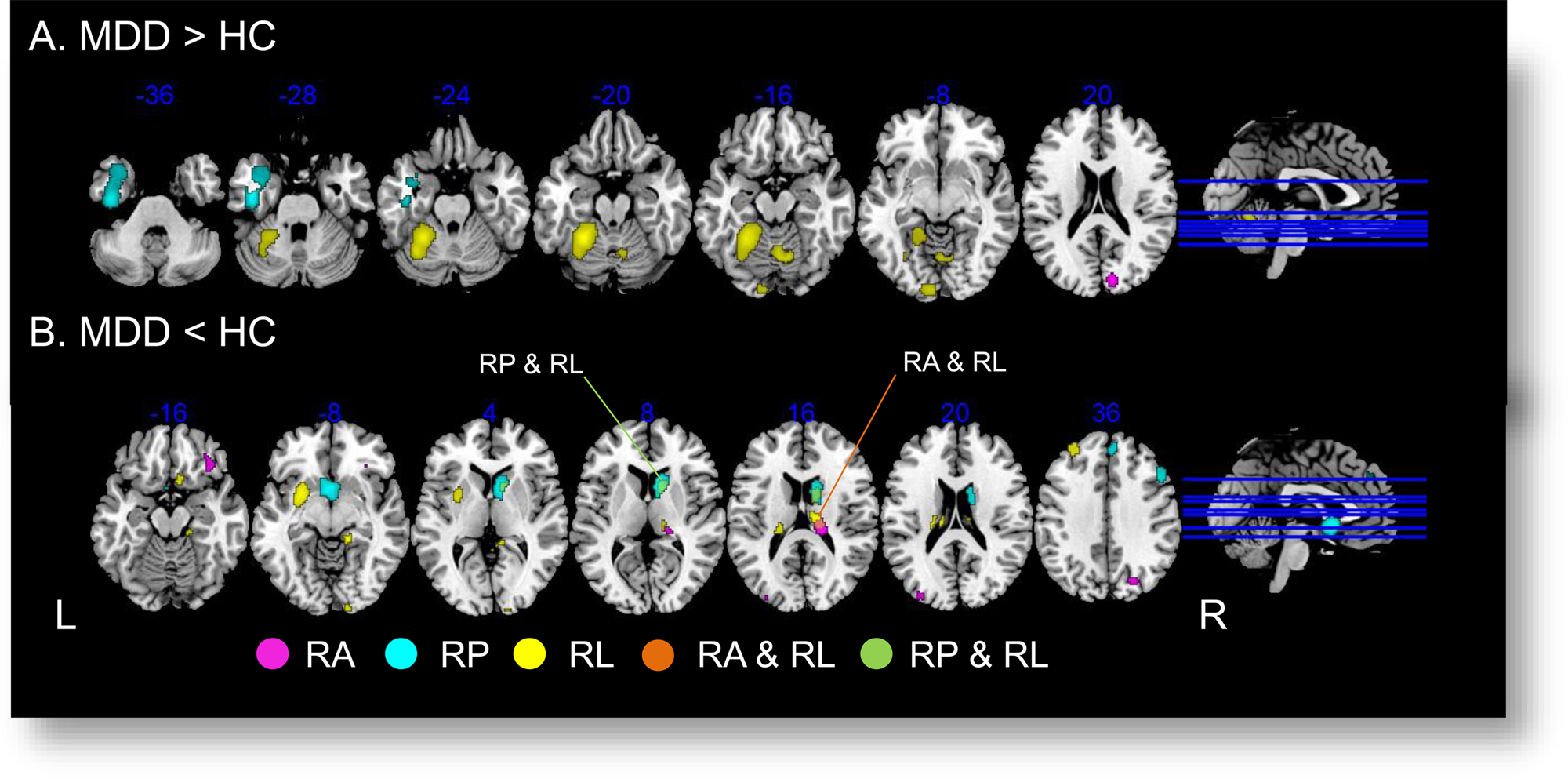
Figure 3. Overlapping analysis across contrasts of interest. Regions showing significant between-group difference in reward anticipation are displayed in Violet . Regions showing significant between-group difference in reward processing are displayed in Cyan
. Regions showing significant between-group difference in reward learning are displayed in Yellow
. RA, reward anticipation; RP, reward processing; RL, reward learning. (a) For MDD > HC, no overlapping brain regions across the three contrasts of interest were found. (b) For MDD < HC, two overlapped brain regions were found: the right thalamus showed group difference in both reward anticipation and learning (Orange
, slice z = 16, 78 voxels); and the right caudate showing group difference in both reward processing and learning (Green
, slice z = 8, 162 voxels).
Exploratory correlation analysis
We extracted the z value of the above right thalamus and caudate nucleus regions, and explored between-study Spearman's correlations of their mean z values and patients' mean symptom severity. While no correlation was found between group difference (MDD v. HC group) in caudate activity and patients' BDI score (|ρ| ⩽ 0.378, p > 0.35), group difference in right thalamus activity during reward anticipation correlated negatively with patients' BDI score (ρ = −0.661, p = 0.027).
Discussion
In this meta-analysis, we found various prefrontal, striatal, occipital, and limbic regions which showed differential activities in reward anticipation, reward processing and learning between MDD patients and HCs. The findings showed high consistency across task paradigm, reward nature, and patient comorbidity. Larger functional changes in MDD were observed among patients with more severe symptoms and higher anhedonia levels. Importantly, the findings highlighted lower activity in the right thalamus during both reward anticipation and learning, and lower activity in the right caudate during both reward processing and learning, in MDD patients relative to HCs.
MDD v. HCs in reward anticipation signals
Compared to HCs, MDD patients displayed lower activities in the lateral prefrontal-thalamus circuitry, which performs integral functions in high-level affective processing of stimuli (Phillips, Kambi, Redinbaugh, Mohanta, & Saalmann, Reference Phillips, Kambi, Redinbaugh, Mohanta and Saalmann2021). Given the basic function of the thalamus in relaying sensory and affective information about incoming stimuli to the PFC, the lower activity in the right thalamus may indicate deficits in information transfer when MDD patients were processing stimuli that signal upcoming rewards (Disner, Beevers, Haigh, & Beck, Reference Disner, Beevers, Haigh and Beck2011). In relation to that, the lateral PFC has long been recognized to play important roles in the evaluation of reward-signaling stimuli, and in incorporating this information into decision-making process (Zoh, Chang, & Crockett, Reference Zoh, Chang and Crockett2022). Therefore, reduced lateral PFC activity in MDD patients during reward anticipation is consistent with past observations that these individuals show altered reward-based decision-making (Der-Avakian & Markou, Reference Der-Avakian and Markou2012). Moreover, we found that studies which included MDD patients with more severe symptoms reported larger decrease among patients in lateral prefrontal-thalamus activities, which added further evidence that functional abnormality of this circuitry is closely linked to worsening of depressive features.
Conversely, MDD patients showed increased primary visual cortex activity in the right cuneus, which was opposite to the reduced activity in more secondary visual cortices observed in MDD patients. These may suggest enhanced early-stage visual processing of affective stimuli in MDD patients, at the cost of reduced later-stage sensory and affective processing (Chen et al., Reference Chen, Liu, Zhang, Zhang, Xue, Lin and Deng2019; Desseilles et al., Reference Desseilles, Balteau, Sterpenich, Dang-Vu, Darsaud, Vandewalle and Schwartz2009). Moreover, studies with more severe MDD patients reported a larger increase in right cuneus activities among patients compared to HCs, which further indicated that elevated primary visual processing of affective stimuli is linked to worsening of depressive characteristics.
Furthermore, the lower activity in the right OFC/VLPFC among MDD patients was replicated when only studies using the MID task were considered, and when only MDD patients comorbid with GAD were included. These suggest that the deficits of MDD patients in brain circuitries involved in reward anticipation represented a generic functional abnormality that is relatively context-independent. Also, the reduced OFC/VLPFC function seemed an intrinsic feature of MDD regardless of whether the patients were comorbid with other affective conditions.
MDD v. HCs in reward processing signals
During reward processing, MDD patients showed lower activity in the right caudate, NAcc, LPFC, and DMPFC, which might contribute to their difficulties in appraising the hedonic value of rewards. Also, studies including more severe MDD patients reported a larger reduction of right IFG activities among patients relative to HCs. The caudate and NAcc are critical components of the medial striatal circuitries which perform essential reward value and motivation functions (Haber & Knutson, Reference Haber and Knutson2010). Reduced activity levels in these regions may be closely associated with the anhedonia symptoms of MDD (Pizzagalli et al., Reference Pizzagalli, Holmes, Dillon, Goetz, Birk, Bogdan and Fava2009; Wacker, Dillon, & Pizzagalli, Reference Wacker, Dillon and Pizzagalli2009). Related to this, the LPFC and DMPFC are key components of the prefrontal-striatal circuitry that is essential for processing and regulation of positive affectivity (Haber & Knutson, Reference Haber and Knutson2010). Reduced activities in these regions may lead to decreased hedonic experience and impaired maintenance of positive emotions. In contrast, MDD patients showed higher activity in the left temporal cortex than HCs. Given the important roles of the left temporal cortex in language and semantic knowledge, it might be that MDD patients tended to rely on linguistic and semantic processing of rewards, rather than the more ‘affective’ and automatic processing of rewarding information in the striatum (Gjelsvik, Lovric, & Williams, Reference Gjelsvik, Lovric and Williams2018), which could be closely associated with the reward deficits in MDD individuals (Buckner, Joiner, Pettit, Lewinsohn, & Schmidt, Reference Buckner, Joiner, Pettit, Lewinsohn and Schmidt2008).
Moreover, all the above findings were replicated when including only studies using the MID paradigm, or only studies including MDD patients comorbid with GAD. These suggested that the MDD patients' reduced right prefrontal-striatal circuitry activity, but increased left temporal activity, during consummatory reward processing represent a stable feature of MDD which was relatively independent of context and patient' clinical characteristics.
MDD v. HCs in reward learning signals
During reward learning, MDD patients showed higher activity in the bilateral cerebellum and the left visual cortex, but reduced activity in the lateral prefrontal-thalamic-striatal circuitry along with the right parahippocampal gyrus and visual cortex. Extensive evidence indicates the essential role of the lateral prefrontal-thalamic-striatal circuitry in learning about rewards. Within this circuitry, the striatum is considered to carry out conditional learning between rewards and the associated stimuli and responses (Delgado, Reference Delgado2007). The lateral PFC is considered to perform more sophisticated learning processes, such as learning based on extended reinforcement histories (Maia & Frank, Reference Maia and Frank2011) and reversal learning (Hornak et al., Reference Hornak, O'Doherty, Bramham, Rolls, Morris, Bullock and Polkey2004), as well as incorporating reward learning with executive control processes in order to achieve goals (Buschman & Miller, Reference Buschman and Miller2014). The thalamus may be mainly involved in relaying information between the lateral PFC and the striatum, and in binding of sensory and affective features of stimuli (Disner et al., Reference Disner, Beevers, Haigh and Beck2011). Thus, the reduced activity throughout the lateral prefrontal–thalamic–striatal circuitry among MDD patients highlights these individuals' pronounced functional abnormalities in reward learning. This in turn may contribute to the deficits in motivation and instrumental actions commonly observed in MDD (Der-Avakian & Markou, Reference Der-Avakian and Markou2012). On the other hand, the right parahippocampal–occipital circuitry may be involved in encoding and retrieving memories about the visual information of rewarding stimuli (Tsukiura & Cabeza, Reference Tsukiura and Cabeza2008). Reduced activity in this circuitry may indicate decreased learning about the sensory properties of rewards, which in turn leads to less positive memories commonly observed in MDD (Dillon & Pizzagalli, Reference Dillon and Pizzagalli2018).
In contrast, MDD patients showed increased left occipital and bilateral cerebellar activities during reward learning relative to HCs. This result was also more pronounced in studies involving more severe patients. The increased left occipital activity might indicate a bias towards learning about the primitive visual information about rewards, rather than about higher-level visual and affective properties of positive stimuli that tend to involve the right occipital regions (Lang et al., Reference Lang, Bradley, Fitzsimmons, Cuthbert, Scott, Moulder and Nangia1998). The increased cerebellar activities during reward learning were observed within the lobules IV, V, and VI which have been associated with sensorimotor and cognitive functions (Stoodley, Valera, & Schmahmann, Reference Stoodley, Valera and Schmahmann2012). This may suggest that MDD is characterized by a tendency towards sensorimotor and cognitive learning, rather than affective learning about the stimulus's reward value.
The higher activities in the bilateral cerebellum and left visual cortex, along with the lower activity in the right thalamic-striatal-lateral PFC circuitry, were observed when using only studies employing monetary reward stimuli or studies including MDD patients comorbid with GAD. These suggest that MDD patients' impaired functioning of the reward learning and memory circuitries represent a stable feature that is relatively independent of reward nature and patient' clinical characteristics.
We additionally showed that studies including patients with higher anhedonia levels reported a larger decrease in left putamen activities among patients relative to HCs during reward learning. This preliminary result provided direct evidence supporting the critical importance of striatal functional impairment in relation to anhedonia symptoms of MDD.
MDD v. HCs in brain activity across multiple reward functions
We observed the right caudate nucleus and the right thalamus showed decreased activities in MDD patients relative to HCs across different reward functions. These findings highlight the key role of the right thalamic-striatal circuitry across multiple reward functions, while deficient functioning of this circuitry can be a core brain mechanism of MDD. Specifically, the right thalamus activity was decreased during both reward anticipation and learning in MDD patients. Previous research indicates that the thalamus performs the important role of binding and transmitting affective and sensory information to the striatum (Disner et al., Reference Disner, Beevers, Haigh and Beck2011). Hence, our findings suggest that decreased information transfer within the thalamic-striatal circuitry would negatively impact learning about reward occurrences and probabilities and utilizing the learned information to guide future reward predictions and instrumental actions.
We additionally observed that the right caudate activity was decreased during both reward processing and learning in MDD patients. It is well known that the caudate is a key substrate for instrumental learning about rewards (Tricomi, Delgado, & Fiez, Reference Tricomi, Delgado and Fiez2004). Past studies consistently reported that the caudate was activated while individuals were processing and learning about histories of reward occurrences and omissions, and when preparing instrumental actions upon receiving reward-related cues (Cox & Witten, Reference Cox and Witten2019). Our findings thus provided strong support for the key role of the right caudate in processing and learning about reward delivery, and in utilizing this information to plan for future actions.
Comparison with past meta-analyses
For reward anticipation, two past meta-analyses both showed reduced striatal (caudate nucleus) activity in MDD patients (Keren et al., Reference Keren, O'Callaghan, Vidal-Ribas, Buzzell, Brotman, Leibenluft and Stringaris2018; Yang et al., Reference Yang, Su, Yang, Song, Yan, Luo and Zeng2022), while our study showed activity decrease among MDD patients primarily in the right thalamus and lateral PFC. Methodological differences such as participant age (including older patients) may account for the difference in findings, given that thalamic-striatal function exhibits known alterations during aging (Fama & Sullivan, Reference Fama and Sullivan2015). For reward processing, two past meta-analyses showed MDD-related activity decreases in the caudate nucleus and lateral PFC (Keren et al., Reference Keren, O'Callaghan, Vidal-Ribas, Buzzell, Brotman, Leibenluft and Stringaris2018; Yang et al., Reference Yang, Su, Yang, Song, Yan, Luo and Zeng2022), which were consistent with our findings. For reward learning, one past meta-analysis failed to find significant changes in brain activity among MDD patients (Yaple et al., Reference Yaple, Tolomeo and Yu2021). This was likely to result from not separating reward and punishment learning signals, which may involve different neural substrates (Robinson, Cools, Carlisi, Sahakian, & Drevets, Reference Robinson, Cools, Carlisi, Sahakian and Drevets2012).
Comparison with apathy in schizophrenia
Anhedonia and apathy are closely associated with each other (Husain & Roiser, Reference Husain and Roiser2018). Schizophrenia and related psychotic disorders are known to be characterized by negative symptoms such as apathy. Consistent with this, past studies also showed that apathy in schizophrenia is associated with reduced functional levels of the caudate nucleus and thalamus (Bègue et al., Reference Bègue, Brakowski, Seifritz, Dagher, Tobler, Kirschner and Kaiser2022; Kos, Van Tol, Marsman, Knegtering, & Aleman, Reference Kos, Van Tol, Marsman, Knegtering and Aleman2016). It should be noted that several previous studies revealed thalamus and caudate hypoactivity in schizophrenia during performing cognitive tasks (Bègue et al., Reference Bègue, Brakowski, Seifritz, Dagher, Tobler, Kirschner and Kaiser2022; Liemburg et al., Reference Liemburg, Dlabac-De Lange, Bais, Knegtering, Van Osch, Renken and Aleman2015). It remains to be established whether functional reduction in the thalamic-caudate circuitry contributes more to cognitive impairment in schizophrenia which is characterized principally by symptoms in cognitive domains.
Limitation
Few existing studies on brain reward functions in MDD utilized a longitudinal design, meaning that our findings were cross-sectional in nature and could not establish direction of causality, which can be addressed by future longitudinal empirical and meta-analysis research. Also, none of the existing studies included homogenous sample of first-episode, medication-naïve patients, thus the potential confounding effect of medication could not be ruled out. Future research should attempt to involve patients who have not received medication treatment.
Conclusion
This meta-analysis demonstrated that MDD patients show reduced functioning of the thalamic-striatal circuitry across reward anticipation, reward processing, and learning. The findings provided support for deficient thalamic-striatal circuitry functioning and the resulted in comprehensive reward functional impairment in MDD.
Supplementary material
The supplementary material for this article can be found at https://doi.org/10.1017/S0033291724001235.
Author contributions
X.Z., S.W., X.L., Z.L., W.L., K.L., and R.S. designed the study. X.Z., K.L., and R.S. performed data identification, screening, and inclusion. X.Z., and R.S. analyzed data and interpreted findings. X.Z., S.W., X.L., Z.L, and R.S. drafted the manuscript. All authors reviewed and evaluated the manuscript. All authors contributed significantly to the intellectual contents of the manuscript.
Funding statement
This project was supported by grants from the National Natural Science Foundation of China (82171531, 81671347), the Science and Technology Program of Guangzhou, China (202007030012), and the Guangzhou Municipal Key Discipline in Medicine (2021–2023) to K.L., and grant from the National Natural Science Foundation of China (82371558) to RS.
Competing interests
The authors have no competing interest to declare.